Complete electrothermal and lifetime model of 18650 nickel manganese cobalt cell based on artificial neural network
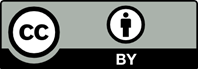
This study presents a comprehensive electrothermal and lifetime model for cylindrical 3 ampere-hours (Ah) lithium-ion cells using artificial neural networks (ANNs) to estimate the cell’s lifespan. The model combines an electrothermal component with an ANN-based lifetime prediction approach, offering a holistic representation of cell behavior over its lifetime by incorporating key parameters, including the state of charge, temperature, current, and cycle life. The ANN is trained offline using extensive experimental data collected from Sony cylindrical 3 Ah cells under various operating conditions. The electrothermal component employs a second-order Thévenin equivalent circuit model topology, enhanced with extended versions of characterization and parameterization procedures. Validation of the coupled model is performed using laboratory tests at different stages of the cells’ life (500, 1000, and 1500 cycles), demonstrating its ability to estimate cell electrical and thermal performance across a broad lifespan range. Results indicate a maximum error of 1% in voltage readings and 3% in temperature evolution during discharge with the complete model. This comprehensive approach not only enhances the understanding of long-term Sony 3 Ah cell dynamics but also provides a computationally efficient tool for battery management systems and control strategies. The model’s capability to predict both electrical and thermal performance simultaneously at different stages of the cell’s lifetime makes it particularly valuable for optimizing battery performance and lifespan in various applications.
- Armand M, Axmann P, Bresser D, et al. Lithium-ion batteries-current state of the art and anticipated developments. J Power Sources. 2020;479:228708. doi: 10.1016/j.jpowsour.2020.228708
- Elalfy DA, Gouda E, Kotb MF, Bureš V, Sedhom BE. Comprehensive review of energy storage systems technologies, objectives, challenges, and future trends. Energy Strateg Rev. 2024;54:101482. doi: 10.1016/j.esr.2024.101482
- Jindal P, Katiyar R. Evaluation of accuracy for Bernardi equation in estimating heat generation rate for continuous and pulse-discharge protocols in LFP and NMC based Li-ion batteries. Appl Therm Eng. 2022;201:117794.
- Giammichele L, D’Alessandro V, Falone M, Ricci R. Thermal behaviour of a cylindrical li-ion battery. Tec Ital J Eng Sci. 2021;65(2-4):218-223. doi: 10.18280/ti-ijes.652-412
- Liu S, Liu X, Dou R, Zhou W, Wen Z, Liu L. Experimental and simulation study on thermal characteristics of 18,650 lithium-iron-phosphate battery with and without spot-welding tabs. Appl Therm Eng. 2020;166:114648. doi: 10.1016/j.applthermaleng.2019.114648
- Lyu P, Huo Y, Qu Z, Rao Z. Investigation on the thermal behavior of Ni-rich NMC lithium ion battery for energy storage. Appl Therm Eng. 2020;166:114749. doi: 10.1016/j.applthermaleng.2019.114749
- Liu J, Yadav S, Salman M. Review of thermal coupled battery models and parameter identification for lithium-ion battery heat generation in EV battery thermal management system. Int J Heat Mass Transf. 2024;218:124748. doi: 10.1016/j.ijheatmasstransfer.2023.124748
- Lin X, Kim Y, Mohan S, Siegel JB, Stefanopoulou AG. Modeling and estimation for advanced battery management. Annu Rev Control Robot Annu Rev Control Robot Auton Syst. 2019;2:393-426. doi: 10.1146/annurev-control-053018
- Thiruvonasundari D, Deepa K. Electric vehicle battery modelling methods based on state of charge-review. J Green Eng. 2020;10:24-61.
