State-of-the-art: A taxonomy of artificial intelligence-assisted robotics for medical therapies and applications
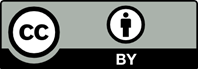
This paper presents a review on the development and major advances in artificial intelligence-assisted robotics for medical therapeutic tasks by focusing on the current challenges emerging from the clinical application process and the research efforts mitigating the problems. In this review, we searched Nature, Science, and Cell using specific keywords (i.e., medical artificial intelligent robots), categorized research works over the past three decades based on therapeutic applications, and discuss the latest development and bottleneck problems of each subtopic. We first present a chronology of the artificial intelligence-assisted techniques developed for medical therapeutic tasks over the past three decades and classify them according to the principles of the algorithm and its corresponding type of medical therapeutic tasks. Artificial intelligence technologies have evolved from classic machine learning methods in the early nineties to data-driven deep learning methods. We subsequently derive a taxonomy of artificial intelligence-assisted therapeutic tasks in the past three decades based on the types of therapeutic tasks and the trending topics in relation to the problems. Using certain search criteria with Nature and Cell databases, one prosperous trend has been abstracted from highly cited research papers and the interpretation of our taxonomy. This unprecedented trend embodies the revolutionary development of artificial intelligence, a closer integration with therapeutic tasks, and a more comprehensive human-robot interaction, all of which benefit sophisticated telesurgery and microsurgery by providing surgeons with higher imaging accuracy and human-like tactile sensation. Our survey discusses the current challenges and future trends of artificial intelligence-assisted therapeutic tasks for the convenience of clinical research and applications, hoping that they would help bridge the gap between entrepreneurial translation and research.
Tottori S, Zhang L, Qiu F, et al., 2012, Magnetic helical micromachines: Fabrication, controlled swimming, and cargo transport. Adv Mater, 24: 811–816. https://doi.org/10.1002/adma.201103818
Huang TY, Qiu F, Tung HW, et al. 2014, Generating mobile fluidic traps for selective three-dimensional transport of microobjects. Appl Phys Lett, 105: 114102. https://doi.org/10.1063/1.4895937
Swangnetr M, Kaber DB. 2013, Emotional state classification in patient–robot interaction using wavelet analysis and statistics-based feature selection. IEEE Trans Hum Mach Syst, 43: 63–75. https://doi.org/10.1109/tsmca.2012.2210408
Menciassi A, Melzer A, Dumont E, et al., 2014, Robotics and Machine Learning Approaches to Improve robustness of USgFUS: Futura. J Ther Ultrasound, 2: A25–A25. https://doi.org/10.1186/2050-5736-2-s1-a25
Svensson CM, Krusekopf S, Lücke J, et al., 2014, Automated Detection of Circulating Tumor Cells with Naive Bayesian Classifiers. Cytometry A, 85: 501–511. https://doi.org/10.1002/cyto.a.22471
Lyndon D, Kumar A, Kim J, et al., 2015, Convolutional Neural Networks for Medical Clustering. In: CLEF.
Romero J, Diago LA, Shinoda J, et al., 2015, Evaluation of Brain Models to Control a Robotic Origami arm Using Holographic Neural Networks. https://doi.org/10.1115/detc2015-48074
Kondo T, Ueno J, Takao S. 2015, Medical Image Diagnosis of Liver Cancer by Hybrid Feedback GMDH-type Neural Network Using Principal Component-regression Analysis. Artif Life Robot, 20: 145–151. https://doi.org/10.1007/s10015-015-0213-1
