Ethnogenetic-specific mutations in Alzheimer’s disease: A marker of clinical outcomes
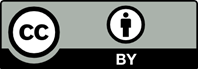
Alzheimer’s disease (AD) presents a substantial global health challenge, with its pathogenesis influenced by a complex interplay of genetic and molecular factors. Approximately 1% of AD cases are attributed to early onset autosomal dominant familial AD (fAD), with genetics contributing to about 70% of overall AD risk. Understanding the genetic basis and molecular mechanisms is paramount for early diagnosis and prognosis improvement. This study explores a previously underexplored area of fAD, examining how different mutations within the same gene, encoding a single protein, may influence the clinical features and progression of fAD subtypes. Our investigation focuses on distinct fAD subtypes — Iranian (T714A), Swedish (KM670/671NL), and Australian (L723P) — and their associated mutations within the C-terminus domain of amyloid precursor protein (APP). Through an extensive analysis of existing literature encompassing clinical severity, pathogenicity, neuropathology, and biological processes, we reveal critical insights into these fAD subtypes. Leveraging bioinformatics tools, we correlate the physicochemical properties of translated mutant proteins with clinical and neuropathological features. Notably, our findings demonstrate that mutations occurring between codons 714 and 717 of the APP gene share a higher similarity, resulting in lower root mean squared deviation scores. These mutations are associated with a broader spectrum of clinical symptoms, including myoclonus and seizures, and an earlier age of onset. Moreover, we observe a direct correlation between the location of genetic mutations on the protein sequence and specific physicochemical properties, clinical presentations, and neuropathological features among fAD subtypes. Mutations with higher structural similarity tend to manifest similar clinical and physical characteristics. While certain neuropathological findings correlate with an increasing epitope toxicity burden, our analysis indicates that epitope toxicity does not significantly impact clinical outcomes. In summary, our study provides novel insights into the heterogeneous nature of fAD subtypes, illuminating the intricate relationship between genetic mutations, physicochemical properties, and clinical manifestations. These findings offer a foundation for further research into tailored therapeutic approaches and personalized medicine for fAD.
- Alonso Vilatela ME, López-López M, Yescas-Gómez P, 2012, Genetics of Alzheimer’s disease. Arch Med Res, 8: 622–631. https://doi.org/10.1016/j.arcmed.2012.10.017
- Giri M, Zhang M, Lü Y, 2016, Genes associated with alzheimer’s disease: An overview and current status. Clin Interv Aging, 11: 665–681. https://doi.org/10.2147/CIA.S105769
- Dementia: Key Facts, 2022. Available from: https://www. who.int/news-room/fact-sheets/detail/dementia [Last accessed on 2022 Dec 30].
- Selkoe DJ, 2011, Alzheimer’s disease. Cold Spring Harb Perspect Biol, 3: a004457. https://doi.org/10.1101/cshperspect.a004457
- Trambauer J, Fukumori A, Steiner H, 2020, Pathogenic Aβ generation in familial Alzheimer’s disease: Novel mechanistic insights and therapeutic implications. Curr Opin Neurobiol, 61: 73–81. https://doi.org/10.1016/j.conb.2020.01.011
- Marechal L, Campion D, Hannequin D, 2020, Familial forms of Alzheimer’s disease. Presse Med, 32: 756–763.