- Hou M, Hu Y, Zhang J, Cao H, Wang Z. Development of electrochemical-thermal modelling for large-format Li-ion battery. Electrochim Acta. 2020;347:136280. doi: 10.1016/j.electacta.2020.136280
- Nie P, Zhang SW, Ran A, et al. Full-cycle electrochemical-thermal coupling analysis for commercial lithium-ion batteries. Appl Therm Eng. 2021;184:116258. doi: 10.1016/j.applthermaleng.2020.116258
- Khodadadi Sadabadi K, Ramesh P, Guezennec Y, Rizzoni G. Development of an electrochemical model for a lithium titanate oxide nickel manganese cobalt battery module. J Energy Storage. 2022;50:104046. doi: 10.1016/j.est.2022.104046
- Kadız GG, Ünverdi S. Genetic algorithm-based multi-objective optimization of fast-charging parameters of a lithium-ion battery by a coupled equivalent electric circuit-heat transfer model. Int Conf Therm Eng. 2023;2023(1):1-3.
- Berckmans G, Jaguemont J, Samba A, et al. Lithium-Ion Capacitor-Analysis of Thermal Behaviour and Development of 3D Thermal Model. Available from: https://www.mdpi. com/journal/energies [Last accessed on 2025 Feb 27].
- Samba A, Omar N, Gualous H, et al. Development of an advanced two-dimensional thermal model for large size lithium-ion pouch cells. Electrochim Acta. 2014;117:246-254. doi: 10.1016/j.electacta.2013.11.113
- Pasdarshahri H, Veilleux É, Mooney W, Fréchette LG, Grondin F, Rancourt D. Coupled electro-thermal-aging battery pack modeling-part 1: Cell level. Batteries. 2024;10(11):1-14. doi: 10.3390/batteries10110404
- Sui X, Świerczyński M, Teodorescu R, Stroe DI. The degradation behavior of lifepo4/c batteries during long-term calendar aging. Energies. 2021;14(6):1732. doi: 10.3390/en14061732
- Hosen MS, Pirooz A, Kalogiannis T, He J, Van Mierlo J, Berecibar M. A strategic pathway from cell to pack-level battery lifetime model development. Appl Sci. 2022;12(9):4781. doi: 10.3390/app12094781
- Motapon SN, Lachance E, Dessaint LA, Al-Haddad K. A Generic cycle life model for lithium-ion batteries based on fatigue theory and equivalent cycle counting. IEEE Open J Ind Electron Soc. 2020;1:207-217. doi: 10.1109/OJIES.2020.3015396
- Khodadadi Sadabadi K, Jin X, Rizzoni G. Prediction of remaining useful life for a composite electrode lithium ion battery cell using an electrochemical model to estimate the state of health. J Power Sources. 2021;481:228861. doi: 10.1016/j.jpowsour.2020.228861
- Keil J, Jossen A. Electrochemical modeling of linear and nonlinear aging of lithium-ion cells. J Electrochem Soc. 2020;167(11):110535. doi: 10.1149/1945-7111/aba44f
- Hu X, Xu L, Lin X, Pecht M. Battery lifetime prognostics. Joule. 2020;4(2):310-346. doi: 10.1016/j.joule.2019.11.018
- Xiong R, Wang S, Takyi-Aninakwa P, et al. Critical review on improved electrochemical impedance spectroscopy-cuckoo search-elman neural network modeling methods for whole-life-cycle health state estimation of lithium-ion battery energy storage systems. Prot Control Mod Power Syst. 2024;9(2):75-100. doi: 10.23919/pcmp.2023.000234
- Gasper P, Collath N, Hesse HC, Jossen A, Smith K. Machine-learning assisted identification of accurate battery lifetime models with uncertainty. J Electrochem Soc. 2022;169(8):080518. doi: 10.1149/1945-7111/ac86a8
- Gasper P, Gering K, Dufek E, Smith K. Challenging practices of algebraic battery life models through statistical validation and model identification via machine-learning. J Electrochem Soc. 2021;168(2):020502. doi: 10.1149/1945-7111/abdde1
- Gou B, Xu Y. An ensemble learning-based data-driven method for online state-of-health estimation of lithium-ion batteries. IEEE Trans Transp Electrif. 2021;7(2):422-436. doi: 10.1109/TTE.2020.3029295
- Yang R, Xiong R, Ma S, Lin X. Characterization of external short circuit faults in electric vehicle Li-ion battery packs and prediction using artificial neural networks. Appl Energy. 2020;260:114253. doi: 10.1016/j.apenergy.2019.114253
- Hosen MS, Jaguemont J, Van Mierlo J, Berecibar M. Battery lifetime prediction and performance assessment of different modeling approaches. iScience. 2021;24(2):102060. doi: 10.1016/j.isci.2021.102060
- Rouholamini M, Wang C, Nehrir H, et al. A review of modeling, management, and applications of grid-connected li-ion battery storage systems. IEEE Trans Smart Grid. 2022;13(6):4505-4524. doi: 10.1109/TSG.2022.3188598
- Okwu MO, Tartibu LK. Metaheuristic Optimization: Nature- Inspired Algorithms Swarm and Computational Intelligence, Theory and Applications. Vol. 927; 2021. Available from: https://link.springer.com/10.1007/978-3-030-61111-8 [Last accessed on 2025 Feb 27].
