An approach for classification of lung nodules
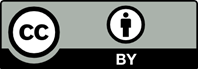
The main objective of the proposed work is to develop an automated computer-aided detection (CAD) system to classify lung nodules using various classifiers from computed tomography (CT) images. One of the most important steps in lung nodule detection is the classification of nodule and non-nodule patterns in CT. The early detection of the condition helps lower the mortality rate. The developed CAD systems consist of segmentation, feature extraction, and classification. In this work, a filter method is used to segment the infected region. Later, we extracted features through and fed into classifiers such as Decision Stump (DS), Random Forest (RF), and Back Propagation Neural Network (BPNN). The experimentation was conducted on LIDC-IDRI dataset, and the results with BPNN outperformed those with DS and RF classifiers.
Akbari R, Ziarati K, 2011, A rank based particle swarm optimization algorithm with dynamic adaptation. J Comput Appl Math., 235(8): 2694–2714. https://doi.org/10.1016/j.cam.2010.11.021
Aoyama M, Li Q, Katsuragawa S, et al., 2003, Computerized scheme for determination of the likelihood measure of malignancy for pulmonary nodules on low-dose CT images. Med Phys, 30(3): 387–394. https://doi.org/10.1118/1.1543575
Armato SG, Giger ML, Moran CJ, et al., 1999, Computerized detection of pulmonary nodules on CT scans. Radiographics, 19(5): 1303–1311. https://doi.org/10.1148/radiographics.19.5.g99se181303
Armato SG 3rd, Hadjiiski L, Tourassi GD, et al., 2015, Guest editorial: Lungx challenge for computerized lung nodule classification: Reflections and lessons learned. J Med Imaging, 2(2): 020103. https://doi.org/10.1117/1.JMI.2.2.020103
Armato SG, McLennan G, Bidaut L, et al., 2011, The lung image database consortium (LIDC) and image database resource initiative (IDRI): A completed reference database of lung nodules on CT scans. Med Phys, 38(2): 915–931. https://doi.org/10.1118/1.3528204
Arumugam MS, Rao MV, 2006, On the performance of the particle swarm optimization algorithm with various inertia weight variants for computing optimal control of a class of hybrid systems. Discrete Dyn Nat Soc., 2006: 079295. https://doi.org/10.1155/DDNS/2006/79295
Choi WJ, Choi TS, 2014, Automated pulmonary nodule detection based on three-dimensional shape-based feature descriptor. Comput Methods Programs Biomed, 113(1): 37–54. https://doi.org/10.1016/j.cmpb.2013.08.01
Criminisi A, Shotton J, Bucciarelli S, 2009, Decision forests with long-range spatial context for organ localization in CT volumes. In: MICCAI Workshop on Probabilistic Models for Medical Image Analysis. vol. 1. Rochester, Minnesota: MICCAI Society.
Cross GR, Jain AK, 1983, Markov random field Texture models. IEEE Trans Pattern Anal Mach Intell, 5(1): 25–39. https://doi.org/10.1109/tpami.1983.4767341
Da Silva Sousa JR, Silva AC, de Paiva AC, et al., 2010, Methodology for automatic detection of lung nodules in computerized tomography images. Comput Methods Programs Biomed, 98(1): 1–14. https://doi.org/10.1016/j.cmpb.2009.07.006
Dai S, Lu K, Dong J, et al., 2015, A novel approach of lung segmentation on chest CT images using graph cuts. Neurocomputing, 168: 799–807. https://doi.org/10.1016/j.neucom.2015.05.044
Daneshmand F, Mehrshad N, Massinaei M, 2013, A new approach for froth image segmentation using fuzzy logic. In: First Iranian Conference on Pattern Recognition and Image Analysis (PRIA). New York City: IEEE.
Dawoud A, 2011, Lung segmentation in chest radiographs by fusing shape information in iterative thresholding. IET Comput Vision, 5(3): 185–190.
