Global convergence property with inexact line search for a new conjugate gradient method
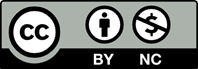
To develop new conjugate gradient (CG) methods that are both theoretically robust and practically effective for solving unconstrained optimization problems, we propose novel hybrid conjugate gradient algorithms. In these algorithms, the scale parameter βk is defined as a convex combination of βkHZ (Hager and Zhang (HZ)) and βkBA (Al-Bayati and Al-Assady (BA)). In one hybrid algorithm, the parameter in the convex combination is determined to satisfy the conjugacy condition, independent of the line search.In the other algorithm, the parameter is computed to ensure that the conjugate gradient direction aligns with the Newton direction. Under certain conditions, the proposed methods guarantee a sufficient descent at each iteration and exhibit global convergence properties. Furthermore, numerical results demonstrate that the hybrid computational scheme based on the conjugacy condition is efficient and performs favorably compared to some well-known algorithms.
[1] Liu, J. K., & Li, S. J. New hybrid conjugate gradient method for unconstrained optimization. (2014). Applied Mathematics and Computation, 245, 36-43. https://doi.org/10.1016/j.amc.2014.07.096
[2] Luo, C., Wang, L., Xie, Y., & Chen, B. (2024). A new conjugate gradient method for moving force identification of vehicle-bridge system. Journal of Vibration Engineering & Technologies, 12(1), 19-36. https://doi.org/10.1007/s42417-022-00824-1
[3] Yuan, Y., Tsang, D. H., & Lau, V. K. (2024). Combining Conjugate Gradient and Momentum for Unconstrained Stochastic Optimization With Applications to Machine Learning. IEEE Internet of Things Journal.
[4] Hanachi, S. B., Sellami, B., & Belloufi, M. (2024). A new family of hybrid conjugate gradient method for unconstrained optimization and its application to regression analysis. RAIRO-Operations Research. 58(1), 613-627 https://doi.org/10.1051/ro/2023196
[5] Jiang, X., Pan, L., Liu, M., & Jian, J. (2024). A family of spectral conjugate gradient method with strong convergence and its applications in image restoration and machine learning. Journal of the Franklin Institute, 107033. https://doi.org/10.1016/j.jfranklin.2024.107033
[6] Balaram, B., Narayanan, M. D., & Rajendrakumar, P. K. (2012). Optimal design of multiparametric nonlinear systems using a parametric continuation based genetic algorithm approach. Nonlinear Dynamics, 67(4), 2759-2777. https://doi.org/10.1007/s11071-011-0187-z
[7] Hestenes, M. R., & Stiefel, E. (1952). Methods of Conjugate Gradients for Solving. Journal of Research of the National Bureau of Standards, 49(1), 409-436. https://doi.org/10.6028/jres.049.044
[8] Fletcher, R., & Reeves, C. M. (1964). Function minimization by conjugate gradients. The computer journal, 7(2), 149-154. https://doi.org/10.1093/comjnl/7.2.149
[9] Salleh, Z., & Alhawarat, A. (2016). An efficient modification of the Hestenes-Stiefel nonlinear conjugate gradient method with restart property. Journal of Inequalities and Applications, 110. https://doi.org/10.1186/s13660-016-1049-5
[10] Wei, Z., Li, G., and Qi, L. (2006). New nonlinear conjugate gradient formulas for large-scale unconstrained optimization problems. Applied Mathematics and Computation, 179(2), 407-430. https://doi.org/10.1016/j.amc.2005.11.150
[11] Yuan, G., Wei, Z., & Zhao, Q. (2014). A modified Polak–Ribi´ere–Polyak conjugate gradient algorithm for large-scale optimization problems. IIE Transactions, 46(4), 397-413. https://doi.org/10.1080/0740817X.2012.726757
[12] Wolfe, P. (1969). Convergence conditions for ascent methods. Society for Industrial and Applied Mathematics Review, 11(2), 226-235. https://doi.org/10.1137/1011036
[13] Wolfe, P. (1971). Convergence conditions for ascent methods. II: Some corrections. Society for Industrial and Applied Mathematics review, 13(2), 185-188. https://doi.org/10.1137/1013035
[14] Dai, Y. H., Yuan, Y. (1999). A nonlinear conjugate gradient method with a strong global convergence property. Society for Industrial and Applied Mathematics Journal on optimization, 10(1), 177-182. https://doi.org/10.1137/S1052623497318992
[15] Liu, Y., & Storey, C. (1991). Efficient generalized conjugate gradient algorithms, Part 1: Theory. Journal of Optimization Theory and Applications, 69, 129-137. https://doi.org/10.1007/BF00940464
