Enhancing patient safety through integrated sensor technology and machine learning for bed-based patient movement detection in inpatient care
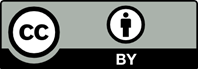
The occurrence of inpatient falls and new-onset seizures are common complications during hospital stays, posing risks to patient safety and potentially leading to prolonged hospital stays and further complications. Given the constraints on medical staff’s ability to provide constant monitoring due to their workload, the implementation of a sensor device equipped with machine learning capabilities to recognize and prevent these events becomes imperative. This study utilized data acquired through the Movella Xsens sensor, which detects real-time motions and 3D movements, in conjunction with the PyCaret machine-learning algorithm. Adult-sized and infant-sized mannequins were used to assess the algorithm’s ability in predicting specific movements associated with breathing, seizures, rolling to the right side, rolling to the left side, rolling off the bed from the left, and rolling off the bed from the right. The study achieved an overall 89% accuracy rate in detecting each specific movement using the combination of PyCaret and Xsens sensors. The application of PyCaret alongside Xsens sensors demonstrates promising results in accurately detecting movements, thereby mitigating falls and post-seizure complications in an inpatient setting, consequently improving patient safety. Further exploration of this technology holds the potential to revolutionize healthcare delivery by incorporating it into a trigger alert system capable of promptly warning medical staff of urgent situations through real-time capture and analysis of potentially harmful motions.
- LeLaurin JH, Shorr RI. Preventing falls in hospitalized patients: State of the science. Clin Geriatr Med. 2019;35(2):273-283. doi: 10.1016/j.cger.2019.01.007
- Centers for Disease Control and Prevention. Web-based Injury Statistics Query and Reporting System (WISQARS). National Center for Injury Prevention and Control, Centers for Disease Control and Prevention (Producer); 2023. Available from: https://www.cdc.gov/ncipc/wisqars [Last accessed on 2023 Dec 22].
- Eost-Telling C, Yang Y, Norman G, et al. Digital technologies to prevent falls in people living with dementia or mild cognitive impairment: A rapid systematic overview of systematic reviews. Age Ageing. 2024;53:afad238. doi: 10.1093/ageing/afad238
- Wang Y, Jiang M, He M, Du M. Design and implementation of an inpatient fall risk management information system. JMIR Med Inform. 2024;12:e46501. doi: 10.2196/46501
- Dykes PC, Burns Z, Adelman J, et al. Evaluation of a patient-centered fall-prevention tool kit to reduce falls and injuries: A nonrandomized controlled trial. JAMA Netw Open. 2020;3(11):e2025889. doi: 10.1001/jamanetworkopen.2020.25889
- Zubrinic M, Vrbanic L, Keshavjee S. Remote telemonitoring is associated with improved patient safety and decreased workload of nurses. JTCVS Open. 2023;16:493-497. doi: 10.1016/j.xjon.2023.09.014
- Morse JM. Preventing Patient Falls. Berlin: Springer Publishing Company; 2008.
- Mcvey L, Alvarado N, Zaman H, et al. Interactions that support older inpatients with cognitive impairments to engage with falls prevention in hospitals: An ethnographic study. J Clin Nurs. 2024;33:1884-1895. doi: 10.1111/jocn.17006
- Fields MC, Labovitz DL, French JA. Hospital-onset seizures: An inpatient study. JAMA Neurol. 2013;70(3):360-364. doi: 10.1001/2013.jamaneurol.337
- Tsai ER, Demirtas D, Hoogendijk N, Tintu AN, Boucherie RJ. Turnaround time prediction for clinical chemistry samples using machine learning. Clin Chem Lab Med. 2022;60(12):1902-1910. doi: 10.1515/cclm-2022-0668
- Evrimler S, Ali Gedik M, Ahmet Serel T, Ertunc O, Alperen Ozturk S, Soyupek S. Bladder urothelial carcinoma: Machine learning-based computed tomography radiomics for prediction of histological variant. Acad Radiol. 2022;29(11):1682-1689. doi: 10.1016/j.acra.2022.02.007
- Rye I, Vik A, Kocinski M, Lundervold AS, Lundervold AJ. Predicting conversion to Alzheimer’s disease in individuals with Mild Cognitive Impairment using clinically transferable features. Sci Rep. 2022;12(1):15566. doi: 10.1038/s41598-022-18805-5.
