Key drivers of volatility in BIST100 firms using machine learning segmentation
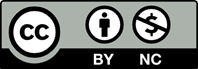
This study conducts a comprehensive volatility analysis among firms listed on the BIST100 index using machine learning techniques and panel regression models. Focusing on the period from 2006 to 2023, the study excludes financial firms, resulting in a dataset of 46 companies. The methodology follows a two-step process: First, firms are clustered into low and high-volatility groups using Principal Component Analysis (PCA) and the K-means algorithm; second, panel regression models are applied to determine the financial ratios influencing stock price volatility. The Parkinson Volatility measure is used as the dependent variable, while independent variables include Return on Assets (ROA), Return on Equity (ROE), liquidity ratios, firm beta, and leverage ratios. Results indicate that firm beta has a statistically significant positive impact on volatility across all models, while the current ratio negatively affects volatility in the model 1. These findings provide valuable insights for investors and policymakers regarding risk management in the Turkish stock market. Applying machine learning and advanced econometric techniques adds to the literature on volatility forecasting and financial decision-making.
[1]. Dixit, J., & Agrawal, V. (2019). Foresight for stock market volatility - a study in the Indian perspective. Foresight, ahead-of-print. https://doi.org/10.1108/FS-05-2019-0040
[2]. Plihal, T. (2021). Scheduled macroeconomic news announcements and Forex volatility forecasting. Journal of Forecasting, 40(8), 1379{1397. https://doi.org/10.1002/FOR.2773
[3]. Saad, S., Ahmad, N., & Maheran, M. J. (2017). Assessing stock market volatility for different sectors in Malaysia. Pertanika Journal of Science and Technology, 25, 631-648.
[4]. Chung, K. H., & Chuwonganant, C. (2018). Market volatility and stock returns: The role of liquidity providers. Journal of Financial Markets, 37, 17{34. https://doi.org/10.1016/J. FINMAR.2017.07.002
[5]. Zheng, Z., Qiao, Z., Takaishi, T., Stanley, H. E., & Li, B. (2014). Realized volatility and absolute return volatility: A comparison indicating market risk. PLOS ONE, 9(7), e102940. https://doi. org/10.1371/journal.pone.0102940
[6]. Huang, S., Liu, N., & Wang, Z. (2023). Empirical analysis of SSE 50 index volatility based on GARCH model. Fluctuation and Noise Letters, 24(4), 2440010. https://doi.org/10.1142/ S0219477524400108
[7]. Su, Z., Fang, T., & Yin, L. (2018). Does NVIX matter for market volatility? Evidence from Asia- Pacific markets. Physica A: Statistical Mechanics and Its Applications, 492, 506{516. https:// doi.org/10.1016/j.physa.2017.10.025
[8]. Gabriel, V. (2015). Sensitivity, persistence and asymmetric effects in international stock market volatility during the global financial crisis. Revista de Metodos Cuantitativos para la Economia y la Empresa, 16, 42{65.
[9]. Bhansali, V., & Harris, L. (2018). Everybody's doing it: Short volatility strategies and shadow financial insurers. SSRN Electronic Journal. https://doi.org/10.2139/ssrn.3071457
[10]. Naveen Kumar, P., & Minithra, R. (2021). Estimation of price volatility of Nifty 50 index using ADF and GARCH (1, 1). Indian Journal of Economics and Development, 17(2), 480-485. https://doi.org/10.35716/IJED/20286
[11]. Hewamana, R., Siriwardhane, D., & Rathnayake, A. (2022). Determinants of stock price volatility: A literature review. Sri Lanka Accounting Forum, 2(1), 44. doi:10.4038/sajf.v2i1.44
[12]. Damiran, S., Dorjdagva, O., Sukhee, B., & Myagmarsuren, T. (2022). Macroeconomic determinants of stock market volatility: Evidence from post-socialist countries. Journal of Eastern European and Central Asian Research, 9(4), 569-580. https://doi.org/10.15549/jeecar. v9i4.966
[13]. Ajao, M. G., Fredrick, E., & Robinson, F. (2022). Dividend policy determinants and stock price volatility in selected African stock markets. International Journal of Finance Research, 3(1), 27-48. https://doi.org/10.47747/ijfr. v3i1.659
[14]. Sutrisno, B. (2020). The determinants of stock price volatility in Indonesia. Economic Analysis Journal, 3(1), 73-79. https://doi. org/10.32493/eaj.v3i1.y2020.p73-79
[15]. Sun, Y., Liu, B., & Prodromou, T. (2021). The determinants of the COVID-19 related stock price overreaction and volatility. Studies in Economics and Finance. https://doi. org/10.1108/SEF-08-2021-0330
[16]. Van der Ploeg, A. P. C. (2004). Multifactor volatility models: Evidence from stock and option markets.
