Artificial intelligence-enabled spatially resolved transcriptomics reveal spatial tissue organization of multiple tumors
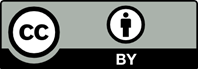
Spatially resolved transcriptomics was honored as the Method of the Year 2020 by Nature Methods. This approach allows biologists to precisely discern mRNA expression at the cellular level within structurally preserved tissues. Leveraging artificial intelligence in spatial transcriptomic analysis enhances the understanding of cellular-level biological interactions and offers novel insights into intricate tissues, such as tumor microenvironments. Nevertheless, numerous existing clustering algorithms employing deep learning exhibit the potential for enhancement. In this paper, we focus on graph deep learning-based spatial domain identification for spatial transcriptomics (ST) data from multiple tumors. This identification enables the recognition of cell subpopulations in distinct spatial coordinates, aiding further studies on tumor progression, such as cell-cell communication, pseudo-time trajectory inference, and single-cell deconvolution. Initially, the gene expression profiles and spatial location information were transformed into a gene feature matrix and a cell adjacency matrix. A variational graph autoencoder was then applied to extract features and reduce the dimensions of these two matrices. Following training in the constructed graph neural networks, the latent embeddings of ST data were generated and could be leveraged for spatial domain identification. Through a comparison with established methods, our approach demonstrated superior clustering accuracy. The utilization of accurately segmented spatial regions enables downstream analyses of multiple tumors, encompassing the trajectory of tumor evolution, and facilitating differential gene expression analysis across various cell types.
- Wang X, Fan J. Spatiotemporal molecular medicine: A new era of clinical and translational medicine. Clin Transl Med. 2021;11(1):e294. doi: 10.1002/ctm2.294
- Wang X. Clinical trans-omics: An integration of clinical phenomes with molecular multiomics. Cell Biol. Toxicol. 2018;34:163-166. doi: 10.1007/s10565-018-9431-3
- Rao A, Barkley D, França GS, et al. Exploring tissue architecture using spatial transcriptomics. Nature. 2021;596:211-220. doi: 10.1038/s41586-021-03634-9
- Eng CHL, Lawson M, Zhu Q, et al. Transcriptome-scale super-resolved imaging in tissues by RNA seqFISH+. Nature. 2019;568:235-239. doi: 10.1038/s41586-019-1049-y
- Chen KH, Boettiger AN, Moffitt JR, et al. RNA imaging. Spatially resolved, highly multiplexed RNA profiling in single cells. Science. 2015;348:aaa6090. doi: 10.1016/B978-0-12-822824-1.00002-X
- Codeluppi S, Borm LE, Zeisel A, et al. Spatial organization of the somatosensory cortex revealed by osmFISH. Nat Methods. 2018;15:932-935. doi: 10.1038/s41592-018-0175-z
- Ghorbani S, Jelinek E, Jain R, et al. Versican promotes T helper 17 cytotoxic inflammation and impedes oligodendrocyte precursor cell remyelination. Nat Commun. 2022;13:2445. doi: 10.1038/s41467-022-30032-0
- Stickels RR, Murray E, Kumar P, et al. Highly sensitive spatial transcriptomics at near-cellular resolution with Slide-seqV2. Nat Biotechnol. 2021;39:313-319. doi: 10.1038/s41587-020-0739-1
- Janesick A, Shelansky R, Gottscho AD, et al. High resolution mapping of the tumor microenvironment using integrated single-cell, spatial and in situ analysis. Nat Commun. 2023;14:8353. doi: 10.1038/s41467-023-43458-x
- Chen A, Liao S, Cheng M, et al. Spatiotemporal transcriptomic atlas of mouse organogenesis using DNA nanoball-patterned arrays. Cell. 2022;185:1777-1792.e21. doi: 10.1016/j.cell.2022.04.003
