The significance of image fusion in nuclear medicine and molecular imaging
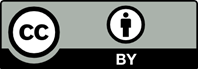
Nuclear medicine molecular imaging (NMMI) typically employs radioactive isotopes to label cells or molecules and then utilizes imaging devices such as positron emission tomography and single photon emission computed tomography to generate images. However, the images produced by these devices often suffer from problems such as signal noise, low resolution, and poor soft-organ contrast. To address these limitations, image fusion technology merges images from different imaging modalities, combining multiple types of medical image information obtained through various imaging techniques. This process generates a more comprehensive and accurate image, significantly improving image quality, reducing noise, and ultimately enhancing diagnostic accuracy and treatment effectiveness. Image fusion technology has found widespread applications in NMMI, achieving significant results in various fields. This review provides an overview of the development of image fusion technology, introduces traditional image fusion techniques, explores deep learning-based image fusion methods, and finally discusses the challenges and future directions of image fusion technology in NMMI.
- Azam MA, Khan KB, Ahmad M, et al., 2021, Multimodal medical image registration and fusion for quality enhancement. Comput Mater Continua, 68: 821–840. https://doi.org/10.32604/cmc.2021.016131
- Bodar YJL, Jansen BHE, van der Voorn JP, et al., 2021, Detection of prostate cancer with 18F-DCFPyL PET/CT compared to final histopathology of radical prostatectomy specimens: is PSMA-targeted biopsy feasible? The DeTeCT trial. World J Urol, 39: 2439–2446. https://doi.org/10.1007/s00345-020-03490-8
- Azam MA, Khan KB, Salahuddin S, et al., 2022, A review on multimodal medical image fusion: Compendious analysis of medical modalities, multimodal databases, fusion techniques and quality metrics. Comput Biol Med, 144: 105253. https://doi.org/10.1016/j.compbiomed.2022.105253
- Huang B, Yang F, Yin M, et al., 2020, A review of multimodal medical image fusion techniques. Comput Math Methods Med, 2020: 8279342. https://doi.org/10.1155/2020/8279342
- James AP, Dasarathy BV, 2014, Medical image fusion: A survey of the state of the art. Inform Fusion, 19: 4–19. https://doi.org/10.1016/j.inffus.2013.12.002
- LeCun Y, Bengio Y, Hinton G, 2015, Deep learning. Nature, 521: 436–444. https://doi.org/10.1038/nature14539
- Zhou T, Ruan S, Canu S, 2019, A review: Deep learning for medical image segmentation using multi-modality fusion. Array, 3–4: 100004. https://doi.org/10.1016/j.array.2019.100004
- Li S, Kang X, Fang L, et al., 2017, Pixel-level image fusion: A survey of the state of the art. Inform Fusion, 33: 100–112. https://doi.org/10.1016/j.inffus.2016.05.004
- Liu Z, Song Y, Sheng VS, et al., 2019, MRI and PET image fusion using the nonparametric density model and the theory of variable-weight. Comput Methods Programs Biomed, 175: 73–82. https://doi.org/10.1016/j.cmpb.2019.04.010
- Haddadpour M, Daneshvar S, Seyedarabi H, 2017, PET and MRI image fusion based on combination of 2-D Hilbert transform and IHS method. Biomed J, 40: 219–225. https://doi.org/10.1016/j.bj.2017.05.002
- Stokking R, Zuiderveld KJ, Viergever MA, 2001, Integrated volume visualization of functional image data and anatomical surfaces using normal fusion. Hum Brain Mapp, 12: 203–218. https://doi.org/10.1002/1097-0193(200104)12:4<203:AID-HBM1016>3.0.CO;2-X
- Chen CI, 2017, Fusion of PET and MR brain images based on IHS and log-gabor transforms. IEEE Sens J, 17: 6995–7010. https://doi.org/10.1109/JSEN.2017.2747220
- Parmar K, Kher R, 2012, A Comparative Analysis of Multimodality Medical Image Fusion Methods. In: Conference: 2012 Sixth Asia Modelling Symposium.
- Liu Y, Yang J, Sun J, 2010, PET/CT Medical Image Fusion Algorithm Based on Multiwavelet Transform. Vol. 2. In: Conference: Advanced Computer Control (ICACC), 2010 2nd International Conference. p264–268.