- Hoogmartens J, Cacace R, Van Broeckhoven C, 2021, Insight into the genetic etiology of Alzheimer’s disease: A comprehensive review of the role of rare variants. Alzheimers Dement (Amst), 13: e12155. https://doi.org/10.1002/dad2.12155
- Jayne T, Newman M, Verdile G, et al., 2016, Evidence for and against a pathogenic role of reduced γ- secretase activity in familial Alzheimer’s disease. J Alzheimers Dis, 52: 781–799. https://doi.org/10.3233/JAD-151186
- Patterson C, Feightner JW, Garcia A, et al., 2008, Diagnosis and treatment of dementia: 1. Risk assessment and primary prevention of Alzheimer disease. CMAJ, 178: 548–556. https://doi.org/10.1503/cmaj.070796
- Li NM, Liu KF, Qiu YJ, et al., 2019, Mutations of beta-amyloid precursor protein alter the consequence of Alzheimer’s disease pathogenesis. Neural Regen Res, 14: 658–665. https://doi.org/10.4103/1673-5374.247469
- Cai Y, An SSA, Kim S, 2015, Mutations in presenilin 2 and its implications in Alzheimer’s disease and other dementia-associated disorders. Clin Interv Aging, 10: 1163–1172. https://doi.org/10.2147/CIA.S85808
- Shea Y, Chu L, Chan A, et al., 2016, A systematic review of familial Alzheimer’s disease: Differences in presentation of clinical features among three mutated genes and potential ethnic differences. J Formos Med Assoc, 115: 67–75. htps://doi.org/10.1016/j.jfma.2015.08.004
- Kasuga K, Kikuchi M, Tokutake T, et al., 2015, Systematic review and meta-analysis of Japanese familial Alzheimer’s disease and ftdp-17. J Hum Genet, 60: 281–283. https://doi.org/10.1038/jhg.2015.15
- Ryan N, Rossor M, 2010, Correlating familial Alzheimer’s disease gene mutations with clinical phenotype. Biomark Med, 4: 99–112. https://doi.org/10.2217/bmm.09.92
- Zou Z, Liu C, Che C, 2014, Clinical genetics of Alzheimer’s disease. Biomed Res Int, 2014: 291862.
- Wu L, Rosa-Neto P, Hsiung GYR, et al., 2014, Early-onset familial Alzheimer’s disease (EOFAD). Can J Neurol Sci, 39: 436–445. https://doi.org/10.1017/s0317167100013949
- Kutoku Y, Ohsawa Y, Kuwano R, et al., 2015, A second pedigree with amyloid-less familial Alzheimer’s disease harboring an identical mutation in the amyloid precursor protein gene (E693delta). Intern Med, 54: 205–208. https://doi.org/10.2169/internalmedicine.54.3021
- Tomiyama T, Shimada H, 2020, APP Osaka mutation in familial Alzheimer’s disease-its discovery, phenotypes, and mechanism of recessive inheritance. Int J Mol Sci, 21: 1413. https://doi.org/10.3390/ijms21041413
- Kowalska A, 2004, Genetic counseling and testing for families with Alzheimer’s disease. Neurol Neurochir Pol, 6: 495–501.
- UniProt Consortium, 2021, Uniprot: The universal protein knowledgebase in 2021. Nucleic Acids Res, 49: D480–D489. https://doi.org/10.1093/nar/gkaa1100
- Mutations: APP, 1996-2022, 2022. Available from: https:// www.alzforum.org/mutations/app [Last accessed on 2023 Mar 30].
- Duvaud S, Gabella C, Lisacek F, et al., 2005, Protein identification and analysis tools on the expasy server. In: Walker JM, (ed). The Proteomics Protocols Handbook. United States: Humana Press, p571–607.
- Roy A, Kucukural A, Zhang Y, 2010, I-TASSER: A unified platform for automated protein structure and function prediction. Nat Protoc, 5: 725–738. https://doi.org/10.1038/nprot.2010.5
- Yang J, Yan R, Roy A, et al., 2015, The I-TASSER suite: Protein structure and function prediction. Nat Methods, 12: 7–8. https://doi.org/10.1038/nmeth.3213
- Zhang Y, 2008, I-TASSER server for protein 3D structure prediction. BMC Bioinformtics, 9: 40. https://doi.org/10.1186/1471-2105-9-40
- Heo L, Park H, Seok C, 2013, Galaxyrefine: Protein structure refinement driven by side-chain repacking. Nucleic Acids Res, 41: W384–W388. https://doi.org/10.1093/nar/gkt458
- Lee GR, Heo L, Seok C, 2016, Effective protein model structure refinement by loop modeling and overall relaxation. Proteins, 84: 294–301. https://doi.org/10.1002/prot.24858
- Laskowski R, Chistyakov V, 2013, PDBsum. European Bioinformatics Institute. Available from: https://www. ebi.ac.uk/thornton-srv/databases/cgi-bin/pdbsum [Last accessed on 2022 Dec 30].
