Artificial intelligence in diagnosis and monitoring of atopic dermatitis: From pixels to predictions
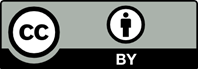
In any ailment, the identification of the symptoms, detection, and diagnosis plays a pivotal role in treatment and therapy. However, certain diseases share similar symptoms, lacking signature key indicators, which can lead to fallacious or incorrect inferences. Skin disorders, such as pruritus, dermatitis, eczema, psoriasis, and ichthyosis, all present similar symptoms, which confound clinicians. One such commonly misunderstood condition is atopic dermatitis (AD), a chronic inflammatory skin condition characterized by its relapsing nature, which heightens the importance of diagnosis and disease monitoring for effective management. Recent strides in artificial intelligence (AI) have opened avenues for precise diagnosis and continuous monitoring of AD. This review explores and evaluates current applications of AI in the diagnosis and monitoring of individuals with AD emphasizing the need to address challenges and collaborate across intra-, inter-, trans-, and multi-disciplinary domains to maximize the benefits of AI in enhancing the precision of AD diagnosis, ultimately leading to improved patient care and satisfaction through technologically-driven biomedical tools in customized healthcare.
- Huchegowda R, Huchegowda S, Pramer J, et al. Review on artificial intelligence and applications in healthcare. Neuro Open J. 2019;6(1):e1-e4. doi: 10.17140/noj-6-e010
- Lopez-Jimenez F, Attia Z, Arruda-Olson AM, et al. Artificial intelligence in cardiology: Present and future. Mayo Clin Proc. 2020;95(5):1015-1039. doi: 10.1016/j.mayocp.2020.01.038
- Rajpurkar P, Chen E, Banerjee O, Topol EJ. AI in health and medicine. Nat Med. 2022;28(1):31-38. doi: 10.1038/s41591-021-01614-0
- Cichocki A. Chief Editor’s foreword to the inaugural issue of artificial intelligence in health. AIH. 2024;1(1):2463. doi: 10.36922/aih.2463
- Esteva A, Kuprel B, Novoa RA, et al. Dermatologist-level classification of skin cancer with deep neural networks. Nature. 2017;542(7639):115-118. doi: 10.1038/nature21056
- DaVeiga SP. Epidemiology of atopic dermatitis: A review. Allergy Asthma Proc. 2012;33(3):227-234. doi: 10.2500/aap.2012.33.3569
- Williams H, Stewart A, Von Mutius E, Cookson W, Anderson HR, International Study of Asthma and Allergies in Childhood (ISAAC) Phase One and Three Study Groups. Is eczema really on the increase worldwide? J Allergy Clin Immunol. 2008;121(4):947-954.e15. doi: 10.1016/j.jaci.2007.11.004
- Furue M, Chiba T, Takeuchi S. Current status of atopic dermatitis in Japan. Asia Pac Allergy. 2011;1(2):64-72. doi: 10.5415/apallergy.2011.1.2.64
- Williams HC, Strachan DP. The natural history of childhood eczema: Observations from the British 1958 birth cohort study. Br J Dermatol. 1998;139(5):834-839. doi: 10.1046/j.1365-2133.1998.02501.x
- Paternoster L, Savenije OE, Heron J, et al. Identification of atopic dermatitis subgroups in children from 2 longitudinal birth cohorts. J Allergy Clin Immunol. 2018;141(3):964-971. doi: 10.1016/j.jaci.2017.09.044
- Kiebert G, Sorensen SV, Revicki D, et al. Atopic dermatitis is associated with a decrement in health-related quality of life. Int J Dermatol. 2002;41(3):151-158. doi: 10.1046/j.1365-4362.2002.01436.x
- Furue M, Chiba T, Tsuji G, et al. Atopic dermatitis: Immune deviation, barrier dysfunction, IgE autoreactivity and new therapies. Allergol Int. 2017;66(3):398-403. doi: 10.1016/j.alit.2016.12.002
