Bioinformatics approach to identifying potential cancer-associated mutations in CCL2
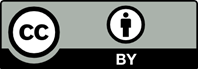
Chemokine C-C motif ligand 2 (CCL2), also known as monocyte chemoattractant protein 1 or small inducible cytokine A2, is a cytokine from the CC chemokine family. It plays a crucial role in recruiting monocytes, memory T-cells, and dendritic cells to inflammatory sites resulting from tissue injury or infection. C-C motif chemokine receptor 2 (CCR2) is a chemokine receptor that may influence lymphocyte function. The interaction between CCL2 and CCR2 is essential for inflammatory responses and cancer regulation, as it attracts monocytes and macrophages to tumor sites, facilitating tumor growth and metastasis. Given the importance of CCL2 in regulating cell trafficking and cancer progression, we employed a bioinformatics approach to examine the effects of oncogenic missense mutations in CCL2 on CCR2 activation. We used precise computational methods to identify the molecular characteristics responsible for the altered activity and interactions, thereby enhancing our understanding of the molecular mechanisms underlying disease progression. We generated a three-dimensional model of the CCL2 protein with the identified mutations using the I-TASSER algorithm. The effects of these mutations on the protein’s stability and functional properties were evaluated using various prediction tools, and molecular dynamics simulations were conducted using WebGro software. Our analysis of 83 CCL2 missense mutations identified 10 disease-causing mutations, including C59G, which was directly linked to cancer. The C59G mutation increases the binding interaction between CCL2 and CCR2. The C59G position was determined to be highly conserved, and substitutions of cysteine (C) 59 with glycine (G) altered CCL2 activity. Our results suggest that this mutation alters the CCL2–CCR2 binding activity, lowering the risk of developing cancer and defending against pathogen invasion during the neutrophil-mediated innate immune response.
- Zhu S, Liu M, Bennett S, Wang Z, Pfleger KDG, Xu J. The molecular structure and role of CCL2 (MCP-1) and C-C chemokine receptor CCR2 in skeletal biology and diseases. J Cell Physiol. 2021;236(10):7211-7222. doi: 10.1002/jcp.30375
- Schubert M, Bleuler-Martinez S, Butschi A, et al. Plasticity of the β-trefoil protein fold in the recognition and control of invertebrate predators and parasites by a fungal defence system. PLoS Pathog. 2012;8(5):e1002706. doi: 10.1371/journal.ppat.1002706
- Joshi N, Kumar D, Poluri KM. Elucidating the molecular interactions of chemokine CCL2 orthologs with flavonoid baicalin. ACS Omega. 2020;5(35):22637-22651. doi: 10.1021/acsomega.0c03428
- Shao Z, Tan Y, Shen Q, et al. Molecular insights into ligand recognition and activation of chemokine receptors CCR2 and CCR3. Cell Discov. 2022;8(1):44. doi: 10.1038/s41421-022-00403-4
- Li H, Wu M, Zhao X. Role of chemokine systems in cancer and inflammatory diseases. MedComm (2020). 2022;3(2):e147. doi: 10.1002/mco2.147
- Turner MD, Nedjai B, Hurst T, Pennington DJ. Cytokines and chemokines: At the crossroads of cell signalling and inflammatory disease. Biochim Biophys Acta. 2014;1843(11):2563-2582. doi: 10.1016/j.bbamcr.2014.05.014
- White GE, Iqbal AJ, Greaves DR. CC chemokine receptors and chronic inflammation-therapeutic opportunities and pharmacological challenges. Pharmacol Rev. 2013;65(1):47-89. doi: 10.1124/pr.111.005074
- Dommel S, Blüher M. Does C-C motif chemokine ligand 2 (CCL2) link obesity to a pro-inflammatory state? Int J Mol Sci. 2021;22(3):1500. doi: 10.3390/ijms22031500
- Xu M, Wang Y, Xia R, Wei Y, Wei X. Role of the CCL2-CCR2 signalling axis in cancer: Mechanisms and therapeutic targeting. Cell Prolif. 2021;54(10):e13115. doi: 10.1111/cpr.13115
- Ben-Baruch A. Host microenvironment in breast cancer development: Inflammatory cells, cytokines and chemokines in breast cancer progression: Reciprocal tumor-microenvironment interactions. Breast Cancer Res. 2002;5(1):31. doi: 10.1186/bcr554
- Yoshimura T, Li C, Wang Y, Matsukawa A. The chemokine monocyte chemoattractant protein-1/CCL2 is a promoter of breast cancer metastasis. Cell Mol Immunol. 2023;20(7):714-38. doi: 10.1038/s41423-023-01013-0
- Soria G, Yaal-Hahoshen N, Azenshtein E, et al. Concomitant expression of the chemokines RANTES and MCP-1 in human breast cancer: A basis for tumor-promoting interactions. Cytokine. 2008;44(1):191-200. doi: 10.1016/j.cyto.2008.08.002
- Furukawa S, Soeda S, Kiko Y, et al. MCP-1 promotes invasion and adhesion of human ovarian cancer cells. Anticancer Res. 2013;33(11):4785-4790.