De Nunzio G, Tommasi E, Agrusti A, et al., 2011, Automatic lung segmentation in CT images with accurate handling of the Hilar Region. J Digit Imaging, 24(1): 11–27. https://doi.org/10.1007/s10278-009-9229-1
Deep G, Kaur L, Gupta S, 2013, Lung nodule segmentation in CT images using rotation invariant local binary pattern. Int J Signal Image Process, 4(1): 20.
Dehmeshki J, Amin H, Valdivieso M, et al., 2008, Segmentation of pulmonary nodules in thoracic CT scans: A region growing approach. IEEE Trans Med Imaging, 27(4): 467–480. https://doi.org/10.1109/TMI.2007.907555
Dehmeshki J, Ye X, Lin X, et al., 2007, Automated detection of lung nodules in CT images using shape-based genetic algorithm. Computer Med Imaging Graph, 31(6): 408–417. https://doi.org/10.1016/j.compmedimag.2007.03.002
Delogu P, Cheran S, De Mitri I, et al., 2005, Preprocessing methods for nodule detection in lung CT. In: International Congress Series. vol. 1281. Netherlands: Elsevier.
Dheepak G, Premkumar S, Ramachandran R, 2015, Lung Cancer Detection by Using Artificial Neural Network and Fuzzy Clustering Method. Int J Power Control Comput, 7: 24–28.
Doi K, 2007, Computer-aided diagnosis in medical imaging: Historical review, current status and future potential. Computer Med Imaging Graph, 31(4): 198–211.
Dolejsi M, Kybic J, Polovincak M, et al., 2009, The lung time: Annotated lung nodule dataset and nodule detection framework. In: SPIE Medical Imaging. Washington USA: International Society for Optics and Photonics.
Elizabeth D, Nehemiah H, Raj CR, et al., 2012, Computer-aided diagnosis of lung cancer based on analysis of the significant slice of chest computed tomography image. IET Image Processing, 6(6): 697–705.
Enquobahrie AA, Reeves AP, Yankelevitz DF, et al., 2007, Automated detection of small pulmonary nodules in whole lung CT scans. Acad Radiol, 14(5): 579–593.
Farag A, Abdelmunim H, Graham J, et al., 2012, An AAM based detection approach of lung nodules from LDCT scans. In: 9th IEEE International Symposium on Biomedical Imaging (ISBI). New York City: IEEE.
Farag A, Ali A, Graham J, et al., 2011, Evaluation of geometric feature descriptors for detection and classification of lung nodules in low dose CT scans of the chest. In: IEEE International Symposium on Biomedical Imaging: From Nano to Macro. New York City: IEEE.
Farag AA, Abdelmunim H, Graham J, et al., 2011b, Variational approach for segmentation of lung nodules. In: IEEE International Conference on Image Processing (ICIP). New York City: IEEE.
Gambhir S, Shepherd J, Shah B, et al., 1998, Analytical decision model for the cost-effective management of solitary pulmonary nodules. J Clin Oncol, 16(6): 2113–2125.
Garro BA, Vazquez RA, 2015, Designing artificial neural networks using particle swarm optimization algorithms. Comput Intell Neurosci, 2015: 369298. https://doi.org/10.1155/2015/369298
Golosio B, Masala GL, Piccioli A, 2009, A novel multithreshold method for nodule detection in lung CT. Med Phys, 36(8): 3607–3618. https://doi.org/10.1118/1.3160107
Gomathi M, Thangaraj P, 2010a, A computer aided diagnosis system for detection of lung cancer nodules using extreme learning machine. Int J Eng Sci Technol, 2(10), 5770–5779.
