Bioinformatics analysis of missense mutations in CXCR1 implicates altered protein stability and function
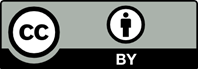
Human CXCR1 is a G-protein α subunit i (Gαi)-coupled receptor (GPCR) that plays an important role in promoting leukocyte recruitment and activation in inflammatory regions; thus, its genetic contribution to human disorders warrants further investigation. In this study, we investigated whether oncogenic missense mutations in CXCR1 would affect its activity and hinder its ability to interact with its ligand. This study utilized a bioinformatics approach and employed precise and thorough computational methods to gain insights into the molecular characteristics of mutated CXCR1 that are responsible for causing diseases. I-TASSER was used to construct a mutant model with the required mutations. Schrödinger’s Desmond software was used to evaluate how mutations affect the stability and function of proteins. In this study, 299 CXCR1 missense mutations were examined; 53 of these were reported to be disease-causing, five of which were directly associated with cancer. The impact of the three cancer-causing mutations (N57D, R135C, and P302S) on protein stability and function was subsequently examined through computational analysis. Positions N57, R135, and P302 were determined to be highly conserved, and substitutions with aspartic acid (D), cysteine (C), and serine (S), respectively, could impair CXCR1 activity. Hence, our findings suggested that these mutations could alter CXCR1 ligand binding activity, lowering the risk of cancer and helping patients defend against pathogen invasion during a neutrophil-mediated innate immune response.
1. Yang D, Zhou Q, Labroska V, et al. G protein-coupled receptors: Structure-and function-based drug discovery. Signal Transduct Target Ther. 2021;6(1):7. doi: 10.1038/s41392-020-00435-w
2. Katritch V, Cherezov V, Stevens RC. Diversity and modularity of G protein-coupled receptor structures. Trends Pharmacol Sci. 2012;33(1):17-27. doi: 10.1016/j.tips.2011.09.003
3. Berman HM, Westbrook J, Feng Z, et al. The protein data bank. Nucleic Acids Res. 2000;28(1):235-242. doi: 10.1093/nar/28.1.235
4. Rajagopalan L, Rajarathnam K. Ligand selectivity and affinity of chemokine receptor CXCR1. Role of N-terminal domain. J Biol Chem. 2004;279(29):30000-30008. doi: 10.1074/jbc.M313883200
5. Holmes WE, Lee J, Kuang WJ, Rice GC, Wood WI. Structure and functional expression of a human interleukin-8 receptor. Science. 1991;253(5025):1278-1280. J Immun. 2009;183(5):2895-2897. doi: 10.1126/science.1840701
6. Ha H, Debnath B, Neamati N. Role of the CXCL8-CXCR1/2 axis in cancer and inflammatory diseases. Theranostics. 2017;7(6):1543-1588. doi: 10.7150/thno.15625
7. Park SH, Das BB, Casagrande F, et al. Structure of the chemokine receptor CXCR1 in phospholipid bilayers. Nature. 2012;491(7426):779-783. doi: 10.1038/nature11580
8. Sojka AC, Brennan KM, Maizels ET, Young CD. The science behind g protein-coupled receptors (GPCRs) and their accurate visual representation in scientific research. J Biocommun. 2017;41(1):e6. doi: 10.5210/jbc.v41i1.7309
9. Hofmann KP, Scheerer P, Hildebrand PW, et al. A G protein-coupled receptor at work: The rhodopsin model. Trends Biochem Sci. 2009;34(11):540-552. doi: 10.1016/j.tibs.2009.07.005
10. Park JH, Scheerer P, Hofmann KP, Choe HW, Ernst OP. Crystal structure of the ligand-free G-protein-coupled receptor opsin. Nature. 2008;454(7201):183-187. doi: 10.1038/nature07063
11. Vauquelin G, Van Liefde I. G protein-coupled receptors: A count of 1001 conformations. Fundam Clin Pharmacol. 2005;19(1):45-56. doi: 10.1111/j.1472-8206.2005.00319.x
12. Rosenbaum DM, Rasmussen SGF, Kobilka BK. The structure and function of G-protein-coupled receptors. Nature. 2009;459(7245):356-363. doi: 10.1038/nature08144
13. Rosenbaum DM, Cherezov V, Hanson MA, et al. GPCR engineering yields high-resolution structural insights into beta2-adrenergic receptor function. Science. 2007;318(5854):1266-1273. doi: 10.1126/science.1150609
14. Landau EM, Rosenbusch JP. Lipidic cubic phases: A novel concept for the crystallization of membrane proteins. Proc Natl Acad Sci U S A. 1996;93(25):14532-14535. doi: 10.1073/pnas.93.25.14532
15. Oldham WM, Hamm HE. Heterotrimeric G protein activation by G-protein-coupled receptors. Nat Rev Mol Cell Biol. 2008;9(1):60-71. doi: 10.1038/nrm2299
16. Leong SR, Kabakoff RC, Hébert CA. Complete mutagenesis of the extracellular domain of interleukin-8 (IL-8) type A receptor identifies charged residues mediating IL-8 binding and signal transduction. J Biol Chem. 1994;269(30):19343-19348.
