Analysis of Spatially and Temporally Varying Precipitation in Bangladesh
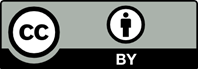
Nowadays, time series analysis of rainfall data is a major tool to explore rainfall variability pattern. In this study, rainfall data at 28 stations of Bangladesh has been evaluated using trend test, spatial analysis and Artificial Neural Network (ANN) model. From the Mann-Kendall’s (M-K) trend test, it was observed that the value of Sen’s slope for the maximum increase of rainfall was 1.813 mm per month in Cox’s Bazar and the maximum decrease of rainfall was -0.896 mm per month in Bhola. Rainfall time series were smoothed using wavelet transformation and then wavelet denoised signals were used for fitting Artificial Neural Network (ANN) model. The predictive capability of ANN model was assessed by PBIAS (Percent of bias), index of agreement (d) and R-squared approach. Finally, a forecast for five years (January 2010-December 2014) was carried out to evaluate the predictive capability. The values of normalized root mean square error (NRMSE) ranges between 0.07% and 0.17% for calibration and 0.07%-0.32% for validation which indicate the fitness of the model. The findings derived from the study are to understand the nature of possible rainfall variations in 28 stations of Bangladesh, which will help the hydrologists as well as the policy makers to make decisions.
Adamowski, J. and C. Karapataki (2010). Comparison of multivariate regression and artificial neural networks for peak urban water-demand forecasting: Evaluation of different ANN learning algorithms. Journal of Hydrologic Engineering, 15(10): 729-743.
Afzal, M., Mansell, M.G. and A.S. Gagnon (2011). Trends and variability in daily precipitation in Scotland. Procedia Environmental Sciences Journal, 6(1): 15-26.
Agrawal, A., Kumar, V., Pandey, A. and I. Khan (2012). An application of time series analysis for weather forecasting. International Journal of Engineering Research and Applications, 2(2), 974-980.
Akhtar, M.K., Corzo, G.A., Andel, S.J.V. and A. Jonoski1 (2009). River flow forecasting with artificial neural networks using satellite observed precipitation pre- processed with flow length and travel time information: Case study of the Ganges river basin. Hydrology and Earth System Sciences, 13(9): 1607-1618.
Alrumiah, R.M. and M.A. Fawzan (2002). Time series forecasting using wavelet denoising an application to Saudi stocks index. Journal of King Saud University, Engineering Sciences, 2(14): 221-234.
Cannarozzo, M., Noto, L.V. and F. Viola (2006). Spatial distribution of rainfall trends in Sicily (1921–2000). Physics and Chemistry of the Earth, Parts A/B/C, 31(18): 1201-1211.
Deo, R.C. and M. Sahin (2015). Application of the artificial neural network model for prediction of monthly standardized precipitation and evapotranspiration index using hydro meteorological parameters and climate indices in eastern Australia. Atmospheric Research, 161-162(1): 65-81.
Dong, Z., Guo, X. and J. Zheng (2001). Calculation of noise resistance by use of the discrete wavelets transform. Electrochemistry Communications, 3(10): 561-565.
Drápela, K. and I. Drápelová (2011). Application of Mann- Kendall test and the Sen’s slope extimates for trend detection in deposition data from Bílý Kříž (Beskdydy Mts., the Czech Republic) 1997-2010. Beskydy, 4(2): 133-146.
ESRI (2001). Using ArcGIS Geostatistical Analyst. The United States of America.
Hand, L.M. and J.M. Shepherd (2009). An investigation of warm-season spatial rainfall variability in Oklahoma City: Possible linkages to urbanization and prevailing wind. Journal of Applied Meteorology and Climatology,
48(2): 251-269.
Intergovernmental Panel on Climate Change (IPCC) (2007). Climate Change 2007: The Physical Science Basis. Contribution of Working Group I to the Fourth Assessment Report of the Intergovernmental Panel on Climate Change, edited by S. Solomon et al. Cambridge Univ. Press, New York.
KİSİ, Ő. (2003). Daily river flow forecasting using artificial neural networks and auto-regressive models. Turkish Journal of Engineering and Environmental Sciences,29(1): 9-20.
Khan, Y.A., Lateh, H., Baten, M.A. and A.A. Kamil (2011). Critical antecedent rainfall conditions for shallow landslides in Chittagong City of Bangladesh. Environmental Earth Sciences, 67(1): 97-106.
Longobardi, A. and P. Villani (2009). Trend analysis of annual and seasonal rainfall time series in the Mediterranean area. International Journal of Climatology, 30(10): 30-139.