Gomathi M, Thangaraj P, 2010b, A computer aided diagnosis system for lung cancer detection using support vector machine. Am J Appl Sci, 7(12): 1532. https://doi.org/10.3844/ajassp.2010.1532.1538
Alves LG, Novo J, Campilho A, 2016, Hessian based approaches for 3d lung nodule segmentation. Expert Syst Appl, 61: 1–15. https://doi.org/10.1016/j.eswa.2016.05.024
Gould MK, Donington J, Lynch WR, et al., 2013, Evaluation of individuals with pulmonary nodules: When is it lung cancer. Chest, 143(5 Suppl): 93S–120S. https://doi.org/10.1378/chest.12-2351
Grigorescu SE, Petkov N, Kruizinga P, 2002, Comparison of texture features based on Gabor filters. IEEE Trans Image Process, 11(10): 1160–1167. https://doi.org/10.1109/TIP.2002.804262
Gu Y, Kumar V, Hall LO, et al., 2013, Automated delineation of lung tumors from CT images using a single click ensemble segmentation approach. Pattern Recogn, 46(3): 692–702.
Gudise VG, Venayagamoorthy VK, 2003, Comparison of particle swarm optimization and backpropagation as training algorithms for neural networks. In: Swarm Intelligence Symposium. New York City: IEEE.
Hua P, Song, M, Sonka EA, et al., 2011, Segmentation of pathological and diseased lung tissue in CT images using a graph-search algorithm. In: IEEE International Symposium on Biomedical Imaging: From Nano to Macro. New York City: IEEE.
Jacobs C, Murphy K, Twellmann T, et al., 2011, Computer-aided detection of solid and ground glass nodules in thoracic CT images using two independent cad systems. In: The Fourth International Workshop on Pulmonary Image Analysis.
Shen S, Bui AA, Cong J, et al., 2015, An automated lung segmentation approach using bidirectional chain codes to improve nodule detection accuracy. Comput Biol Med, 57: 139–149. https://doi.org/10.1016/j.compbiomed.2014.12.008
Shen S, Sandham W, Granat M, et al., 2005, MRI fuzzy segmentation of brain tissue using neighborhood attraction with neural-network optimization. IEEE Trans Inform Technol Biomed, 9(3): 459–467. https://doi.org/10.1109/titb.2005.847500.
Shi, Y, Eberhart R, 1998, A modified particle swarm optimizer. In Evolutionary Computation Proceedings, 1998. In: IEEE World Congress on Computational Intelligence, The 1998 IEEE International Conference. New York City: IEEE.
Shih-Chung BL, Freedman MT, Lin JS, Mun SK, 1993, Automatic lung nodule detection using profile matching and back-propagation neural network techniques. J Digit Imaging, 6(1): 48–54.
Yuan J, 2013, Active contour driven by local divergence energies for ultrasound image segmentation. IET Image Processing, 7(3): 252–259. https://doi.org/10.1049/iet-ipr.2012.0120
Zhou S, Cheng Y, Tamura S, 2014, Automated lung segmentation and smoothing techniques for inclusion of juxtapleural nodules and pulmonary vessels on chest CT images. Biomed Signal Process Control, 13: 62–67. https://doi.org/10.1016/j.bspc.2014.03.010
Flohr TG, Schaller S, Stierstorfer K, et al., 2005, Multi-detector row CT systems and image-reconstruction techniques. Radiology, 235(3): 756–773. https://doi.org/10.1148/radiol.2353040037
Kawane K, Fukuyama H, Yoshida H, et al., 2003, Impaired thymic development in mouse embryos deficient in apoptotic DNA degradation. Nat Immunol, 4(2): 138–144. https://doi.org/10.1038/ni881
Shi J, Malik J, 2000, Normalized cuts and image segmentation. IEEE Trans Pattern Anal Machine Intell, 22(8): 888–905. https://doi.org/10.1109/34.868688
Bercoff J, Tanter M, Fink M, 2004, Supersonic shear imaging: A new technique for soft tissue elasticity mapping. IEEE Trans Ultrason Ferroelectr Freq Control, 51(4): 396–409. https://doi.org/10.1109/tuffc.2004.1295425