[17]. Ghosh, I., & Datta Chaudhuri, T. (2016). Understanding and forecasting stock market volatility through wavelet decomposition, statistical learning and econometric methods. SSRN Electronic Journal. https://doi. org/10.2139/ssrn.2930876
[18]. Situm, M. (2010). Time series volatility forecasting using linear regression and GARCH. SSRN Electronic Journal. https://doi. org/10.2139/ssrn.1549385
[19]. Popovici, O. C. (2015). A volatility analysis of the euro currency and the bond market. Economic Computation and Economic Cybernetics Studies and Research, 49(1), 67-79.
[20]. Amiram, D., Cserna, B., & Levy, A. (2016). Volatility, liquidity, and liquidity risk. SSRN Electronic Journal. https://doi.org/10.2139/ ssrn.2618424
[21]. Adam, K., Marcet, A., & Nicolini, J. P. (2008). Stock market volatility and learning. Research Papers in Economics.
[22]. De Lepper, M. R. (2016). Predictive analysis for financial volatility.
[23]. Smith, T. A., Caligiuri, A., & Montana, J. R. (2018). Using a multiple linear regression model to calculate stock market volatility. International Journal of Mathematics Trends and Technology, 57(4), 220-224. https://doi. org/10.14445/22315373/IJMTT-V57P531
[24]. Ou, C., Frausto-Solis, J., & Vazquez-Rodarte, I. (2015). Volatility forecasting using support vector regression and a hybrid genetic algorithm. Computing in Economics and Finance, 45(1), 111-133. https://doi.org/10.1007/S10614- 013-9411-X
[25]. Santamaria-Bonfil, G., Frausto-Solis, J., & Vazquez-Rodarte, I. (2015). Volatility forecasting using support vector regression and a hybrid genetic algorithm. Computing in Economics and Finance, 45(1), 111-133. https://doi. org/10.1007/S10614-013-9411-X
[26]. Rekabsaz, N., Lupu, M., Baklanov, A., DuÄr, A., Andersson, L., & Hanbury, A. (2017). Volatility prediction using financial disclosures sentiments with word embedding-based IR models. Proceedings of the 55th Annual Meeting of the Association for Computational Linguistics, 157-164. https://doi.org/10.18653/v1/P17- 1157
[27]. Douglas, K., Ziegelmann, F. A., & dos Santos, F. A. (2014). Volatility forecasting via MIDAS, HAR and their combination: An empirical comparative study for IBOVESPA. Journal of Forecasting, 33(3), 192-207. https://doi. org/10.1002/for.2287
[28]. Burtniak, I., & Suduk, N. (2022). Model 16 of determining stock market volatility. Visnyk Khmelnytskoho Natsionalnoho Universytetu, 302(1), 316-320. https://doi. org/10.31891/2307-5740-2022-302-1-53
[29]. Arevalo, J. L. S., de Souza, G. M., & Meurer, R. M. (2020). The Brazilian stock market indicator: Determinants to measure variation and direction. International Journal of Statistics and Management Systems, 3(5), 48-59. https:// doi.org/10.51386/25815946/IJSMS-V3I5P105
[30]. Mehmood, A., Ullah, M. H., & Sabeeh, N. U. (2019). Determinants of stock price volatility: Evidence from the cement industry. Accounting, 5(4), 145-152. https://doi.org/10.5267/j. ac.2019.02.002
[31]. Badshah, I. (2013). Quantile regression analysis of the asymmetric return-volatility relation. Journal of Futures Markets, 33(3), 235-265. https://doi.org/10.1002/fut.21551
[32]. Padmakumari, L., & Maheswaran, S. (2016). A regression-based approach to capturing the level dependence in the volatility of stock returns. Asian Economic and Financial Review, 6(12), 706-718. https://doi.org/10.18488/journal. aefr/2016.6.12/102.12.706.718
[33]. Sen, R. (2009). Functional data analysis for volatility. SSRN Electronic Journal.
[34]. Zhang, C. (2019). Continuous-time volatility regression in large panels. SSRN Electronic Journal. https://doi.org/10.2139/ ssrn.3385804
[35]. Makau, A. (2017). Multivariate regression analysis of oil price volatility on GDP growth in Kenya. American Journal of Theoretical and Applied Statistics, 6(1), 44. https://doi. org/10.11648/j.ajtas.20170601.16
[36]. Josheski, D., & Lazarov, D. (2012). Exchange rate volatility and trade: A meta-regression analysis. SSRN Electronic Journal. https:// doi.org/10.2139/ssrn.2077678
[37]. Allen, D. E., Singh, A. K., Powell, R., McAleer, M., Taylor, J. E., & Thomas, L. C. (2012). The volatility-return relationship: Insights from linear and non-linear quantile regressions. Research Papers in Economics.
[38]. Waweru, N. N. (2013). Analysis of the determinants of stock price volatility at Nairobi Securities Exchange.