[16] Ibrahim, S. M., Yakubu, U. A., & Mamat, M. (2020). Application of spectral conjugate gradient methods for solving unconstrained optimization problems. An International Journal of Optimization and Control: Theories & Applications (IJOCTA), 10(2), 198-205. https://doi.org/10.11121/ijocta.01.2020.00859
[17] Kaelo, P., Narayanan, S., & Thuto, M. V. (2017). A modified quadratic hybridization of Polak-Ribiere-Polyak and Fletcher-Reeves conjugate gradient method for unconstrained optimization problems. An International Journal of Optimization and Control: Theories & Applications (IJOCTA), 7(2), 177-185. https://doi.org/10.11121/ijocta.01.2017.00339
[18] Belloufi, M., Rachid, B., & Yamina, L. (2013). Modi cation of the Armijo line search to satisfy the convergence properties of HS method. An International Journal of Optimization and Control: Theories & Applications (IJOCTA), 3(2), 145-152. https://doi.org/10.11121/ijocta.01.2013.00141
[19] Hamel, N., Benrabia, N., Ghiat, M., & Guebbai, H. (2024). Developing a new conjugate gradient algorithm with the bebefit of some desirable properties of the newton algorithm for unconstrained optimization. Journal of Applied Analysis and Computation, 14(1), 458-472. https://doi.org/10.11948/20230268
[20] Hallal, A., Belloufi, M., & Sellami, B. (2024). A new hybrid CG method as convex combination. Mathematical Foundations of Computing, 7(4), 522-530. https://doi.org/10.3934/mfc.2023028
[21] Guefassa, I., Chaib, Y., & Bechouat, T. (2023). A hybrid conjugate gradient method between MLS and FR in nonparametric statistics. Communications in Combinatorics and Optimization.
[22] Delladji, S., Belloufi, M., & Sellami, B. (2021). Behavior of the combination of PRP and HZ methods for unconstrained optimization. Numerical Algebra, Control and Optimization, 11(3), 377-389. https://doi.org/10.3934/naco.2020032
[23] Delladji, S., Belloufi, M., & Sellami, B. (2021). New hybrid conjugate gradient method as a convex combination of FR and BA methods. Journal of Information and Optimization Sciences, 42(3), 591-602. https://doi.org/10.1080/02522667.2020.1778841
[24] Djordjevi´c, S. S. (2018). New hybrid conjugate gradient method as a convex combination of HS and FR conjugate gradient methods. Journal of Applied Mathematics and Computation, 2, 366- 378. https://doi.org/10.26855/jamc.2018.09.002
[25] Al-Bayati, A. Y., & Al-Assady, N. H. (1986). Conjugate gradient method. Technical Research. School of Computer Studies, Leeds University, UK.
[26] Hager, W. W., & Zhang, H. (2005). A new conjugate gradient method with guaranteed descent and an efficient line search. Society for Industrial and Applied Mathematics Journal on Optimization, 16(1), 170-192. https://doi.org/10.1137/030601880
[27] Andrei, N. (2009). New hybrid conjugate gradient algorithms for unconstrained optimization. Advanced Modeling and Optimization, 8-10.
[28] Bongartz, I., Conn, A. R., Gould, N., & Toint, P. L. (1995). CUTE: constrained and unconstrained testing environments. ACM Transactions on Mathematical Software (TOMS), 21(1), 123-160. https://doi.org/10.1145/200979.201043
[29] Delladji, S., Belloufi, M., & Sellami, B. (2021). New hybrid conjugate gradient method as a convex combination of FR and BA methods. Journal of Information and Optimization Sciences, 42(3), p. 591-602. https://doi.org/10.1080/02522667.2020.1778841
[30] Djordjevi´c, S. S. (2016). New hybrid conjugate gradient method as a convex combination of FR and PRP methods. Filomat, 30(11), 3083-3100. https://doi.org/10.2298/FIL1611083D
[31] Hamdi, H., Sellami, B., & Bellofi, M. (2020). A new hybrid conjugate gradient method as a convex combination of DY and BA methods. Communications in Optimization Theory, 2020, 1-9.
[32] Andrei, N. (2008). An unconstrained optimization test functions collection. Advanced Modeling and Optimization, 10, 147-161.
[33] Dolan, E. D., & Mor´e, J. J. (2002). Benchmarking optimization software with performance profiles. Mathematical Programming, 91, 201-213. https://doi.org/10.1007/s101070100263
[34] Dai, Y. H., & Yuan, Y. (2001). An efficient hybrid conjugate gradient method for unconstrained optimization. Annals of Operations Research, 103, 33-47. https://doi.org/10.1023/A:1012930416777
[35] Hanachi, S. B., Sellami, B., & Belloufi, M. (2022). New iterative conjugate gradient method for nonlinear unconstrained optimization. RAIRO-Operations Research, 56(4), 2315-2327. https://doi.org/10.1051/ro/2022109
[36] Chaib, Y., & Bechouat, T. (2023). Two modified conjugate gradient methods for solving unconstrained optimization and application. RAIRO-Operations Research, 57(2), 333-350. https://doi.org/10.1051/ro/2023010