Rethinking industrial artificial intelligence: A unified foundation framework
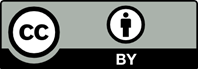
Recent advancements in industrial artificial intelligence (AI) are reshaping the industry by driving smarter manufacturing, predictive maintenance, and intelligent decision-making. However, existing approaches often focus primarily on algorithms and models while overlooking the importance of systematically integrating domain knowledge, data, and models to develop more comprehensive and effective AI solutions. Therefore, the effective development and deployment of industrial AI require a more comprehensive and systematic approach. To address this gap, this paper reviews previous research, rethinks the role of industrial AI, and proposes a unified industrial AI foundation framework comprising three core modules: the knowledge module, data module, and model module. These modules help to extend and enhance the industrial AI methodology platform, supporting various industrial applications. In addition, a case study on rotating machinery diagnosis is presented to demonstrate the effectiveness of the proposed framework, and several future directions are highlighted for the development of the industrial AI foundation framework.
- Jan Z, Ahamed F, Mayer W, et al. Artificial intelligence for industry 4.0: Systematic review of applications, challenges, and opportunities. Expert Syst Appl. 2022;216:119456. doi: 10.1016/j.eswa.2022.119456
- Peres RS, Jia X, Lee J, Sun K, Colombo AW, Barata J. Industrial artificial intelligence in industry 4.0 - systematic review, challenges and outlook. IEEE Access. 2020;8: 220121-220139. doi: 10.1109/access.2020.3042874
- Leng J, Zhu X, Huang Z, et al. Unlocking the power of industrial artificial intelligence towards Industry 5.0: Insights, pathways, and challenges. J Manuf Syst. 2024;73:349-363. doi: 10.1016/j.jmsy.2024.02.010
- Lee J, Davari H, Singh J, Pandhare V. Industrial artificial intelligence for industry 4.0-based manufacturing systems. Manuf Lett. 2018;18:20-23. doi: 10.1016/j.mfglet.2018.09.002
- Sisinni E, Saifullah A, Han S, Jennehag U, Gidlund M. Industrial internet of things: Challenges, opportunities, and directions. IEEE Trans Industr Inform. 2018;14(11): 4724-4734. doi: 10.1109/tii.2018.2852491
- Khalil RA, Saeed N, Masood M, Fard YM, Alouini MS, Al-Naffouri TY. Deep learning in the industrial internet of Things: Potentials, challenges, and emerging applications. IEEE Internet Things J. 2021;8(14):11016-11040. doi: 10.1109/jiot.2021.3051414
- Lee J, Su H. A unified industrial large knowledge model framework in Industry 4.0 and smart manufacturing. Int J AI Mater Des. 2024;1(2):41-47. doi: 10.36922/ijamd.3681
- Chang Y, Wang X, Wang J, et al. A survey on evaluation of large language models. ACM Trans Intell Syst Technol. 2024;15(3):1-45. doi: 10.1145/3641289
- Zio E. Prognostics and Health Management (PHM): Where are we and where do we (need to) go in theory and practice. Reliab Eng Syst Saf. 2021;218:108119. doi: 10.1016/j.ress.2021.108119
- Su H, Lee J. Machine learning approaches for diagnostics and prognostics of industrial systems using open source data from PHM data challenges: A review. Int J Progn Health Manag. 2024;15(2):1-26. doi: 10.36001/ijphm.2024.v15i2.3993
- Yan J, Meng Y, Lu L, Li L. Industrial big data in an industry 4.0 environment: Challenges, schemes, and applications for predictive maintenance. IEEE Access. 2017;5:23484-23491. doi: 10.1109/access.2017.2765544
- Wang J, Xu C, Zhang J, Zhong R. Big data analytics for intelligent manufacturing systems: A review. J Manuf Syst. 2021;62:738-752. doi: 10.1016/j.jmsy.2021.03.005
- Pivoto DGS, de Almeida LFF, da Rosa Righi R, Rodrigues JJPC, Lugli AB, Alberti AM. Cyber-physical systems architectures for industrial internet of things applications in Industry 4.0: A literature review. J Manuf Syst. 2021;58:176-192. doi: 10.1016/j.jmsy.2020.11.017