17. Skelton NJ, Quan C, Reilly D, Lowman H. Structure of a CXC chemokine-receptor fragment in complex with interleukin-8. Structure. 1999;7(2):157-168. doi: 10.1016/S0969-2126(99)80022-7
18. Hébert CA, Vitangcol RV, Baker JB. Scanning mutagenesis of interleukin-8 identifies a cluster of residues required for receptor binding. J Biol Chem. 1991;266(28):18989-18994.
19. Nicholls DJ, Tomkinson NP, Wiley KE, et al. Identification of a putative intracellular allosteric antagonist binding-site in the CXC chemokine receptors 1 and 2. Mol Pharmacol. 2008;74(5):1193-1202. doi: 10.1124/mol.107.044610
20. Han X, Feng Y, Chen X, Gerard C, Boisvert WA. Characterization of G protein coupling mediated by the conserved D134(3.49) of DRY motif, M241(6.34), and F251(6.44) residues on human CXCR1. FEBS Open Bio. 2015;5:182-190. doi: 10.1016/j.fob.2015.03.001
21. Huynh N, Mallik B, Zhang L, Martins-Green M, Morikis D. Computational studies of CXCR1, the receptor of IL-8/ CXCL8, using molecular dynamics and electrostatics. Biopolymers. 2008;89(1):52-61. doi: 10.1002/bip.20851
22. Pandurangan AP, Blundell TL. Prediction of impacts of mutations on protein structure and interactions: SDM, a statistical approach, and mCSM, using machine learning. Protein Sci. 2020;29(1):247-257. doi: 10.1002/pro.3774
23. Capriotti E, Altman RB, Bromberg Y. Collective judgment predicts disease-associated single nucleotide variants. BMC Genomics. 2013;14(Suppl 3):S2. doi: 10.1186/1471-2164-14-S3-S2
24. López-Ferrando V, Gazzo A, de la Cruz X, Orozco M, Gelpí JL. PMut: A web-based tool for the annotation of pathological variants on proteins, 2017 update. Nucleic Acids Res. 2017;45(W1):W222-W228. doi: 10.1093/nar/gkx313
25. Choi Y, Chan AP. PROVEAN web server: A tool to predict the functional effect of amino acid substitutions and indels. Bioinformatics. 2015;31(16):2745-2747. doi: 10.1093/bioinformatics/btv195
26. Rogers MF, Shihab HA, Mort M, Cooper DN, Gaunt TR, Campbell C. FATHMM-XF: Accurate prediction of pathogenic point mutations via extended features. Bioinformatics. 2018;34(3):511-513. doi: 10.1093/bioinformatics/btx536
27. Pires DE, Ascher DB, Blundell TL. mCSM: Predicting the effects of mutations in proteins using graph-based signatures. Bioinformatics. 2014;30(3):335-342. doi: 10.1093/bioinformatics/btt691
28. Worth CL, Preissner R, Blundell TL. SDM--a server for predicting effects of mutations on protein stability and malfunction. Nucleic Acids Res. 2011;39:W215-222. doi: 10.1093/nar/gkr363
29. Pires DE, Ascher DB, Blundell TL. DUET: A server for predicting effects of mutations on protein stability using an integrated computational approach. Nucleic Acids Res. 2014;42:W314-W319. doi: 10.1093/nar/gku411
30. Martin FJ, Amode MR, Aneja A, et al. Ensembl 2023. Nucleic Acids Res. 2023;51(D1):D933-D941. doi: 10.1093/nar/gkac958
31. Sherry ST, Ward MH, Kholodov M, et al. dbSNP: The NCBI database of genetic variation. Nucleic Acids Res. 2001;29(1):308-311. doi: 10.1093/nar/29.1.308