[39]. Parkinson, M. (1980). The Extreme Value Method for Estimating the Variance of the Rate of Return. The Journal of Business, 53(1), 61-65. http://www.jstor.org/stable/2352357
[40]. Jolliffe, I. (2013). Principal component analysis. Springer New York.
[41]. Hastie, T., Friedman, J., & Tibshirani, R. (2001). The elements of statistical learning. Springer. https://doi.org/10.1007/978-0-387-21606-5
[42]. Sakamoto, Y., et al. (2024). Applications of the elbow method in K-means clustering.
[43]. Umargono, E., Suseno, J., & Gunawan, S. K. (2020). K-means clustering optimization using the elbow method and early centroid determination based on mean and median formula. Proceedings of the International Conference on Engineering, Technology and Industrial Application, 19-26. https://doi. org/10.2991/assehr.k.201010.019
[44]. Rousseeuw, P. J. (1987). Silhouettes: A graphical aid to the interpretation and validation of cluster analysis. Journal of Computational and Applied Mathematics, 20, 53-65.
[45]. Lenssen, L., & Schubert, E. (2024). Medoid silhouette clustering with automatic cluster number selection. Information Systems, 120, 102290.
[46]. Davies, D. L., & Bouldin, D. W. (1979). A cluster separation measure. IEEE Transactions on Pattern Analysis and Machine Intelligence, PAMI-1(2), 224-227.
[47]. Wijaya, Y. A., et al. (2021). Cluster validation techniques in data analysis using Davies- Bouldin Index. Proceedings of the International Conference on Industrial Technology and Application, 101-109.
[48]. Sarikovanlik, V., Koy, A., Akkaya, M., Yildirim, H.H., & Kantar, L. (2019). Finans Biliminde Ekonometri Uygulamalari, Seckin Yayincilik, Ankara.
[49]. Breusch, T.S., & Pagan, A. R. (1980). The Lagrange Multiplier Test and Its Applications to Model Specification in Econometrics. The Review of Economic Studies, 239-253. https:// doi.org/10.2307/2297111
[50]. Peseran, M. H. H. M. (2004). General Diagnostic Test for Cross Section Dependence in Panels. Working Paper, University of Cambridge, Cambridge, United Kingdom.
[51]. Harris, R. D. F., & Tzavalis, E. (1999). Inference for Unit Roots in Dynamic Panels Where The Time Dimension is Fixed, Journal of Econometrics, 91, 201-226. https://doi. org/10.1016/S0304-4076(98)00076-1
[52]. Hausman, J. A. (1978). Specification Test in Econometrics, Econometrica, 46(6), 1251-1271.
[53]. Tatog¸lu, F. Y. (2012). Panel Veri Ekonometrisi, Beta Yayincilik, Istanbul.
[54]. Akin, I., & Akin, M. (2024). Behavioral finance impacts on US stock market volatility: An analysis of market anomalies. Behavioural Public Policy, 1-25. https://doi.org/10.1017/bpp.2024.13
[55]. Dichev, I. D., & Zheng, X. (2024). The volatility of stock investor returns. Journal of Financial Markets, 70, 100927. https://doi.org/10.1016/j. finmar.2024.100927
[56]. Nur, T., Ege, I_., & Topalog¸lu, E. E. (2023). The moderator role of managerial overconfidence in the relationship between r&d volatility and firm value: An application in borsa istanbul. Akademik Arastirmalar ve Calismalar Dergisi (AKAD), 15(28), 1-11. https://doi.org/10.20990/ kilisiibfakademik.1232263
[57]. Gahlot, P., Sachdeva, K., Agnihotri, S., & Giri, J. N. (2024). Influence of behavioral biases on investor decision-making in delhi-ncr. Icinde R. Sharma & K. Mehta (Ed.), Deep Learning Tools for Predicting Stock Market Movements (1. bs, ss. 363-389). Wiley. https://doi.org/10.1002/9781394214334.ch15
[58]. Takahashi, H. (2013). Analyzing the influence of Value at Risk on financial markets through agent-based modeling. International Journal of Knowledge-based and Intelligent Engineering Systems, 17(4), 257-266. https://doi.org/10.3233/KES-130276
[59]. Bulut, E. (2024). Sectoral volatility in borsa istanbul: A garch-based comparative analysis. Ahi Evran UÄniversitesi Sosyal Bilimler EnstituÄsuÄ Dergisi, 10(2), 507-522. https://doi. org/10.31592/aeusbed.1355079
[60]. Ikizlerli, D. (2022). The relation between trading volume and return volatility: Evidence from borsa istanbul. Business and Economics Research Journal, 4. https://doi.org/10.20409/ berj.2022.392
[61]. Kaya, A., & Yarbasi, I_. Y. (2021). Forecasting of volatility in stock exchange markets by ms-garch approach: An application of borsa istanbul. Ekonomi, Politika & Finans Arastirmalari Dergisi. https://doi.org/10.30784/ epfad.740815