- Bekbolatova M, Mayer J, Ong CW, Toma M. Transformative potential of AI in healthcare: Definitions, applications, and navigating the ethical landscape and public perspectives. Healthcare. 2024;12(2):125. doi: 10.3390/healthcare12020125
- Toma M, Wei OC. Predictive modeling in medicine. Encyclopedia. 2023;3(2):590-601. doi: 10.3390/encyclopedia3020042
- Mayer J, Jose R, Kurgansky G, et al. Evaluating the feasibility of euler angles for bed-based patient movement monitoring. Signals. 2023;4(4):788-799. doi: 10.3390/signals4040043
- Chou CY, Hsu DY, Chou CH. Predicting the onset of diabetes with machine learning methods. J Pers Med. 2023;13(3):406. doi: 10.3390/jpm13030406
- Abraham A, Jose R, Ahmad J, et al. Comparative analysis of machine learning models for image detection of colonic polyps vs. Resected polyps. J Imaging. 2023;9(10):215. doi: 10.3390/jimaging9100215
- Jose R, Syed F, Thomas A, Toma M. Cardiovascular health management in diabetic patients with machine-learning-driven predictions and interventions. Appl Sci. 2024;14(5):2132. doi: 10.3390/app14052132
- Jose R, Thomas A, Guo J, Steinberg R, Toma M. Evaluating machine learning models for prediction of coronary artery disease. Glob Transl Med. 2024;3(1):2669. doi: 10.36922/gtm.2669
- Freitas ME, Ruiz-Lopez M, Dalmau J, et al. Seizures and movement disorders: Phenomenology, diagnostic challenges and therapeutic approaches. J Neurol Neurosurg Psychiatry. 2019;90(8):920-928. doi: 10.1136/jnnp-2018-320039
- Song W, Latham NK, Liu L, et al. Improved accuracy and efficiency of primary care fall risk screening of older adults using a machine learning approach. J Am Geriatr Soc. 2024;72:1145-1154. doi: 10.1111/jgs.18776
- Durán-Vega LA, Santana-Mancilla PC, Buenrostro-Mariscal R, et al. An IoT system for remote health monitoring in elderly adults through a wearable device and mobile application. Geriatrics (Basel). 2019;4(2):34. doi: 10.3390/geriatrics4020034
- Oliver D, Healey F, Haines TP. Preventing falls and fall-related injuries in hospitals. Clin Geriatr Med. 2010;26(4):645-692. doi: 10.1016/j.cger.2010.06.005
- Majidi SA, Fakoorfard Z, Safarmohammadi H, Kazemnezhad Leily E. The relationship between moral intelligence and patient safety culture in nurses. J Caring Sci. 2023;12: 241-247. doi: 10.34172/jcs.2023.30501
- Hiyama A. Using the analytic hierarchy process to measure nurses’ decision-making regarding fall risks and care strategies for fall prevention. J Nurs Meas. 2024;31: 1113-1125. doi: 10.1891/JNM-2023-0006
- Orts-Cortés MI, Cabañero-Martínez MJ, Meseguer-Liza C, Arredondo-González CP, de la Cuesta-Benjumea C, Abad- Corpa E. Effectiveness of nursing interventions in the prevention of falls in older adults in the community and in health care settings: A systematic review and meta-analysis of RCT. Enferm Clín (Engl Ed). 2024;34:4-13. doi: 10.1016/j.enfcle.2024.01.001
- Abbe JR, O’Keeffe C. Continuous video monitoring: Implementation strategies for safe patient care and identified best practices. J Nurs Care Qual. 2021;36(2):137-142. doi: 10.1097/NCQ.0000000000000502
- Camp K, Murphy S, Pate B. Integrating fall prevention strategies into EMS services to reduce falls and associated healthcare costs for older adults. Clin Interv Aging. 2024;19:561-569. doi: 10.2147/CIA.S453961
- Wabe N, Meulenbroeks I, Huang G, et al. Development and internal validation of a dynamic fall risk prediction and monitoring tool in aged care using routinely collected electronic health data: A landmarking approach. J Am Med Inform Assoc. 2024:ocae058. doi: 10.1093/jamia/ocae058
- Coleman A. Reducing falls among residents of retirement homes: A DNP project. Nurse Pract. 2024;49(4):39-47. doi: 10.1097/01.NPR.0000000000000161
- Lee-Confer J. Strength in arms: Empowering older adults against the risk of slipping and falling-a theoretical perspective. Front Sports Act Living. 2024;6:1371730. doi: 10.3389/fspor.2024.1371730
- Xu D, Wang Y, Zhu S, Zhao M, Wang K. Relationship between fear of falling and quality of life in nursing home residents: The role of activity restriction. Geriatr Nurs. 2024;57:45-50. doi: 10.1016/j.gerinurse.2024.03.006