- Lee J, Bagheri B, Kao HA. A cyber-physical systems architecture for industry 4.0-based manufacturing systems. Manuf Lett. 2014;3:18-23. doi: 10.1016/j.mfglet.2014.12.001
- Zhang X, Ming X, Liu Z, Yin D, Chen Z, Chang Y. A reference framework and overall planning of industrial artificial intelligence (I-AI) for new application scenarios. Int J Adv Manuf Technol. 2018;101(9-12):2367-2389. doi: 10.1007/s00170-018-3106-3
- Yang T, Yi X, Lu S, Johansson KH, Chai T. Intelligent manufacturing for the process industry driven by industrial artificial intelligence. Engineering. 2021;7(9):1224-1230. doi: 10.1016/j.eng.2021.04.023
- Ahmed I, Jeon G, Piccialli F. From artificial intelligence to explainable artificial intelligence in industry 4.0: A survey on what, how, and where. IEEE Trans Industr Inform. 2022;18(8):5031-5042. doi: 10.1109/tii.2022.3146552
- Tao F, Zhang H, Liu A, Nee AYC. Digital twin in industry: State-of-the-art. IEEE Trans Industr Inform. 2019;15(4): 2405-2415. doi: 10.1109/tii.2018.2873186
- Lee J, Azamfar M, Singh J, Siahpour S. Integration of digital twin and deep learning in cyber-physical systems: Towards smart manufacturing. IET Collab Intell Manuf. 2020;2:34-36. doi: 10.1049/iet-cim.2020.0009
- Lee J, Gore P, Jia X, Siahpour S, Kundu P, Sun K. Stream-of-quality methodology for industrial internet-based manufacturing system. Manuf Lett. 2022;34:58-61. doi: 10.1016/j.mfglet.2022.09.004
- Buchgeher G, Gabauer D, Martinez-Gil J, Ehrlinger L. Knowledge graphs in manufacturing and production: A systematic literature review. IEEE Access. 2021;9:55537-55554. doi: 10.1109/access.2021.3070395
- Zhong L, Wu J, Li Q, Peng H, Wu X. A comprehensive survey on automatic knowledge graph construction. ACM Comput Surv. 2023;56(4):1-62. doi: 10.1145/3618295
- Pan S, Luo L, Wang Y, Chen C, Wang J, Wu X. Unifying large language models and knowledge graphs: A roadmap. IEEE Trans Knowl Data Eng. 2024;36(7):3580-3599. doi: 10.1109/tkde.2024.3352100
- Kirk JR, Wray RE, Lindes P, Laird JE. Improving knowledge extraction from LLMs for task learning through agent analysis. Proc AAAI Conf Artif Intell. 2024;38(16): 18390-18398. doi: 10.1609/aaai.v38i16.29799
- Han X, Cao S, Lv X, et al. OpenKE: An Open Toolkit for Knowledge Embedding. In: Proceedings of the 2018 Conference on Empirical Methods in Natural Language Processing: System Demonstrations. Association for Computational Linguistics; 2018. doi: 10.18653/v1/D18-2024
- Zhang H, Khashabi D, Song Y, Roth D. TransOMCS: From Linguistic Graphs to Commonsense Knowledge. In: Proceedings of the Twenty-Ninth International Joint Conference on Artificial Intelligence (IJCAI-20). 2020. doi: 10.24963/ijcai.2020/554
- Li Y, Zou L. gBuilder: A Scalable knowledge graph construction system for unstructured corpus. arXiv. 2023. doi: 10.48550/arXiv.2208.09705
- Brown T, Mann B, Ryder N, et al. Language models are few-shot learners. arXiv. 2020. doi: 10.48550/arXiv.2005.14165
- Achiam J, Adler S, Agarwal S, et al. GPT-4 technical report. arXiv. 2024. doi: 10.48550/arXiv.2303.08774
- Touvron H, Lavril T, Izacard G, et al. LLaMA: Open and efficient foundation language models. arXiv. 2023. doi: 10.48550/arXiv.2302.13971
- Touvron H, Martin L, Stone K, et al. LLaMA 2: Open foundation and fine-tuned chat models. arXiv. 2023. doi: 10.48550/arXiv.2307.09288
- Chowdhery A, Narang S, Devlin J, et al. PaLM: Scaling language modeling with pathways. arXiv. 2022. doi: 10.48550/arXiv.2204.02311
- Liu A, Feng B, Wang B, et al. DeepSeek-V2: A strong, economical, and efficient mixture-of-experts language model. arXiv. 2024. doi: 10.48550/arXiv.2405.04434
- Giner-Miguelez J, Gómez A, Cabot J. Using large language models to enrich the documentation of datasets for machine learning. arXiv. 2024. doi: 10.48550/arXiv.2404.15320
- Vaithilingam P, Zhang T, Glassman EL. Expectation vs. Experience: Evaluating the Usability of Code Generation Tools Powered by Large Language Models. In: CHI Conference on Human Factors in Computing Systems Extended Abstracts (CHI ’22 Extended Abstracts). 2022. doi: 10.1145/3491101.3519665