Kondo T, Ueno J, Takao S. 2015, Logistic GMDH-type Neural Network Using Principal Component-regression Analysis and its Application to Medical Image Diagnosis of Lung Cancer. Artif Life Robot, 20: 137–144. https://doi.org/10.1007/s10015-015-0200-6
Garza-Burgos M, Sanchez-Orozco E, Bayro-Corrochano E. 2016, Medical Robot Vision Using the Conformai Geometric Algebra Framework. In: 2016 IEEE-RAS 16th International Conference on Humanoid Robots (Humanoids). p1087– 1093. https://doi.org/10.1109/humanoids.2016.7803406
Nokata N, Kato S, Feng LK, et al., 2016, Measurement of Mechanical Characteristics for Soft Materials by Using Medical Robot with Piezoelectric Tactile Sensors. In: 2016 International Symposium on Micro-NanoMechatronics and Human Science (MHS). Piscataway: IEEE. p1–4. https://doi.org/10.1109/mhs.2016.7824164
Nguyen PB, Park JO, Park S, et al., 2016, Medical Micro-robot Navigation Using Image Processing-blood Vessel Extraction and X-ray Calibration. In: 2016 6th IEEE International Conference on Biomedical Robotics and Biomechatronics (BioRob). Piscataway: IEEE. p365–370. https://doi.org/10.1109/biorob.2016.7523653
Miyashita S, Guitron S, Yoshida K, et al. Ingestible, Controllable, and Degradable Origami Robot for Patching Stomach Wounds. In: 2016 IEEE International Conference on Robotics and Automation (ICRA). Piscataway: IEEE. p909–916. https://doi.org/10.1109/icra.2016.7487222
Jiang G, Luo M, Bai K, et al., 2017, A Precise Positioning Method for a Puncture Robot Based on a Pso-optimized bp Neural Network Algorithm. Appl Sci, 7: 969. https://doi.org/10.3390/app7100969
Sarikaya D, Corso JJ, Guru KA. 2017, Detection and Localization of Robotic Tools in Robot-assisted Surgery Videos Using Deep Neural Networks for Region Proposal and deTection. IEEE Trans Med Imaging, 36: 1542–1549. https://doi.org/10.1109/tmi.2017.2665671
Tang L, Qian J, Li L, et al., 2017, Multimodal Medical Image Fusion Based on Discrete Tchebichef Moments and Pulse Coupled Neural Network. Int J Imaging Syst Technol, 27: 57–65. https://doi.org/10.1002/ima.22210
Jayanthi PR, Bommannaraja K, Manju R. 2017, Early Detection of Macular Edema in Fundus Image Datasets Using Neural Network.
Namozov A, Cho YI. 2018, Convolutional Neural Network Algorithm with Parameterized Activation Function for Melanoma Classification. In: 2018 International Conference on Information and Communication Technology Convergence (ICTC). p417–419. https://doi.org/10.1109/ictc.2018.8539451
Qamar S, Jin H, Zheng R, et al., 2018, 3d Hyper-dense Connected Convolutional Neural Network for Brain Tumor Segmentation. In: 2018 14th International Conference on Semantics, Knowledge and Grids (SKG). p123–130. https://doi.org/10.1109/skg.2018.00024
Lu Y, Yu Q, Gao Y, et al., 2018, Identification of Metastatic Lymph Nodes in MR Imaging with Faster Region-Based Convolutional Neural Networks. Cancer Res, 78: 5135–5143. https://doi.org/10.1158/0008-5472.can-18-0494
Alom MZ, Yakopcic C, Taha T, et al., 2018, Nuclei Segmentation with Recurrent Residual Convolutional Neural Networks based U-Net (R2U-Net). In: NAECON 2018- IEEE National Aerospace and Electronics Conference. p228-233. https://doi.org/10.1109/naecon.2018.8556686
Sim Y, Chung MJ, Kotter E, et al., 2019. Deep Convolutional Neural Network-based Software Improves Radiologist Detection of Malignant Lung Nodules on Chest Radiographs. Radiology, 294: 182465. https://doi.org/10.1148/radiol.2019182465
Pratt H, Coenen F, Harding SP, et al., 2019, Feature Visualisation of Classification of Diabetic Retinopathy Using a Convolutional Neural Network. In: CEUR Workshop Proceedings. p23–29.
