Prognostic evaluation using radiomics after stereotactic body radiotherapy in early-stage lung cancer
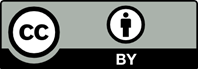
Non-small cell lung cancer (NSCLC), the leading cause of cancer-related deaths, is the most common subtype of lung cancer with an incidence of 85%. Stereotactic body radiotherapy (SBRT) is a curative treatment option for patients with early-stage NSCLC who cannot undergo surgery due to medical reasons or who refuse surgery. Radiomics non-invasively extracts advanced imaging features invisible to the human eye from medical images. Radiomics has prognostic value in predicting oncological outcomes after lung SBRT. Although studies on this subject are available in the literature, they are quite heterogeneous. There is a need for large-scale multicenter studies in which standard imaging techniques are used to obtain radiomic features, artificial intelligence-based segmentations are used to eliminate differences between contours, and SBRT dose schemes with appropriate therapeutic indexes are applied. This review aimed to interpret the existing studies and emphasize the clinical importance of radiomics, which can contribute to personalized treatment. A comprehensive literature search was conducted through the PubMed database using a wide range of keywords, which yielded 11 peer-reviewed articles published between 2017 and 2024. Seven articles evaluated computed tomography radiomics, and four evaluated fluorodeoxyglucose positron emission tomography-computed tomography radiomics. Oncological outcomes are not always identical in patients with a similar history receiving similar treatments at the same stage and age. Clinical, demographic, or treatment-related data are insufficient to predict prognosis and determine personalized treatment. Incorporating radiomics to these data can help establish models with higher accuracy and achieve personalized treatment.
- Chang JY, Mehran RJ, Feng L, et al. Stereotactic ablative radiotherapy for operable stage I non-small-cell lung cancer (revised STARS): Long-term results of a single-arm, prospective trial with prespecified comparison to surgery. Lancet Oncol. 2021;22(10):1448-1457. doi: 10.1016/S1470-2045(21)00401-0
- Li C, Wang L, Wu Q, et al. A meta-analysis comparing stereotactic body radiotherapy vs conventional radiotherapy in inoperable stage I non-small cell lung cancer. Medicine (Baltimore). 2020;99(34):e21715. doi: 10.1097/MD.0000000000021715
- Jameson JL, Longo DL. Precision medicine-- personalized, problematic, and promising. N Engl J Med. 2015;372(23):2229-2234. doi: 10.1056/NEJMsb1503104
- Bertolini M, Trojani V, Botti A, et al. Novel harmonization method for multi-centric radiomic studies in non-small cell lung cancer. Curr Oncol. 2022;29(8):5179-5194. doi: 10.3390/curroncol29080410
- Lambin P, Rios-Velazquez E, Leijenaar R, et al. Radiomics: Extracting more information from medical images using advanced feature analysis. Eur J Cancer. 2012;48(4):441-446. doi: 10.1016/j.ejca.2011.11.036
- Fave X, Cook M, Frederick A, et al. Preliminary investigation into sources of uncertainty in quantitative imaging features. Comput Med Imaging Graph. 2015;44:54-61. doi: 10.1016/j.compmedimag.2015.04.006
- Zhao B, Tan Y, Tsai WY, et al. Reproducibility of radiomics for deciphering tumor phenotype with imaging. Sci Rep. 2016;6:23428. doi: 10.1038/srep23428
- Mackin D, Fave X, Zhang L, et al. Measuring computed tomography scanner variability of radiomics features. Invest Radiol. 2015;50(11):757-65. doi: 10.1097/RLI.0000000000000180
- Huynh E, Coroller TP, Narayan V, et al. Associations of radiomic data extracted from static and respiratory-gated CT scans with disease recurrence in lung cancer patients treated with SBRT. PLoS One. 2017;12(1):e0169172. doi: 10.1371/journal.pone.0169172
- Kakino R, Nakamura M, Mitsuyoshi T, et al. Application and limitation of radiomics approach to prognostic prediction for lung stereotactic body radiotherapy using breath-hold CT images with random survival forest: A multi-institutional study. Med Phys. 2020;47(9):4634-4643. doi: 10.1002/mp.14380
