Revolutionizing new drug discovery: Harnessing AI and machine learning to overcome traditional challenges and accelerate targeted therapies
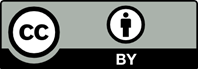
Designing highly targeted, selective drugs with desirable absorption, distribution, metabolism, excretion, and pharmacokinetic (PK) profiles; single-digit nanomolar efficacy; and a wider therapeutic index are challenging. In the traditional drug discovery process, researchers screen thousands of chemical compounds during pre-clinical development, progressing through hit identification, lead optimization, and candidate selection to shortlist – potential clinical candidates with favorable PK profiles, high tolerability, and manageable toxicity. The selected candidate must demonstrate sufficient efficacy in treating the target disease in humans. Despite these efforts, the success rate of the pre-clinical candidate to sail through Phase I, Phase II, and Phase III in clinical trials remains exceedingly low. Supported by powerful data-driven tools, artificial intelligence (AI) has transformed this traditional drug discovery process by enabling the analysis of large quantities of omics, phenotypic, and expression data to identify the biological mechanisms of pathological conditions and in turn identify druggable proteins or genes. The generative AI-powered toolbox creates novel compounds from scratch, assists scientists in optimizing druggability attributes, and bridges the differences between animal and human physiology and anatomy to predict potential toxicity in humans with high accuracy. This review discusses the bottlenecks in the traditional drug discovery approach, the impact of AI and machine learning (ML) in drug discovery, and potential challenges associated with AI/ML adoption.
- Schlander M, Hernandez-Villafuerte K, Cheng CY, Mestre- Ferrandiz J, Baumann M. How much does it cost to research and develop a new drug? A systematic review and assessment. Pharmacoeconomics. 2021;39:1243-1269. doi: 10.1007/s40273-021-01065-y
- Sun D, Gao W, Hu H, Zhou S. Why 90% of clinical drug development fails and how to improve it? Acta Pharm Sin B. 2022;12:3049-3062. doi: 10.1016/j.apsb.2022.02.002
- Waring MJ, Arrowsmith J, Leach AR et al. An analysis of the attrition of drug candidates from four major pharmaceutical companies. Nat Rev Drug Discov. 2015;14:475-486. doi: 10.1038/nrd4609
- Dueñas ME, Peltier-Heap RE, Leveridge M, Annan RS, Büttner FH, Trost M. Advances in high‐throughput mass spectrometry in drug discovery. EMBO Mol Med. 2023;15(1):e14850. doi: 10.15252/emmm.202114850
- Komura H, Watanabe R, Mizuguchi K. The trends and future prospective of in silico models from the viewpoint of ADME evaluation in drug discovery. Pharmaceutics. 2023;15(11):2619. doi: 10.3390/pharmaceutics15112619
- Wang S, Dong G, Shenga C. Structural simplification: An efficient strategy in lead optimization. Acta Pharm Sin B. 2019;9(5):880-901. doi: 10.1016/j.apsb.2019.05.004
- Khalil AS, Jaenisch R, Mooney DJ. Engineered tissues and strategies to overcome challenges in drug development. Adv Drug Deliv Rev. 2020;158:116-139. doi: 10.1016/j.addr.2020.09.012
- Wang L, Hu D, Xu J, Hu J, Wang Y. Complex in vitro model: A transformative model in drug development and precision medicine. Clin Transl Sci. 2024;17(2):e13695. doi: 10.1111/cts.13695
- Onakpoya IJ, Heneghan CJ, Aronson JK. Worldwide withdrawal of medicinal products because of adverse drug reactions: A systematic review and analysis. Crit Rev Toxicol. 2016;46(6):477-489. doi: 10.3109/10408444.2016.1149452
- Amacher DE. The primary role of hepatic metabolism in idiosyncratic drug-induced liver injury. Expert Opin Drug Metab Toxicol. 2012;8(3):335-347. doi: 10.1517/17425255.2012.658041
- Weaver RJ, Blomme EA, Chadwick AE, et al. Managing the challenge of drug-induced liver injury: A roadmap for the development and deployment of preclinical predictive models. Nat Rev Drug Discov. 2020;19(2):131-148. doi: 10.1038/s41573-019-0048-x
