Evaluating the effectiveness of artificial intelligence imaging in the qualitative diagnosis of pulmonary nodules
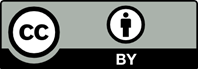
Our study aimed to evaluate the effectiveness of artificial intelligence (AI) image diagnostic systems in the qualitative diagnosis of pulmonary nodules. We analyzed 291 cases from June 2023 to January 2024 at Chongqing University Three Gorges Hospital. All patients in the study underwent low-dose chest computed tomography scans, which identified lung nodules, followed by thoracic surgery for pathological confirmation. We compared the predictive accuracy of AI-based diagnosis with that of physician-based diagnosis in distinguishing between benign and malignant lung nodules. Among the 291 lung nodules examined, 226 were cancerous, and 65 were benign. Receiver operating characteristic (ROC) curves, plotted based on the malignancy probabilities predicted by both methods, revealed that the AI group achieved an area under the ROC curve (AUC) of 0.727, with a sensitivity of 90.27% and a specificity of 58.46%. In comparison, the physician-reading group had an AUC of 0.737, with a sensitivity of 83.19% and a specificity of 66.15%. Our findings demonstrate that the AI diagnostic system effectively calculates malignancy probabilities for lung nodules, highlighting its significant predictive potential. This system can serve as a valuable adjunct tool for clinicians and imaging physicians in the diagnostic process.
- Cai L, Zhu CX, Zhang XL, Fang Y, Yang HY, Guo LW. Interpretation of global lung cancer statistics. Zhonghua Liu Xing Bing Xue Za Zhi. 2024;45(04):585-590. doi: 10.3760/cma.j.cn112338-20230920-00172
- Zheng RS, Chen R, Han BF, et al. Cancer incidence and mortality in China, 2022. Zhonghua Zhong Liu Za Zhi. 2024;46(3):221-231. doi: 10.3760/cma.j.cn112152-20240119-00035
- Yang W, Qian F, Teng J, et al. Community-based lung cancer screening with low-dose CT in China: Results of the baseline screening. Lung Cancer. 2018;117:20-26. doi: 10.1016/j.lungcan.2018.01.003
- WHO Classification of Tumours Editorial Board. WHO Classification of Tumours Thoracic Tumours. 5th ed. Lyon: IARC Press; 2021.
- Tsao MS, Nicholson AG, Maleszewski JJ, Marx A, Travis WD. Introduction to 2021 WHO classification of thoracic tumors. J Thorac Oncol. 2022;17(1):e1-e4. doi: 10.1016/j.jtho.2021.09.017
- Yotsukura M, Asamura H, Motoi N, et al. Long-term prognosis of patients with resected adenocarcinoma in situ and minimally invasive adenocarcinoma of the lung. J Thorac Oncol. 2021;16(8):1312-1320. doi: 10.1016/j.jtho.2021.04.007
- Travis WD, Brambilla E, Noguchi M, et al. International association for the study of lung cancer/American thoracic society/European respiratory society international multidisciplinary classification of lung adenocarcinoma. J Thorac Oncol. 2011;6(2):244-285. doi: 10.1097/JTO.0b013e318206a221
- Henschke CI, Yankelevitz DF, Libby DM, et al. Survival of patients with stage I lung cancer detected on CT screening. N Engl J Med. 2006;355(17):1763-1771. doi: 10.1056/NEJMoa060476
- Siegel RL, Miller KD, Jemal A. Cancer statistics, 2017. CA Cancer J Clin. 2017;67(1):7-30. doi: 10.3322/caac.21387
- Yankelevitz DF, Yip R, Henschke CI. Impact of duration of diagnostic workup on prognosis for early lung cancer. J Thorac Oncol. 2023;18(4):527-537. doi: 10.1016/j.jtho.2022.12.020
- De Koning HJ, van der Aalst CM, de Jong PA, et al. Reduced lung-cancer mortality with volume CT screening in a randomized trial. N Engl J Med. 2020;382(6):503-513. doi: 10.1056/NEJMoa1911793
- Horst C, Nair A, Janes SM. Lessons on managing pulmonary nodules from NELSON: We have come a long way. Thorax. 2019;74(5):427-429. doi: 10.1136/thoraxjnl-2018-212783
- Cardiothoracic Group of Chinese Medical Association Radiology Branch. Expert consensus on low dose spiral CT lung cancer screening. Chin J Radiol. 2015;49(5):328-335.
- Chinese Alliance Against Lung Cancer, Chinese Medical Association of Respiratory Disease Branch Lung Cancer Study Group, Chinese Medical Doctor Association of Respiratory Doctor Branch Lung Cancer Working Committee. Chinese expert consensus on screening and management of lung cancer. Int J Respir. 2019;39(21):1604-1615.