- Nakahara T, Fujita H, Arima K, Taguchi Y, Motoyama S, Furue M. Treatment satisfaction in atopic dermatitis relates to patient-reported severity: A cross-sectional study. Allergy. 2019;74(6):1179-1181. doi: 10.1111/all.13712
- Furue M, Onozuka D, Takeuchi S, et al. Poor adherence to oral and topical medication in 3096 dermatological patients as assessed by the Morisky Medication Adherence Scale‐8. Br J Dermatol. 2014;172(1):272-275. doi: 10.1111/bjd.13377
- Murota H, Takeuchi S, Sugaya M, et al. Characterization of socioeconomic status of Japanese patients with atopic dermatitis showing poor medical adherence and reasons for drug discontinuation. J Dermatol Sci. 2015;79(3):279-287. doi: 10.1016/j.jdermsci.2015.05.010
- Takeuchi S, Oba J, Esaki H, Furue, M. Non-corticosteroid adherence and itch severity influence perception of itch in atopic dermatitis. J Dermatol. 2017;45(2):158-164. doi: 10.1111/1346-8138.14124
- Furue M, Iida K, Imaji M, Nakahara T. Microbiome analysis of forehead skin in patients with atopic dermatitis and healthy subjects: Implication of Staphylococcus and Corynebacterium. J Dermatol. 2018;45(7):876-877. doi: 10.1111/1346-8138.14486
- Iwamoto K, Moriwaki M, Miyake R, Hide M. Staphylococcus aureus in atopic dermatitis: Strain-specific cell wall proteins and skin immunity. Allergol Int. 2019;68(3):309-315. doi: 10.1016/j.alit.2019.02.006
- Furue M, Kadono T. “Inflammatory skin march” in atopic dermatitis and psoriasis. J Inflamm Res. 2017;66(10):833-842. doi: 10.1007/s00011-017-1065-z
- Emam S, Du AX, Surmanowicz P, Thomsen SF, Greiner R, Gniadecki R. Predicting the long-term outcomes of biologics in patients with psoriasis using machine learning. Br J Dermatol. 2020;182(5):1305-1307. doi: 10.1111/bjd.18741
- Pierce EJ, Boytsov NN, Vasey JJ, et al. A qualitative analysis of provider notes of atopic dermatitis-related visits using natural language processing methods. Dermatol Ther (Heidelb). 2021;11(4):1305-1318. doi: 10.1007/s13555-021-00553-5
- LeCun Y, Bengio Y, Hinton G. Deep learning. Nature. 2015;521(7553):436-444. doi: 10.1038/nature14539
- Esteva A, Robicquet A, Ramsundar B, et al. A guide to deep learning in healthcare. Nat Med. 2019;25(1):24-29. doi: 10.1038/s41591-018-0316-z
- Wolf T, Debut L, Sanh V, et al. Transformers: State-of-the- Art Natural Language Processing. In: Proceedings of the 2020 Conference on Empirical Methods in Natural Language Processing: System Demonstrations; 2020. p. 38-45. doi: 10.18653/v1/2020.emnlp-demos.6
- Gu J, Wang Z, Kuen J, et al. Recent advances in convolutional neural networks. Pattern Recognit. 2018;77:354-377. doi: 10.1016/j.patcog.2017.10.013
- Nadkarni PM, Ohno-Machado L, Chapman WW. Natural language processing: An introduction. J Am Med Inform Assoc. 2011;18(5):544-551. doi: 10.1136/amiajnl-2011-000464
- Introducing ChatGPT. Released on November by 2022 OpenAI. Available from: https://chat.openai.com [Last accessed on 2024 Jan 05].
- Moor M, Huang Q, Wu S, et al. Med-Flamingo: A Multimodal Medical Few-Shot Learner. In: Machine Learning for Health (ML4H); 2023. p. 353-367. Available from: https:// proceedings.mlr.press/v225/moor23a.html
- Li C. LLaVA-Med: Training a Large Language-and-Vision Assistant for Biomedicine in One Day. In: Advances in Neural Information Processing Systems; 2023. p. 36
- Tu T. Towards Generalist Biomedical AI; 2023. Available from: https://arxiv.org/abs/2307.14334 [Last accessed on 2024 Jan 05].
