The Assessment of Effective Factors on Anzali Wetland Pollution Using Artificial Neural Networks
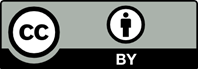
Anzali wetland, which is located in north part of Iran, is one of the most important ecosystems in the world considering economical and environmental features, and is in the list of Ramsar Convention. Receiving wastewater with minimum treatment caused increasing water pollution in the wetland. In this regard, having enough and accurate data and precise interpretation are necessary which may help water quality management. There are many methods for interpretation of data. In this work, prediction of COD parameter in Siakeshim station in south part of the wetland was studied using Artificial Neural Network (ANN) considering effects of five parameters TP, TN, (NO3-N), (NO2-N) and (NH4-N) as the most important features of nutritional materials and creation of utrification and also predictors of pollution. The ten years average monthly data of five mentioned parameters are applied as input of Multi Layer Perception (MLP) models. The results of the study showed that using MLP methods obtained precise predictions for COD parameter and also the rate of effects of each input parameters in water pollution. Hence, prediction of water quality using ANN model may be useful for water quality planning and management.
Akbarzadeh, A. (1995). Management and Treatment Strategy of Anzali Pound through modulation of nutrient materials methods, Report, Iran.
ASCE Task Committee on Application of Artificial Neural Networks in Hydrology. (2000a). Artificial Neural Networks in Hyrology: Preliminary Concepts. Part 1. J. Hydrologic Eng., ASCE, 5(2): 115-123.
ASCE Task Committee on Application of Artificial Neural Networks in Hydrology. (2000b). Artificial Neural Networks in Hydrology: Hydrologic Applications. Part II, J. Hydrologic Eng., ASCE, 5(2): 123-137.
Akratos, C.S., Papaspyros, N.E. and Vassilios A. Tsihrintzis (2008). An Artificial Neural Network Model & Design Equipment for BOD & COD Removal Prediction in Horizontal Subsurface Flow Constructed Wetland. Chemical Engineering Journal, 143 (1 & 3): 96-111.
Cüneyt, K., Selçuk, S. and Y. Co skun (1999). Neural network models as a management tool in lakes. Journal Hydrobiologia, 408-409: 139-409.
Hornik, K.M., Stinchcombe, M. and H. White (1989). Multilayer Feedforward Networks are Universal Approximators, Neural Networks, Elsevier Science Publisher, 2(5): 359-366.
Hosseinzadeh, H. (2005). The Theory and Suggestion on modification and operation of Anzali pound. The Fishery Research Centre of Gilan, Report, Iran.
Kennedy, J.B. and A.D. Neville (1964). Basic statistical methods for engineers and scientists. IEP, 2nd ed.