Evaluating machine learning models for prediction of coronary artery disease
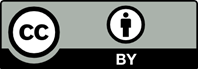
Coronary artery disease (CAD) is a prevailing global health issue and a leading cause of death worldwide. Its accurate and timely diagnosis is crucial for effectively managing the disease and improving patient outcomes. In this study, we conducted a comparative analysis of machine learning (ML)-based approaches to detect and diagnose CAD. A dataset of 918 instances from the UCI ML repository, comprising 11 typical risk factors and CAD predictors, was used for this investigation. The study deployed ML models in Google Colaboratory and PyCaret, testing their efficacy in diagnosing CAD. Our study provides a detailed overview of these ML methodologies, their strengths, and limitations, underscoring the potential of these algorithms to revolutionize CAD diagnosis and treatment. The overall goal of the study is to create a model that can predict the presence or chance of presence of CAD based on different parameters of the patient’s history. Findings include the showcased logistic regression model, which was proven to be particularly effective, with an area under curve of 0.88, indicating a high ability to differentiate between patients with and without CAD, and a successful ability to identify clinically key features of CAD such as the presence of exertional angina and chest pain. This study emphasizes the importance of further research in this field to establish ML as a cornerstone of modern healthcare diagnostics.
- World Health Organization. The Top 10 Causes of Death. Available from: https://www.who.int/news-room/fact-sheets/detail/the-top-10-causes-of-death [Last accessed on 2023 Dec 22].
- Ralapanawa U, Sivakanesan R. Epidemiology and the magnitude of coronary artery disease and acute coronary syndrome: A narrative review. J Epidemiol Glob Health. 2021;11(2):169-177. doi: 10.2991/jegh.k.201217.001
- Tsao CW, Aday AW, Almarzooq ZI, et al. Heart disease and stroke statistics-2023 update: A report from the American heart association. Circulation. 2023;147:e93-e621. doi: 10.1161/CIR.0000000000001123.
- Moriguchi JD, Kobashigawa JA, Ro TK, et al. At what creatinine level is angiographic dye safe for coronary angiography in cardiac transplant recipients? Transplantation. 1998;65(12):S160.
- Alizadehsani R, Abdar M, Roshanzamir M, et al. Machine learning-based coronary artery disease diagnosis: A comprehensive review. Comput Biol Med. 2019;111:103346. doi: 10.1016/j.compbiomed.2019.103346
- Alizadehsani R, Habibi J, Hosseini MJ, et al. A data mining approach for diagnosis of coronary artery disease. Comput Methods Programs Biomed. 2013;111(1):52-61. doi: 10.1016/j.cmpb.2013.03.004
- Goff DC Jr., Lloyd-Jones DM, Bennett G, et al. 2013 ACC/AHA guideline on the assessment of cardiovascular risk: A report of the American College of Cardiology/American Heart Association Task Force on Practice Guidelines. Circulation. 2014;129(25_suppl_2):S49-S73. doi: 10.1161/01.cir.0000437741.48606.98
- DeFilippis AP, Young R, Carrubba CJ, et al. An analysis of calibration and discrimination among multiple cardiovascular risk scores in a modern multiethnic cohort. Ann Intern Med. 2015;162(4):266-275. doi: 10.7326/M14-1281
- Toma M, Wei OC. Predictive modeling in medicine. Encyclopedia. 2023;3(2):590-601. doi: 10.3390/encyclopedia3020042
- Bekbolatova M, Mayer J, Ong CW, Toma M. Transformative potential of AI in healthcare: Definitions, applications, and navigating the ethical landscape and public perspectives. Healthcare (Basel). 2024;12(2):125. doi: 10.3390/healthcare12020125
- Abraham A, Jose R, Ahmad J, et al. Comparative analysis of machine learning models for image detection of colonic polyps vs. Resected polyps. J Imaging. 2023;9(10):215. doi: 10.3390/jimaging9100215
- Gautam N, Saluja P, Malkawi A, et al. Current and future applications of artificial intelligence in coronary artery disease. Healthcare (Basel). 2022;10(2):232. doi: 10.3390/healthcare10020232
- Davenport T, Kalakota R. The potential for artificial intelligence in healthcare. Future Healthc J. 2019;6(2):94-98. doi: 10.7861/futurehosp.6-2-94