- Bowie JU, Lüthy R, Eisenberg D, 1991, A method to identify protein sequences that fold into a known three-dimensional structure. Science, 253: 164–170. https://doi.org/10.1126/science.1853201
- Lüthy R, Bowie JU, Eisenberg D, 1992, Assessment of protein models with three-dimensional profiles. Nature, 356: 83–85. https://doi.org/10.1038/356083a0
- Colovos C, Yeates TO, 1993, Verification of protein structures: Patterns of nonbonded atomic interactions. Protein Sci, 2: 1511–1519. https://doi.org/10.1002/pro.5560020916
- Sippl MJ, 1993, Recognition of errors in three-dimensional structures of proteins. (ProSA). Proteins, 17: 355–362. https://doi.org/10.1002/prot.340170404
- Wiederstein M, Sippl MJ, 2007, ProSA-web: Interactive web service for the recognition of errors in three-dimensional structures of proteins. Nucleic Acids Res, 35: 407–410.
- DeLano WL, 2002, Pymol: An open-source molecular graphics tool. CCP4 Newsl Protein Crystallogr, 40: 82–92.
- Nielsen M, Lundegaard C, Worning P, et al., 2003, Reliable prediction of T-cell epitopes using neural networks with novel sequence representations. Protein Sci, 12: 1007–1017. https://doi.org/10.1110/ps.0239403
- Ponomarenko J, Bui H, Li W, et al., 2008. Ellipro: A new structure-based tool for the prediction of antibody epitopes. BMC Bioinformatics, 9: 514. https://doi.org/10.1186/1471-2105-9-514
- Vita R, Mahajan S, Overton J, et al., 2018, The immune epitope database (IEDB): 2018 update. Nucleic Acids Res, 47: D339–D343. https://doi.org/10.1093/nar/gky1006
- Jespersen MC, Peters B, Nielsen M, et al., 2017, Bepipred-2.0: Improving sequence-based B-cell epitope prediction using conformational epitopes. Nucleic Acids Res, 45: W24–W29. https://doi.org/10.1093/nar/gkx346
- Gupta S, Kapoor P, Chaudhary K, et al., 2013, In silico approach for predicting toxicity of peptides and proteins. PLoS One, 8: e73957. https://doi.org/10.1371/journal.pone.0073957
- Gupta S, Kapoor P, Chaudhary K, et al., 2015, Peptide toxicity prediction. Methods Mol Biol, 1268: 143–157. https://doi.org/10.1007/978-1-4939-2285-7_7
- Lanoiselée HM, Nicolas G, Wallon D, et al., 2017, APP, PSEN1, and PSEN2 mutations in early-onset Alzheimer disease: A genetic screening study of familial and sporadic cases. PLoS Med, 14: e1002270. https://doi.org/10.1371/journal.pmed.1002270
- Yoshikai S, Sasaki H, Doh-Ura K, et al., 1990, Genomic organization of the human amyloid beta-protein precursor gene. Gene, 87: 257–263. https://doi.org/10.1016/0378-1119(90)90310-n
- Levy E, Carman MD, Fernandez-Madrid IJ, et al., 1990, Mutation of the Alzheimer’s disease amyloid gene in hereditary cerebral hemorrhage, Dutch type. Science, 248: 1124–1126. https://doi.org/10.1126/science.2111584
- Wisniewski T, Ghiso J, Frangione B, 1991, Peptides homologous to the amyloid protein of Alzheimer’s disease containing a glutamine for glutamic acid substitution have accelerated amyloid fibril formation. Biochem Biophys Res Commun, 179: 1247–1254. https://doi.org/10.1016/0006-291x(91)91706-i
- Haass C, Hung AY, Selkoe DJ, et al., 1994, Mutations associated with a locus for familial Alzheimer’s disease result in alternative processing of amyloid beta-protein precursor. J Biol Chem, 269: 17741–17748.
