Evaluating the cognitive and electrophysiological plausibility of a thalamic computational model
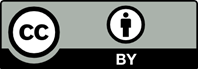
The thalamus acts as a gateway to the cortex, relaying information from the brainstem and spinal cord to cortical regions. Evidence suggests that thalamic networks play a role in pattern recognition by extracting key elements from sensory input. We propose that thalamic networks function as a central orthogonalizer in the brain, enabling the application of the Hebbian learning rule without contamination or “interference” between the correct output for one input pattern and the output for other input patterns. This study aims to describe a biologically plausible artificial neural network that mimics aspects of the physiological activity of the thalamic circuit. To validate this proposal, the network was tested in four different scenarios. The model successfully replicated electrophysiological processes, including: (i) inhibitory facilitation; (ii) waveform sculpting in reticular cells; (iii) pattern completion of thalamic input; and (iv) computational processing of stabilized images on the retina. In human experiments, stabilized retinal images were perceived as sequences of patterns that appeared and disappeared suddenly. These patterns closely resembled or were related to the presented image, suggesting that they represent a mix of principal components derived from learned images. Such components emerge when a complete image, or only a portion of it, reaches the thalamus. Our model effectively reconstructs images based on partial eye-to-thalamus information, mirroring human visual responses.

- Martin SJ, Grimwood PD, Morris RGM. Synaptic plasticity and memory: An evaluation of the hypothesis. Annu Rev Neurosci. 2000;23:649-711. doi: 10.1146/ANNUREV.NEURO.23.1.649
- Martin SJ, Morris RGM. New life in an old idea: The synaptic plasticity and memory hypothesis revisited. Hippocampus. 2002;12(5):609-636. doi: 10.1002/HIPO.10107
- Hebb DO. The Organization of Behavior: A Neuropsychological Theory. United States: Wiley; 1949.
- Bliss TVP, Lømo T. Long-lasting potentiation of synaptic transmission in the dentate area of the anaesthetized rabbit following stimulation of the perforant path. J Physiol. 1973;232(2):331-356. doi: 10.1113/JPHYSIOL.1973.SP010273
- Gallistel CR, Matzel LD. The neuroscience of learning: Beyond the Hebbian synapse. Annu Rev Psychol. 2013;64:169-200. doi: 10.1146/ANNUREV-PSYCH-113011-143807
- McClelland JL, Rumelhart DE, Group PR. Parallel Distributed Processing, Volume 2: Explorations in the Microstructure of Cognition: Psychological and Biological Models. United States: The MIT Press; 1986. doi: 10.7551/MITPRESS/5237.001.0001
- Haykin S. Neural Networks and Learning Machines. 3rd ed. Hoboken: Pearson Prentice Hall; 2009.
- Pelaez JR, Simoes MG. Pattern Completion through Thalamo-Cortical Interaction. In: IJCNN’99. International Joint Conference on Neural Networks. Proceedings (Cat. No.99CH36339). Vol. 1. IEEE; 1999. p. 125-130. doi: 10.1109/IJCNN.1999.831469
- McClelland JL. Explorations in Parallel Distributed Processing: A Handbook of Models, Programs, and Exercises. 2nd ed. California: Stanford Psychology Department; 2015.
- Pelaez JR. Schizophrenia Positive Symptoms Interpreted as Cognitive Hallucinations. Berlin, Heidelberg: Springer; 2003. p. 1156-1163. doi: 10.1007/978-3-540-45226-3_157
- Pelaez JR. Plato’s theory of ideas revisited. Neural Netw. 1997;10(7):1269-1288. doi: 10.1016/S0893-6080(97)00052-X
- Peláez JR. Towards a neural network based therapy for hallucinatory disorders. Neural Netw. 2000;13(8-9):1047-1061. doi: 10.1016/S0893-6080(00)00069-1
- Felten DL, O’Banion MK, Maida MS. Netter’s Atlas of Neuroscience. 3rd ed. Amsterdam: Elsevier; 2015. doi: 10.1016/B978-0-323-26511-9.00018-7
- Bear MF, Connors BW, Paradiso MA. Neuroscience: Exploring the Brain. 4th ed. United States: Jones and Bartlett Learning; 2020.
- Courtiol E, Wilson DA. The olfactory thalamus: Unanswered questions about the role of the mediodorsal thalamic nucleus in olfaction. Front Neural Circuits. 2015;9:156303. doi: 10.3389/FNCIR.2015.00049/BIBTEX
- Sherman MS, Guillery RW. Exploring the Thalamus and Its Role in Cortical Function. 2nd ed. United States: The MIT Press; 2009.