- Fan W, Ding Y, Ning L, et al. A survey on RAG Meeting LLMs: Towards Retrieval-Augmented Large Language Models. In: Proceedings of the 28th ACM SIGKDD Conference on Knowledge Discovery and Data Mining. 2024. p. 6491-6501. doi: 10.1145/3637528.3671470
- Strobelt H, Webson A, Sanh V, et al. Interactive and visual prompt engineering for ad-hoc task adaptation with large language models. IEEE Trans Vis Comput Graph. 2022;29:1146-1156. doi: 10.1109/tvcg.2022.3209479
- He Q, Zeng J, Huang W, et al. Can large language models understand real-world complex instructions? Proc AAAI Conf Artif Intell. 2024;38(16):18188-18196. doi: 10.1609/aaai.v38i16.29777
- Cofre-Martel S, Lopez Droguett E, Modarres M. Big machinery data preprocessing methodology for data-driven models in prognostics and health management. Sensors (Basel). 2021;21(20):6841. doi: 10.3390/s21206841
- Mohammed S, Budach L, Feuerpfeil M, et al. The effects of data quality on machine learning performance. arXiv. 2024. doi: 10.48550/arXiv.2207.14529
- Li P, Rao X, Blase J, Zhang Y, Chu X, Zhang C. CleanML: A Study for Evaluating the Impact of Data Cleaning on ML Classification Tasks. In: 2021 IEEE 37th International Conference on Data Engineering (ICDE). 2021. doi: 10.1109/icde51399.2021.00009
- Foroni D, Lissandrini M, Velegrakis Y. Estimating the Extent of the Effects of Data Quality through Observations. In: 2021 IEEE 37th International Conference on Data Engineering (ICDE). 2021. doi: 10.1109/icde51399.2021.00176
- Zheng A, Casari A. Feature Engineering for Machine Learning Principles and Techniques for Data Scientists. Beijing, Boston, Farnham: O’Reilly; 2018.
- Allen L, Atkinson J, Jayasundara D, Cordiner J, Moghadam PZ. Data visualization for Industry 4.0: A stepping-stone toward a digital future, bridging the gap between academia and industry. Patterns (N Y). 2021;2(5):100266. doi: 10.1016/j.patter.2021.100266
- Bertolini M, Mezzogori D, Neroni M, Zammori F. Machine learning for industrial applications: A comprehensive literature review. Expert Syst Appl. 2021;175:114820. doi: 10.1016/j.eswa.2021.114820
- Sarker IH. Deep learning: A comprehensive overview on techniques, taxonomy, applications and research directions. SN Comput Sci. 2021;2(6):420. doi: 10.1007/s42979-021-00815-1
- Xu Y, Kohtz S, Boakye J, Gardoni P, Wang P. Physics-informed machine learning for reliability and systems safety applications: State of the art and challenges. Reliab Eng Syst Saf. 2022;230:108900. doi: 10.1016/j.ress.2022.108900
- Li H, Zhang Z, Li T, Si X. A review on physics-informed data-driven remaining useful life prediction: Challenges and opportunities. Mech Syst Signal Process. 2024;209: 111120-111120. doi: 10.1016/j.ymssp.2024.111120
- Ektefaie Y, Dasoulas G, Noori A, Farhat M, Zitnik M. Multimodal learning with graphs. Nat Mach Intell. 2023;5(4):340-350. doi: 10.1038/s42256-023-00624-6
- Su H, Song B, Ahmed F. Multi-modal Machine Learning for Vehicle Rating Predictions Using Image, Text, and Parametric Data. In: International Design Engineering Technical Conferences and Computers and Information in Engineering Conference. Vol. 87295. American Society of Mechanical Engineers; 2023. doi: 10.1115/DETC2023-115076
- Zhang H, Semujju SD, Wang Z, et al. Large scale foundation models for intelligent manufacturing applications: A survey. J Intell Manuf. 2025:1-52. doi: 10.1007/s10845-024-02536-7
- Linardatos P, Papastefanopoulos V, Kotsiantis S. Explainable AI: A review of machine learning interpretability methods. Entropy (Basel). 2020;23(1):18. doi: 10.3390/e23010018
- Li X, Xiong H, Li X., Interpretable deep learning: Interpretation, interpretability, trustworthiness, and beyond. Knowl Inf Syst. 2022;64(12):3197-3234. doi: 10.1007/s10115-022-01756-8
- Lundberg SM, Lee SI. A Unified Approach to Interpreting Model Predictions. In: Advances in Neural Information Processing Systems 30 (NIPS 2017). Vol. 30. Curran Associates, Inc.; 2017.