- Hefler L, Tempfer C, Heinze G, et al. Monocyte chemoattractant protein-1 serum levels in ovarian cancer patients. Br J Cancer. 1999;81(5):855-829. doi: 10.1038/sj.bjc.6690776
- Bhat AA, Nisar S, Maacha S, et al. Cytokine-chemokine network driven metastasis in esophageal cancer; promising avenue for targeted therapy. Mol Cancer. 2021;20:2. doi: 10.1186/s12943-020-01294-3
- Singh S, Anshita D, Ravichandiran V. MCP-1: Function, regulation, and involvement in disease. Int Immunopharmacol. 2021;101(Pt B):107598. doi: 10.1016/j.intimp.2021.107598
- Kitadai Y. Cancer-stromal cell interaction and tumor angiogenesis in gastric cancer. Cancer Microenviron. 2010;3:109-116. doi: 10.1007/s12307-009-0032-9
- Tesch GH, Schwarting A, Kinoshita K, Lan HY, Rollins BJ, Kelley VR. Monocyte chemoattractant protein-1 promotes macrophage-mediated tubular injury, but not glomerular injury, in nephrotoxic serum nephritis. J Clin Invest. 1999;103(1):73-80. doi: 10.1172/jci4876
- Niiya M, Niiya K, Kiguchi T, et al. Induction of TNF-alpha, uPA, IL-8 and MCP-1 by doxorubicin in human lung carcinoma cells. Cancer Chemother Pharmacol. 2003;52(5):391-398. doi: 10.1007/s00280-003-0665-1
- Huang S, Singh RK, Xie K, et al. Expression of the JE/ MCP-1 gene suppresses metastatic potential in murine colon carcinoma cells. Cancer Immunol Immunother. 1994;39(4):231-238. doi: 10.1007/bf01525986
- Pandurangan AP, Blundell TL. Prediction of impacts of mutations on protein structure and interactions: SDM, a statistical approach, and mCSM, using machine learning. Protein Sci. 2020;29(1):247-257. doi: 10.1002/pro.3774
- Capriotti E, Altman RB, Bromberg Y. Collective judgment predicts disease-associated single nucleotide variants. BMC Genomics. 2013;14 Suppl 3:S2. doi: 10.1186/1471-2164-14-s3-s2
- Adzhubei I, Jordan DM, Sunyaev SR. Predicting functional effect of human missense mutations using PolyPhen-2. Curr Protoc Hum Genet. 2013;Chapter 7:Unit7.20. doi: 10.1002/0471142905.hg0720s76
- López-Ferrando V, Gazzo A, de la Cruz X, Orozco M, Gelpí JL. PMut: A web-based tool for the annotation of pathological variants on proteins, 2017 update. Nucleic Acids Res. 2017;45(W1):W222-W228. doi: 10.1093/nar/gkx313
- Rogers MF, Shihab HA, Mort M, Cooper DN, Gaunt TR, Campbell C. FATHMM-XF: Accurate prediction of pathogenic point mutations via extended features. Bioinformatics. 2018;34(3):511-513. doi: 10.1093/bioinformatics/btx536
- Pires DE, Ascher DB, Blundell TL. mCSM: Predicting the effects of mutations in proteins using graph-based signatures. Bioinformatics. 2014;30(3):335-342. doi: 10.1093/bioinformatics/btt691
- Worth CL, Preissner R, Blundell TL. SDM--a server for predicting effects of mutations on protein stability and malfunction. Nucleic Acids Res. 2011;39(Web Server issue):W215-W222. doi: 10.1093/nar/gkr363
- Pires DE, Ascher DB, Blundell TL. DUET: A server for predicting effects of mutations on protein stability using an integrated computational approach. Nucleic Acids Res. 2014;42(Web Server issue):W314-W319. doi: 10.1093/nar/gku411
- Yang J, Zhang Y. I-TASSER server: New development for protein structure and function predictions. Nucleic Acids Res. 2015;43(W1):W174-W181. doi: 10.1093/nar/gkv342
- Gorai S, Junghare V, Kundu K, et al. Synthesis of dihydrobenzofuro[3,2-b]chromenes as potential 3CLpro inhibitors of SARS-CoV-2: A molecular docking and molecular dynamics study. ChemMedChem. 2022;17(8):e202100782. doi: 10.1002/cmdc.202100782