- Sawayanagi S, Yamashita H, Nozawa Y, et al. Establishment body radiation therapy for primary non-small cell lung cancer using radiomics analysis. Cancers (Basel). 2022;14(16):3859. doi: 10.3390/cancers14163859
- Luo LM, Huang BT, Chen CZ, et al. A combined model to improve the prediction of local control for lung cancer patients undergoing stereotactic body radiotherapy based on radiomic signature plus clinical and dosimetric parameters. Front Oncol. 2022;11:819047. doi: 10.3389/fonc.2021.819047
- Isoyama-Shirakawa Y, Yoshitake T, Ninomiya K, et al. Combination of clinical factors and radiomics can predict local recurrence and metastasis after stereotactic body radiotherapy for non-small cell lung cancer. Anticancer Res. 2023;43(11):5003-5013. doi: 10.21873/anticanres.16699
- Lafata KJ, Hong JC, Geng R, et al. Association of pre-treatment radiomic features with lung cancer recurrence following stereotactic body radiation therapy. Phys Med Biol. 2019;64(2):025007. doi: 10.1088/1361-6560/aaf5a5
- Li Q, Kim J, Balagurunathan Y, Qi J, et al. CT imaging features associated with recurrence in non-small cell lung cancer patients after stereotactic body radiotherapy. Radiat Oncol. 2017;12(1):158. doi: 10.1186/s13014-017-0892-y
- Na F, Wang J, Li C, Deng L, Xue J, Lu Y. Primary tumor standardized uptake value measured on F18- Fluorodeoxyglucose positron emission tomography is of prediction value for survival and local control in non-small-cell lung cancer receiving radiotherapy: Meta-analysis. J Thorac Oncol. 2014;9(6):834-842. doi: 10.1097/JTO.0000000000000185
- Agarwal M, Brahmanday G, Bajaj SK, Ravikrishnan KP, Wong CY. Revisiting the prognostic value of preoperative (18)F-fluoro-2-deoxyglucose ((18)F-FDG) positron emission tomography (PET) in early-stage (I & II) non-small cell lung cancers (NSCLC). Eur J Nucl Med Mol Imaging. 2010;37(4):691-698. doi: 10.1007/s00259-009-1291-x
- Wu J, Aguilera T, Shultz D, et al. Early-stage non-small cell lung cancer: Quantitative imaging characteristics of (18)F fluorodeoxyglucose PET/CT allow prediction of distant metastasis. Radiology. 2016;281(1):270-278. doi: 10.1148/radiol.2016151829
- Pyka T, Bundschuh RA, Andratschke N, et al. Textural features in pre-treatment [F18]-FDG-PET/CT are correlated with risk of local recurrence and disease-specific survival in early stage NSCLC patients receiving primary stereotactic radiation therapy. Radiat Oncol. 2015;10:100. doi: 10.1186/s13014-015-0407-7
- Dissaux G, Visvikis D, Da-Ano R, et al. Pretreatment 18F-FDG PET/CT radiomics predict local recurrence in patients treated with stereotactic body radiotherapy for early-stage non-small cell lung cancer: A multicentric study. J Nucl Med. 2020;61(6):814-820. doi: 10.2967/jnumed.119.228106
- Oikonomou A, Khalvati F, Tyrrell PN, et al. Radiomics analysis at PET/CT contributes to prognosis of recurrence and survival in lung cancer treated with stereotactic body radiotherapy. Sci Rep. 2018;8(1):4003. doi: 10.1038/s41598-018-22357-y
- Lucia F, Louis T, Cousin F, et al. Multicentric development and evaluation of [18F]FDG PET/CT and CT radiomic models to predict regional and/or distant recurrence in early-stage non-small cell lung cancer treated by stereotactic body radiation therapy. Eur J Nucl Med Mol Imaging. 2024;51(4):1097-1108. doi: 10.1007/s00259-023-06510-y
- Nemoto H, Saito M, Satoh Y, et al. Evaluation of the performance of both machine learning models using PET and CT radiomics for predicting recurrence following lung stereotactic body radiation therapy: A single-institutional study. J Appl Clin Med Phys. 2024;25:e14322. doi: 10.1002/acm2.14322
- Zhang YP, Zhang XY, Cheng YT, et al. Artificial intelligence-driven radiomics study in cancer: The role of feature engineering and modeling. Mil Med Res. 2023;10(1):22. doi: 10.1186/s40779-023-00458-8
- Shen C, Liu Z, Guan M, et al. 2D and 3D CT radiomics features prognostic performance comparison in non-small cell lung cancer. Transl Oncol. 2017;10(6):886-894. doi: 10.1016/j.tranon.2017.08.007
