Genome-wide analysis identifies non-reference transposable element polymorphisms associated with Parkinson’s disease
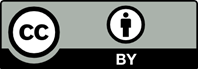
Parkinson’s disease (PD) is a common neurodegenerative disease that primarily affects the elderly, significantly impacting patients’ health and quality of life. While most genetic studies on PD have focused on single nucleotide polymorphisms, the effects of other forms of genomic variation in PD are yet to be fully elucidated. Transposable elements (TEs) are one of the main sources of human genome structural variation, with known associations with many human diseases. However, their potential connection to PD remains unclear. In this study, we investigated non-reference TE polymorphisms in three independent PD cohorts and explored their associations with both PD risk and progression. Our findings revealed that one non-reference TE is associated with the risk of PD, while two TEs are associated with disease progression. Furthermore, through expression quantitative trait locus (eQTL) analysis, we identified 18 cis TE-eQTLs in an interaction model and 290 cis TE-eQTLs in a non-interaction model. Several non-reference TE polymorphisms are correlated with specific PD-gene expression patterns in trans. These results indicate the feasibility of delving into the genetics of PD through the study of complex genomic variations. Advances in genomics research have the potential to deepen our understanding of this disease and pave the way for further translational medicine research in PD.
- Emamzadeh FN, Surguchov A, 2018, Parkinson’s disease: Biomarkers, treatment, and risk factors. Front Neurosci, 12: 612. https://doi.org/10.3389/fnins.2018.00612
- de Lau LM, Breteler MMB, 2006, Epidemiology of Parkinson’s disease. Lancet Neurol, 5: 525–535. https://doi.org/10.1016/S1474-4422(06)70471-9
- GBD 2016 Neurology Collaborators, 2019, Global, regional, and national burden of neurological disorders, 1990-2016: A systematic analysis for the Global Burden of Disease Study 2016. Lancet Neurol, 18: 459–480. https://doi.org/10.1016/S1474-4422(18)30499-X
- Dorsey ER, Bloem BR, 2018, The Parkinson pandemic-a call to action. JAMA Neurol, 75: 9–10. https://doi.org/10.1001/jamaneurol.2017.3299
- Armstrong MJ, Okun MS, 2020, Diagnosis and treatment of Parkinson disease: A review. JAMA, 323: 548–560. https://doi.org/10.1001/jama.2019.22360
- Schapira AHV, Chaudhuri KR, Jenner P, 2017, Non-motor features of Parkinson disease. Nat Rev Neurosci, 18: 435–450. https://doi.org/10.1038/nrn.2017.62
- Dickson DW, Braak H, Duda JE, et al., 2009, Neuropathological assessment of Parkinson’s disease: Refining the diagnostic criteria. Lancet Neurol, 8: 1150–1157. https://doi.org/10.1016/S1474-4422(09)70238-8
- Obeso JA, Stamelou M, Goetz CG, et al., 2017, Past, present, and future of Parkinson’s disease: A special essay on the 200th Anniversary of the Shaking Palsy. Mov Disord, 32: 1264–1310. https://doi.org/10.1002/mds.27115
- Poewe W, Seppi K, Tanner CM, et al., 2017, Parkinson disease. Nat Rev Dis Primers, 3: 17013. https://doi.org/10.1038/nrdp.2017.13
- Deng H, Wang P, Jankovic J, 2018, The genetics of Parkinson disease. Ageing Res Rev, 42: 72–85. https://doi.org/10.1016/j.arr.2017.12.007
- Polymeropoulos MH, Lavedan C, Leroy E, et al., 1997, Mutation in the alpha-synuclein gene identified in families with Parkinson’s disease. Science, 276: 2045–2047. https://doi.org/10.1126/science.276.5321.2045