- Javaid M, Haleem A, Pratap Singh R, Suman R, Rab S. Significance of machine learning in healthcare: Features, pillars and applications. Int J Intell Netw. 2022;3:58-73. doi: 10.1016/j.ijin.2022.05.002
- Srinivasan S, Gunasekaran S, Mathivanan SK, Malar MB, Jayagopal P, Dalu GT. An active learning machine technique based prediction of cardiovascular heart disease from UCI-repository database. Sci Rep. 2023;13:13588. doi: 10.1038/s41598-023-40717-1
- Garavand A, Behmanesh A, Aslani N, Sadeghsalehi H, Ghaderzadeh M. Towards diagnostic aided systems in coronary artery disease detection: A comprehensive multiview survey of the state of the art. Int J Intell Syst. 2023;2023:6442756. doi: 10.1155/2023/6442756
- Kakadiaris IA, Vrigkas M, Yen AA, Kuznetsova T, Budoff M, Naghavi M. Machine learning outperforms ACC/ AHA CVD risk calculator in MESA. J Am Heart Assoc. 2018;7(22):e009476. doi: 10.1161/JAHA.118.009476
- Baskaran L, Ying X, Xu Z, et al. Machine learning insight into the role of imaging and clinical variables for the prediction of obstructive coronary artery disease and revascularization: An exploratory analysis of the CONSERVE study. PLoS One. 2020;15:e0233791. doi: 10.1371/journal.pone.0233791
- Al’Aref SJ, Maliakal G, Singh G, et al. Machine learning of clinical variables and coronary artery calcium scoring for the prediction of obstructive coronary artery disease on coronary computed tomography angiography: Analysis from the CONFIRM registry. Eur Heart J. 2020;41:359-367. doi: 10.1093/eurheartj/ehz565
- Alaa AM, Bolton T, Di Angelantonio E, Rudd JHF, van der Schaar M. Cardiovascular disease risk prediction using automated machine learning: A prospective study of 423,604 UK Biobank participants. PLoS One. 2019;14(5):e0213653. doi: 10.1371/journal.pone.0213653
- Motwani M, Dey D, Berman DS, et al. Machine learning for prediction of all-cause mortality in patients with suspected coronary artery disease: A 5-year multicentre prospective registry analysis. Eur Heart J. 2017;38(7):500-507. doi: 10.1093/eurheartj/ehw188
- Forrest IS, Petrazzini BO, Duffy Á, et al. Machine learning-based marker for coronary artery disease: Derivation and validation in two longitudinal cohorts. Lancet. 2023;401(10372):215-225. doi: 10.1016/S0140-6736(22)02079-7
- Cho SY, Kim SH, Kang SH, et al. Pre-existing and machine learning-based models for cardiovascular risk prediction. Sci Rep. 2021;11(1):8886. doi: 10.1038/s41598-021-88257-w
- Lee YH, Tsai TH, Chen JH, et al. Machine learning of treadmill exercise test to improve selection for testing for coronary artery disease. Atherosclerosis. 2022;340:23-27. doi: 10.1016/j.atherosclerosis.2021.11.028
- Özbilgin F, Kurnaz Ç, Aydın, E. Prediction of coronary artery disease using machine learning techniques with iris analysis. Diagnostics. 2023;13(6):1081. doi: 10.3390/diagnostics13061081
- Sun Z, Silberstein J, Vaccarezza M. Cardiovascular computed tomography in the diagnosis of cardiovascular disease: Beyond lumen assessment. J Cardiovasc Dev Dis. 2024;11(1):22. doi: 10.3390/jcdd11010022
- Ahsan MM, Siddique Z. Machine learning-based heart disease diagnosis: A systematic literature review. Artif Intell Med. 2022;128:102289. doi: 10.1016/j.artmed.2022.102289
- Janosi A, Steinbrunn W, Pfisterer M, Detrano R. Heart Disease. UCI Machine Learning Repositoryl; 1988. doi: 10.24432/C52P4X
- Unknown. (n.d.). Statlog (Heart) [Dataset]. UCI Machine Learning Repository. doi: 10.24432/C57303
- Hicks SA, Strümke I, Thambawita V, et al. On evaluation metrics for medical applications of artificial intelligence. Sci Rep. 2022;12(1):5979. doi: 10.1038/s41598-022-09954-8
- Delgado R, Tibau XA. Why Cohen’s Kappa should be avoided as performance measure in classification. PLoS One. 2019;14(9):e0222916. doi: 10.1371/journal.pone.0222916
- Chicco D, Jurman G. The Matthews correlation coefficient (MCC) should replace the ROC AUC as the standard metric for assessing binary classification. BioData Min. 2023;16(1):4. doi: 10.1186/s13040-023-00322-4
- McHugh ML. Interrater reliability: The kappa statistic. Biochem Med (Zagreb). 2012;22(3):276-282.
