Multi-choice stochastic transportation problem involving Weibull distribution
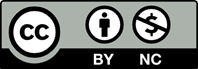
This paper explains the important role in application of stochastic distribution and multichoice framework on the field of transportation environment. The purpose of this paper is to provide a solution procedure to multi-choice stochastic transportation problem involving the parameters as supply and demand of Weibull distribution and cost coefficients of a single criterion of minimization of objective function which are multi-choice in nature. At first, all stochastic constraints are transformed into deterministic constraints by using the stochastic approach. Recently, Mahapatra et al. [14] have proposed a methodology to transfer the multi-choice stochastic transportation problem to an equivalent mathematical programming model which can accumulate a maximum of eight choices on the cost coefficients of the objective function. In this paper, a generalized transformation technique is also present to discuss the two types of transformation technique. Using any one of the transformation technique, the decision maker can handle a parameter of the cost coefficients of objective function with finite number of choice associated with additional restriction for obtaining the equivalent deterministic form. Finally, a numerical example is provided to validate the theoretical development and solution procedure.
[1] Acharya, S and Acharya, M.M., Generalized transformation technique for multi-choice linear programming problem. An International Journal of Optimization and Control: Theories & Applications, 3(1), 45-54 (2013).
[2] Biswal, M.P. and Acharya, S., Transformation of a multi-choice linear programming problem. Applied Mathematics and Computation, 210, 182-188 (2009).
[3] Biswal, M.P. and Acharya, S., Solving probabilistic programming problems involving multi-choice parameters. Opsearch, 210, 1-19 (2011).
[4] Chang, C.-T., Multi-choice goal programming. Omega, The International Journal of Management Science., 35, 389-396 (2007).
[5] Chang, C.-T., Binary fuzzy programming. European Journal of Operation Research, 180, 29-37 (2007).
[6] Chang, C.-T., Revised multi-choice goal programming. Applied Mathematical Modeling, 32, 2587-2595 (2008).
[7] Chang, Ching-Ter, Chen, Huang-Mu , and Zhuang, Zheng-Yun, Multi-coefficients goal programming. Computers and Industrial Engineering, 62(2), 616-623 (2012).
[8] Liao, Chin-Nung, Revised multi-segment goal programming and applications. Prob. Stat. Forum, 4, 110-119 (2011).
[9] Fok, S.L. and Smart, J., Accuracy of failure predictions based on weibull statistic. J. Eur. Ceram. Soc., 15, 905-908 (1995).
[10] Goicoechea, A., Hansen, D.R. and Duckstein, L., Multi-objective Decision Analysis with Engineering and Business Application. New York: John Wiley and Sons (1982).
[11] Hitchcock, F.L., The distribution of a product from several sources to numerous localities. J. Math. Physics, 20, 224-230 (1941).
[12] Koopmans, T.C., Optimum utilization of the transportation system. Ecpnometrica, 17, 3-4 (1949).
[13] Mahapatra, D.R., Roy, S.K. and Biswal, M.P., Computation of multi-objective stochastic transportation problem involving normal distribution with joint constraints. The Journal of Fuzzy Mathematics. 19(4), 865-876 (2011).
[14] Mahapatra, D.R., Roy, S.K. and Biswal, M.P., Multi-choice stochastic transportation problem involving extreme value distribution. Applied Mathematical Modelling, 37(4), 2230-2240 (2013).
[15] Rabindran, A., Philips, D.T. and Solberg, J.J., Operations Research; Principles and Practice. Second Edition, New York: John Wiley and Sons (1987).
[16] Schrage, L., Optimization Modeling with LINGO. LINDO System, Chicago, I11, USA, 6th edition.
[17] Weibull, W., A statistical distribution function of wide applicability. J. of Appl. Mach., 15, 293-302 (1951).