- Kitada T, Asakawa S, Hattori N, et al., 1998, Mutations in the parkin gene cause autosomal recessive juvenile parkinsonism. Nature, 392: 605–608. https://doi.org/10.1038/33416
- Zimprich A, Biskup S, Leitner P, et al., 2004, Mutations in LRRK2 cause autosomal-dominant parkinsonism with pleomorphic pathology. Neuron, 44: 601–607. https://doi.org/10.1016/j.neuron.2004.11.005
- Fernandez-Santiago R, Sharma M, 2022, What have we learned from genome-wide association studies (GWAS) in Parkinson’s disease? Ageing Res Rev, 79: 101648. https://doi.org/10.1016/j.arr.2022.101648
- Nalls MA, Blauwendraat C, Vallerga CL, et al., 2019, Identification of novel risk loci, causal insights, and heritable risk for Parkinson’s disease: A meta-analysis of genome-wide association studies. Lancet Neurol, 18: 1091–1102. https://doi.org/10.1016/S1474-4422(19)30320-5
- Bustos BI, Billingsley K, Blauwendraat C, et al., 2023, Genome-wide contribution of common short-tandem repeats to Parkinson’s disease genetic risk. Brain, 146: 65–74. https://doi.org/10.1093/brain/awac301
- Le Guen Y, Napolioni V, Belloy ME, et al., 2021, Common X-chromosome variants are associated with Parkinson disease risk. Ann Neurol, 90: 22–34. https://doi.org/10.1002/ana.26051
- Burns KH, Boeke JD, 2012, Human transposon tectonics. Cell, 149: 740–752. https://doi.org/10.1016/j.cell.2012.04.019
- Nurk S, Koren S, Rhie A, et al., 2022, The complete sequence of a human genome. Science, 376: 44–53. https://doi.org/10.1126/science.abj6987
- Bourque G, Burns KH, Gehring M, et al., 2018, Ten things you should know about transposable elements. Genome Biol, 19: 199. https://doi.org/10.1186/s13059-018-1577-z
- Finnegan DJ, 1989, Eukaryotic transposable elements and genome evolution. Trends Genet, 5: 103–107. https://doi.org/10.1016/0168-9525(89)90039-5
- Wicker T, Sabot F, Hua-Van A, et al., 2007, A unified classification system for eukaryotic transposable elements. Nat Rev Genet, 8: 973–982. https://doi.org/10.1038/nrg2165
- Wells JN, Feschotte C, 2020, A field guide to eukaryotic transposable elements. Annu Rev Genet, 54: 539–561. https://doi.org/10.1146/annurev-genet-040620-022145
- Chuong EB, Elde NC, Feschotte C, 2017, Regulatory activities of transposable elements: From conflicts to benefits. Nat Rev Genet, 18: 71–86. https://doi.org/10.1038/nrg.2016.139
- Hoyt SJ, Storer JM, Hartley GA, et al., 2022, From telomere to telomere: The transcriptional and epigenetic state of human repeat elements. Science, 376: eabk3112. https://doi.org/10.1126/science.abk3112
- Mills RE, Bennett EA, Iskow RC, et al., 2007, Which transposable elements are active in the human genome? Trends Genet, 23: 183–191. https://doi.org/10.1016/j.tig.2007.02.006
- Kazazian HH Jr., Wong C, Youssoufian H, et al., 1988, Haemophilia A resulting from de novo insertion of L1 sequences represents a novel mechanism for mutation in man. Nature, 332: 164–166. https://doi.org/10.1038/332164a0
- Payer LM, Steranka JP, Yang WR, et al., 2017, Structural variants caused by Alu insertions are associated with risks for many human diseases. Proc Natl Acad Sci U S A, 114: E3984–E3992. https://doi.org/10.1073/pnas.1704117114
- Payer LM, Burns KH, 2019, Transposable elements in human genetic disease. Nat Rev Genet, 20: 760–772. https://doi.org/10.1038/s41576-019-0165-8
- Burns KH, 2020, Our conflict with transposable elements and its implications for human disease. Annu Rev Pathol, 15: 51–70. https://doi.org/10.1146/annurev-pathmechdis-012419-032633