- Farajzadeh-Dehkordi M, Mafakher L, Harifi A, Samiee-Rad F, Rahmani B. Computational analysis of the functional and structural impact of the most deleterious missense mutations in the human Protein C. PLoS One. 2023;18(11):e0294417. doi: 10.1371/journal.pone.0294417
- Martin FJ, Amode MR, Aneja A, et al. Ensembl 2023. Nucleic Acids Res. 2023;51(D1):D933-D941. doi: 10.1093/nar/gkac958
- Sherry ST, Ward MH, Kholodov M, et al. dbSNP: The NCBI database of genetic variation. Nucleic Acids Res. 2001;29(1):308-311. doi: 10.1093/nar/29.1.308
- UniProt Consortium. UniProt: A hub for protein information. Nucleic Acids Res. 2015;43(Database issue):D204-D212. doi: 10.1093/nar/gku989
- Goodsell DS, Zardecki C, Di Costanzo L, et al. RCSB Protein Data Bank: Enabling biomedical research and drug discovery. Protein Sci. 2020;29(1):52-65. doi: 10.1002/pro.3730
- Hecht M, Bromberg Y, Rost B. Better prediction of functional effects for sequence variants. BMC Genomics. 2015;16 Suppl 8(Suppl 8):S1. doi: 10.1186/1471-2164-16-s8-s1
- Mi H, Lazareva-Ulitsky B, Loo R, et al. The PANTHER database of protein families, subfamilies, functions and pathways. Nucleic Acids Res. 2005;33(Database issue):D284-D288. doi: 10.1093/nar/gki078
- Ng PC, Henikoff S. SIFT: Predicting amino acid changes that affect protein function. Nucleic Acids Res. 2003;31(13):3812-3814. doi: 10.1093/nar/gkg509
- Bendl J, Stourac J, Salanda O, et al. PredictSNP: Robust and accurate consensus classifier for prediction of disease-related mutations. PLoS Comput Biol. 2014;10(1):e1003440. doi: 10.1371/journal.pcbi.1003440
- Pejaver V, Mooney SD, Radivojac P. Missense variant pathogenicity predictors generalize well across a range of function-specific prediction challenges. Hum Mutat. 2017;38(9):1092-108. doi: 10.1002/humu.23258
- Adzhubei IA, Schmidt S, Peshkin L, et al. A method and server for predicting damaging missense mutations. Nat Methods. 2010;7(4):248-249. doi: 10.1038/nmeth0410-248
- Vila JA. Proteins’ evolution upon point mutations. ACS Omega. 2022;7(16):14371-14376. doi: 10.1021/acsomega.2c01407
- Pandurangan AP, Ochoa-Montaño B, Ascher DB, Blundell TL. SDM: A server for predicting effects of mutations on protein stability. Nucleic Acids Res. 2017;45(W1):W229-W235. doi: 10.1093/nar/gkx439
- Choudhury A, Mohammad T, Anjum F, et al. Comparative analysis of web-based programs for single amino acid substitutions in proteins. PLoS One. 2022;17(5):e0267084. doi: 10.1371/journal.pone.0267084
- Rodrigues CHM, Myung Y, Pires DEV, Ascher DB. mCSM-PPI2: Predicting the effects of mutations on protein-protein interactions. Nucleic Acids Res. 2019;47(W1):W338-W344. doi: 10.1093/nar/gkz383
- Rodrigues CHM, Pires DEV, Ascher DB. mmCSM-PPI: Predicting the effects of multiple point mutations on protein-protein interactions. Nucleic Acids Res. 2021;49(W1):W417-W424. doi: 10.1093/nar/gkab273
- Chen CW, Lin J, Chu YW. iStable: Off-the-shelf predictor integration for predicting protein stability changes. BMC Bioinformatics. 2013;14 Suppl 2(Suppl 2):S5. doi: 10.1186/1471-2105-14-s2-s5
- Panchal NK, Bhale A, Verma VK, Beevi SS. Computational and molecular dynamics simulation approach to analyze the impact of XPD gene mutation on protein stability and function. 2020. doi: 10.1101/2020.07.18.209841
- Shihab HA, Gough J, Cooper DN, Day IN, Gaunt TR. Predicting the functional consequences of cancer-associated amino acid substitutions. Bioinformatics. 2013;29(12):1504-1510. doi: 10.1093/bioinformatics/btt182
