Leveraging summary of radiology reports with transformers
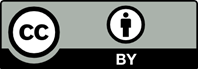
Two fundamental problems in health-care stem from patient handoff and triage. Doctors are often required to perform complex findings summarization to facilitate efficient communication with specialists and decision-making on the urgency of each case. To address these challenges, we present a state-of-the-art radiology report summarization model utilizing adjusted bidirectional encoder representation from transformers BERT-to-BERT encoder–decoder architecture. Our approach includes a novel method for augmenting medical data and a comprehensive performance analysis. Our best-performing model achieved a recall-oriented understudy for gisting evaluation-L F1 score of 58.75/100, outperforming specialized checkpoints with more sophisticated attention mechanisms. We also provide a data processing pipeline for future models developed on the MIMIC-chest X-ray dataset. The model introduced in this paper demonstrates significantly improved capacity in radiology report summarization, highlighting the potential for ensuring better clinical workflows and enhanced patient care.
- Takacs N, Makary MS. Are we Prepared for a Looming Radiologist Shortage? Radiology Today. Available from: https://www.radiologytoday.net/archive/rt0619p10.shtml [Last accessed on 2024 Jun 03].
- Devlin J, Chang MW, Lee K, Toutanova K. BERT: Pre-training of Deep Bidirectional Transformers for Language Understanding. arXiv preprint arXiv:1810.04805; 2019. Available from: https://aclanthology.org/N19-1423 [Last accessed on 2024 Jun 03].
- Johnson AEW, Pollard TJ, Shen L, et al. MIMIC-CXR-JPG, a large publicly available database of labeled chest radiographs. Nat Sci Data. 2019;24(1):1-18.
- De Padua RS, Qureshi I. Colab Notebook with Fine- Tuned T5 Model for Radiology Summarization. Available from: https://colab.research.google.com/ drive/14A3j4bsTiC3hh3GdbLxwWGtwZoFiwciv [Last accessed on 2024 Jun 03].
- Chen Z, Gong Z, Zhuk A. Predicting Doctor’s Impression for Radiology Reports with Abstractive Text Summarization. CS224N: Natural Language Processing with Deep Learning. Stanford University; 2021. Available from: https://web. stanford.edu/class/archive/cs/cs224n/cs224n.1214/reports/ final_reports/report005.pdf [Last accessed on 2024 Jun 03].
- Alsentzer E, Murphy JR, Boag W, et al. Publicly Available Clinical BERT Embeddings. In: Proceedings of the 2nd Clinical Natural Language Processing Workshop (ClinicalNLP); 2019. p. 72-78. Available from: https://aclanthology.org/W19- 1909 [Last accessed on 2024 Jun 03].
- Lin CY. ROUGE: A Package for Automatic Evaluation of Summaries. Text Summarization Branches Out (2004): 74-81. Barcelona, Spain: Association for Computational Linguistics. Proceedings of the ACL Workshop: Text Summarization Braches Out; 2004. p. 10.
- Raffel C, Shazeer N, Roberts A, et al. Exploring the limits of transfer learning with a unified text-to-text transformer. J Mach Learn Res. 2020;21(140):1-67.
- Li Y, Wehbe RM, Ahmad FS, Wang H, Luo Y. Clinical-longformer and clinical-bigbird: Transformers for long clinical sequences. J Am Med Inform Assoc. 2022;29(2):273-281. doi: 10.48550/arXiv.2201.11838
- Yalunin A, Umerenkov D, Kokh V. Abstractive Summarization of Hospitalisation Histories with Transformer Networks. doi: 10.48550/arXiv.2204.02208
- Kraljevic Z, Newham M, Fox D, et al. Multimodal representation learning for medical text summarization. J Biomed Inform. 2021;116:103713.
- Zhang T, Kishore V, Wu F, Weinberger KQ, Artzi Y. BERTScore: Evaluating Text Generation with BERT. In: International Conference on Learning Representations (ICLR); 2020. doi: 10.48550/arXiv.1904.09675
- Lewis M, Liu Y, Goyal N, et al. BART: Denoising Sequence-to-Sequence Pre-training for Natural Language Generation, Translation, and Comprehension. In: Proceedings of the 58th Annual Meeting of the Association for Computational Linguistics; 2020. p. 7871-7880. doi: 10.48550/arXiv.1910.13461
- Lamb AM, Goyal A, Zhang Y, Zhang S, Courville A, Bengio Y. Professor forcing: A new algorithm for training recurrent networks. Adv Neural Inform Process Syst. 2016;29:4601-4609. doi: 10.48550/arXiv.1610.09038
- Wolf T, Debut L, Sanh V, et al. Transformers: State-of-the- Art Natural Language Processing. In: Proceedings of the 2020 Conference on Empirical Methods in Natural Language Processing: System Demonstrations; 2020. p. 38-45. doi: 10.48550/arXiv.1910.03771
- Zaheer M, Guruganesh G, Dubey A, et al. Big bird: Transformers for longer sequences. Adv Neural Inform Process Syst. 2020;33:17283-17297. doi: 10.48550/arXiv.2007.14062
- Dahal P. Classification and Loss Evaluation - Softmax and Cross Entropy Loss. Available from: https://deepnotes.io/ softmax-crossentropy [Last accessed on 2024 Jun 03].
- Wolk K, Marasek K. Enhanced Bilingual evaluation understudy. arXiv preprint arXiv: 1509.09088; 2015. doi: 10.48550/arXiv.1509.09088
- Tay Y, Dehghani M, Bahri D, Metzler D. Efficient Transformers: A Survey. arXiv preprint arXiv:2009.06732; 2020. doi: 10.48550/arXiv.2009.06732
- Kaplan J, McCandlish S, Henighan T, et al. Scaling Laws for Neural Language Models. arXiv preprint arXiv:2001.08361; 2020. doi: 10.48550/arXiv.2001.08361
- Vig J. A Multiscale Visualization of Attention in the Transformer Model. In: Proceedings of the 57th Annual Meeting of the Association for Computational Linguistics: System Demonstrations; 2019. p. 37-42. doi: 10.48550/arXiv.1906.05714