- Zhu D, Chen J, Shen X, Li X, Elhoseiny M. MiniGPT-4: Enhancing Vision-Language Understanding with Advanced Large Language Models. arXiv:2304 [Preprint]; 2023. doi: 10.48550/arXiv.2304.10592
- Bommasani R, Hudson DA, Adeli E, et al. On the Opportunities and Risks of Foundation Models. arXiv:2108. [Preprint]; 2021. doi: 10.48550/arXiv.2108.07258
- Dev K, Ho CJ, Bi R, et al. Machine learning assisted handheld confocal raman micro-spectroscopy for identification of clinically relevant atopic eczema biomarkers. Sensors (Basel). 2022;22(13):4674. doi: 10.3390/s22134674
- Guimarães P, Batista A, Zieger M, Kaatz M, Koenig K. Artificial intelligence in multiphoton tomography: Atopic dermatitis diagnosis. Sci Rep. 2020;10(1):7968. doi: 10.1038/s41598-020-64937-x
- Nomura I, Tanaka K, Tomita H, et al. Evaluation of the Staphylococcal exotoxins and their specific IgE in childhood atopic dermatitis. J Allergy Clin Immunol. 1999;104(2):441-446. doi: 10.1016/s0091-6749(99)70390-8
- Orfali RL, Sato MN, Santos VG, et al. Staphylococcal enterotoxin B induces specific IgG4 and IgE antibody serum levels in atopic dermatitis. Int J Dermatol. 2014;54(8):898-904. doi: 10.1111/ijd.12533
- Kakinuma T, Nakamura K, Wakugawa M, et al. Thymus and activation-regulated chemokine in atopic dermatitis: Serum thymus and activation-regulated chemokine level is closely related with disease activity. J Allergy Clin Immunol. 2001;107(3):535-541. doi: 10.1067/mai.2001.113237
- Hijnen D, De Bruin-Weller M, Oosting B, et al. Serum thymus and activation-regulated chemokine (TARC) and cutaneous T cell-attracting chemokine (CTACK) levels in allergic diseases TARC and CTACK are disease-specific markers for atopic dermatitis. J Allergy Clin Immunol. 2004;113(2):334-340. doi: 10.1016/j.jaci.2003.12.007
- Thijs JL, Nierkens S, Herath A, et al. A panel of biomarkers for disease severity in atopic dermatitis. Clin Exp Allergy. 2015;45(3):698-701. doi: 10.1111/cea.12486
- Krishnan A, Woo CW, Chang LJ, et al. Somatic and vicarious pain are represented by dissociable multivariate brain patterns. Elife. 2016;5:e15166. doi: 10.7554/elife.15166
- Wager TD, Atlas LY, Lindquist MA, Roy M, Woo CW, Kross E. An fMRI-based neurologic signature of physical pain. N Engl J Med. 2013;368(15):1388-1397. doi: 10.1056/nejmoa1204471
- Lee IS, Yeom M, Kim K, Hahm DH, Kang S, Park HJ. Prediction of disease severity using serum biomarkers in patients with mild-moderate atopic dermatitis: A pilot study. PLoS One. 2023;18(11):e0293332. doi: 10.1371/journal.pone.0293332
- Kang S, Kim YK, Yeom M, et al. Acupuncture improves symptoms in patients with mild-to-moderate atopic dermatitis: A randomized, sham-controlled preliminary trial. Complement Ther Med. 2018;41:90-98. doi: 10.1016/j.ctim.2018.08.013
- Kim J, Kwon SK, Lee IS, et al. Effect of acupuncture on gut-brain axis parameters in patients with atopic dermatitis: A study protocol for a randomized, participant-and assessor-blind, sham-controlled trial. Evid Based Complement Alternat Med. 2021;2021:5584247. doi: 10.1155/2021/5584247
- Litvak Y, Byndloss MX, Bäumler AJ. Colonocyte metabolism shapes the gut microbiota. Science. 2018;362(6418):eaat9076. doi: 10.1126/science.aat9076