- Ahmadi A, De Toma I, Vilor-Tejedor N, et al., 2020, Transposable elements in brain health and disease. Ageing Res Rev, 64: 101153. https://doi.org/10.1016/j.arr.2020.101153
- Pfaff AL, Bubb VJ, Quinn JP, et al., 2021, Reference SVA insertion polymorphisms are associated with Parkinson’s disease progression and differential gene expression. NPJ Parkinsons Dis, 7: 44. https://doi.org/10.1038/s41531-021-00189-4
- Koks S, Pfaff AL, Singleton LM, et al., 2022, Non-reference genome transposable elements (TEs) have a significant impact on the progression of the Parkinson’s disease. Exp Biol Med, 247: 1680–1690. https://doi.org/10.1177/15353702221117147
- Parkinson Progression Marker Initiative, 2011, The Parkinson progression marker initiative (PPMI). Prog Neurobiol, 95: 629–635. https://doi.org/10.1016/j.pneurobio.2011.09.005
- Rosenthal LS, Drake D, Alcalay RN, et al., 2016, The NINDS Parkinson’s disease biomarkers program. Mov Disord, 31: 915–923. https://doi.org/10.1002/mds.26438
- Kang UJ, Goldman JG, Alcalay RN, et al., 2016, The BioFIND study: Characteristics of a clinically typical Parkinson’s disease biomarker cohort. Mov Disord, 31: 924–932. https://doi.org/10.1002/mds.26613
- Li H, Durbin R, 2009, Fast and accurate short read alignment with Burrows-Wheeler transform. Bioinformatics, 25: 1754–1760. https://doi.org/10.1093/bioinformatics/btp324
- DePristo MA, Banks E, Poplin R, et al., 2011, A framework for variation discovery and genotyping using next-generation DNA sequencing data. Nat Genet, 43: 491–498. https://doi.org/10.1038/ng.806
- Regier AA, Farjoun Y, Larson DE, et al., 2018, Functional equivalence of genome sequencing analysis pipelines enables harmonized variant calling across human genetics projects. Nat Commun, 9: 4038. https://doi.org/10.1038/s41467-018-06159-4
- Gardner EJ, Lam VK, Harris DN, et al., 2017, The mobile element locator tool (MELT): Population-scale mobile element discovery and biology. Genome Res, 27: 1916–1929. https://doi.org/10.1101/gr.218032.116
- Sudmant PH, Rausch T, Gardner EJ, et al., 2015, An integrated map of structural variation in 2,504 human genomes. Nature, 526: 75–81. https://doi.org/10.1038/nature15394
- Niu Y, Teng X, Zhou H, et al., 2022, Characterizing mobile element insertions in 5675 genomes. Nucleic Acids Res, 50: 2493–2508. https://doi.org/10.1093/nar/gkac128
- Danecek P, Auton A, Abecasis G, et al., 2011, The variant call format and VCFtools. Bioinformatics, 27: 2156–2158. https://doi.org/10.1093/bioinformatics/btr330
- Purcell S, Neale B, Todd-Brown K, et al., 2007, PLINK: A tool set for whole-genome association and population-based linkage analyses. Am J Hum Genet, 81: 559–575. https://doi.org/10.1086/519795
- McLaren W, Gil L, Hunt SE, et al., 2016, The ensembl variant effect predictor. Genome Biol, 17: 122. https://doi.org/10.1186/s13059-016-0974-4
- Hubisz MJ, Pollard KS, Siepel A, 2011, PHAST and RPHAST: Phylogenetic analysis with space/time models. Brief Bioinform, 12: 41–51. https://doi.org/10.1093/bib/bbq072
- Pollard KS, Hubisz MJ, Rosenbloom KR, et al., 2010, Detection of nonneutral substitution rates on mammalian phylogenies. Genome Res, 20: 110–121. https://doi.org/10.1101/gr.097857.109
- Navarro Gonzalez J, Zweig AS, Speir ML, et al., 2021, The UCSC genome browser database: 2021 update. Nucleic Acids Res, 49: D1046–D1057. https://doi.org/10.1093/nar/gkaa1070
- Qiao Y, Ren C, Huang S, et al., 2020, High-resolution annotation of the mouse preimplantation embryo transcriptome using long-read sequencing. Nat Commun, 11: 2653. https://doi.org/10.1038/s41467-020-16444-w