Parakh A, Lee H, Lee JH, et al., 2019, Urinary Stone Detection on ct Images Using Deep Convolutional Neural Networks: Evaluation of Model Performance and Generalization. Radiology. Artif Intell, 1: e180066. https://doi.org/10.1148/ryai.2019180066
Yin C, Qian B, Wei J, et al., 2019, Automatic Generation Of Medical Imaging Diagnostic Report with Hierarchical Recurrent Neural Network. In: 2019 IEEE International Conference on Data Mining (ICDM). p728–737. https://doi.org/10.1109/icdm.2019.00083
Zhang K, Zhou X, Wu J. 2019, U-module: Better Parameters Initialization of Convolutional Neural Network for Medical Image Classification. In: 2019 IEEE International Conference on Image Processing (ICIP). p799–803. https://doi.org/10.1109/icip.2019.8803799
Lee H, Jang M, Kim HC, et al., 2019, Association of Imaging Factors Derived from Convolutional Neural Network with Visual Outcomes in Age-related Macular degeneration and Polypoidal Choroidal Vasculopathy. Sci Rep, 9: 1–9. https://doi.org/10.1038/s41598-019-56420-z
Weng J, Ding YJ, Hu C, et al. Meta-neural-network for Real-time and Passive Deep-Learning-based Object Recognition. Nat Commun, 11: 6309. https://doi.org/10.1038/s41467-020-19693-x
Shafiei SB, Lone Z, Elsayed AS, et al., 2020, Identifying Mental Health Status Using Deep Neural Network Trained by Visual Metrics. Trans Psychiatry, 10: 430. https://doi.org/10.1038/s41398-020-01117-5
Fu F, Wei J, Zhang M, et al., 2020, Rapid Vessel Segmentation and Reconstruction of Head and Neck Angiograms Using 3d Convolutional Neural Network. Nat Commun, 11: 4829. https://doi.org/10.1038/s41467-020-18606-2
Ribeiro AH, Ribeiro MH, Paixão GM, et al., 2020, Automatic Diagnosis of the 12-lead ECG Using a Deep Neural Network. Nat Commun, 11: 1760. https://doi.org/10.1038/s41467-020-15432-4
Noyan MA, Durdu M, Eskiocak AH. 2020, Tzancknet: A Convolutional Neural Network to Identify Cells in the Cytology of Erosive-vesiculobullous Diseases. Sci Rep, 10: 18314. https://doi.org/10.1101/2020.06.22.20137570
Le Page AL, Ballot E, Truntzer C, et al., 2021, Using a Convolutional Neural Network for Classification of Squamous and Non-squamous Non-small Cell Lung Cancer Based on Diagnostic Histopathology Hes Images. Sci Rep, 11: 23912. https://doi.org/10.21203/rs.3.rs-646715/v1
Kanakasabapathy MK, Thirumalaraju P, Kandula H, et al., 2021, Adaptive Adversarial Neural Networks for the Analysis of Lossy and Domain-shifted Datasets of Medical Images. Nat Biomed Engin, 5: 571–585. https://doi.org/10.1038/s41551-021-00733-w
Elmarakeby HA, Hwang JH, Arafeh R, et al., 2021, Biologically Informed Deep Neural Network for Prostate Cancer Discovery. Nature, 598: 348–352. https://doi.org/10.1038/s41586-021-03922-4
Lu H, Uddin S. 2021, A Weighted Patient Network-based Framework for Predicting Chronic Diseases Using Graph Neural Networks. Sci Rep, 11: 22607. https://doi.org/10.1038/s41598-021-01964-2
Ahmed S, Muhammod R, Khan Z, et al., 2020, Acp- MHCNN: An Accurate Multi-headed Deep-convolutional Neural Network to Predict Anticancer Peptides. Sci Rep, 11: 313668. https://doi.org/10.1101/2020.09.25.313668
Xu W, Zhang H, Yuan H, et al., 2021, A Compliant Adaptive Gripper and its Intrinsic Force Sensing Method. IEEE Trans Robot, 37: 1584–1603. https://doi.org/10.1109/tro.2021.3060971
Ebihara Y, Shichinohe T, Kurashima Y, et al., 2021, Laparoscopic Real-time Vessel Navigation Using Indocyanine Green Fluorescence During Laparoscopy-assisted Gastric Tube Reconstruction: First Experience. J Minim Access Surg, 17: 576–579. https://doi.org/10.4103/jmas.jmas_210_20
Li X, Zhong J, Wang Y, et al., 2021, Rapid, Accurate, Multifunctional and Self-assisted Vision Assessment and Screening with Interactive Desktop Autostereoscopy. Ann Trans Med, 9: 23. https://doi.org/10.21037/atm-20-3555
Yin L, Wang Y, Zhan J, et al., 2022, Chest-scale self-compensated Epidermal Electronics for Standard 6-precordial-lead ECG. npj Flex Electron, 6: 1–9. https://doi.org/10.1038/s41528-022-00159-7