- Ghosh D, Bernstein JA, Khurana Hershey GK, Rothenberg ME, Mersha TB. Leveraging multilayered “Omics” data for atopic dermatitis: A road map to precision medicine. Front. Immunol. 2018;9:2727. doi: 10.3389/fimmu.2018.02727
- Sacco KA, Milner JD. Gene-environment interactions in primary atopic disorders. Curr Opin Immunol. 2019;60:148-155. doi: 10.1016/j.coi.2019.06.002
- Lloyd-Price J, Arze C, Ananthakrishnan AN, et al. Multi-omics of the gut microbial ecosystem in inflammatory bowel diseases. Nature. 2019;569(7758):655-662. doi: 10.1038/s41586-019-1237-9
- Kang MJ, Lee SY, Park YM, et al. Interactions between IL-17 variants and Streptococcus in the gut contribute to the development of atopic dermatitis in infancy. Allergy Asthma Immunol Res. 2021;13(3):404-149. doi: 10.4168/aair.2021.13.3.404
- Jiang Z, Li J, Kong N, et al. Accurate diagnosis of atopic dermatitis by combining transcriptome and microbiota data with supervised machine learning. Sci Rep. 2022;12:290. doi: 10.1038/s41598-021-04373-7
- Dautović A, Dondras B, Dervisbegovic F, et al. Diagnosis of atopic dermatitis using artificial neural network. IFAC Pap OnLine. 2022;55(4):51-55. doi: 10.1016/j.ifacol.2022.06.008
- Pan K, Hurault G, Arulkumaran K, Williams HC, Tanaka RJ. EczemaNet: A Detection and Severity Assessment of Atopic Dermatitis. In: International Workshop on Machine Learning in Medical Imaging; 2020. p. 220-230. doi: 10.1007/978-3-030-59861-7_23
- Padilla D, Yumang A, Diaz AL, Inlong G. Differentiating Atopic Dermatitis and Psoriasis Chronic Plaque using Convolutional Neural Network MobileNet Architecture. In: IEEE 11th International Conference on Humanoid, Nanotechnology, Information Technology, Communication and Control, Environment, and Management (HNICEM); 2019. p. 1-6.
- Patella V, Florio G, Palmieri M, et al. Atopic dermatitis severity during exposure to air pollutants and weather changes with an artificial neural network (ANN) analysis. Pediatr Allergy Immunol. 2020;31(8):938-945. doi: 10.1111/pai.13314
- Slattery MJ, Essex MJ, Paletz EM, et al. Depression, anxiety, and dermatologic quality of life in adolescents with atopic dermatitis. J Allergy Clin Immunol. 2011;128(3):668-671. doi: 10.1016/j.jaci.2011.05.003
- Yaghmaie P, Koudelka CW, Simpson EL. Mental health comorbidity in patients with atopic dermatitis. J Allergy Clin Immunol. 2013;131(2):428-433. doi: 10.1016/j.jaci.2012.10.041
- Thyssen JP, Hamann CR, Linneberg A, et al. Atopic dermatitis is associated with anxiety, depression, and suicidal ideation, but not with psychiatric hospitalization or suicide. Allergy. 2017;73(1):214-220. doi: 10.1111/all.13231
- Silverberg JI. Practice gaps in pruritus. Dermatol Clin. 2016;34(3):257-261. doi: 10.1016/j.det.2016.02.008
- Yosipovitch G, Reaney M, Mastey V, et al. Peak pruritus numerical rating scale: Psychometric validation and responder definition for assessing itch in moderate‐to‐severe atopic dermatitis. Br J Dermatol. 2019;181(4):761-769. doi: 10.1111/bjd.17744
- Ikoma A, Rukwied R, Ständer S, Steinhoff M, Miyachi Y, Schmelz M. Neuronal sensitization for histamine-induced itch in lesional skin of patients with atopic dermatitis. Arch Dermatol. 2003;139(11):1455-1458. doi: 10.1001/archderm.139.11.1455