- Haribabu M, Bindu CH, Prasad KS, 2012, Multimodal Medical Image Fusion of MRI-PET Using Wavelet Transform. In: 2012 International Conference on Advances in Mobile Network, Communication and its Applications.
- Sahu A, Bhateja V, Krishn A, et al., 2014, Medical image fusion with Laplacian Pyramids. In: 2014 International Conference on Medical Imaging, m-Health and Emerging Communication Systems (MedCom).
- Mahmoudi FT, Samadzadegan F, Reinartz P, 2015, Object recognition based on the context aware decision-level fusion in multiviews imagery. IEEE J Sel Top Appl Earth Obs Remote Sens, 8: 12–22. https://doi.org/10.1109/JSTARS.2014.2362103
- Shabanzade F, Khateri M, Liu Z, 2019, MR and PET image fusion using nonparametric bayesian joint dictionary learning. IEEE Sens Lett, 3: 1–4. https://doi.org/10.1109/LSENS.2019.2925072
- Zhang Q, Liu Y, Blum RS, et al., 2018, Sparse representation based multi-sensor image fusion for multi-focus and multi-modality images: A review. Inform Fusion, 40: 57–75. https://doi.org/10.1016/j.inffus.2017.05.006
- Zong JJ, Qiu TS, 2017, Medical image fusion based on sparse representation of classified image patches. Biomed Sig Process Control, 34: 195–205. https://doi.org/10.1016/j.bspc.2017.02.005
- Zhu Z, Yin H, Chai Y, et al., 2018, A novel multi-modality image fusion method based on image decomposition and sparse representation. Inform Sci, 432: 516–529. https://doi.org/10.1016/j.ins.2017.09.010
- Daneshvar S, Ghassemian H, 2010, MRI and PET image fusion by combining IHS and retina-inspired models. Inform Fusion, 11: 114–123. https://doi.org/10.1016/j.inffus.2009.05.003
- Liu Z, Cao Y, Li Y, et al., 2020, Automatic diagnosis of fungal keratitis using data augmentation and image fusion with deep convolutional neural network. Comput Methods Programs Biomed, 187: 105019. https://doi.org/10.1016/j.cmpb.2019.105019
- Li Y, Zhao J, Lv Z, et al., 2021, Multimodal medical supervised image fusion method by CNN. Front Neurosci, 15: 638976. https://doi.org/10.3389/fnins.2021.638976
- Dian R, Li S, Kang X, 2021, Regularizing hyperspectral and multispectral image fusion by CNN denoiser. IEEE Trans Neural Netw Learn Syst, 32: 1124–1135. https://doi.org/10.1109/TNNLS.2020.2980398
- Li J, Yuan G, Fan H, 2019, Multifocus image fusion using wavelet-domain-based deep CNN. Comput Intell Neurosci, 2019: 4179397. https://doi.org/10.1155/2019/4179397
- Liu M, Wang X, Zhang H, 2018, Taxonomy of multi-focal nematode image stacks by a CNN based image fusion approach. Comput Methods Programs Biomed, 156: 209–215. https://doi.org/10.1016/j.cmpb.2018.01.016
- Lahoud F, Süsstrunk S, 2019, Zero-Learning Fast Medical Image Fusion. In: 2019 22th International Conference on Information Fusion (FUSION).
- Teng J, Wang S, Zhang J, et al., 2010, Neuro-Fuzzy Logic Based Fusion Algorithm of Medical Images. In: 2010 3rd International Congress on Image and Signal Processing.
