Machine learning-driven prediction of gel fraction in conductive gelatin methacryloyl hydrogels
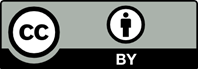
Gelatin methacryloyl (GelMA) hydrogels, combined with conductive fillers like Poly(3,4-ethylenedioxythiophene) polystyrene sulfonate (PEDOT:SPSS), present significant promise for tissue regeneration due to their biocompatibility, biodegradability, and electrical conductivity. However, optimizing the curing process of the hydrogel is challenging due to a lack of an existing model for gel fraction prediction. This complexity is further heightened when additional variables such as bioink formulation and crosslinking parameters are considered. This study leverages machine learning (ML) to predict the gel fraction of GelMA-PEDOT:SPSS hydrogel based on the combination of three types of features: Bioink formulation, crosslinking parameters, and absorption coefficient. The two key objectives of this study are to develop an ML model to predict gel fraction from bioink formulation and crosslinking parameters such as ultraviolet (UV) power intensity and UV irradiation duration, and to create an ML model to predict gel fraction through the absorption coefficient instead of crosslinking parameter. In the first ML model, support vector regression achieved the highest accuracy with a mean absolute percentage error (MAPE) of 3.13% and an R² of 0.79. This model allows the user to select optimum bioink formulation and crosslinking parameters to achieve the required gel fraction with minimal experiment. For the second ML model that utilizes a combination of absorption coefficient and bioink formulation, deep neural network models achieved a MAPE of 6.31% and an R² of 0.54. The absorption coefficient model shows promise for a non-destructive, real-time assessment of gel fraction, enabling more precise control over the hydrogel properties during the curing process. These results demonstrate ML’s capability to efficiently optimize hydrogel formulations, significantly cut down experimental efforts, and improve precision in 3D bioprinting and other hydrogel applications, thereby advancing the field of tissue regeneration.
- Wales DJ, Keshavarz M, Howe C, Yeatman E. 3D printability assessment of poly(octamethylene maleate (anhydride) citrate) and poly(ethylene glycol) diacrylate copolymers for biomedical applications. ACS Appl Polym Mater. 2022;4(8):5457-5470. doi: 10.1021/acsapm.2c00531
- Dutta SD, Ganguly K, Hexiu J, Randhawa A, Moniruzzaman M, Lim KT. A 3D bioprinted nanoengineered hydrogel with photoactivated drug delivery for tumor apoptosis and simultaneous bone regeneration via macrophage immunomodulation. Macromol Biosci. 2023;23(9):2300096. doi: 10.1002/mabi.202300096
- Ganguly S, Margel S. 3D printed magnetic polymer composite hydrogels for hyperthermia and magnetic field driven structural manipulation. Prog Polym Sci. 2022;131:101574. doi: 10.1016/j.progpolymsci.2022.101574
- Kurian AG, Singh RK, Patel KD, Lee JH, Kim HW. Multifunctional GelMA platforms with nanomaterials for advanced tissue therapeutics. Bioact Mater. 2022;8:267-295. doi: 10.1016/j.bioactmat.2021.06.027
- Yang X, Sun X, Liu J, et al. Photo-crosslinked GelMA/ collagen membrane loaded with lysozyme as an antibacterial corneal implant. Int J Biol Macromol. 2021;191:1006-1016. doi: 10.1016/j.ijbiomac.2021.09.144
- Shin SR, Zihlmann C, Akbari M, et al. Reduced graphene oxide‐gelMA hybrid hydrogels as scaffolds for cardiac tissue engineering. Small. 2016;12(27):3677-3689. doi: 10.1002/smll.201600178
- Ku SH, Lee SH, Park CB. Synergic effects of nanofiber alignment and electroactivity on myoblast differentiation. Biomaterials. 2012;33(26):6098-6104. doi: 10.1016/j.biomaterials.2012.05.018
- Rowlands AS, Cooper-White JJ. Directing phenotype of vascular smooth muscle cells using electrically stimulated conducting polymer. Biomaterials. 2008;29(34):4510-4520. doi: 10.1016/j.biomaterials.2008.07.052
- Zhu W, Ye T, Lee SJ, et al. Enhanced neural stem cell functions in conductive annealed carbon nanofibrous scaffolds with electrical stimulation. Nanomedicine. 2018;14(7):2485-2494. doi: 10.1016/j.nano.2017.03.018
- Chen C, Bai X, Ding Y, Lee IS. Electrical stimulation as a novel tool for regulating cell behavior in tissue engineering. Biomater Res. 2019;23(1):25. doi: 10.1186/s40824-019-0176-8