- Williams CG, Lee HJ, Asatsuma T, et al. An introduction to spatial transcriptomics for biomedical research. Genome Med. 2022;14:68. doi: 10.1186/s13073-022-01075-1
- Bu Z, Li HJ, Zhang C, et al. Graph K-means based on leader identification, dynamic game, and opinion dynamics. IEEE Trans Knowl Data Eng. 2020;32:1348-1361. doi: 10.1109/TKDE.2019.2903712
- Dries R, Zhu Q, Dong R, et al. Giotto: A toolbox for integrative analysis and visualization of spatial expression data. Genome Biol. 2021;22:78. doi: 10.1186/s13059-021-02286-2
- Pham D, Tan X, Balderson B, et al. Robust mapping of spatiotemporal trajectories and cell–cell interactions in healthy and diseased tissues. Nat Commun. 2023;14:7739. doi: 10.1038/s41467-023-43120-6
- Zhao E, Stone MR, Ren X, et al. Spatial transcriptomics at subspot resolution with BayesSpace. Nat Biotechnol. 2021;39:1375-1384. doi: 10.1038/s41587-021-00935-2
- Liu Y, Wang T, Duggan B, Sharpnack M, Huang K, Zhang J, et al. SPCS: A spatial and pattern combined smoothing method for spatial transcriptomic expression. Brief Bioinform. 2022;23:bbac116. doi: 10.1093/bib/bbac116
- Wu Z, Pan S, Chen F, et al. A comprehensive survey on graph neural networks. IEEE Trans Neural Netw Learn Syst. 2021;32:4-24. doi: 10.1109/TNNLS.2020.2978386
- Hu J, Li X, Coleman K, et al. 2021. SpaGCN: Integrating gene expression, spatial location and histology to identify spatial domains and spatially variable genes by graph convolutional network. Nat Methods. 18:1342-1351. doi: 10.1038/s41592-021-01255-8
- Li J, Chen S, Pan X, et al. Cell clustering for spatial transcriptomics data with graph neural networks. Nat Comput Sci. 2022;2:399-408. doi: 10.1038/s43588-022-00266-5
- Dong K, Zhang S. Deciphering spatial domains from spatially resolved transcriptomics with an adaptive graph attention auto-encoder. Nat Commun. 2022;13:1739. doi: 10.1038/s41467-022-29439-6
- Long Y, Ang KS, Li M, et al. Spatially informed clustering, integration, and deconvolution of spatial transcriptomics with GraphST. Nat Commun. 2023;14:1155. doi: 10.1038/s41467-023-36796-3
- Thomas NK, Welling, M. Variational Graph Auto-Encoders. [arXiv Preprint] arXiv. 2016;2:1611.07308. doi: 10.48550/arXiv.1611.07308
- Fey M, Lenssen JE. Fast graph representation learning with pytorch geometric. [arXiv Preprint] arXiv. 2019;1903.02428. doi: 10.48550/arXiv.1903.02428
- Wu F, Souza A, Zhang T, et al. Simplifying Graph Convolutional Networks. In: International Conference on Machine Learning. Vol. 97. New York: PMLR; 2019. p. 6861-6871. doi: 10.48550/arXiv.1902.07153
- Pardo B, Spangler A, Weber LM, et al. SpatialLIBD: An R/Bioconductor package to visualize spatially-resolved transcriptomics data. BMC Genomics. 2022;23(1):1-5. doi: 10.1186/s12864-022-08601-w
- Xu H, Fu H, Long Y, et al. Unsupervised spatially embedded deep representation of spatial transcriptomics. Genome Med. 2024;16:12. doi: 10.1186/s13073-024-01283-x
- Wolf FA, Angerer P, Theis FJ. SCANPY: Large-scale single-cell gene expression data analysis. Genome Biol. 2018;19:15. doi: 10.1186/s13059-017-1382-0
- Pedregosa F, Varoquaux G, Gramfort A, et al. Scikit-learn: Machine learning in python. J Mach Learn Res. 2011;12:2825-2830.
- Addagarla SK, Amalanathan A. Probabilistic unsupervised machine learning approach for a similar image recommender system for e-commerce. Symmetry. 2020;12:1783. doi: 10.3390/sym12111783
- Xu C, Jin X, Wei S, et al. DeepST: Identifying spatial domains in spatial transcriptomics by deep learning. Nucleic Acids Res. 2022;50:e131. doi: 10.1093/nar/gkac901