- Gan N, Sun Z, Zhang Z, Xu S, Liu P, Qin Z. Data-driven fault diagnosis of lithium-ion battery overdischarge in electric vehicles. IEEE Trans Power Electron. 2022;37(4):4575-4588. doi: 10.1109/TPEL.2021.3121701
- Severson KA, Attia PM, Jin N, et al. Data-driven prediction of battery cycle life before capacity degradation. Nat Energy. 2019;4:383-391. doi: 10.1038/s41560-019-0356-8
- Attia PM, Grover A, Jin N, et al. Closed-loop optimization of fast-charging protocols for batteries with machine learning. Nature. 2020;578(7795):397-402. doi: 10.1038/s41586-020-1994-5
- Dai H, Zhao G, Lin M, Wu J, Zheng G. A novel estimation method for the state of health of lithium-ion battery using prior knowledge-based neural network and markov chain. IEEE Trans Ind Electron. 2019;66(10):7706-7716. doi: 10.1109/TIE.2018.2880703
- Guo M, Sikha G, White RE. Single-particle model for a lithium-ion cell: Thermal behavior. J Electrochem Soc. 2011;158(2):A122. doi: 10.1149/1.3521314
- Wu J, Wang Y, Zhang X, Chen Z. A novel state of health estimation method of Li-ion battery using group method of data handling. J Power Sources. 2016;327:457-464. doi: 10.1016/j.jpowsour.2016.07.065
- Li X, Yuan C, Wang Z. Multi-time-scale framework for prognostic health condition of lithium battery using modified Gaussian process regression and nonlinear regression. J Power Sources. 2020;467:228358. doi: 10.1016/j.jpowsour.2020.228358
- Type C. Sony VTC6 Battery Technical Information; 2015. Available from: https://www.kronium.cz/uploads/SONY_ US18650VTC6.pdf [Last accessed on 2025 Feb 27].
- Roberts C, Petrovich S, Ebrahimi K. Battery modeling for emulators in vehicle test cell. Batteries. 2024;10(6):199. doi: 10.3390/batteries10060199
- Sungur B. State of charge estimation for lithium-ion batteries using optimized model based on optimal HPPC conditions created using taguchi method and multi-objective optimization. Appl Sci. 2024;14:9245.
- Baccouche I, Jemmali S, Manai B, Omar N, Essoukri Ben Amara N. Improved OCV model of a Li-ion NMC battery for online SOC estimation using the extended Kalman filter. Energies. 2017;10(6):1-22. doi: 10.3390/en10060764
- Zhang C, Jiang J, Zhang L, Liu S, Wang L, Loh PC. A generalized SOC-OCV model for lithium-ion batteries and the SOC estimation for LNMCO battery. Energies. 2016;9(11):900. doi: 10.3390/en9110900
- Qin Y, Du J, Lu L, et al. A rapid lithium-ion battery heating method based on bidirectional pulsed current: Heating effect and impact on battery life. Appl Energy. 2020;280:115957. doi: 10.1016/j.apenergy.2020.115957
- Akbarzadeh M, Kalogiannis T, Jaguemont J, et al. Thermal modeling of a high-energy prismatic lithium-ion battery cell and module based on a new thermal characterization methodology. J Energy Storage. 2020;32:101707. doi: 10.1016/j.est.2020.101707
- Jaguemont J, Nikolian A, Omar N, Goutam S, Van Mierlo J, Van Den Bossche P. Development of a two-dimensional-thermal model of three battery chemistries. IEEE Trans Energy Convers. 2017;32(4):1447-1455. doi: 10.1109/TEC.2017.2697944
- FreedomCAR. FreedomCAR Battery Test Manual For Power- Assist Hybrid Electric Vehicles Disclaimer; 2003. Available from: https://avt.inl.gov/sites/default/files/pdf/battery/freedomcar_ manual_04_15_03.pdf [Last accessed on 2025 Feb 27].