- Ikoma A, Steinhoff M, Ständer S, Yosipovitch G, Schmelz M. The neurobiology of itch. Nat Rev Neurosci. 2006;7(7):535-547. doi: 10.1038/nrn1950
- Paus R, Schmelz M, Biro T, Steinhoff M. Frontiers in pruritus research: Scratching the brain for more effective itch therapy. J Clin Invest. 2006;116(5):1174-1186. doi: 10.1172/jci28553
- Potenzieri C, Undem BJ. Basic mechanisms of itch. Clin Exp Allergy. 2012;42(1):8-19. doi: 10.1111/j.1365-2222.2011.03791.x
- Sanders KM, Nattkemper LA, Yosipovitch G. Advances in understanding itching and scratching: a new era of targeted treatments. F1000Res. 2016;5:2042. doi: 10.12688/f1000research.8659.1
- Weisshaar E, Gieler U, Kupfer J, et al. Questionnaires to assess chronic itch: A consensus paper of the special interest group of the international forum on the study of itch. Acta Derm Venereol. 2012;92(5):493-496. doi: 10.2340/00015555-1402
- Ständer S, Augustin M, Reich A, et al. Pruritus assessment in clinical trials: Consensus recommendations from the international forum for the study of itch (IFSI) special interest group scoring itch in clinical trials. Acta Derm Venereol. 2013;93(5):509-514. doi: 10.2340/00015555-1620
- Umeda K, Noro Y, Murakami T, et al. A novel acoustic evaluation system of scratching in mouse dermatitis: Rapid and specific detection of invisibly rapid scratch in an atopic dermatitis model mouse. Life Sci. 2006;79(22):2144-2150. doi: 10.1016/j.lfs.2006.07.010
- Noro Y, Omoto Y, Umeda K, et al. Novel acoustic evaluation system for scratching behavior in itching dermatitis: Rapid and accurate analysis for nocturnal scratching of atopic dermatitis patients. J Dermatol. 2014;41(3):233-238. doi: 10.1111/1346-8138.12405
- Lu TC, Fu CM, Ma M, Fang CC, Turner A. Healthcare applications of smart watches. Appl Clin Inform. 2016;7(3):850-869. doi: 10.4338/aci-2016-03-r-0042
- Reeder B, David A. Health at hand: A systematic review of smart watch uses for health and wellness. J Biomed Inform. 2016;63:269-276. doi: 10.1016/j.jbi.2016.09.001
- Lee J, Cho D, Song S, Kim S, Im E, Kim J. Mobile System Design for Scratch Recognition. In: Proceedings of the 33rd Annual ACM Conference Extended Abstracts on Human Factors in Computing Systems; 2015. p. 1567-1572. doi: 10.1145/2702613.2732820
- Ikoma A, Ebata T, Chantalat L, et al. Measurement of nocturnal scratching in patients with pruritus using a smartwatch: Initial clinical studies with the itch tracker app. Acta Derm Venereol. 2019;99(3):268-273. doi: 10.2340/00015555-3105
- Reich A, Szepietowski JC. Pruritus intensity assessment: Challenge for clinicians. Expert Rev Dermatol. 2013;8(3):291-299. doi: 10.1586/edm.13.2
- Schneider G, Ständer S, Burgmer M, Driesch G, Heuft G, Weckesser M. Significant differences in central imaging of histamine-induced itch between atopic dermatitis and healthy subjects. Eur J Pain. 2008;12(7):834-841. doi: 10.1016/j.ejpain.2007.12.003
- Ishiuji Y, Coghill R, Patel T, Oshiro Y, Kraft R, Yosipovitch G. Distinct patterns of brain activity evoked by histamine-induced itch reveal an association with itch intensity and disease severity in atopic dermatitis. Br J Dermatol. 2009;161(5):1072-1080. doi: 10.1111/j.1365-2133.2009.09308.x
- Papoiu AD, Nattkemper LA, Sanders KM, et al. Brain’s reward circuits mediate itch relief. A functional MRI study of active scratching. PLoS One. 2013;8(12):e82389. doi: 10.1371/journal.pone.0082389