32. UniProt Consortium. UniProt: A hub for protein information. Nucleic Acids Res. 2015;43:D204-D212. doi: 10.1093/nar/gku989
33. Goodsell DS, Zardecki C, Di Costanzo L, et al. RCSB protein data bank: Enabling biomedical research and drug discovery. Protein Sci. 2020;29(1):52-65. doi: 10.1002/pro.3730
34. Hecht M, Bromberg Y, Rost B. Better prediction of functional effects for sequence variants. BMC Genomics. 2015;16(Suppl 8):S1. doi: 10.1186/1471-2164-16-S8-S1
35. Mi H, Lazareva-Ulitsky B, Loo R, et al. The PANTHER database of protein families, subfamilies, functions and pathways. Nucleic Acids Res. 2005;33:D284-D288. doi: 10.1093/nar/gki078
36. Ng PC, Henikoff S. SIFT: Predicting amino acid changes that affect protein function. Nucleic Acids Res. 2003;31(13):3812-3814. doi: 10.1093/nar/gkg509
37. Bendl J, Stourac J, Salanda O, et al. PredictSNP: Robust and accurate consensus classifier for prediction of disease-related mutations. PLoS Comput Biol. 2014;10(1):e1003440. doi: 10.1371/journal.pcbi.1003440
38. Pejaver V, Mooney SD, Radivojac P. Missense variant pathogenicity predictors generalize well across a range of function-specific prediction challenges. Hum Mutat. 2017;38(9):1092-1108. doi: 10.1002/humu.23258
39. Ghosh M, Sodhi SS, Sharma N, et al. An integrated in silico approach for functional and structural impact of non-synonymous SNPs in the MYH1 gene in Jeju Native Pigs. BMC Genet. 2016;17:35. doi: 10.1186/s12863-016-0341-1
40. Vila JA. Proteins’ evolution upon point mutations. ACS Omega. 2022;7(16):14371-14376. doi: 10.1021/acsomega.2c01407
41. Pandurangan AP, Ochoa-Montaño B, Ascher DB, Blundell TL. SDM: A server for predicting effects of mutations on protein stability. Nucleic Acids Res. 2017;45(W1):W229-W235. doi: 10.1093/nar/gkx439
42. Choudhury A, Mohammad T, Anjum F, et al. Comparative analysis of web-based programs for single amino acid substitutions in proteins. PLoS One. 2022;17(5):e0267084. doi: 10.1371/journal.pone.0267084
43. AlAjmi MF, Khan S, Choudhury A, et al. Impact of deleterious mutations on structure, function and stability of serum/glucocorticoid regulated kinase 1: A gene to diseases correlation. Front Mol Biosci. 2021;8:780284. doi: 10.3389/fmolb.2021.780284
44. Rodrigues CHM, Myung Y, Pires DEV, Ascher DB. mCSM-PPI2: Predicting the effects of mutations on protein-protein interactions. Nucleic Acids Res. 2019;47(W1):W338-W344. doi: 10.1093/nar/gkz383
45. Mutlu G, Acı Çİ. SVM-SMO-SGD: A hybrid-parallel support vector machine algorithm using sequential minimal optimization with stochastic gradient descent. Parallel Comput. 2022;113:102955. doi: 10.1016/j.parco.2022.102955
46. Shihab HA, Gough J, Cooper DN, Day INM, Gaunt TR. Predicting the functional consequences of cancer-associated amino acid substitutions. Bioinformatics. 2013;29(12):1504-1510. doi: 10.1093/bioinformatics/btt182
47. Zhang Y. I-TASSER server for protein 3D structure prediction. BMC Bioinformats. 2008;9:40. doi: 10.1186/1471-2105-9-40
48. Ishimoto N, Park JH, Kawakami K, et al. Structural basis of CXC chemokine receptor 1 ligand binding and activation. Nat Commun. 2023;14(1):4107. doi: 10.1038/s41467-023-39799-2