- Ruiz V. Standards for the Performance and Durability Assessment of Electric Vehicle Batteries-Possible Performance Criteria for an Ecodesign Regulation. Eng Mater Sci. 2018;68.
- Abolhassani Monfared N, Gharib N, Moqtaderi H, et al. Prediction of state-of-charge effects on lead-acid battery characteristics using neural network parameter modifier. J Power Sources. 2006;158(2 Specail issue):932-935. doi: 10.1016/j.jpowsour.2005.11.023
- Nikolian A, Jaguemont J, de Hoog J, et al. Complete cell-level lithium-ion electrical ECM model for different chemistries (NMC, LFP, LTO) and temperatures (−5 °C to 45 °C)-Optimized modelling techniques. Int J Electr Power Energy Syst. 2018;98:133-146. doi: 10.1016/j.ijepes.2017.11.031
- He H, Xiong R, Fan J. Evaluation of lithium-ion battery equivalent circuit models for state of charge estimation by an experimental approach. Energies. 2011;4(4):582-598. doi: 10.3390/en4040582
- Jaguemont J, Darwiche A, Barde F. Optimal fast-charging strategy for cylindrical li-ion cells at different temperatures. World Electr Veh J. 2024;15(8):330. doi: 10.3390/wevj15080330
- Jaguemont J, Darwiche A, Bardé F. Optimal fast-charging strategy for cylindrical li-ion cells. Highlights Veh. 2024;2:24-34.
- White G, Hales A. Novel methods for measuring the thermal diffusivity and the thermal conductivity of a lithium-ion battery. Appl Therm Eng. 2022;212:118573.
- Wu MS, Liu KH, Wang YY, Wan CC. Heat dissipation design for lithium-ion batteries. J Power Sources. 2002;109(1):160-166. doi: 10.1016/S0378-7753(02)00048-4
- De Hoog J, Jaguemont J, Abdel-Monem M, Van Den Bossche P, Van Mierlo J, Omar N. Combining an electrothermal and impedance aging model to investigate thermal degradation caused by fast charging. Energies. 2018;11(4):804. doi: 10.3390/en11040804
- Marotta A, Tutuianu M. Europe-Centric Light Duty Test Cycle and Differences with Respect to the WLTP Cycle. Vol. 7-10. Luxembourg: Institute for Energy and Transport; 2012. doi: 10.2790/53651
- de Hoog J, Timmermans JM, Ioan-Stroe D, et al. Combined cycling and calendar capacity fade modeling of a Nickel- Manganese-Cobalt Oxide Cell with real-life profile validation. Appl Energy. 2017;200:47-61. doi: 10.1016/j.apenergy.2017.05.018
- Tu H, Moura S, Wang Y, Fang H. Integrating physics-based modeling with machine learning for lithium-ion batteries. Appl Energy. 2023;329:1-25. doi: 10.1016/j.apenergy.2022.120289
- Pour MY. Electro-Thermal Modeling of Lithium-Ion Batteries. 2015. Available from: https://www.sfu.ca/~mbahrami/pdf/ Theses/Thesis - M. Yazdan Pour - Electro-thermal Modeling of Lithium-ion Battery.pdf [Last accessed on 2025 Feb 27].
- Zhang L, Peng H, Ning Z, Mu Z, Sun C. Comparative research on RC equivalent circuit models for lithium-ion batteries of electric vehicles. Appl Sci. 2017;7(10):1002. doi: 10.3390/app7101002