- Mochizuki H, Papoiu AD, Nattkemper LA, et al. Scratching induces overactivity in motor-related regions and reward system in chronic itch patients. J Invest Dermatol. 2015;135(11):2814-2823. doi: 10.1038/jid.2015.255
- Ching T, Himmelstein DS, Beaulieu-Jones BK, et al. Opportunities and obstacles for deep learning in biology and medicine. J R Soc Interface. 2018;15(141):20170387. doi: 10.1098/rsif.2017.0387
- Winkler JK, Fink C, Toberer F, et al. Association between surgical skin markings in dermoscopic images and diagnostic performance of a deep learning convolutional neural network for melanoma recognition. JAMA Dermatol. 2019;155(10):1135-1141. doi: 10.1001/jamadermatol.2019.1735
- Haw WY, Al-Janabi A, Arents BW, et al. Global guidelines in dermatology mapping project (GUIDEMAP): A scoping review of dermatology clinical practice guidelines. Br J Dermatol. 2021;185(4):736-744. doi: 10.1111/bjd.20428
- Bera K, Schalper KA, Rimm DL, Velcheti V, Madabhushi A. Artificial intelligence in digital pathology-new tools for diagnosis and precision oncology. Nat Rev Clin Oncol. 2019;16(11):703-715. doi: 10.1038/s41571-019-0252-y
- Niazi MK, Parwani AV, Gurcan MN. Digital pathology and artificial intelligence. Lancet Oncol. 2019;20(5):e253-e261. doi: 10.1016/s1470-2045(19)30154-8
- Steele L, Velazquez‐Pimentel D, Thomas B. Do AI models recognise rare, aggressive skin cancers? An assessment of a direct-to-consumer application in the diagnosis of Merkel cell carcinoma and amelanotic melanoma. J Eur Acad Dermatol Venereol. 2021;35(12):e877-e879. doi: 10.1111/jdv.17517
- Li Z, Koban KC, Schenck TL, Giunta RE, Li Q, Sun Y. Artificial intelligence in dermatology image analysis: Current developments and future trends. J Clin Med. 2022;11(22):6826. doi: 10.3390/jcm11226826
- Kleinberg G, Diaz MJ, Batchu S, Lucke-Wold B. Racial underrepresentation in dermatological datasets leads to biased machine learning models and inequitable healthcare. J Biomed Res. 2022;3(1):42-47. doi: 10.46439/biomedres.3.025
- Omiye JA, Gui H, Daneshjou R, Cai ZR, Muralidharan, V. Principles, applications, and future of artificial intelligence in dermatology. Front Med (Lausanne). 2023;10:1278232. doi: 10.3389/fmed.2023.1278232
- Shorten C, Khoshgoftaar TM. A survey on image data augmentation for deep learning. J Big Data. 2019;6(1):60. doi: 10.1186/s40537-019-0197-0
- Yosinski J, Clune J, Bengio Y, Lipson H. How transferable are features in deep neural networks? Adv Neural Inf Process Syst, 2014;27:3320-3328.
- Settles B. Active Learning Literature Survey. Computer Sciences Technical Report 1648, University of Wisconsin- Madison; 2009. Available from: http://digital.library.wic. edu/1793/60660 [Last accessed on 2024 Jan 09].
- Pan SJ, Yang Q. A survey on transfer learning. IEEE Trans Knowl Data Eng. 2010;22(10):1345-1359. doi: 10.1109/tkde.2009.191
- Fawcett T. An introduction to ROC analysis. Pattern Recognit Lett. 2006;27(8):861-874. doi: 10.1016/j.patrec.2005.10.010
- Srivastava N, Hinton G, Krizhevsky A, Sutskever I, Salakhutdinov R. Dropout: A simple way to prevent neural networks from overfitting. J Mach Learn Res. 2014;15(1):1929-1958.