- Levin M, Stevenson CG. Regulation of cell behavior and tissue patterning by bioelectrical signals: Challenges and opportunities for biomedical engineering. Annu Rev Biomed Eng. 2012;14:295-323. doi: 10.1146/annurev-bioeng-071811-150114
- Dvir T, Timko BP, Brigham MD, et al. Nanowired three-dimensional cardiac patches. Nat Nanotechnol. 2011;6(11):720-725. doi: 10.1038/nnano.2011.160
- Irimia-Vladu M. “Green” electronics: Biodegradable and biocompatible materials and devices for sustainable future. Chem Soc Rev. 2014;43(2):588-610. doi: 10.1039/C3CS60235D
- Sun K, Zhang S, Li P, et al. Review on application of PEDOTs and PEDOT: PSS in energy conversion and storage devices. J Mater Sci Mater Electron. 2015;26:4438-4462. doi: 10.1007/s10854-015-2895-5
- Heo DN, Acquah N, Kim J, Lee SJ, Castro NJ, Zhang LG. Directly induced neural differentiation of human adipose-derived stem cells using three-dimensional culture system of conductive microwell with electrical stimulation. Tissue Eng Part A. 2018;24(7-8):537-545. doi: 10.1089/ten.TEA.2017.0150
- Spencer AR, Shirzaei Sani E, Soucy JR, et al. Bioprinting of a cell-laden conductive hydrogel composite. ACS Appl Mater Interfaces. 2019;11(34):30518-30533. doi: 10.1021/acsami.9b07353
- Lee JJ, Ng HY, Lin YH, et al. The 3D printed conductive grooved topography hydrogel combined with electrical stimulation for synergistically enhancing wound healing of dermal fibroblast cells. Biomater Adv. 2022;142:213132. doi: 10.1016/j.bioadv.2022.213132
- Spencer AR, Primbetova A, Koppes AN, Koppes RA, Fenniri H, Annabi N. Electroconductive gelatin methacryloyl-PEDOT: PSS composite hydrogels: Design, synthesis, and properties. ACS Biomater Sci Eng. 2018;4(5):1558-1567. doi: 10.1021/acsbiomaterials.8b00135
- Zhang S, Chen Y, Liu H, et al. Room‐temperature‐formed PEDOT: PSS hydrogels enable injectable, soft, and healable organic bioelectronics. Adv Mater. 2020;32(1):1904752. doi: 10.1002/adma.201904752
- Fu F, Wang J, Zeng H, Yu J. Functional conductive hydrogels for bioelectronics. ACS Mater Lett. 2020;2(10):1287-1301. doi: 10.1021/acsmaterialslett.0c00309
- Sagdic K, Fernández-Lavado E, Mariello M, Akouissi O, Lacour SP. Hydrogels and conductive hydrogels for implantable bioelectronics. Mrs Bull. 2023;48(5):495-505. doi: 10.1557/s43577-023-00536-1
- Zhang H, Guo J, Wang Y, Sun L, Zhao Y. Stretchable and conductive composite structural color hydrogel films as bionic electronic skins. Adv Sci (Weinh). 2021;8(20):2102156. doi: 10.1002/advs.202102156
- Linde E, Celina MC, Appelhans LN, Roach DJ, Cook AW. In situ characterization of material extrusion printing by near-infrared spectroscopy. Addit Manuf. 2023;63:103420. doi: 10.1016/j.addma.2023.103420
- Camposeo A, Arkadii A, Romano L, et al. Impact of size effects on photopolymerization and its optical monitoring in-situ. Addit Manuf. 2022;58:103020. doi: 10.1016/j.addma.2022.103020
- Vallabh CK, Zhang Y, Zhao X. In-situ ultrasonic monitoring for vat photopolymerization. Add Manuf. 2022;55:102801. doi: 10.1016/j.addma.2022.102801
- El-Sherbiny IM, Yacoub MH. Hydrogel scaffolds for tissue engineering: Progress and challenges. Glob Cardiol Sci Pract. 2013;2013(3):316-342. doi: 10.5339/gcsp.2013.38
- Wu Y, Xiang Y, Fang J, et al. The influence of the stiffness of GelMA substrate on the outgrowth of PC12 cells. Biosci Rep. 2019;39(1):BSR20181748. doi: 10.1042/bsr20181748
- Pepelanova I, Kruppa K, Scheper T, Lavrentieva A. Gelatin-methacryloyl (GelMA) hydrogels with defined degree of functionalization as a versatile toolkit for 3d cell culture and extrusion bioprinting. Bioengineering (Basel). 2018;5(3):55. doi: 10.3390/bioengineering5030055
- Lantoine J, Grevesse T, Villers A, et al. Matrix stiffness modulates formation and activity of neuronal networks of controlled architectures. Biomaterials. 2016;89:14-24. doi: 10.1016/j.biomaterials.2016.02.041
- Monteiro N, Thrivikraman G, Athirasala A, et al. Photopolymerization of cell-laden gelatin methacryloyl hydrogels using a dental curing light for regenerative dentistry. Dent Mater. 2018;34(3):389-399. doi: 10.1016/j.dental.2017.11.020
- Annabi N, Tamayol A, Uquillas JA, et al. 25th anniversary article: Rational design and applications of hydrogels in regenerative medicine. Adv Mater. 2014;26(1):85-123. doi: 10.1002/adma.201303233