49. Lovell SC, Davis IW, Arendall WB 3rd, et al. Structure validation by Calpha geometry: Phi,psi and Cbeta deviation. Proteins. 2003;50(3):437-450. doi: 10.1002/prot.10286
50. Kc DB. Recent advances in sequence-based protein structure prediction. Brief Bioinform. 2017;18(6):1021-1032. doi: 10.1093/bib/bbw070
51. Hollingsworth SA, Dror RO. Molecular Dynamics Simulation for All. Neuron. 2018;99(6):1129-1143. doi: 10.1016/j.neuron.2018.08.011
52. Muhammed MT, Aki-Yalcin E. Homology modeling in drug discovery: Overview, current applications, and future perspectives. Chem Biol Drug Des. 2019;93(1):12-20. doi: 10.1111/cbdd.13388
53. Brooks BR, Brooks CL 3rd, Mackerell AD Jr, et al. CHARMM: The biomolecular simulation program. J Comput Chem. 2009;30(10):1545-1614. doi: 10.1002/jcc.21287
54. Rasheed MA, Iqbal MN, Saddick S, et al. Identification of lead compounds against Scm (fms10) in Enterococcus faecium using computer aided drug designing. Life (Basel). 2021;11(2):77. doi: 10.3390/life11020077
55. Hildebrand PW, Rose AS, Tiemann JKS. Bringing molecular dynamics simulation data into view. Trends Biochem Sci. 2019;44(11):902-913. doi: 10.1016/j.tibs.2019.06.004
56. Ferreira LG, Dos Santos RN, Oliva G, Andricopulo AD. Molecular docking and structure-based drug design strategies. Molecules. 2015;20(7):13384-13421. doi: 10.3390/molecules200713384
57. Shivakumar D, Williams J, Wu Y, Damm W, Shelley J, Sherman W. Prediction of absolute solvation free energies using molecular dynamics free energy perturbation and the OPLS force field. J Chem Theory Comput. 2010;6(5):1509-1519. doi: 10.1021/ct900587b
58. Huang Y, Li Z, Hong Q, et al. A stepwise docking molecular dynamics approach for simulating antibody recognition with substantial conformational changes. Comput Struct Biotechnol J. 2022;20:710-720. doi: 10.1016/j.csbj.2022.01.012
59. Hung LH, Guerquin M, Samudrala R. GPU-Q-J, a fast method for calculating root mean square deviation (RMSD) after optimal superposition. BMC Res Notes. 2011;4:97. doi: 10.1186/1756-0500-4-97
60. Carter P, Andersen CAF, Rost B. DSSPcont: Continuous secondary structure assignments for proteins. Nucleic Acids Res. 2003;31(13):3293-3295. doi: 10.1093/nar/gkg626
61. Ashkenazy H, Abadi S, Martz E, et al. ConSurf 2016: An improved methodology to estimate and visualize evolutionary conservation in macromolecules. Nucleic Acids Res. 2016;44(W1):W344-W350. doi: 10.1093/nar/gkw408
62. Wang Y, Park JH, Lupala CS, et al. Computer aided protein engineering to enhance the thermo-stability of CXCR1-T4 lysozyme complex. Sci Rep. 2019;9(1):5317. doi: 10.1038/s41598-019-41838-2
63. Yang J, Zhang Y. Protein structure and function prediction using I-TASSER. Curr Protoc Bioinformatics. 2015;52:5.8.1- 5.8.15. doi: 10.1002/0471250953.bi0508s52
64. Roy A, Kucukural A, Zhang Y. I-TASSER: A unified platform for automated protein structure and function prediction. Nat Protoc. 2010;5(4):725-738. doi: 10.1038/nprot.2010.5
