Interpretability analysis of deep models for COVID-19 detection
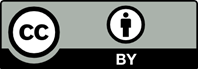
During the coronavirus disease 2019 (COVID-19) pandemic, various research disciplines collaborated to address the impacts of severe acute respiratory syndrome coronavirus-2 infections. This paper presents an interpretability analysis of a convolutional neural network-based model designed for COVID-19 detection using audio data. We explore the input features that play a crucial role in the model’s decision-making process, including spectrograms, fundamental frequency (F0), F0 standard deviation, sex, and age. Subsequently, we examine the model’s decision patterns by generating heat maps to visualize its focus during the decision-making process. Emphasizing an explainable artificial intelligence approach, our findings demonstrate that the examined models can make unbiased decisions even in the presence of noise in training set audios, provided appropriate preprocessing steps are undertaken. Our top-performing model achieves a detection accuracy of 94.44%. Our analysis indicates that the analyzed models prioritize high-energy areas in spectrograms during the decision process, particularly focusing on high-energy regions associated with prosodic domains, while also effectively utilizing F0 for COVID-19 detection.
- Who Director-General’s Opening Remarks at the Media Briefing on Covid-19. World Health Organization; 2020. Available from: https://www.who.int/director-general/ speeches/detail/who-director-general-s-opening-remarks-at-the-media-briefing-on-covid-19-11-march-2020 [Last accessed on 2024 Jul 19].
- Brinati D, Campagner A, Ferrari D, Locatelli M, Banfi G, Cabitza F. Detection of COVID-19 infection from routine blood exams with machine learning: A feasibility study. J Med Syst. 2020;44(8):135. doi: 10.1007/s10916-020-01597-4
- Zoabi Y, Deri-Rozov S, Shomron N. Machine learning-based prediction of COVID-19 diagnosis based on symptoms. NPJ Digit Med. 2021;4(1):3. doi: 10.1038/s41746-020-00372-6
- Ozturk T, Talo M, Yildirim EA, Baloglu UB, Yildirim O, Acharya UR. Automated detection of COVID-19 cases using deep neural networks with x-ray images. Comput Biol Med. 2020;121:103792. doi: 10.1016/j.compbiomed.2020.103792
- Acar E, Şahin E, Yılmaz İ. Improving effectiveness of different deep learning-based models for detecting COVID-19 from computed tomography (CT) images. Neural Comput Appl. 2021;33:17589-17609. doi: 10.1007/s00521-021-06344-5
- Han J, Brown C, Chauhan J, et al. Exploring Automatic COVID-19 Diagnosis via Voice and Symptoms from Crowdsourced Data. In: IEEE International Conference on Acoustics, Speech and Signal Processing. p. 8328-8332. doi: 10.1109/ICASSP39728.2021.9414576
- Brown C, Chauhan J, Grammenos A, et al. Exploring Automatic Diagnosis of COVID-19 from Crowdsourced Respiratory Sound Data. In: Proceedings of the 26th ACM SIGKDD International Conference on Knowledge Discovery and Data Mining, KDD; 2020. p. 3474-3484. doi: 10.1145/3394486.3412865
- Aluísio SM, Camargo Neto AC, Casanova E, et al. Detecting Respiratory Insufficiency via Voice Analysis: The SPIRA Project. In: Practical Machine Learning for Developing Countries on the Tenth International Conference on Learning Representations; 2022.
- Casanova E, Gris L, Camargo A, et al. Deep learning against COVID-19: Respiratory insufficiency detection in Brazilian Portuguese speech. In: Findings of the Association for Computational Linguistics: ACL-IJCNLP; 2021. p. 625-633. doi: 10.18653/v1/2021.findings-acl.55
- Selvaraju RR, Cogswell M, Das A, Vedantam R, Parikh D, Batra D. Grad-cam: Visual Explanations from Deep Networks Via Gradient-Based Localization. In: Proceedings of the IEEE International Conference on Computer Vision; 2017. p. 618-626. doi: 10.1109/ICCV.2017.74
- Zheng F, Zhang G, Song Z. Comparison of different implementations of MFCC. J Comput Sci Technol. 2001;16(6):582-589. doi: 10.1007/BF02943243
- Gauy MM, Finger M. Audio MFCC-Gram Transformers for Respiratory Insufficiency Detection in COVID-19. In: Proceedings XIII Simpósio Brasileiro de Tecnologia da Informação e da Linguagem Humana, STIL; 2021. p. 143-152. doi: 10.5753/stil.2021.17793
- Kong Q, Cao Y, Iqbal T, Wang Y, Wang W, Plumbley MD. PANNs: Large-Scale Pretrained Audio Neural Networks for Audio Pattern Recognition. Vol. 28. In: IEEE/ACM Transactions on Audio, Speech, and Language Processing; 2020. p. 2880-2894. doi: 10.1109/TASLP.2020.3030497
- Vaswani A, Shazeer N, Parmar N, et al. Attention is all you need. Adv Neural Inform Process Syst. 2017;30:5998-6008. doi: 10.5555/3295222.3295349
- Bartl-Pokorny KD, Pokorny FB, Batliner A, et al. The voice of COVID-19: Acoustic correlates of infection. J Acoust Soc Am. 2021;149(6):4377. doi: 10.1121/10.0005194
- Berti LC, Spazzapan EA, Pereira PL, et al. Mudanças Nos Parâmetros Acústicos da voz em Brasileiros com COVID-19. In: XXIX Congresso Brasileiro e o IX Congresso Internacional de Fonoaudiologia; 2021. p. 2819-2819.