- Karczewski KJ, Francioli LC, Tiao G, et al., 2020, The mutational constraint spectrum quantified from variation in 141,456 humans. Nature, 581: 434–443. https://doi.org/10.1038/s41586-020-2308-7
- Quinlan AR, Hall IM, 2010, BEDTools: A flexible suite of utilities for comparing genomic features. Bioinformatics, 26: 841–842. https://doi.org/10.1093/bioinformatics/btq033
- Price AL, Patterson NJ, Plenge RM, et al., 2006, Principal components analysis corrects for stratification in genome-wide association studies. Nat Genet, 38: 904–909. https://doi.org/10.1038/ng1847
- Bates D, Mächler M, Bolker B, et al., 2015, Fitting linear mixed-effects models using lme4. J Stat Softw, 67: 1–48. https://doi.org/10.18637/jss.v067.i01
- Kuznetsova A, Brockhoff PB, Christensen RHB, 2017, ImerTest Package: Tests in linear mixed effects models. J Stat Softw, 82: 1–26. https://doi.org/10.18637/jss.v082.i13
- Wang L, Rishishwar L, Marino-Ramirez L, et al., 2017, Human population-specific gene expression and transcriptional network modification with polymorphic transposable elements. Nucleic Acids Res, 45: 2318–2328. https://doi.org/10.1093/nar/gkw1286
- Shabalin AA, 2012, Matrix eQTL: Ultra fast eQTL analysis via large matrix operations. Bioinformatics, 28: 1353–1358. https://doi.org/10.1093/bioinformatics/bts163
- Lander ES, Linton LM, Birren B, et al., 2001, Initial sequencing and analysis of the human genome. Nature, 409: 860–921. https://doi.org/10.1038/35057062
- Vialle RA, de Paiva Lopes K, Bennett DA, et al., 2022, Integrating whole-genome sequencing with multi-omic data reveals the impact of structural variants on gene regulation in the human brain. Nat Neurosci, 25: 504–514. https://doi.org/10.1038/s41593-022-01031-7
- Bernard BJ, Nigam N, Burkitt K, et al., 2021, SMYD3: A regulator of epigenetic and signaling pathways in cancer. Clin Epigenetics, 13: 45. https://doi.org/10.1186/s13148-021-01021-9
- Yang D, Wei G, Long F, et al., 2020, Histone methyltransferase Smyd3 is a new regulator for vascular senescence. Aging Cell, 19: e13212. https://doi.org/10.1111/acel.13212
- Song W, Li Q, Wang T, et al., 2022, Putative complement control protein CSMD3 dysfunction impairs synaptogenesis and induces neurodevelopmental disorders. Brain Behav Immun, 102: 237–250. https://doi.org/10.1016/j.bbi.2022.02.027
- Lawson HA, Liang Y, Wang T, 2023, Transposable elements in mammalian chromatin organization. Nat Rev Genet, 24: 712–723. https://doi.org/10.1038/s41576-023-00609-6
- Tan EK, Chao YX, West A, et al., 2020, Parkinson disease and the immune system - associations, mechanisms and therapeutics. Nat Rev Neurol, 16: 303–318. https://doi.org/10.1038/s41582-020-0344-4
- Tansey MG, Wallings RL, Houser MC, et al., 2022, Inflammation and immune dysfunction in Parkinson disease. Nat Rev Immunol, 22: 657–673. https://doi.org/10.1038/s41577-022-00684-6
- Ryan E, Seehra G, Sharma P, et al., 2019, GBA1-associated parkinsonism: New insights and therapeutic opportunities. Curr Opin Neurol, 32: 589–596. https://doi.org/10.1097/WCO.0000000000000715
- Liu G, Boot B, Locascio JJ, et al., 2016, Specifically neuropathic Gaucher’s mutations accelerate cognitive decline in Parkinson’s. Ann Neurol, 80: 674–685. https://doi.org/10.1002/ana.24781
- Lange LM, Avenali M, Ellis M, et al., 2023, Elucidating causative gene variants in hereditary Parkinson’s disease in the Global Parkinson’s Genetics Program (GP2). NPJ Parkinsons Dis, 9: 100. https://doi.org/10.1038/s41531-023-00526-9