65. Sobolev OV, Afonine PV, Moriarty NW, et al. A global Ramachandran score identifies protein structures with unlikely stereochemistry. Structure. 2020;28(11):1249-1258.e2. doi: 10.1016/j.str.2020.08.005
66. Guzzi AF, Oliveira FSL, Amaro MMS, Tavares-Filho PF, Gabriel JE. In silico prediction of the functional and structural consequences of the non-synonymous single nucleotide polymorphism A122V in bovine CXC chemokine receptor type 1. Braz J Biol. 2020;80(1):39-46. doi: 10.1590/1519-6984.188655
67. Kato K, Nakayoshi T, Kurimoto E, Oda A. Mechanisms of deamidation of asparagine residues and effects of main-chain conformation on activation energy. Int J Mol Sci. 2020;21(19):7035. doi: 10.3390/ijms21197035
68. Chakkalakal SA, Heilig J, Baumann U, Paulsson M, Zaucke F. Impact of arginine to cysteine mutations in collagen II on protein secretion and cell survival. Int J Mol Sci. 2018;19(2):541. doi: 10.3390/ijms19020541
69. Ganguly HK, Basu G. Conformational landscape of substituted prolines. Biophys Rev. 2020;12(1):25-39. doi: 10.1007/s12551-020-00621-8
70. Zaki OK, Krishnamoorthy N, El Abd HS, et al. Two patients with Canavan disease and structural modeling of a novel mutation. Metab Brain Dis. 2017;32(1):171-177. doi: 10.1007/s11011-016-9896-9
71. Agrahari AK, Kumar A, Siva R, Zayed H, Doss CGP. Substitution impact of highly conserved arginine residue at position 75 in GJB1 gene in association with X-linked Charcot-Marie-tooth disease: A computational study. J Theor Biol. 2018;437:305-317. doi: 10.1016/j.jtbi.2017.10.028
72. Suetomi K, Rojo D, Navarro J. Identification of a signal transduction switch in the chemokine receptor CXCR1. J Biol Chem. 2002;277(35):31563-31566. doi: 10.1074/jbc.M204713200
73. Mirzadegan T, Benkö G, Filipek S, Palczewski K. Sequence analyses of G-protein-coupled receptors: Similarities to rhodopsin. Biochemistry. 2003;42(10):2759-2767. doi: 10.1021/bi027224+
74. Rovati GE, Capra V, Neubig RR. The highly conserved DRY motif of class A G protein-coupled receptors: Beyond the ground state. Mol Pharmacol. 2007;71(4):959-964. doi: 10.1124/mol.106.029470
75. Arshad M, Bhatti A, John P. Identification and in silico analysis of functional SNPs of human TAGAP protein: A comprehensive study. PLoS One. 2018;13(1):e0188143. doi: 10.1371/journal.pone.0188143
76. Mosaeilhy A, Mohamed MM, Doss CGP, et al. Genotype-phenotype correlation in 18 Egyptian patients with glutaric acidemia type I. Metab Brain Dis. 2017;32(5):1417-1426. doi: 10.1007/s11011-017-0006-4
77. Zhao F, Zheng L, Goncearenco A, Panchenko AR, Li M. Computational approaches to prioritize cancer driver missense mutations. Int J Mol Sci. 2018;19(7):2113. doi: 10.3390/ijms19072113
78. Chun S, Fay JC. Identification of deleterious mutations within three human genomes. Genome Res. 2009;19(9):1553- 1561. doi: 10.1101/gr.092619.109.
79. Li J, Wei Z, Hakonarson H. Application of computational methods in genetic study of inflammatory bowel disease. World J Gastroenterol. 2016;22(3):949-960. doi: 10.3748/wjg.v22.i3.949