- Zhu Y, Yao W, Xu BC, et al. Predicting response to immunotherapy plus chemotherapy in patients with esophageal squamous cell carcinoma using non-invasive radiomic biomarkers. BMC Cancer. 2021;21(1):1167. doi: 10.1186/s12885-021-08899-x
- Avanzo M, Wei L, Stancanello J, et al. Machine and deep learning methods for radiomics. Med Phys. 2020;47(5):e185-e202. doi: 10.1002/mp.13678
- Gao Y, Kalbasi A, Hsu W, et al. Treatment effect prediction for sarcoma patients treated with preoperative radiotherapy using radiomics features from longitudinal diffusion-weighted MRIs. Phys Med Biol. 2020;65(17):175006. doi: 10.1088/1361-6560/ab9e58
- Lorenz JW, Schott D, Rein L, et al. Serial T2-weighted magnetic resonance images acquired on a 1.5 tesla magnetic resonance linear accelerator reveal radiomic feature variation in organs at risk: An exploratory analysis of novel metrics of tissue response in prostate cancer. Cureus. 2019;11(4):e4510. doi: 10.7759/cureus.4510
- Mazzei MA, Nardone V, Di Giacomo L, et al. The role of delta radiomics in gastric cancer. Quant Imaging Med Surg. 2018;8(7):719-721. doi: 10.21037/qims.2018.07.08
- Ravanelli M, Agazzi GM, Tononcelli E, et al. Texture features of colorectal liver metastases on pretreatment contrast-enhanced CT may predict response and prognosis in patients treated with bevacizumab-containing chemotherapy: A pilot study including comparison with standard chemotherapy. Radiol Med. 2019;124(9):877-886. doi: 10.1007/s11547-019-01046-4
- Fave X, Zhang L, Yang J, et al. Delta-radiomics features for the prediction of patient outcomes in non-small cell lung cancer. Sci Rep. 2017;7(1):588. doi: 10.1038/s41598-017-00665-z
- Ma Y, Ma W, Xu X, Cao F. How does the delta-radiomics better differentiate pre-invasive GGNs from invasive GGNs? Front Oncol. 2020;10:1017. doi: 10.3389/fonc.2020.01017
- Nasief H, Zheng C, Schott D, et al. A machine learning based delta-radiomics process for early prediction of treatment response of pancreatic cancer. NPJ Precis Oncol. 2019;3:25. doi: 10.1038/s41698-019-0096-z
- Lin P, Yang PF, Chen S, et al. A Delta-radiomics model for preoperative evaluation of neoadjuvant chemotherapy response in high-grade osteosarcoma. Cancer Imaging. 2020;20(1):7. doi: 10.1186/s40644-019-0283-8
- Liu Y, Wu M, Zhang Y, et al. Imaging biomarkers to predict and evaluate the effectiveness of immunotherapy in advanced non-small-cell lung cancer. Front Oncol. 2021;11:657615. doi: 10.3389/fonc.2021.657615
- Colen RR, Rolfo C, Ak M, et al. Radiomics analysis for predicting pembrolizumab response in patients with advanced rare cancers. J Immunother Cancer. 2021;9(4):e001752. doi: 10.1136/jitc-2020-001752
- Fatima K, Dasgupta A, DiCenzo D, et al. Ultrasound delta-radiomics during radiotherapy to predict recurrence in patients with head and neck squamous cell carcinoma. Clin Transl Radiat Oncol. 2021;28:62-70. doi: 10.1016/j.ctro.2021.03.002
- Rao SX, Lambregts DM, Schnerr RS, et al. CT texture analysis in colorectal liver metastases: A better way than size and volume measurements to assess response to chemotherapy? United European Gastroenterol J. 2016;4(2):257-263. doi: 10.1177/2050640615601603
- Goh V, Ganeshan B, Nathan P, Juttla JK, Vinayan A, Miles KA. Assessment of response to tyrosine kinase inhibitors in metastatic renal cell cancer: CT texture as a predictive biomarker. Radiology. 2011;261(1):165-171. doi: 10.1148/radiol.11110264
- Cunliffe A, Armato SG 3rd, Castillo R, Pham N, Guerrero T, Al-Hallaq HA. Lung texture in serial thoracic computed tomography scans: Correlation of radiomics-based features with radiation therapy dose and radiation pneumonitis development. Int J Radiat Oncol Biol Phys. 2015;91(5):1048-1056. doi: 10.1016/j.ijrobp.2014.11.030
- Keskinbora KH. Medical ethics considerations on artificial intelligence. J Clin Neurosci. 2019;64:277-282. doi: 10.1016/j.jocn.2019.03.001
- IEEE Global Initiative. A Vision for Prioritizing Human Well-being with Artificial Intelligence and Autonomous Systems. In: The IEEE Global Initiative for Ethical Considerations in Artificial Intelligence and Autonomous Systems IEEE Glob Initiat Ethical Considerations. Vol. 13. 2016.