Brief risk rating scale: A preliminary screening and monitoring tool emphasizing individual differences for better prognosis in Alzheimer’s disease
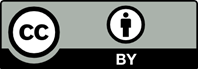
Over the last several decades, significant progress has been made in the diagnostic criteria of Alzheimer’s disease (AD) to identify its early stages, including subjective cognitive decline and mild cognitive impairment. However, the previous research rarely took account of individual differences when evaluating AD-spectrum patients at different stages, thereby resulting in similar treatment, which was not only ineffective but also resulted in the missed window of opportunity for intervention. In this review, we propose the Brief Risk Rating Scale (BRRS), which is predominantly based on extant literature concerning AD risk factors and brain alterations, with the aim of providing a preliminary screening and monitoring tool that can facilitate the assessment of individual’s risk level, the prediction and tracking of disease progression, as well as precise treatment in a timely manner. Meanwhile, due to its simplicity and ease of use, it can be widely promoted and likewise accessible to clinicians in grassroots clinics. In general, the scale comprises two parts: The original score (O) related to patients’ risk factors and the variation score (V) related to brain abnormalities tested by different sequences of magnetic resonance imaging. In addition, the advantages along with its clinical application, such as introducing BRRS into cognitive training and brain stimulation, are also discussed. We conclude that BRRS positively contributes to enhancing the accuracy of clinical diagnosis and the efficiency of personalized treatment in AD-spectrum patients, with individual differences fully considered and little additional burden added. However, the weight coefficient of each item in BRRS should be thoroughly studied in future research.
Aisen PS, Jimenez-Maggiora GA, Rafii MS, et al., 2022, Early-stage alzheimer disease: getting trial-ready. Nat Rev Neurol, 18(7): 389–399. https://doi.org/10.1038/s41582-022-00645-6
Vermunt L, Sikkes SAM, van den Hout A, et al., 2019, Duration of preclinical, prodromal, and dementia stages of alzheimer’s disease in relation to age, sex, and APOE genotype. Alzheimers Dement, 15(7): 888–898. https://doi.org/10.1016/j.jalz.2019.04.001
Studart AN, Nitrini R, 2016, Subjective cognitive decline: The first clinical manifestation of alzheimer’s disease? Dement Neuropsychol, 10(3): 170–177. https://doi.org/10.1590/s1980-5764-2016dn1003002
Rabin LA, Smart CM, Amariglio RE, 2017, Subjective cognitive decline in preclinical alzheimer’s disease. Annu Rev Clin Psychol, 13: 369–396. https://doi.org/10.1146/annurev-clinpsy-032816-045136
Ferreira D, Pereira JB, Volpe G, et al., 2019, Subtypes of alzheimer’s disease display distinct network abnormalities extending beyond their pattern of brain atrophy. Front Neurol, 10: 524. https://doi.org/10.3389/fneur.2019.00524
Lehmann M, Madison C, Ghosh PM, et al., 2015, Loss of functional connectivity is greater outside the default mode network in nonfamilial early-onset alzheimer’s disease variants. Neurobiol Aging, 36(10): 2678–2686. https://doi.org/10.1016/j.neurobiolaging.2015.06.029
Vogel JW, Hansson O, 2022, Subtypes of alzheimer’s disease: questions, controversy, and meaning. Trends Neurosci, 45(5): 342–345. https://doi.org/10.1016/j.tins.2022.02.001
Duara R, Barker W, 2022, Heterogeneity in alzheimer’s disease diagnosis and progression rates: Implications for therapeutic trials. Neurotherapeutics, 19(1): 8–25. https://doi.org/10.1007/s13311-022-01185-z
Srivastava S, Ahmad R, Khare SK, 2021, Alzheimer’s disease and its treatment by different approaches: A review. Eur J Med Chem, 216: 113320. https://doi.org/10.1016/j.ejmech.2021.113320
Scheltens P, Blennow K, Breteler MM, et al., 2016, Alzheimer’s disease. Lancet, 388(10043): 505–517. https://doi.org/10.1016/s0140-6736(15)01124-1