- Polesie S, Gillstedt M, Kittler H, et al. Attitudes towards artificial intelligence within dermatology: An international online survey. Br J Dermatol. 2020;183(1):159-161. doi: 10.1111/bjd.18875
- Polesie S, McKee PH, Gardner JM, et al. Attitudes toward artificial intelligence within dermatopathology: An international online survey. Front Med (Lausanne). 2020;7:591952. doi: 10.3389/fmed.2020.591952
- Nelson CA, Pérez-Chada LM, Creadore A, et al. Patient perspectives on the use of artificial intelligence for skin cancer screening: A qualitative study. JAMA Dermatol. 2020;156(5):501-512. doi: 10.1001/jamadermatol.2019.5014
- Koguchi-Yoshioka H, Watanabe R, Fujisawa Y, et al. Skin resident memory T-cell population is not constructed effectively in systemic sclerosis. Br J Dermatol. 2019;180(1):219-220. doi: 10.1111/bjd.17100
- Tschandl P, Rosendahl C, Kittler H. The HAM10000 dataset, a large collection of multi-source dermatoscopic images of common pigmented skin lesions. Sci Data. 2018;5:180161. doi: 10.1038/sdata.2018.161
- ISIC-International Skin Imaging Collaboration; 2018. Available from: https://www.isic-archive.com/#!/ topwithheader/widecontenttop/main [Last accessed on 2024 Jan 09].
- Mendonça T, Ferreira PM, Marques JS, Marcal AR, Rozeira J. PH2-A Dermoscopic Image Database for Research and Benchmarking. In: 2013 35th Annual International Conference IEEE Engineering Medical Biology Society (EMBC). United States: IEEE; 2013. p. 5437-5440. doi: 10.1109/embc.2013.6610779
- Ioffe S, Szegedy C. Batch Normalization: Accelerating deep Network Training by Reducing Internal Covariate Shift. In: International Conference on Machine Learning; 2015. p. 448- 456. Available from: http://arxiv.org/abs/1502.03167 [Last accessed on 2024 Jan 10].
- Lemley J, Bazrafkan S, Corcoran P. Smart augmentation-learning an optimal data augmentation strategy. IEEE Access. 2017;5:5858-5869.
- Han SS, Lim W, Kim MS, Park I, Park GH, Chang SE. Interpretation of the outputs of a deep learning model trained with a skin cancer dataset. J Invest Dermatol. 2018;138(10):2275-2277. doi: 10.1016/j.jid.2018.05.014
- Navarrete-Dechent C, Dusza SW, Liopyris K, Marghoob AA, Halpern AC, Marchetti MA. Automated dermatological diagnosis: Hype or reality? J Invest Dermatol. 2018;138(10):2277-2279. doi: 10.1016/j.jid.2018.04.040
- Hogarty DT, Mackey DA, Hewitt AW. Current state and future prospects of artificial intelligence in ophthalmology: A review. Clin Exp Ophthalmol. 2019;47(1):128-139. doi: 10.1111/ceo.13381
- Angus DC. Randomized clinical trials of artificial intelligence. JAMA. 2020;323(11):1043. doi: 10.1001/jama.2020.1039
- Matheny ME, Whicher D, Thadaney Israni S. Artificial intelligence in health care. A report from the national academy of medicine. JAMA. 2020;323(6):509-510. doi: 10.1001/jama.2019.21579
- Bergl PA, Wijesekera TP, Nassery N, Cosby KS. Controversies in diagnosis: Contemporary debates in the diagnostic safety literature. Diagnosis (Berl). 2020;7(1):3-9. doi: 10.1515/dx-2019-0016
- Ferrante G, Licari A, Marseglia GL, La Grutta S. Artificial intelligence as an emerging diagnostic approach in paediatric pulmonology. Respirology. 2020;25(10):1029-1030.