- Ashkenazy H, Abadi S, Martz E, et al. ConSurf 2016: An improved methodology to estimate and visualize evolutionary conservation in macromolecules. Nucleic Acids Res. 2016;44(W1):W344-W350. doi: 10.1093/nar/gkw408
- Ishimoto N, Park JH, Kawakami K, et al. Structural basis of CXC chemokine receptor 1 ligand binding and activation. Nat Commun. 2023;14(1):4107. doi: 10.1038/s41467-023-39799-2
- Lovell SC, Davis IW, Arendall WB 3rd, et al. Structure validation by Calpha geometry: phi,psi and Cbeta deviation. Proteins. 2003;50(3):437-50. doi: 10.1002/prot.10286
- Konopka BM, Nebel JC, Kotulska M. Quality assessment of protein model-structures based on structural and functional similarities. BMC Bioinformatics. 2012;13(1):242. doi: 10.1186/1471-2105-13-242
- Ravikumar A, Ramakrishnan C, Srinivasan N. Stereochemical assessment of (φ,ψ) outliers in protein structures using bond geometry-specific Ramachandran steric-maps. Structure. 2019;27:1875-1884. doi: 10.1016/j.str.2019.09.009
- Durrant JD, McCammon JA. Molecular dynamics simulations and drug discovery. BMC Biol. 2011;9(1):71. doi: 10.1186/1741-7007-9-71
- Hollingsworth SA, Dror RO. Molecular dynamics simulation for all. Neuron. 2018;99(6):1129-1143. doi: 10.1016/j.neuron.2018.08.011
- Muhammed MT, Aki-Yalcin E. Homology modeling in drug discovery: Overview, current applications, and future perspectives. Chem Biol Drug Des. 2019;93(1):12-20. doi: 10.1111/cbdd.13388
- Binbay FA, Rathod DC, George AAP, Imhof D. Quality assessment of selected protein structures derived from homology modeling and AlphaFold. Pharmaceuticals (Basel). 2023;16(12):1662. doi: 10.3390/ph16121662
- Brooks BR, Brooks CL 3rd, Mackerell AD Jr., et al. CHARMM: The biomolecular simulation program. J Comput Chem. 2009;30(10):1545-1614. doi: 10.1002/jcc.21287
- Vieira IHP, Botelho EB, de Souza Gomes TJ, Kist R, Caceres RA, Zanchi FB. Visual dynamics: A WEB application for molecular dynamics simulation using GROMACS. BMC Bioinformatics. 2023;24(1):107. doi: 10.1186/s12859-023-05234-y
- Bagewadi ZK, Khan TM, Gangadharappa B, Kamalapurkar A, Shamsudeen SM, Yaraguppi DM. Molecular dynamics and simulation analysis against superoxide dismutase (SOD) target of Micrococcus luteus with secondary metabolites from Bacillus licheniformis recognized by genome mining approach. Saudi J Biol Sci. 2023;30:103753. doi: 10.1016/j.sjbs.2023.103753
- Shahraki O, Zargari F, Edraki N, Khoshneviszadeh M, Firuzi O. Molecular dynamics simulation and molecular docking studies of 1,4- dihydropyridines as P-glycoprotein’s allosteric inhibitors. J Biomol Struct Dyn. 2016;36:112-125. doi: 10.1080/07391102.2016.1268976
- Ferreira LG, Dos Santos RN, Oliva G, Andricopulo AD. Molecular docking and structure-based drug design strategies. Molecules. 2015;20(7):13384-13421. doi: 10.3390/molecules200713384
- Hildebrand PW, Rose AS, Tiemann JKS. Bringing molecular dynamics simulation data into view. Trends Biochem Sci. 2019;44(11):902-913. doi: 10.1016/j.tibs.2019.06.004
- Tumskiy RS, Tumskaia AV. Multistep rational molecular design and combined docking for discovery of novel classes of inhibitors of SARS-CoV-2 main protease 3CLpro. Chem Phys Lett. 2021;780:138894. doi: 10.1016/j.cplett.2021.138894
- Carter P, Andersen CA, Rost B. DSSPcont: Continuous secondary structure assignments for proteins. Nucleic Acids Res. 2003;31(13):3293-3295. doi: 10.1093/nar/gkg626
- Yan Y, Wen Z, Wang X, Huang SY. Addressing recent docking challenges: A hybrid strategy to integrate template-based and free protein-protein docking. Proteins. 2016;85:497-512. doi: 10.1002/prot.25234