2022, 2022 alzheimer’s disease facts and figures. Alzheimers Dement, 18(4): 700–789. https://doi.org/10.1002/alz.12638
Keine D, Walker JQ, Kennedy BK, et al., 2018, Development, application, and results from a precision-medicine platform that personalizes multi-modal treatment plans for mild alzheimer’s disease and at-risk individuals. Curr Aging Sci, 11(3): 173–181. https://doi.org/10.2174/1874609811666181019101430
Sabbagh M, Sadowsky C, Tousi B, et al., 2020, Effects of a combined transcranial magnetic stimulation (TMS) and cognitive training intervention in patients with alzheimer’s disease. Alzheimers Dement, 16(4): 641–650. https://doi.org/10.1016/j.jalz.2019.08.197
Yu JT, Xu W, Tan CC, et al., 2020, Evidence-based prevention of alzheimer’s disease: Systematic review and meta-analysis of 243 observational prospective studies and 153 randomised controlled trials. J Neurol Neurosurg Psychiatry, 91(11): 1201–1209. https://doi.org/10.1136/jnnp-2019-321913
Livingston G, Huntley J, Sommerlad A, et al., 2020, Dementia prevention, intervention, and care: 2020 report of the Lancet Commission. Lancet, 396(10248): 413–446. https://doi.org/10.1016/s0140-6736(20)30367-6
Malpetti M, Ballarini T, Presotto L, et al., 2017, Gender differences in healthy aging and alzheimer’s dementia: A (18) F-FDG-PET study of brain and cognitive reserve. Hum Brain Mapp, 38(8): 4212–4227. https://doi.org/10.1002/hbm.23659
Rahman A, Schelbaum E, Hoffman K, et al., 2020, Sex-driven modifiers of alzheimer risk: A multimodality brain imaging study. Neurology, 95(2): e166–e178. https://doi.org/10.1212/wnl.0000000000009781
Bloomberg M, Dugravot A, Dumurgier J, et al., 2021, Sex differences and the role of education in cognitive ageing: Analysis of two UK-based prospective cohort studies. Lancet Public Health, 6(2): e106–e115. https://doi.org/10.1016/s2468-2667(20)30258-9
Perani D, Farsad M, Ballarini T, et al., 2017, The impact of bilingualism on brain reserve and metabolic connectivity in alzheimer’s dementia. Proc Natl Acad Sci U S A, 114(7): 1690–1695. https://doi.org/10.1073/pnas.1610909114
Tucholka A, Grau-Rivera O, Falcon C, et al., 2018, Structural connectivity alterations along the alzheimer’s disease continuum: Reproducibility across two independent samples and correlation with cerebrospinal fluid amyloid-β and tau. J Alzheimers Dis, 61(4): 1575–1587. https://doi.org/10.3233/jad-170553
Olsson B, Lautner R, Andreasson U, et al., 2016, CSF and blood biomarkers for the diagnosis of alzheimer’s disease: A systematic review and meta-analysis. Lancet Neurol, 15(7): 673–684. https://doi.org/10.1016/s1474-4422(16)00070-3
Jack CR, Jr., 2022, Advances in alzheimer’s disease research over the past two decades. Lancet Neurol, 21(10): 866–869. https://doi.org/10.1016/s1474-4422(22)00298-8
Bai F, Shi Y, Yuan Y, et al., 2016, Immunity factor contributes to altered brain functional networks in individuals at risk for alzheimer’s disease: Neuroimaging-genetic evidence. Brain Behav Immun, 56: 84–95. https://doi.org/10.1016/j.bbi.2016.02.015
Hu YS, Xin J, Hu Y, et al., 2017, Analyzing the genes related to alzheimer’s disease via a network and pathway-based approach. Alzheimers Res Ther, 9(1): 29. https://doi.org/10.1186/s13195-017-0252-z
Yamazaki Y, Zhao N, Caulfield TR, et al., 2019, Apolipoprotein E and alzheimer disease: Pathobiology and targeting strategies. Nat Rev Neurol, 15(9): 501–518. https://doi.org/10.1038/s41582-019-0228-7
Beisteiner R, Matt E, Fan C, et al., 2020, Transcranial pulse stimulation with ultrasound in alzheimer’s disease-a new navigated focal brain therapy. Adv Sci (Weinh), 7(3): 1902583. https://doi.org/10.1002/advs.201902583
Erk S, Spottke A, Meisen A, et al., 2011, Evidence of neuronal compensation during episodic memory in subjective memory impairment. Arch Gen Psychiatry, 68(8): 845–852. https://doi.org/10.1001/archgenpsychiatry.2011.80