Shitiri E, Cho HS. 2021, A Tdma-based Data Gathering Protocol for Molecular Communication Via Diffusion-based Nano-sensor Networks. IEEE Sens J, 21: 19582–19595. https://doi.org/10.1109/jsen.2021.3091494
Jahromi AM, Khedri M, Ghasemi M, et al., 2021, Molecular Insight into COF Monolayers for Urea Sorption in Artificial Kidneys. Sci Rep, 11: 12085. https://doi.org/10.1038/s41598-021-91617-1
Suzuki H, Wood RJ. 2020, Origami-inspired miniature manipulator for teleoperated microsurgery. Nat Mach Intell, 2: 437–446. https://doi.org/10.1038/s42256-020-0203-4
Matsunaga T, Ohnishi K, Wada N, et al., 2019, Development of Small-diameter Haptic Flexible Gripping Forceps Robot. IEEE J Trans Ind Appl, 2019. https://doi.org/10.1002/eej.23269
Kwak B, Choi S, Maeng J, et al., 2021, Marangoni Effect Inspired Robotic Self-propulsion Over a Water Surface Using a Flow-imbibition-powered Microfluidic Pump. Sci Rep, 11: 1–13. https://doi.org/10.1038/s41598-021-96553-8
Zhang Y, Yang J, Hou X, et al., 2022, Highly Stable Flexible Pressure Sensors with a Quasi-homogeneous Composition and Interlinked Interfaces. Nat Commun, 13: 1317. https://doi.org/10.1038/s41467-022-29093-y
Barragan JA, Yang J, Yu D, et al., 2022, A Neurotechnological Aid for Semi- autonomous Suction in Robotic-assisted Surgery. Sci Rep, 12: 4504. https://doi.org/10.21203/rs.3.rs-1021937/v1
Chun S, Kim JS, Yoo YH, et al., 2021, An Artificial Neural Tactile Sensing System. Nat Electron, 4: 429–438. https://doi. org/10.1038/s41928-021-00585-x
Massari L, Fransvea G, D’Abbraccio J, et al., 2022, Functional Mimicry of Ruffini Receptors with Fiber Bragg Gratings and Deep Neural Networks Enables a Bio-inspired Large-area Tactile Sensitive Skin. ArXiv, abs/2203.12752. https://doi.org/10.1038/s42256-022-00487-3
Kim TH, Bao C, Chen Z, et al. 3d Printed Leech-inspired Origami Dry Electrodes for Electrophysiology Sensing Robots. npj Flex Electron, 6: 1–10. https://doi.org/10.1038/s41528-022-00139-x
Lee S, Kim S, Kim S, et al., 2018, A Capsule-type Microrobot with Pick-and-drop Motion for Targeted Drug and Cell Delivery. Adv Healthc Mater, 7: 1700985. https://doi.org/10.1002/adhm.201700985
Huaulmé A, Despinoy F, Perez SA, et al., 2019, Automatic annotation of surgical activities using virtual reality environments. Int J Comput Assist Radiol Surg, 14: 1663– 1671. https://doi.org/10.1007/s11548-019-02008-x.
Lu B, Chu HK, Huang KC, et al., 2019, Vision-based Surgical Suture Looping Through Trajectory Planning for Wound Suturing. IEEE Trans Automa Sci Eng, 16: 542–556. https://doi.org/10.1109/tase.2018.2840532
Borra D, Andalò A, Paci M, et al., 2020, A fully Automated Left Atrium Segmentation Approach from Late Gadolinium Enhanced Magnetic Resonance Imaging Based on a Convolutional Neural Network. Quant Imaging Med Surg, 10: 1894–1907. https://doi.org/10.21037/qims-20-168
Wang J, Yue C, Wang G, et al., 2022, Task Autonomous Medical Robot for Both Incision Stapling and Staples Removal. IEEE Robot Autom Lett, 7: 3279–3285. https://doi.org/10.1109/lra.2022.3141452
Zhang X, Wang J, Wang T, et al., 2019, A Markerless Automatic Deformable Registration Framework for Augmented Reality Navigation of Laparoscopy Partial Nephrectomy. Int J Comput Assist Radiol Surg, 14: 1285–1294. https://doi.org/10.1007/s11548-019-01974-6
Hannaford B, Rosen J, Friedman DW, et al., 2013, Raven-ii: An Open Platform for Surgical Robotics Research. IEEE Trans Biomed Eng, 60: 954–959. https://doi.org/10.1109/tbme.2012.2228858
Kazanzides P, Chen Z, Deguet A, et al., 2014, An Open-source Research Kit for the da Vinci® Surgical System. In: 2014 IEEE International Conference on Robotics and Automation (ICRA). p6434–6439. https://doi.org/10.1109/icra.2014.6907809
Steiner JA, Pham LN, Abbott JJ, et al., 2021, Modeling and Analysis of a Soft Endoluminal Inchworm Robot Propelled by a Rotating Magnetic Dipole Field. J Mech Robot, 14: 11. https://doi.org/10.1115/1.4053114