- Case DA, Cheatham TE 3rd, Darden T, et al. The Amber biomolecular simulation programs. J Comput Chem. 2005;26(16):1668-1688. doi: 10.1002/jcc.20290
- Huang SY, Zou X. MDockPP: A hierarchical approach for protein-protein docking and its application to CAPRI rounds 15-19. Proteins. 2010;78(15):3096-3103. doi: 10.1002/prot.22797
- Huang SY, Yan C, Grinter SZ, Chang S, Jiang L, Zou X. Inclusion of the orientational entropic effect and low-resolution experimental information for protein-protein docking in Critical Assessment of PRedicted Interactions (CAPRI). Proteins. 2013;81(12):2183-2191. doi: 10.1002/prot.24435
- Martí-Renom MA, Stuart AC, Fiser A, Sánchez R, Melo F, Sali A. Comparative protein structure modeling of genes and genomes. Annu Rev Biophys Biomol Struct. 2000;29:291-325. doi: 10.1146/annurev.biophys.29.1.291
- Shah K, Amanullah A, Qingqing W, et al. Bioinformatics analysis of missense mutations in CXCR1 implicates altered protein stability and function. Tumor Discov. 2024;3:2512. doi: 10.36922/td.2512
- Sobitan A, Edwards W, Jalal MS, et al. Prediction of the effects of missense mutations on human myeloperoxidase protein stability using in silico saturation mutagenesis. Genes (Basel). 2022;13(8):1412. doi: 10.3390/genes13081412
- Yang J, Zhang Y. Protein structure and function prediction using I‐TASSER. Curr Protoc Bioinformatics. 2015;52:5.8.1-5.8.15. doi: 10.1002/0471250953.bi0508s52
- Zhang Y. I-TASSER server for protein 3D structure prediction. BMC Bioinformatics. 2008;9:40. doi: 10.1186/1471-2105-9-40
- Sobolev OV, Afonine PV, Moriarty NW, et al. A global Ramachandran score identifies protein structures with unlikely stereochemistry. Structure. 2020;28(11):1249-1258.e2. doi: 10.1016/j.str.2020.08.005
- Yang Z, Lasker K, Schneidman-Duhovny D, et al. UCSF Chimera, MODELLER, and IMP: An integrated modeling system. J Struct Biol. 2012;179(3):269-278. doi: 10.1016/j.jsb.2011.09.006
- Wang L, Ding MY, Wang J, Gao JG, Liu RM, Li HT. Effects of site-directed mutagenesis of cysteine on the structure of sip proteins. Front Microbiol. 2022;13:805325. doi: 10.3389/fmicb.2022.805325
- Konrat R, Kräutler B, Weiskirchen R, Bister K. Structure of cysteine-and glycine-rich protein CRP2. Backbone dynamics reveal motional freedom and independent spatial orientation of the lim domains. J Biol Chem. 1998;273(36):23233-23240. doi: 10.1074/jbc.273.36.23233
- Qiu H, Honey DM, Kingsbury JS, et al. Impact of cysteine variants on the structure, activity, and stability of recombinant human α-galactosidase A. Protein Sci. 2015;24(9):1401-1411. doi: 10.1002/pro.2719
- Gerlza T, Winkler S, Atlic A, et al. Designing a mutant CCL2-HSA chimera with high glycosaminoglycan-binding affinity and selectivity. Protein Eng Des Sel. 2015;28(8):231-240. doi: 10.1093/protein/gzv025
- Hao Q, Vadgama JV, Wang P. CCL2/CCR2 signaling in cancer pathogenesis. Cell Commun Signal. 2020;18(1):82. doi: 10.1186/s12964-020-00589-8
- Lim SY, Yuzhalin AE, Gordon-Weeks AN, Muschel RJ. Targeting the CCL2-CCR2 signaling axis in cancer metastasis. Oncotarget. 2016;7(19):28697-28710. doi: 10.18632/oncotarget.7376
- Kadomoto S, Izumi K, Mizokami A. Roles of CCL2- CCR2 axis in the tumor microenvironment. Int J Mol Sci. 2021;22(16):8530. doi: 10.3390/ijms22168530
- Iwamoto H, Izumi K, Mizokami A. Is the C-C motif ligand 2–C-C chemokine receptor 2 axis a promising target for cancer therapy and diagnosis? Int J Mol Sci. 2020;21(23):9328. doi: 10.3390/ijms21239328