Gu Y, Liu R, Qin R, et al., 2019, Characteristic changes in the default mode network in hypertensive patients with cognitive impairment. Hypertens Res, 42(4): 530–540. https://doi.org/10.1038/s41440-018-0176-4
Sheng X, Chen H, Shao P, et al., 2021, Brain structural network compensation is associated with cognitive impairment and alzheimer’s disease pathology. Front Neurosci, 15: 630278. https://doi.org/10.3389/fnins.2021.630278
Hafkemeijer A, Altmann-Schneider I, Oleksik AM, et al., 2013, Increased functional connectivity and brain atrophy in elderly with subjective memory complaints. Brain Connect, 3(4): 353–362. https://doi.org/10.1089/brain.2013.0144
Mormino EC, Smiljic A, Hayenga AO, et al., 2011, Relationships between β-amyloid and functional connectivity in different components of the default mode network in aging. Cereb Cortex, 21(10): 2399–2407. https://doi.org/10.1093/cercor/bhr025
Bachurin SO, Gavrilova SI, Samsonova A, et al., 2018, Mild cognitive impairment due to alzheimer disease: Contemporary approaches to diagnostics and pharmacological intervention. Pharmacol Res, 129: 216–226. https://doi.org/10.1016/j.phrs.2017.11.021
Graff-Radford J, Arenaza-Urquijo EM, Knopman DS, et al., 2019, White matter hyperintensities: Relationship to amyloid and tau burden. Brain, 142(8): 2483–2491. https://doi.org/10.1093/brain/awz162
Kuchtova B, Wurst Z, Mrzilkova J, et al., 2018, Compensatory shift of subcallosal area and paraterminal gyrus white matter parameters on DTI in patients with alzheimer disease. Curr Alzheimer Res, 15(6): 590–599. https://doi.org/10.2174/1567205015666171227155510
Bos D, Wolters FJ, Darweesh SKL, et al., 2018, Cerebral small vessel disease and the risk of dementia: A systematic review and meta-analysis of population-based evidence. Alzheimers Dement, 14(11): 1482–1492. https://doi.org/10.1016/j.jalz.2018.04.007
Del Val LP, Cantero JL, Baena D, et al., 2018, Damage of the temporal lobe and APOE status determine neural compensation in mild cognitive impairment. Cortex, 101: 136–153. https://doi.org/10.1016/j.cortex.2018.01.018
Farrar DC, Mian AZ, Budson AE, et al., 2018, Retained executive abilities in mild cognitive impairment are associated with increased white matter network connectivity. Eur Radiol, 28(1): 340–347. https://doi.org/10.1007/s00330-017-4951-4
Fu L, Maes JH, Kessels RP, et al., 2017, To boost or to CRUNCH? Effect of effortful encoding on episodic memory in older adults is dependent on executive functioning. PLoS One, 12(3): e0174217. https://doi.org/10.1371/journal.pone.0174217
Alcalá-Lozano R, Morelos-Santana E, Cortés-Sotres JF, et al., 2018, Similar clinical improvement and maintenance after rTMS at 5 Hz using a simple vs. complex protocol in alzheimer’s disease. Brain Stimul, 11(3): 625–627. https://doi.org/10.1016/j.brs.2017.12.011
Sandrini M, Umiltà C, Rusconi E, 2011, The use of transcranial magnetic stimulation in cognitive neuroscience: A new synthesis of methodological issues. Neurosci Biobehav Rev, 35(3): 516–536. https://doi.org/10.1016/j.neubiorev.2010.06.005
Sullivan MD, Anderson JAE, Turner GR, et al., 2019, Intrinsic neurocognitive network connectivity differences between normal aging and mild cognitive impairment are associated with cognitive status and age. Neurobiol Aging, 73: 219–228. https://doi.org/10.1016/j.neurobiolaging.2018.10.001
Pennisi G, Ferri R, Lanza G, et al., 2011, Transcranial magnetic stimulation in alzheimer’s disease: A neurophysiological marker of cortical hyperexcitability. J Neural Transm (Vienna), 118(4): 587–598. https://doi.org/10.1007/s00702-010-0554-9
Chu CS, Li CT, Brunoni AR, et al., 2021, Cognitive effects and acceptability of non-invasive brain stimulation on alzheimer’s disease and mild cognitive impairment: A component network meta-analysis. J Neurol Neurosurg Psychiatry, 92(2): 195–203. https://doi.org/10.1136/jnnp-2020-323870
Wang XQ, Huang WJ, Su L, et al., 2020, Neuroimaging advances regarding subjective cognitive decline in preclinical alzheimer’s disease. Mol Neurodegener, 15(1): 27. https://doi.org/10.1186/s13024-020-00395-3