- Rubin GD. Lung nodule and cancer detection in computed tomography screening. J Thorac Imaging. 2015;30(2):130-188. doi: 10.1097/RTI.0000000000000140
- Ko JP, Bagga B, Gozansky E, Moore WH. Solitary pulmonary nodule evaluation: Pearls and pitfalls. Semin Ultrasound CT MR. 2022;43:230-245. doi: 10.1053/j.sult.2022.01.006
- Busby LP, Courtier JL, Glastonbury CM. Bias in radiology: The how and why of misses and misinterpretations. Radiographics. 2018;38:236-247. doi: 10.1148/rg.2018170107
- Bruls RJM, Kwee RM. Workload for radiologists during on-call hours: Dramatic increase in the past 15 years. Insights Imaging. 2020;11(1):121. doi: 10.1186/s13244-020-00925-z
- Pei Q, Luo Y, Chen Y, Li J, Xie D, Ye T. Artificial intelligence in clinical applications for lung cancer: Diagnosis, treatment and prognosis. Clin Chem Lab Med. 2022;60(12):1974-1983. doi: 10.1515/cclm-2022-0291
- Niu PH, Zhao LL, Wu HL, Zhao DB, Chen YT. Artificial intelligence in gastric cancer: Application and future perspectives. World J Gastroenterol. 2020;26(36):5408-5419. doi: 10.3748/wjg.v26.i36.5408
- Qiu H, Ding S, Liu J, Wang L, Wang X. Applications of artificial intelligence in screening, diagnosis, treatment, and prognosis of colorectal cancer. Curr Oncol. 2022;29(3):1773-1795. doi: 10.3390/curroncol29030146
- Pak S, Park SG, Park J, Cho ST, Lee YG, Ahn H. Applications of artificial intelligence in urologic oncology. Investig Clin Urol. 2024;65(3):202-216. doi: 10.4111/icu.20230435
- Liu T, Ye J, Hu C, et al. Artificial intelligence-enabled spatially resolved transcriptomics reveal spatial tissue organization of multiple tumors. Tumor Discov. 2024;3(1):2049. doi: 10.36922/td.2049
- Hou Q, Deng K, Ye M, Dai G, Wang M, Xing J. Preliminary study on computer-aided diagnosis of small peripheral pulmonary adenocarcinoma by CT images based on deep learning. Chin Comput Med Imaging. 2018;24(5):378-382.
- Schwyzer M, Martini K, Benz DC, et al. Artificial intelligence for detecting small FDG-positive lung nodules in digital PET/CT: Impact of image reconstructions on diagnostic performance. Eur Radiol. 2020;30(4):2031-2040. doi: 10.1007/s00330-019-06498-w
- Zhang Y, Jiang B, Zhang L, et al. Lung nodule detectability of artificial intelligence-assisted CT image reading in lung cancer screening. Curr Med Imaging. 2022;18(3):327-334. doi: 10.2174/1573405617666210806125953
- Abadia AF, Yacoub B, Stringer N, et al. Diagnostic accuracy and performance of artificial intelligence in detecting lung nodules in patients with complex lung disease: A noninferiority study. J Thoracic Imaging. 2022;37(3):154-161. doi: 10.1097/RTI.0000000000000613
- Sung H, Ferlay J, Siegel RL, et al. Global cancer statistics 2020: GLOBOCAN estimates of incidence and mortality worldwide for 36 cancers in 185 countries. CA Cancer J Clin. 2021;71(3):209-249. doi: 10.3322/caac.21660
- Xia C, Dong X, Li H, et al. Cancer statistics in China and United States, 2022: Profiles, trends, and determinants. Chin Med J (Engl). 2022;135(5):584-590. doi: 10.1097/CM9.0000000000002108
- Siegel RL, Miller KD, Wagle NS, Jemal A. Cancer statistics, 2023. CA Cancer J Clin. 2023;73(1):17-48. doi: 10.3322/caac.21763
- Liang W, Chen Z, Li C, et al. Accurate diagnosis of pulmonary nodules using a noninvasive DNA methylation test. J Clin Invest. 2021;131(10):e145973. doi: 10.1172/JCI145973
- Allemani C, Matsuda T, Di Carlo V, et al. Global surveillance of trends in cancer survival 2000-14 (CONCORD-3): Analysis of individual records for 37 513 025 patients diagnosed with one of 18 cancers from 322 population-based registries in 71 countries. Lancet. 2018;391(10125):1023-1075. doi: 10.1016/S0140-6736(17)33326-3
- Li D, Mikela Vilmun B, Frederik Carlsen J, et al. The performance of deep learning algorithms on automatic pulmonary nodule detection and classification tested on different datasets that are not derived from lidc-idri: A systematic review. Diagnostics (Basel). 2019;9(4):207. doi: 10.3390/diagnostics9040207
- Li X, Guo F, Zhou Z, et al. Performance of deep-learning-based artificial intelligence on detection of pulmonary nodules in chest CT. Zhongguo Fei Ai Za Zhi. 2019;22(6):336-340. doi: 10.3779/j.issn.1009-3419.2019.06.02
- Ardila D, Kiraly AP, Bharadwaj S, et al. End-to-end lung cancer screening with three-dimensional deep learning on low-dose chest computed tomography. Nat Med. 2019;25:954-961. doi: 10.1038/s41591-019-0447-x
- Zhang C, Sun X, Dang K, et al. Toward an expert level of lung cancer detection and classification using a deep convolutional neural network. Oncologist. 2019;24:1159-1165. doi: 10.1634/theoncologist.2018-0908
- Svoboda E. Artificial intelligence is improving the detection of lung cancer. Nature. 2020;587(7834):S20-S22. doi: 10.1038/d41586-020-03157-9
- Ziegelmayer S, Graf M, Makowski M, Gawlitza J, Gassert F. Cost-effectiveness of artificial intelligence support in computed tomography-based lung cancer screening. Cancers (Basel). 2022;14(7):1729. doi: 10.3390/cancers14071729
- Jianghong C, Zhaohui Z, Guilian J, Zhenghan Y, Zhenchang W, Dawei W. Predicting malignant probability of subsolid nodules with artificial intelligence-assisted pulmonary nodule diagnosis system. Chin J Med Imaging Technol. 2020;36(4):535-539.
- Liu X, Zhou H, Hu Z, Jin Q, Wang J, Ye B. Clinical application of artificial intelligence recognition technology in the diagnosis of stage T1 lung cancer. Chin J Lung Carcer. 2019;22(5):319-323. doi: 10.3779/j.issn.1009-3419.2019.05.09