Personalization of learning situations within a virtual environment for training based on fuzzy clustering
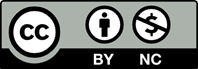
Advances in networks, computers and multimedia technology have changed traditional methods for learning and skills training. Nowadays, Virtual Environments for Training (VET) have been popular, they can provide an environment where virtual reality can be used to create interactive interfaces and real-time software that can control every response and action made by the user. VET have proven to be advantageous to put learners into varied training situations to acquire knowledge and competencies, especially when these situations are taking place in uncontrolled circumstances, or when they are dangerous, unrealizable, or expensive to establish in reality. However individual learners find it difficult to select suitable learning situations for their particular situation because often, there is no personalized service to response to the user needs. Personalization of learning in a VET is a very important way of improving the effectiveness and the quality of the training, yet it also a complex process that requires consideration of several factors such as learners profiles. The goal is to associate suitable learning situations to each learner based on his profile. However, personalization of learning becomes an issue with the uncertainty and imprecision of data that may contains a learner profile. To address this issue, this study is an attempt to integrate a fuzzy clustering into the process of the personalization of learning.
[1] Fuchs, P., & Moreau, G. (2006). Le trait de la ralit virtuelle. Les Presses de lEcole des Mines de Paris, Paris.
[2] Marion, N. (2010). Modlisation de scnarios pdagogiques pour les environnements de ralit virtuelle dapprentissage humain, Phd thesis, universit de Bretagne Occidentale.
[3] Naji, A., & Ramdani, M. (2013). Using the ant colony algorithm to establish the best path of learning activities. Applied Mathematical Sciences, 7(78), 3873- 3881.
[4] Karampiperis, P., & Sampson, D. (2005). Automatic learning object selection and sequencing in web-based intelligent learning systems. In: Zongmin Ma, Ed. Web-Based Intelligent e-Learning Systems: Technologies and Applications, Information Science Publishing, 56-71.
[5] De-Marcos, L., Martinez, J.-J., & Gutierrez, J.-A. (2008). Swarm intelligence in e-learning: A learning object sequencing agent based on competencies. In : Proc. The 10th annual conference on Genetic and evolutionary computation. 17-24.
[6] Valigiani. G., Lutton. E., Fonlupt, C., & Collet, P. (2007). Optimisation par hommilire de chemins pdagogiques pour un logiciel d’e-learning. RSTI TSI, 26(10), 1245-1268.
[7] Bouhdidi, J. E., Ghailani, M., & Fennan, A. (2013). An intelligent architecture for generating evolutionary personalized learning paths based on learner profiles. Journal of Theoretical and Applied Information Technology (JATIT). 57(2), 294-304.
[8] Talhi, S., Djoudi, M., Ouadfel, S., & Zidat, S. (2007). Un canevas de tuteur intelligent hypermdia pour lapprentissage distance universitaire. In : Proc. 4th International Conference on Computer Integrated Manufacturing.
[9] Kardan, A. A., Ebrahim, M. A., & Imani, M. B. (2014). A new personalized learning path generation method: Acomap. Indian Journal Science Research. 5(1), 17-24.
[10] Kumar, A,. Nalavade, J., Yeola, V.,Vivek, V., & Y. Srivastava. (2013). An adaptive learning system based on ant colony algorithm. International Journal of Soft Computing and Engineering (IJSCE). 3(2), 212-214.
[11] Pushpa, M. (2012). Aco in e-learning: Towards an adaptive learning path. International Journal on Computer Science and Engineering (IJCSE), 4(3), 458-462.
[12] Ouraiba, E.-A., Chikh, A., Taleb-Ahmed A., & Yeb dri, Z. E. (2009). Automatic personalization of learning scenarios using svm. In : Proc. International Conference of Advanced Learning Technologies, 183-185.
[13] Ghailani, M., Bouhdidi, J. E., & Fennan, A. (2014). Towards an adaptive e-learning solution based on ontologies and competencies approach. International Journal of Computer Applications, 98(21), 42-52.
[14] Chrysafiadi, K., & Virvou, M. (2012). Evaluating the integration of fuzzy logic into the student model of a web-based learning environment. Expert Systems with Applications, 39(18), 13127-13134.
[15] Al-Aubidy, Kasim M. (2005). Applying Fuzzy Logic for Learner Modeling and Decision Support in Online Learning Systems. Journal of Educational Technology, 2(3), 76-85.
[16] Khan, F. A., Shahzad, F., & Altaf, M. (2017). Fuzzy based approach for adaptivity evaluation of web based open source Learning Management Systems. Cluster Computing, 1-11.
[17] Samarakou, M., Papadakis, A., Prentakis, P., Karolidis, D., & Athineos, S. (2009). A Fuzzy Model for Enhanced Student Evaluation. International Journal of Learning, 16(10). 103-118.
[18] Omidiora, E. O., Olabiyisi, S. O. Okediran, O. O., & Isiaka, R. M. (2013). Learner Activities Evaluation Model: A Neuro-Fuzzy Approach. International Journal of e-Education, e-Business, e-Management and eLearning (IJEEEE). 3(5), 421-424.
[19] Crockett, K., Latham, A., & Whitton, N. (2017). On predicting learning styles in conversational intelligent tutoring systems using fuzzy decision trees. International Journal of Human-Computer Studies, 97, 98- 115.
[20] Deborah, J., Sathiyaseelan, R., Audithan, S., & Vijayakumar, P. (2015). Fuzzy-logic based learning style prediction in e-learning using web interface information. Electrical and Computer Sciences, 40(2), 379- 394.
[21] Zadeh, L. A. (1965). Fuzzy set , Information and Control.338-353.
[22] Zadeh, L. A. (1975). The concept of a linguistic variable and its application to approximate reasoning, Information Sciences, 199-49.
[23] Mamdani E. H., & Assilion, S. (1974). An Experiment in Linguistic Synthesis With a Fuzzy Logic Controller, Intl J. ManMachine Stud. 7, 1-13.
[24] Lin, C. T., Fan, K. W., Yeh, C. M., Pu, H. C., & Wu, F.Y. (2006). High-accuracy skew estimation of document images, International Journal of Fuzzy Systems, 8(3), 119-126.
[25] LinLin C. T., & Chin, C. L. (2006). Using fuzzy inference and cubic curve to detect and compensate backlight image, International Journal of Fuzzy Systems, 8(1), 2-13.
[26] Wallace, M., Ioannou, S., Karpouzis, K., & Kollias, S. (2006). Possibilistic rule evaluation: A case study in facial expressionanalysis, International Journal of Fuzzy Systems. 8(4),219-223.
[27] Bezdek, J. C.(1981). Pattern recognition with fuzzy objective function. New York: Plenum. Kluwer Academic Publishers Norwell, MA, USA.
[28] Fahim, M., Jakimi, A., & El bermi, L.(2016). Pedagogical Scenarization for Virtual Environments for Training: Towards Genericity, Coherence and Adaptivity. International Journal of Advanced Engineering Research and Science (IJAERS), 12(3), 96-103.