Luís, M.D., Raventós, J., González-Hidalgo, J.C., Sánchez, J.R. and J. Cortina (2000). Spatial analysis of rainfall trends in the region of Valencia (East Spain). International Journal of Climatology, 20(12): 1451-1469.
Marquı́nez, J., Lastra, J. and P. Garcı́a (2003). Estimation models for precipitation in mountainous regions: The use of GIS and multivariate analysis. Journal of Hydrology, 270(1-2): 1-11.
Miao, L., Jun, X. and M. Dejuan (2012). Long-term Trend Analysis of Seasonal Precipitation for Beijing, China. Journal of Resources and Ecology, 3(1): 64-72.
Modarres, R. and A. Sarhadi (2009). Rainfall trends analysis of Iran in the last half of the twentieth century. Journal of Geophysical Research, 114(D3): 1-9.
Nakhei, M. and S.A. Nasr (2012). A combined Wavelet- Artificial Neural Network model and its application to the prediction of groundwater level fluctuations. Journal of Geopersia, 2(2): 77-91.
Nasri, M. and R. Modarres (2009). Dry spell trend analysis of Isfahan Province, Iran. International Journal of Climatology, 29(10): 1430-1438.
Nejad, F.H. and V. Nourani (2012). Elevation of wavelet denoising performance via an ANN based stream flow forecasting model. International Journal of Computer Science & Management, 1(4): 764-770.
Nury, A.H., Hasan, K. and M.J.B. Alam (2015). Comparative study of wavelet-ARIMA and wavelet-ANN models for temperature time series data in northeastern Bangladesh. Journal of King Saud University-Science (http://dx.doi. org/10.1016/j.jksus.2015.12.002).
Nury, A.H. and K. Hasan (2015). Analysis of drought in northwestern Bangladesh using standardized precipitation index and its relation to southern oscillation index. Environmental Engineering Research. Doi: http://dx.doi. org/10.4491/eer.2015.115.
Panigrahi, S., Karali, Y. and H.S. Behera (2013). Time series forecasting using evolutionary neural network. International Journal of Computer Applications, 75(10): 13-17.
Partal, T. and E. Kahya (2005). Trend analysis in Turkish precipitation data. Hydrological Processes, 20(9): 2011-
2026.
Pinto, S.C., Adamowski, J. and G. Oron (2012). Forecasting urban water demand via wavelet-denoising and neural network models. Water Resources Management, 26(12): 3539-3558.
Ramana, R.V., Krishna, B., Kumar, S.R. and N.G. Pandey (2013). Monthly rainfall prediction using wavelet neural network analysis. Water Resource Management, 27(10): 3697-3711.
Ramazanipour, M. and M. Roshani (2011). Test and trend analysis of precipitation and discharge in the north of
Iran. World Applied Sciences Journal, 14(9): 1286-1290.
Salmi, T., Määttää , A., Anttila, P., Airola, T.R. and T. Amnell (2002). Detecting trends of annual values of atmospheric pollutants by the Mann-Kendall test and Sen’s slope estimates – the Excel template application MAKESENS. Finnish Meteorological Institute, Helsinki. ISBN: 951- 697-563-1.
Shahid, S. and O.S. Khairulmaini (2009). Spatio-temporal variability of rainfall over Bangladesh during the time period 1969-2003. Asia-Pacific Journal of Atmospheric Sciences, 45(3): 375-389.
Shamsuddin, S. (2007). Spatial and temporal characteristics of droughts in the western part of Bangladesh. Hydrological Processes, 22(13): 2235-2247.
Shamsuddin, S. (2011). Trends in extreme rainfall events of Bangladesh. Theoretical and Applied Climatology, 104(3): 489-499.
Stern, N. (2007). Report of the Stern Review: The Economics of Climate Change. HM Treasury, London. ISBN:
9780521700801.
Wadi, A.S., Ismail, T.M., Alkhahazaleh, H.M. and A.S. Karim (2011). Selecting wavelet transforms model in forecasting financial time series data based on ARIMA model. Applied Mathematical Sciences, 5(7): 315-326.
Yu, I.K., Kim, C.I. and Y.H. Song (2000). A novel short- term load forecasting technique using wavelet transform analysis. Electric Power Systems Research-Journal, 28(6): 537-549.
Yue, S., Pilon, P. and G. Cavadias (2002). Power of the Mann- Kendall and Spearman’s rho tests for detecting monotonic trends in hydrological series. Journal of Hydrology, 259(1-4): 254-271.
Yue, S. and C. Wang (2004). The Mann-Kendall Test Modified by Effective Sample Size to Detect Trend in Serially Correlated Hydrological Series. Water Resources Management, 18(3): 201-218.