- Berti LC, Spazzapan EA, Queiroz M, et al. Fundamental frequency related parameters in Brazilians with COVID-19. J Acoust Soc Am. 2023;153:576-585. doi: 10.1121/10.0016848
- Fernandes-Svartman FR, Berti LC, Martins MVM, de Medeiros BR, Queiroz M. Temporal Prosodic Cues for COVID-19 in Brazilian Portuguese Speakers. In: Proceedings Speech Prosody; 2022. p. 210-214. doi: 10.21437/SpeechProsody.2022-43
- Schuller BW, Batliner A, Bergler C, et al. The INTERSPEECH 2021 Computational Paralinguistics Challenge: COVID-19 Cough, COVID-19 Speech, Escalation and Primates. In: 22nd Annual Conference of the International Speech Communication Association, INTERSPEECH; 2021. doi: 10.21437/Interspeech.2021-19
- Casanova E, Cândido A, Fernandes RC, et al. Transfer Learning and Data Augmentation Techniques to the COVID-19 Identification Tasks in COMPARE 2021. In: 22nd Annual Conference of the International Speech Communication Association; 2021. p. 4301-4305. doi: 10.21437/Interspeech.2021-1798
- Gauy MM, Berti LC, Cândido Júnior A, et al. Discriminant Audio Properties in Deep Learning Based Respiratory Insufficiency Detection in Brazilian Portuguese. In: Artificial Intelligence in Medicine: 21st International Conference on Artificial Intelligence in Medicine; 2023. p. 271-275. doi: 10.1007/978-3-031-34344-5_32
- Sobahi N, Atila O, Deniz E, Sengur A, Acharya UR. Explainable COVID-19 detection using fractal dimension and vision Transformer with Grad-CAM on cough sounds. Biocybern Biomed Eng. 2022;42(3):1066-1080. doi: 10.1016/j.bbe.2022.08.005
- Moujahid H, Cherradi B, Al-Sarem M, et al. Combining CNN and Grad-CAM for COVID-19 disease prediction and visual explanation. Intell Autom Soft Comput. 2022;32(2):723-745. doi: 10.32604/iasc.2022.022179
- Panwar H, Gupta P, Siddiqui MK, Morales-Menendez R, Bhardwaj P, Singh V. A deep learning and Grad-CAM based color visualization approach for fast detection of COVID-19 cases using chest x-ray and ct-scan images. Chaos Solitons Fractals. 2020;140:110190. doi: 10.1016/j.chaos.2020.110190
- Gauy MM, Finger M. Pretrained Audio Neural Networks for Speech Emotion Recognition in Portuguese. In: First Workshop on Automatic Speech Recognition for Spontaneous and Prepared Speech Speech emotion recognition in Portuguese, SE&R; 2022.
- Xu X, Dinkel H, Wu M, Xie Z, Yu K. Investigating Local and Global Information for Automated Audio Captioning with Transfer Learning. In: IEEE International Conference on Acoustics, Speech and Signal Processing (ICASSP); 2021. p. 905-909. doi: 10.1109/ICASSP39728.2021.9413982
- Zhang H, Cisse M, Dauphin YN, Lopez-Paz D. mixup: Beyond Empirical Risk Minimization. In: International Conference on Learning Representations; 2018.
- Xu K, Feng D, Mi H, et al. Mixup-based acoustic scene classification using multi-channel convolutional neural network. In: Advances in Multimedia Information Processing. Vol. 11166. Cham: Springer; 2018. p. 14-23. doi: 10.1007/978-3-030-00764-5_2
- Park DS, Chan W, ZhangY, et al. SpecAugment: A simple data augmentation method for automatic speech recognition. Proc Interspeech. 2019;1:2613-2617. doi: 10.21437/Interspeech.2019-2680
- Brigham EO, Morrow R. The fast fourier transform. IEEE Spectrum. 1967;4(12):63-70. doi: 10.1109/MSPEC.1967.5217220
- Kingma DP, Ba J. Adam: A Method for Stochastic Optimization. In: International Conference on Learning Representations; 2015.
- Frota S. Prosody and Focus in European Portuguese: Phonological Phrasing and Intonation. London: Routledge; 2014.
- Tenani L. Domínios Prosódicos no Português do Brasil: Implicações Para Prosódia e Para a Aplicação de Processos Fonológicos. Sínteses; 2023. p. 8. Available from: https:// revistas.iel.unicamp.br/index.php/sinteses/article/ view/6275 [Last accessed on 2024 July 29].