- Ribeiro MT, Singh S, Guestrin C. “Why Should I Trust You?”: Explaining the Predictions of Any Classifier. In: Proceedings of the 22nd ACM SIGKDD International Conference on Knowledge Discovery and Data Mining - KDD ’16. 2016. p. 1135-1144. doi: 10.1145/2939672.2939778
- Li Z, Guo X, Qiang S. A survey of deep causal models and their industrial applications. Artif Intell Rev. 2024;57(11):298. doi: 10.1007/s10462-024-10886-0
- Singhal P, Walambe R, Ramanna S, Kotecha K. Domain adaptation: Challenges, methods, datasets, and applications. IEEE Access. 2023;11:6973-7020. doi: 10.1109/access.2023.3237025
- Li W, Huang R, Li J, et al. A perspective survey on deep transfer learning for fault diagnosis in industrial scenarios: Theories, applications and challenges. Mech Syst Signal Process. 2022;167:108487. doi: 10.1016/j.ymssp.2021.108487
- Banabilah S, Aloqaily M, Alsayed E, Malik N, Jararweh Y. Federated learning review: Fundamentals, enabling technologies, and future applications. Inf Process Manag. 2022;59(6):103061. doi: 10.1016/j.ipm.2022.103061
- Song Y, Wang T, Cai P, Mondal SK, Sahoo JP. A comprehensive survey of few-shot learning: Evolution, applications, challenges, and opportunities. ACM Comput Surv. 2023;55:1-40. doi: 10.1145/3582688
- Wang W, Zheng VW, Yu H, Miao C. A survey of zero-shot learning: Settings, methods, and applications. ACM Trans Intell Syst Technol. 2019;10(2):1-37. doi: 10.1145/3293318
- Hoi SCH, Sahoo D, Lu J, Zhao P. Online learning: A comprehensive survey. Neurocomputing. 2021;459(1):249-289. doi: 10.1016/j.neucom.2021.04.112
- Wang L, Zhang X, Su H, Zhu J. A comprehensive survey of continual learning: Theory, method and application. IEEE Trans Pattern Anal Mach Intell. 2024;46(8):5362-5383. doi: 10.1109/tpami.2024.3367329
- Yang L, Shami A. On hyperparameter optimization of machine learning algorithms: Theory and practice. Neurocomputing. 2020;415:295-316. doi: 10.1016/j.neucom.2020.07.061
- Elsken T, Metzen JH, Hutter F. Neural architecture search: A survey. J Mach Learn Res. 2019;20(55):1-21. doi: 10.48550/arXiv.1808.05377
- Deng BL, Li G, Han S, Shi L, Xie Y. Model compression and hardware acceleration for neural networks: A comprehensive survey. Proc IEEE. 2020;108(4):485-532. doi: 10.1109/jproc.2020.2976475
- Gou J, Gou J, Yu B, Maybank SJ, Tao D. Knowledge distillation: A survey. Int J Comput Vis. 2021;129(6):1789-1819. doi: 10.1007/S11263-021-01453-Z
- Kim S, Kim I, You D. Multi-condition multi-objective optimization using deep reinforcement learning. J Comput Phys. 2022;462:111263. doi: 10.1016/j.jcp.2022.111263
- Su H, Lee J. An advanced diagnostic model for gearbox degradation prediction under various operating conditions and degradation levels. Annu Conf PHM Soc. 2024;16(1). doi: 10.36001/phmconf.2024.v16i1.3869
- Vaerenberg R, Marx D, Hosseinli SA, et al. Preprocessing and modeling approach for gearbox pitting severity prediction under unseen operating conditions and fault severities. Int J Progn Health Manag. 2024;15(1):1-12. doi: 10.36001/ijphm.2024.v15i1.3808
- Gauriat CM, Pencolé Y, Ribot P, Brouillet G. Multi-class Neural Additive Models: An Interpretable Supervised Learning Method for Gearbox Degradation Detection. In: 2024 IEEE International Conference on Prognostics and Health Management (ICPHM). 2024. p. 41-48. doi: 10.1109/icphm61352.2024.10627522