- Wang K, Zheng M, Wei H, et al., 2020, Multi-modality medical image fusion using convolutional neural network and contrast pyramid. Sensors, 20, 2169. https://doi.org/10.3390/s20082169
- Liu Y, Chen X, Ward RK, et al., 2016, Image fusion with convolutional sparse representation. IEEE Signal Process Lett, 23: 1882–1886. https://doi.org/10.1109/LSP.2016.2618776
- Liu S, Liu S, Cai W, et al., 2015, Multimodal neuroimaging feature learning for multiclass diagnosis of Alzheimer’s disease. IEEE Trans Biomed Eng, 62: 1132–1140. https://doi.org/10.1109/TBME.2014.2372011
- Zhang H, Yuan J, Tian X, et al., 2021, GAN-FM: Infrared and visible image fusion using GAN with full-scale skip connection and dual markovian discriminators. IEEE Trans Computat Imaging, 7: 1134–1147. https://doi.org/10.1109/TCI.2021.3119954
- Ma J, Yu W, Liang P, et al., 2019, FusionGAN: A generative adversarial network for infrared and visible image fusion. Inform Fusion, 48: 11–26. https://doi.org/10.1016/j.inffus.2018.09.004
- Huang J, Le Z, Ma Y, et al., 2020, MGMDcGAN: Medical image fusion using multi-generator multi-discriminator conditional generative adversarial network. IEEE Access, 8: 55145–55157. https://doi.org/10.1109/ACCESS.2020.2982016
- Lü X, Long L, Deng R, et al., 2022, Image feature extraction based on fuzzy restricted Boltzmann machine. Measurement, 204: 112063. https://doi.org/10.1016/j.measurement.2022.112063
- Wu W, Qiu Z, Zhao M, et al., 2018, Visible and infrared image fusion using NSST and deep Boltzmann machine. Optik, 157: 334–342. https://doi.org/10.1016/j.ijleo.2017.11.087
- Sakai Y, Yamanishi K, 2014, Data Fusion Using Restricted Boltzmann Machines. In: 2014 IEEE International Conference on Data Mining.
- Fakhari A, Kiani K, 2021, A new restricted boltzmann machine training algorithm for image restoration. Multimedia Tools Appl, 80: 2047–2062. https://doi.org/10.1007/s11042-020-09685-w
- Suk HI, Lee SW, Shen D, 2014, Hierarchical feature representation and multimodal fusion with deep learning for AD/MCI diagnosis. Neuroimage, 101: 569–582. https://doi.org/10.1016/j.neuroimage.2014.06.077
- He C, Liu Q, Li H, et al., 2010, Multimodal medical image fusion based on IHS and PCA. Procedia Eng, 7: 280–285. https://doi.org/10.1016/j.proeng.2010.11.045
- Xiong Y, Wu Y, Wang Y, et al., 2017, A Medical Image Fusion Method Based on SIST and Adaptive PCNN. In: 2017 29th Chinese Control and Decision Conference (CCDC).
- Bhavana V, Krishnappa HK, 2015, Multi-modality medical image fusion using discrete wavelet transform. Procedia Comput Sci, 70: 625–631. https://doi.org/10.1016/j.procs.2015.10.057
- Wang A, Haijing S, Yueyang G, 2006, The Application of Wavelet Transform to Multi-Modality Medical Image Fusion. In: 2006 IEEE International Conference on Networking, Sensing and Control.
- Du J, Li W, Xiao B, 2018, Fusion of anatomical and functional images using parallel saliency features. Inform Sci, 430–431: 567–576. https://doi.org/10.1016/j.ins.2017.12.008
- Shahdoosti HR, Mehrabi A, 2018, Multimodal image fusion using sparse representation classification in tetrolet domain. Digit Signal Process, 79: 9–22. https://doi.org/10.1016/j.dsp.2018.04.002
- Chaitanya CK, Reddy GS, Bhavana V, et al., 2017, PET and MRI medical image fusion using STDCT and STSVD. In: 2017 International Conference on Computer Communication and Informatics (ICCCI).
- Liu Y, Chen X, Cheng J, et al., 2017, A Medical Image Fusion Method Based on Convolutional Neural Networks. In: 2017 20th International Conference on Information Fusion (Fusion).
- Xu H, Ma J, 2021, EMFusion: An unsupervised enhanced medical image fusion network. Inform Fusion, 76: 177–186. https://doi.org/10.1016/j.inffus.2021.06.001
- Xia J, Lu Y, Tan L, 2020, Research of multimodal medical image fusion based on parameter-adaptive pulse-coupled neural network and convolutional sparse representation. Comput Math Methods Med, 2020: 3290136. https://doi.org/10.1155/2020/3290136
- Ma J, Xu H, Jiang J, et al., 2020, DDcGAN: A dual-discriminator conditional generative adversarial network for multi-resolution image fusion. IEEE Trans Image Process, 29: 4980–4995. https://doi.org/10.1109/TIP.2020.2977573
- Kang J, Lu W, Zhang W, 2020, Fusion of brain PET and MRI images using tissue-aware conditional generative adversarial network with joint loss. IEEE Access, 8: 6368–6378. https://doi.org/10.1109/ACCESS.2019.2963741