- Halassa MM, editor. The Thalamus. United Kingdom: Cambridge University Press; 2022. doi: 10.1017/9781108674287
- Müller EJ, Munn BR, Redinbaugh MJ, et al. The non-specific matrix thalamus facilitates the cortical information processing modes relevant for conscious awareness. Cell Rep. 2023;42(8):112844. doi: 10.1016/j.celrep.2023.112844
- Sherman SM, Guillery RW. Functional Connections of Cortical Areas: A New View from the Thalamus. United States: The MIT Press; 2013. doi: 10.7551/MITPRESS/9780262019309.001.0001
- Izhikevich EM, Edelman GM. Large-scale model of mammalian thalamocortical systems. Proc Natl Acad Sci U S A. 2008;105(9):3593-3598. doi: 10.1073/pnas.0712231105
- Crick F, Asanuma C. Certain aspects of the anatomy and physiology of the cerebral cortex. In: McClelland JL, Rumelhart DE, editors. Parallel Distributed Processing. Vol. 2. United States: MIT Press; 1999. p. 611.
- Llinás R, Ribary U, Contreras D, Pedroarena G. The neuronal basis for consciousness. Philos Trans R Soc Lond B Biol Sci. 1998;353(1377):1841-1849. doi: 10.1098/rstb.1998.0336
- Llinás R, Leznik El, Urbano FJ. Temporal binding via cortical coincidence detection of specific and nonspecific thalamocortical inputs: A voltage-dependent dye-imaging study in mouse brain slices. Proc Natl Acad Sci U S A. 2002;99(1):449-454. doi: 10.1073/pnas.012604899
- Sherman SM. Functioning of circuits connecting thalamus and cortex. Compr Physiol. 2017;7(2):713-739. doi: 10.1002/cphy.c160032
- Shepherd G, Grillner S. Handbook of Brain Microcircuits. United Kingdom: Oxford University Press; 2010. doi: 10.1093/MED/9780195389883.001.0001
- Shepherd GM, Rowe TB. Neocortical lamination: Insights from neuron types and evolutionary precursors. Front Neuroanat. 2017;11:100. doi: 10.3389/fnana.2017.00100
- Zhu JJ, Lo FS. Three GABA receptor-mediated postsynaptic potentials in interneurons in the rat lateral geniculate nucleus. J Neurosci. 1999;19(14):5721-5730. doi: 10.1523/jneurosci.19-14-05721.1999
- Sherman SM, Usrey WM. Exploring Thalamocortical Interactions. United Kingdom: Oxford University Press; 2021. doi: 10.1093/med/9780197503874.001.0001
- Sur M, Leamey CA. Development and plasticity of cortical areas and networks. Nat Rev Neurosci. 2001;2(4):251-262. doi: 10.1038/35067562
- Mulle C, Madariaga A, Deschenes M. Morphology and electrophysiological properties of reticularis thalami neurons in cat: In vivo study of a thalamic pacemaker. J Neurosci. 1986;6(8):2134-2145. doi: 10.1523/JNEUROSCI.06-08-02134.1986
- Deschênes M, Madariaga-Domich A, Steriade M. Dendrodendritic synapses in the cat reticularis thalami nucleus: A structural basis for thalamic spindle synchronization. Brain Res. 1985;334(1):165-168. doi: 10.1016/0006-8993(85)90580-3
- Cox CL, Zhou Q, Sherman SM. Glutamate locally activates dendritic outputs of thalamic interneurons. Nature. 1998;394(6692):478-482. doi: 10.1038/28855
- Diamantaras KI, Kung SY. Principal Component Neural Networks: Theory and Applications. United States: John Wiley and Sons, Inc; 1996.