Pham LN, Steiner JA, Leang KK, et al., 2020, Soft Endoluminal Robots Propelled by Rotating Magnetic Dipole Fields. IEEE Trans Med Robot Bionic, 2: 598–607. https://doi.org/10.1109/tmrb.2020.3027871
Kaouk JH, Haber GP, Goel RK, et al., 2010, Pure Natural Orifice Translumenal Endoscopic Surgery (Notes) Transvaginal Nephrectomy. Eur Urol, 57: 723–726. https://doi.org/10.1016/j.eururo.2009.10.027
Rahimy E, Wilson J, Tsao TC, et al., 2013, Robot-assisted Intraocular Surgery: Development of the Iriss and Feasibility Studies in an Animal Model. Eye, 27: 972–978. https://doi.org/10.1038/eye.2013.105
Wavhale R, Dhobale KD, Rahane CS, et al., 2021, Water-powered Self-propelled Magnetic Nanobot for Rapid and Highly Efficient Capture of Circulating Tumor Cells. Commun Chem, 4: 1–9. https://doi.org/10.1038/s42004-021-00598-9
Shen T, Hennings DL, Nelson CA, et al., 2018, Performance of a Multifunctional Robot for Natural Orifice Transluminal Endoscopic Surgery. Surg Innov, 25: 364–373. https://doi.org/10.1177/1553350618781225
Shen T, Hennings DL, Nelson CA, et al., 2018, Performance of a Multifunctional Robot for Natural Orifice Transluminal Endoscopic Surgery. Surg Innov, 25: 364–373. https://doi.org/10.1177/1553350618781225
Bai W, Wang Z, Cao Q, et al., 20225, Anthropomorphic Dual-arm Coordinated Control for a Single-port Surgical Robot Based on Dual-step Optimization. IEEE Trans Med Robot Bionic, 4: 72–84. https://doi.org/10.1109/tmrb.2022.3145673
Wang F, Toombs NJ, Kesavadas T, et al., 2019, Mechanical Design and Modeling of a Manipulator Tool for a Compact Multiple-tool Single Port Laparoscopic Robot Platform. 2019 41st Annual International Conference of the IEEE Engineering in Medicine and Biology Society (EMBC). p5836–5841. https://doi.org/10.1109/embc.2019.8857539
Hueso M, Navarro E, Sandoval D, et al., 2018, Progress in the Development and Challenges for the use of Artificial Kidneys and Wearable Dialysis Devices. Kidney Dis (Basel), 5: 3–10. https://doi.org/10.1159/000492932
Budhathoki-Uprety J, Shah J, Korsen JA, et al. Synthetic Molecular Recognition Nanosensor Paint for Microalbuminuria. Nat Commun, 10: 3605. https://doi.org/10.1038/s41467-019-11583-1
Siontis KC, Noseworthy PA, Attia ZI, et al., 2021, Artificial Intelligence- enhanced Electrocardiography in Cardiovascular Disease Management. Nat Rev Cardiol, 18: 465–478. https://doi.org/10.1038/s41569-020-00503-2
Siontis KC, Noseworthy PA, Attia ZI, et al., 2021, Artificial Intelligence- enhanced Electrocardiography in Cardiovascular Disease Management. Nat Rev Cardiol, 18: 465–478. https://doi.org/10.1038/s41569-020-00503-2
Hussein SE, Chen P, Medeiros LJ, et al., 2022, Artificial Intelligence-assisted Mapping of Proliferation Centers Allows the Distinction of Accelerated Phase from Large Cell Transformation in Chronic Lymphocytic Leukemia. Mod Pathol, 35: 1121–1125. https://doi.org/10.1038/s41379-022-01015-9
Bayoumy K, Gaber M, Elshafeey A, et al., 2021, Smart Wearable Devices in Cardiovascular Care: Where we are and How to Move Forward. Nat Rev Cardiol, 18: 581–599. https://doi.org/10.1038/s41569-021-00522-7
Himmelfarb J, Ratner B. 2020, Wearable Artificial Kidney: Problems, Progress and Prospects. Nat Rev Nephrol, 16: 558–559. https://doi.org/10.1038/s41581-020-0318-1
Mintchev S, Salerno M, Cherpillod A, et al., 2019, A Portable Three-degrees-of-freedom force Feedback Origami Robot for Human-robot Interactions. Nat Mach Intell, 1: 584–593. https://doi.org/10.1038/s42256-019-0125-1
Heiligenstein X, Paul-Gilloteaux P, Belle M, et al., 2017, Ec-clem: Flexible Multidimensional Registration Software for Correlative Microscopies with Refined Accuracy Mapping. Microsc Microanal, 23: 360–361. https://doi.org/10.1017/s1431927617002483
Pore A, Corsi D, Marchesini E, et al., 2021, Safe Reinforcement Learning Using Formal Verification for Tissue Retraction in Autonomous Robotic-assisted Surgery. In: 2021 IEEE/RSJ International Conference on Intelligent Robots and Systems (IROS). p4025–4031. https://doi.org/10.1109/iros51168.2021.9636175