- Rocchi A, Pellegrini S, Siciliano G, et al., 2003, Causative and susceptibility genes for Alzheimer’s disease: A review. Brain Res Bull, 61: 1–24. https://doi.org/10.1016/s0361-9230(03)00067-4
- Kumar-Singh S, Cras P, Wang R, et al., 2002, Dense-core senile plaques in the Flemish variant of Alzheimer’s disease are vasocentric. Am J Pathol, 161: 507–520. https://doi.org/10.1016/S0002-9440(10)64207-1
- Castellani RJ, Honda K, Zhu X, et al., 2004, Contribution of redox-active iron and copper to oxidative damage in Alzheimer disease. Ageing Res Rev, 3: 319–326. https://doi.org/10.1016/j.arr.2004.01.002
- Ryan NS, Biessels GJ, Kim L, et al., 2015, Genetic determinants of white matter hyperintensities and amyloid angiopathy in familial Alzheimer’s disease. Neurobiol Aging, 36: 3140–3151. https://doi.org/10.1016/j.neurobiolaging.2015.08.026
- Ryan NS, Nicholas JM, Weston PS, et al., 2016, Clinical phenotype and genetic associations in autosomal dominant familial Alzheimer’s disease: A case series. Lancet Neurol, 15: 1326–1335. https://doi.org/10.1016/S1474-4422(16)30193-4
- Żekanowski C, Styczyńska M, Pepłońska B, et al., 2003, Mutations in presenilin 1, presenilin 2 and amyloid precursor protein genes in patients with early-onset Alzheimer’s disease in Poland. Exp Neurol, 184: 991–996. https://doi.org/10.1016/S0014-4886(03)00384-4
- Lindquist SG, Nielsen JE, Stokholm J, et al., 2008, Atypical early-onset Alzheimer’s disease caused by the Iranian APP mutation. J Neurol Sci, 268: 124–130. https://doi.org/10.1016/j.jns.2007.11.021
- Edwards-Lee T, Ringman JM, Chung J, et al., 2005, An African American family with early-onset Alzheimer disease and an APP (T714I) mutation. Neurology, 64: 377–379. https://doi.org/10.1212/01.WNL.0000149761.70566.3E
- Valerija D, Stefanova E, Jankovic M, et al., 2012, Genetic testing in familial and young-onset Alzheimer’s disease: Mutation spectrum in a serbian cohort. Neurobiol Aging, 33: 1481.e7–e12. https://doi.org/10.1016/j.neurobiolaging.2011.12.007
- Guerreiro RJ, Baquero M, Blesa R, et al., 2010, Genetic screening of Alzheimer’s disease genes in Iberian and African samples yields novel mutations in presenilins and APP. Neurobiol Aging, 31: 725–731. https://doi.org/10.1016/j.neurobiolaging.2008.06.012
- Wang Q, Jia J, Qin W, et al., 2015, A novel AβPP M722K mutation affects amyloid-β secretion and Tau phosphorylation and may cause early-onset familial Alzheimer’s disease in Chinese individuals. J Alzheimers Dis, 47: 157–165. https://doi.org/10.3233/JAD-143231
- Larner AJ, 2013, Presenilin-1 mutations in Alzheimer’s disease: An update on genotype-phenotype relationships. J Alzheimers Dis, 47: 653–659. https://doi.org/10.3233/JAD-130746
- Canevelli M, Piscopo P, Talarico G, et al., 2014, Familial Alzheimer’s disease sustained by presenilin 2 mutations: Systematic review of literature and genotype-phenotype correlation. Neurosci Behav Rev, 42: 170–179. https://doi.org/10.1016/j.neubiorev.2014.02.010
- Mehrgou A, Akouchekian M, 2016, The importance of BRCA1 and BRCA2 genes mutations in breast cancer development. Med J Islam Repub Iran, 30: 369.
- Gayther S, Warren W, Mazoyer S, et al., 1995, Germline mutations of the BRCA1 gene in breast and ovarian cancer families provide evidence for a genotype-phenotype correlation. Nat Genet, 11: 428–433. https://doi.org/10.1038/ng1295-428
- Linger RJ, Kruk PA, 2010, BRCA1 16 years later: Risk-associated BRCA1 mutations and their functional implications. FEBS J, 277: 3086–3096. https://doi.org/10.1111/j.1742-4658.2010.07735.x
- Hunter JC, Manandhar A, Carrasco MA, et al., 2015, Biochemical and structural analysis of common cancer-associated KRAS mutations. Mol Cancer Res, 13: 1325–1335. https://doi.org/10.1158/1541-7786.MCR-15-0203