- Xia Y. Correlation and association analyses in microbiome study integrating multiomics in health and disease. Prog Mol Biol Transl Sci. 2020;171:309-491. doi: 10.1016/bs.pmbts.2020.04.003
- Mao L. Matthews Correlation Coefficient; 2019. Available from: https://leimao.github.io/blog/matthews-correlation-coefficient [Last accessed on 2024 Jan 03].
- Schober P, Boer C, Schwarte LA. Correlation coefficients: Appropriate use and interpretation. Anesth Analg. 2018;126(5):1763-1768. doi: 10.1213/ANE.0000000000002864
- Nahm FS. Receiver operating characteristic curve: Overview and practical use for clinicians. Korean J Anesthesiol. 2022;75(1):25-36. doi: 10.4097/kja.21209
- Viering T, Loog M. The shape of learning curves: A review. IEEE Trans Pattern Anal Mach Intell. 2023;45(6):7799-7819. doi: 10.1109/TPAMI.2022.3220744
- Bengfort B, Gray L, Bilbro R, et al. Yellowbrick v1.5. Zenodo; 2022. Available from: https://www.scikit-yb.org/en/latest/ api/model_selection/learning_curve.html [Last accessed on 2024 Jan 04].
- Kanade VA. Logistic Regression: Equation, Assumptions, Types, and Best Practices. Austin: Spiceworks. Available from: https://www.spiceworks.com/tech/artificial-intelligence/ articles/what-is-logistic-regression [Last accessed on 2022 Apr 18].
- Rymarczyk T, Kozłowski E, Kłosowski G, Niderla K. Logistic regression for machine learning in process tomography. Sensors. 2019;19(15):3400. doi: 10.3390/s19153400
- Tharwat A, Gaber T, Ibrahim A, Hassanien AE. Linear discriminant analysis: A detailed tutorial. AI Commun. 2017;30:169-190. doi: 10.3233/AIC-170729
- Xanthopoulos P, Pardalos PM, Trafalis TB. Linear discriminant analysis. In: Robust Data Mining. SpringerBriefs in Optimization. New York: Springer; 2013. doi: 10.1007/978-1-4419-9878-1_4
- Peng C, Cheng Q. Discriminative ridge machine: A classifier for high-dimensional data or imbalanced data. IEEE Trans Neural Netw Learn Syst. 2021;32(6):2595-2609. doi: 10.1109/TNNLS.2020.3006877
- Singh A, Prakash BS, Chandrasekaran K. A Comparison of Linear Discriminant Analysis and Ridge Classifier on Twitter Data. In: 2016 International Conference on Computing, Communication and Automation (ICCCA). Greater Noida, India: IEEE; 2016. p. 133-138. doi: 10.1109/CCAA.2016.7813704
- Akella A, Akella S. Machine learning algorithms for predicting coronary artery disease: Efforts toward an open source solution. Future Science OA. 2021;7(6):FSO698. doi: 10.2144/fsoa-2020-0206