- Tsytsarev V. Methodological aspects of studying the mechanisms of consciousness. Behav Brain Res. 2022;419:113684. doi: 10.1016/j.bbr.2021.113684
- Redinbaugh MJ, Phillips JM, Kambi NA, et al. Thalamus modulates consciousness via layer-specific control of cortex. Neuron. 2020;106(1):66-75.e12. doi: 10.1016/j.neuron.2020.01.005
- Yamamoto T, Katayama Y, Obuchi T, Kobayashi K, Oshima H, Fukaya C. Deep brain stimulation and spinal cord stimulation for vegetative state and minimally conscious state. World Neurosurg. 2013;80(3-4):S30.e1-S30.e9. doi: 10.1016/j.wneu.2012.04.010
- Schiff ND. Central thalamic deep brain stimulation for support of forebrain arousal regulation in the minimally conscious state. Handb Clin Neurol. 2013;116:295-306. doi: 10.1016/B978-0-444-53497-2.00024-3
- Alkire MT, Hudetz AG, Tononi G. Consciousness and Anesthesia. Science. 2008;322(5903):876-880. doi: 10.1126/science.1149213
- Mashour GA, Hudetz AG. Bottom-up and top-down mechanisms of general anesthetics modulate different dimensions of consciousness. Front Neural Circuits. 2017;11:44. doi: 10.3389/fncir.2017.00044
- Pritchard RM. Stabilized images on the retina. Sci Am. 1961;204:72-78. doi: 10.1038/SCIENTIFICAMERICAN0661-72
- Bazhenov M, Timofeev I, Steriade M, Sejnowski TJ. computational models of thalamocortical augmenting responses. J Neurosci. 1998;18(16):6444-6465. doi: 10.1523/JNEUROSCI.18-16-06444.1998
- Sumner RL, Spriggs MJ, Shaw AD. Modelling thalamocortical circuitry shows that visually induced LTP changes laminar connectivity in human visual cortex. PLoS Comput Biol. 2021;17(1):e1008414. doi: 10.1371/journal.pcbi.1008414
- Henning Proske J, Jeanmonod D, Verschure PFMJ. A computational model of thalamocortical dysrhythmia. Eur J Neurosci. 2011;33(7):1281-1290. doi: 10.1111/j.1460-9568.2010.07588.x
- Li Q, Westover MB, Zhang R, Chu CJ. Computational evidence for a competitive thalamocortical model of spikes and spindle activity in rolandic epilepsy. Front Comput Neurosci. 2021;15:680549. doi: 10.3389/fncom.2021.680549
- Han C, English G, Saal HP, et al. Modelling novelty detection in the thalamocortical loop. PLoS Comput Biol. 2023;19(5):e1009616. doi: 10.1371/journal.pcbi.1009616
- Lakshminarasimhan KJ, Xie M, Cohen JD, et al. Specific connectivity optimizes learning in thalamocortical loops. Cell Rep. 2024;43(4):114059. doi: 10.1016/j.celrep.2024.114059
- Murray JM. Local online learning in recurrent networks with random feedback. Elife. 2019;8:e43299. doi: 10.7554/eLife.43299
- Bhattacharya S, Cauchois MBL, Iglesias PA, Chen ZS. The impact of a closed-loop thalamocortical model on the spatiotemporal dynamics of cortical and thalamic traveling waves. Sci Rep. 2021;11(1):14359. doi: 10.1038/s41598-021-93618-6
- Izhikevich EM. Simple model of spiking neurons. IEEE Trans Neural Netw. 2003;14(6):1569-1572. doi: 10.1109/TNN.2003.820440
- Mitchell AS, Sherman SM, Sommer MA, Mair RG, Vertes RP, Chudasama Y. Advances in understanding mechanisms of thalamic relays in cognition and behavior. J Neurosci. 2014;34(46):15340-15346. doi: 10.1523/JNEUROSCI.3289-14.2014
- Usrey WM, Sherman SM, editors. The Cerebral Cortex and Thalamus. New York: Oxford University Press; 2023. doi: 10.1093/med/9780197676158.001.0001
- Wolff M, Vann SD. The cognitive thalamus as a gateway to mental representations. J Neurosci. 2019;39(1):3-14. doi: 10.1523/JNEUROSCI.0479-18.2018
- Aguiar-Furucho MA, Peláez FJR. Alzheimer’s disease as a result of stimulus reduction in a GABA-A-deficient brain: A neurocomputational model. Neural Plast. 2020;2020:8895369. doi: 10.1155/2020/8895369
- Peláez FJR, Aguiar-Furucho MA, Andina D. Intrinsic plasticity for natural competition in koniocortex-like neural networks. Int J Neural Syst. 2016;26(05):1650040. doi: 10.1142/S0129065716500404
- Pelaez JR, Andina D. Do biological synapses perform probabilistic computations? Neurocomputing. 2013;114:24-31. doi: 10.1016/j.neucom.2012.08.042
- Artola A, Bröcher S, Singer W. Different voltage-dependent thresholds for inducing long-term depression and long-term potentiation in slices of rat visual cortex. Nature. 1990;347(6288):69-72. doi: 10.1038/347069a0
- Kim U, Mccormick DA. The functional influence of burst and tonic firing mode on synaptic interactions in the thalamus. J Neurosci. 1998;18(22):9500-9516. doi: 10.1523/JNEUROSCI.18-22-09500.1998
- Jackman SL, Regehr WG. The mechanisms and functions of synaptic facilitation. Neuron. 2017;94(3):447-464. doi: 10.1016/J.NEURON.2017.02.047
- Ramachandran VS, Blakeslee S. Phantoms in the Brain: Probing the Misteries of Human Mind. New York: William Morrows and Company; 1999.
- Wolff M, Morceau S, Folkard R, Martin-Cortecero J, Groh A. A thalamic bridge from sensory perception to cognition. Neurosci Biobehav Rev. 2021;120:222-235. doi: 10.1016/j.neubiorev.2020.11.013
- Kriegeskorte N, Douglas PK. Cognitive computational neuroscience. Nat Neurosci. 2018;21:1148-1160. doi: 10.1038/s41593-018-0210-5