- Meunier L, Larrey D. Drug-induced liver injury: Biomarkers, requirements, candidates, and validation. Front Pharmacol. 2019;10:1482. doi: 10.3389/fphar.2019.01482
- Kotsampasakou E, Ecker GF. Predicting drug-induced cholestasis with the help of hepatic transporters-an in silico modelling approach. J Chem Inf Model. 2017;57(3):608-615. doi: 10.1021/acs.jcim.6b00518
- Aleo MD, Shah F, Allen S, et al. Moving beyond binary predictions of human drug-induced liver injury (DILI) toward contrasting relative risk potential. Chem Res Toxicol. 2020;33(1):223-328. doi: 10.1021/acs.chemrestox.9b00262
- Cook D, Brown D, Alexander R, et al. Lessons learned from the fate of AstraZeneca’s drug pipeline: A five-dimensional framework. Nat Rev Drug Discov. 2014;13:419-431. doi: 10.1038/nrd4309
- Van Norman GA. Limitations of animal studies for predicting toxicity in clinical trials: Part 2: Potential alternatives to the use of animals in preclinical trials. JACC Basic Transl Sci. 2019;4(7):845-854. doi: 10.1016/j.jacbts.2020.03.010
- Van de Vliet M, Joossens M. The resemblance between bacterial gut colonization in pigs and humans. Microorganisms. 2022;10(9):1831. doi: 10.3390/microorganisms10091831
- Rao M, Nassiri V, Alhambra C, et al. AI/ML models to predict the severity of drug-induced liver injury for small molecules. Chem Res Toxicol. 2023;36(7):1129-1139. doi: 10.1021/acs.chemrestox.3c00098
- Singh S, Gupta H, Sharma P, Sahi S. Advances in Artificial Intelligence (AI)-assisted approaches in drug screening. Artif Intell Chem. 2024;2(1):100039. doi: 10.1016/j.aichem.2023.100039
- Paul D, Sanap G, Shenoy S, Kalyane D, Kalia K, Tekade RK. Artificial intelligence in drug discovery and development. Drug Discov Today. 2021;26(1):80-93. doi: 10.1016/j.drudis.2020.10.010
- Visan AI, Negut I. Integrating artificial intelligence for drug discovery in the context of revolutionizing drug delivery. Life (Basel). 2024;14:233. doi: 10.3390/life14020233
- Blanco-González A, Cabezón A, Seco-González A, et al. The role of AI in drug discovery: Challenges, opportunities, and strategies. Pharmaceuticals (Basel). 2023;16(6):891. doi: 10.3390/ph16060891
- Hasselgren C, Oprea TI. Artificial intelligence for drug discovery: Are we there yet? Annu Rev Pharmacol Toxicol. 2024;64:527-550. doi: 10.1146/annurev-pharmtox-040323-040828
- Qiu X, Li H, Steeg GV, Godzik A. Advances in AI for protein structure prediction: Implications for cancer drug discovery and development. Biomolecules. 2024;14(3):339. doi: 10.3390/biom14030339
- Janet JP, Mervin L, Engkvist O. Artificial intelligence in molecular de novo design: Integration with experiment. Curr Opin Struct Biol. 2023;80:102575. doi: 10.1016/j.sbi.2023.102575
- Wang M, Wang Z, Sun H, et al. Deep learning approaches for de novo drug design: An overview. Curr Opin Struct Biol. 2022;72:135-144. doi: 10.1016/j.sbi.2021.10.001
- Corso G, Stärk H, Jing B, Barzilay R, Jaakkola T. DiffDock: Diffusion steps, twists and turns for molecular docking. Conference paper at ICLR; 2023. doi: 10.48550/arXiv.2210.01776
- Turon G, Hlozek J, Woodland JG, Kumar A, Chibale K, Duran-Frigola M. First fully-automated AI/ML virtual screening cascade implemented at a drug discovery centre in Africa. Nat Commun. 2023;14(1):5736. doi: 10.1038/s41467-023-41512-2
- Zhou G, Rusnac DV, Park H, et al. An artificial intelligence accelerated virtual screening platform for drug discovery. Nat Commun. 2024;15:7761. doi: 10.1038/s41467-024-52061-7
- Yan Y, Zhao Y, Yao H, et al. RPBP: Deep retrosynthesis reaction prediction based on byproducts. J Chem Inf Model. 2023;63(19):5956-5970. doi: 10.1021/acs.jcim.3c00274
- Jiang Y, Yu Y, Kong M, et al. Artificial Intelligence for retrosynthesis prediction. Engineering. 2023;25:32-50. doi: 10.1016/j.eng.2022.04.021
- Tran TTV, Surya Wibowo A, Tayara H, Chong KT. Artificial intelligence in drug toxicity prediction: Recent advances, challenges, and future perspectives. J Chem Inf Model. 2023;63(9):2628-2643. doi: 10.1021/acs.jcim.3c00200
- Lin Z, Chou WC. Machine learning and artificial intelligence in toxicological sciences. Toxicol Sci. 2022;189(1):7-19. doi: 10.1093/toxsci/kfac075