- Anderson EM, Thomassian S, Gong J, Hendifar A, Osipov A. Advances in pancreatic ductal adenocarcinoma treatment. Cancers (Basel). 2021;13(21):5510. doi: 10.3390/cancers13215510
- Li X, Zhong J, Deng X, et al. Targeting myeloid-derived suppressor cells to enhance the antitumor efficacy of immune checkpoint blockade therapy. Front Immunol. 2021;12:754196. doi: 10.3389/fimmu.2021.754196
- Chen W, Pan X, Cui X. RCC immune microenvironment subsequent to targeted therapy: A friend or a foe? Front Oncol. 2020;10:573690. doi: 10.3389/fonc.2020.573690
- Jin J, Lin J, Xu A, et al. CCL2: An important mediator between tumor cells and host cells in tumor microenvironment. Front Oncol. 2021;11:722916. doi: 10.3389/fonc.2021.722916
- Toya M, Zhang N, Tsubosaka M, et al. CCL2 promotes osteogenesis by facilitating macrophage migration during acute inflammation. Front Cell Dev Biol. 2023;11:1213641. doi: 10.3389/fcell.2023.1213641
- Butcher MJ, Galkina EV. wRAPping up early monocyte and neutrophil recruitment in atherogenesis via Annexin A1/ FPR2 signaling. Circ Res. 2015;116(5):774-777. doi: 10.1161/circresaha.115.305920
- Deshmane SL, Kremlev S, Amini S, Sawaya BE. Monocyte chemoattractant protein-1 (MCP-1): An overview. J Interferon Cytokine Res. 2009;29(6):313-326. doi: 10.1089/jir.2008.0027
- Suetomi K, Rojo D, Navarro J. Identification of a signal transduction switch in the chemokine receptor CXCR1. J Biol Chem. 2002;277(35):31563-31566. doi: 10.1074/jbc.M204713200
- Studer RA, Dessailly BH, Orengo CA. Residue mutations and their impact on protein structure and function: Detecting beneficial and pathogenic changes. Biochem J. 2013;449:581-594. doi: 10.1042/BJ20121221
- Gerasimavicius L, Livesey BJ, Marsh JA. Loss-of-function, gain-of-function and dominant-negative mutations have profoundly different effects on protein structure. Nat Commun. 2022;13(1):3895. doi: 10.1038/s41467-022-31686-6
- Arshad M, Bhatti A, John P. Identification and in silico analysis of functional SNPs of human TAGAP protein: A comprehensive study. PLoS One. 2018;13(1):e0188143. doi: 10.1371/journal.pone.0188143
- Schwaigerlehner L, Pechlaner M, Mayrhofer P, Oostenbrink C, Kunert R. Lessons learned from merging wet lab experiments with molecular simulation to improve mAb humanization. Protein Eng Des Sel. 2018;31(7-8):257-265. doi: 10.1093/protein/gzy009
- Cárdenas R, Martínez-Seoane J, Amero C. Combining experimental data and computational methods for the non-computer specialist. Molecules. 2020;25(20):4783. doi: 10.3390/molecules25204783
- Kamaraj B, Purohit R. Computational screening of disease-associated mutations in OCA2 gene. Cell Biochem Biophys. 2013;68:97-109. doi: 10.1007/s12013-013-9697-2
- Stefl S, Nishi H, Petukh M, Panchenko A, Alexov E. Molecular mechanisms of disease-causing missense mutations. J Mol Biol. 2013;425:3919-3936. doi: 10.1016/j.jmb.2013.07.014
- Peterson TA, Doughty E, Kann MG. Towards precision medicine: Advances in computational approaches for the analysis of human variants. J Mol Biol. 2013;425(21):4047-4063. doi: 10.1016/j.jmb.2013.08.008
- Mugumbate G, Nyathi B, Zindoga A, Munyuki G. Application of computational methods in understanding mutations in Mycobacterium tuberculosis drug resistance. Front Mol Biosci. 2021;8:643849. doi: 10.3389/fmolb.2021.643849
- Luo P, Chen B, Liao B, Wu FX. Predicting disease‐associated genes: Computational methods, databases, and evaluations. WIREs Data Min Knowl Discov. 2020;11:e1383. doi: 10.1002/widm.1383