- Fairbanks BD, Schwartz MP, Bowman CN, Anseth KS. Photoinitiated polymerization of PEG-diacrylate with lithium phenyl-2,4,6-trimethylbenzoylphosphinate: Polymerization rate and cytocompatibility. Biomaterials. 2009;30(35):6702-6707. doi: 10.1016/j.biomaterials.2009.08.055
- Goh GL, Huang X, Toh W, et al. Joint angle prediction for a cable-driven gripper with variable joint stiffness through numerical modeling and machine learning. Int J AI Mater Des. 2024;1(1):2328. doi: 10.36922/ijamd.2328
- Goh GL, Goh GD, Pan JW, Teng PS, Kong PW. Automated service height fault detection using computer vision and machine learning for badminton matches. Sensors. 2023;23(24):9759. doi: 10.3390/s23249759
- Goh GD, Lee JM, Goh GL, Huang X, Lee S, Yeong WY. Machine learning for bioelectronics on wearable and implantable devices: Challenges and potential. Tissue Eng Part A. 2023;29(1-2):20-46. doi: 10.1089/ten.TEA.2022.0119
- Goh GL, Zhang H, Goh GD, Yeong WY, Chong TH. Multi-objective optimization of intense pulsed light sintering process for aerosol jet printed thin film. Mater Sci Addit Manuf. 2022;1(2):10. doi: 10.36922/msam.26
- Sinha AK, Goh GL, Yeong WY, Cai Y. Ultra‐low‐cost, crosstalk‐free, fast‐responding, wide‐sensing‐range tactile fingertip sensor for smart gloves. Adv Mater Interfaces. 2022;9(21):2200621. doi: 10.1002/admi.202200621
- Liang F, Valdes JP, Cheng S, et al. Liquid-liquid dispersion performance prediction and uncertainty quantification using recurrent neural networks. Ind Eng Chem Res. 2024;63(17):7853-7875. doi: 10.1021/acs.iecr.4c00014
- Cheng S, Quilodrán-Casas C, Ouala S, et al. Machine learning with data assimilation and uncertainty quantification for dynamical systems: A review. IEEE/CAA J Autom Sin. 2023;10(6):1361-1387. doi: 10.48550/arXiv.2303.10462
- Nathanael K, Cheng S, Kovalchuk NM, Arcucci R, Simmons MJ. Optimization of microfluidic synthesis of silver nanoparticles: A generic approach using machine learning. Chem Eng Res Des. 2023;193:65-74. doi: 10.1016/j.cherd.2023.03.007
- Xia Z, Ma K, Cheng S, et al. Accurate identification and measurement of the precipitate area by two-stage deep neural networks in novel chromium-based alloys. Phys Chem Chem Phys. 2023;25(23):15970-15987. doi: 10.1039/D3CP00402C
- Wei J, Chu X, Sun XY, et al. Machine learning in materials science. InfoMat. 2019;1(3):338-358. doi: 10.1002/inf2.12028
- Ng WL, Goh GL, Goh GD, Ten JS, Yeong WY. Progress and opportunities for machine learning in materials and processes of additive manufacturing. Adv Mater. 2024;e2310006. doi: 10.1002/adma.202310006
- Huang X, Ng WL, Yeong WY. Predicting the number of printed cells during inkjet-based bioprinting process based on droplet velocity profile using machine learning approaches. J Intell Manuf. 2023;35:2349-2364. doi: 10.1007/s10845-023-02167-4
- Shi J, Song J, Song B, Lu WF. Multi-objective optimization design through machine learning for drop-on-demand bioprinting. Engineering. 2019;5(3):586-593. doi: 10.1016/j.eng.2018.12.009
- Ning H, Zhou T, Joo SW. Machine learning boosts three-dimensional bioprinting. Int J Bioprint. 2023;9(4):739. doi: 10.18063/ijb.739
- Loessner D, Meinert C, Kaemmerer E, et al. Functionalization, preparation and use of cell-laden gelatin methacryloyl-based hydrogels as modular tissue culture platforms. Nat Protoc. 2016;11(4):727-746. doi: 10.1038/nprot.2016.037
- Ghosh RN, Thomas J, Vaidehi BR, et al. An insight into synthesis, properties and applications of gelatin methacryloyl hydrogel for 3D bioprinting. Mater Adv. 2023;4(22):5496-5529. doi: 10.1039/D3MA00715D
- Hogan NJ, Urban AS, Ayala-Orozco C, Pimpinelli A, Nordlander P, Halas NJ. Nanoparticles heat through light localization. Nano Lett. 2014;14(8):4640-4645. doi: 10.1021/nl5016975
- Pedregosa F, Varoquaux G, Gramfort A, et al. Scikit-learn: Machine learning in Python. J Mach Learn Res. 2011;12:2825-2830.
- Smola AJ, Schölkopf B. A tutorial on support vector regression. Stat Comput. 2004;14(3):199-222. doi: 10.1023/B: STCO.0000035301.49549.88
- Breiman L. Random forests. Mach Learn. 2001;45(1):5-32. doi: 10.1023/A:1010933404324
- Singh AV, Bhardwaj P, Upadhyay AK, et al. Navigating regulatory challenges in molecularly tailored nanomedicine. Explor Biomat X. 2024;1(2):124-134. doi: 10.37349/ebmx.2024.00009