- Askin S, Burkhalter D, Calado G, El Dakrouni S. Artificial intelligence applied to clinical trials: Opportunities and challenges. Health Technol (Berl). 2023;13(2):203-213. doi: 10.1007/s12553-023-00738-2
- Vo TH, Nguyen NTK, Le NQK. Improved prediction of drug-drug interactions using ensemble deep neural networks. Med Drug Discov. 2023;17:100149. doi: 10.1016/j.medidd.2022.100149
- Pun FW, Ozerov IV, Zhavoronkov A. AI-powered therapeutic target discovery. Trends Pharmacol Sci. 2023;44(9):561-572. doi: 10.1016/j.tips.2023.06.010
- Vitali E, Ficarelli F, Bisson M, et al. GPU-optimized approaches to molecular docking-based virtual screening in drug discovery: A comparative analysis. J Parallel Distrib Comput. 2024;186:104819. doi: 10.1016/j.jpdc.2023.104819
- Santos-Martins D, Solis-Vasquez L, Tillack AF, Sanner MF, Koch A, Forli S. Accelerating AutoDock4 with GPUs and gradient-based local search. J Chem Theory Comput. 2021;17(2):1060-1073. doi: 10.1021/acs.jctc.0c01006
- Jumper J, Evans R, Pritzel A, et al. Highly accurate protein structure prediction with AlphaFold. Nature. 2021;596:583-589. doi: 10.1038/s41586-021-03819-2
- Díaz-Rovira AM, Martín H, Beuming T, Díaz L, Guallar V, Ray SS. Are deep learning structural models sufficiently accurate for virtual screening? Application of docking algorithms to AlphaFold2 predicted structures. J Chem Inf Model. 2023;63:1668-1674. doi: 10.1021/acs.jcim.2c01270
- Yang Z, Zeng X, Zhao Y, Chen R. AlphaFold2 and its applications in the fields of biology and medicine. Signal Transduct Target Ther. 2023;8:115. doi: 10.1038/s41392-023-01381-z
- Yang H, Sun L, Li W, Liu G, Tang Y. Corrigendum: In silico prediction of chemical toxicity for drug design using machine learning methods and structural alerts. Front Chem. 2018;6:129. doi: 10.3389/fchem.2018.00129
- Atas Guvenilir H, Doğan T. How to approach machine learning-based prediction of drug/compound-target interactions. J Cheminform. 2023;15(1):16-36. doi: 10.1186/s13321-023-00689-w
- Sarker IH. Deep learning: A comprehensive overview on techniques, taxonomy, applications and research directions. SN Comput Sci. 2021;2:420. doi: 10.1007/s42979-021-00815-1
- Lin X. DeepGS: Deep representation learning of graphs and sequences for drug-target binding affinity prediction. arXiv. 2020. doi: 10.48550/arXiv.2003.13902
- Öztürk H, Özgür A, Ozkirimli E. DeepDTA: Deep drug-target binding affinity prediction. Bioinformatics. 2018;34(17):i821-i829. doi: 10.1093/bioinformatics/bty593
- Thafar MA, Alshahrani M, Albaradei S, Gojobori T, Essack M, Gao X. Affinity2Vec: Drug-target binding affinity prediction through representation learning, graph mining, and machine learning. Sci Rep. 2022;12(1):4751. doi: 10.1038/s41598-022-08787-9
- Powers AS, Yu HH, Suriana P, et al. Geometric deep learning for structure-based ligand design. ACS Cent Sci. 2023;9(12):2257-2267. doi: 10.1021/acscentsci.3c00572
- Askr H, Elgeldawi E, Aboul Ella H, Elshaier YA, Gomaa MM, Hassanien AE. Deep learning in drug discovery: An integrative review and future challenges. Artif Intell Rev. 2023;56(7):5975-6037. doi: 10.1007/s10462-022-10306-1
- Zhavoronkov A, Ivanenkov YA, Aliper A, et al. Deep learning enables rapid identification of potent DDR1 kinase inhibitors. Nat Biotechnol. 2019;37:1038-1040. doi: 10.1038/s41587-019-0224-x
- Hargrave-Thomas E, Yu B, Reynisson J. Serendipity in anticancer drug discovery. World J Clin Oncol. 2012;3(1):1-6. doi: 10.5306/wjco.v3.i1.1
- Dwivedi YK, Kshetri N, Hughes L, et al. Opinion Paper: “So what if ChatGPT wrote it?” Multidisciplinary perspectives on opportunities, challenges and implications of generative conversational AI for research, practice and policy. Int J Inf Manag. 2023;71:102642. doi: 10.1016/j.ijinfomgt.2023.102642
- Jayatunga MK, Ayers M, Bruens L, Jayanth D, Meier C. How successful are AI-discovered drugs in clinical trials? A first analysis and emerging lessons. Drug Discov Today. 2024;29(6):104009. doi: 10.1016/j.drudis.2024.104009