Artificial intelligence in the battle against COVID-19: A comprehensive review
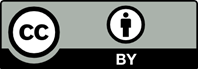
The COVID-19 pandemic has precipitated a global crisis, affecting all facets of human life. The rapid spread of the virus necessitated urgent responses from the healthcare sector, with artificial intelligence (AI) taking center stage as a pivotal tool in this fight. This paper provides a comprehensive review of the multifaceted role of AI during the pandemic, spanning from early detection and diagnosis to treatment, management, and the development of vaccines. We delve into the ethical and societal implications of deploying AI in such critical scenarios, discussing data privacy, algorithmic bias, and accessibility. The paper also presents various case studies, highlighting country-specific implementations and the dichotomy of success stories and failures. Furthermore, we explore the future directions of AI in healthcare, emphasizing emerging technologies and policy recommendations that could shape post-pandemic health-care systems. The conclusion synthesizes these insights, reflecting on the lessons learned and the prospective landscape of AI in global health. This paper aims to serve as a cornerstone for policymakers, health-care providers, and AI researchers, guiding the responsible and effective integration of AI in future health-care strategies.
- Marques ES, Moraes CL, Hasselmann MH, Deslandes SF, Reichenheim ME. Violence against women, children, and adolescents during the COVID-19 pandemic: Overview, contributing factors, and mitigating measures. Cad Saude Publica. 2020;36(4):e00074420. doi: 10.1590/0102-311X00074420
- Maital S, Barzani E. The global economic impact of COVID-19: A summary of research. Samuel Neaman Inst Nat Policy Res. 2020;2020:1-12.
- Khan KS, Mamun MA, Griffiths MD, Ullah I. The mental health impact of the COVID-19 pandemic across different cohorts. Int J Ment Health Addict. 2022;20:380-386. doi: 10.1007/s11469-020-00367-0
- Msemburi W, Karlinsky A, Knutson V, Aleshin- Guendel S, Chatterji S, Wakefield J. The WHO estimates of excess mortality associated with the COVID-19 pandemic. Nature. 2023;613:130-137. doi: 10.1038/s41586-022-05522-2
- Assefa Y, Gilks CF, Pas R, Reid S, Gete DG, Van Damme W. Reimagining global health systems for the 21st century: lessons from the COVID-19 pandemic. BMJ Glob Health. 2021;6:e004882. doi: 10.1136/bmjgh-2021-004882
- Vaishya R, Javaid M, Khan IH, Haleem A. Artificial intelligence (AI) applications for COVID-19 pandemic. Diabetes Metab Syndr Clin Res Rev. 2020;14:337-339. doi: 10.1016/j.dsx.2020.04.012
- Darapaneni N, Sreevanth AT, Paduri AR, et al. Explainable Diagnosis, Lesion Segmentation and Quantification of COVID-19 Infection from CT Images using Convolutional Neural Networks. In: 2022 IEEE 13th Annual Information Technology, Electronics and Mobile Communication Conference (IEMCON). IEEE; 2022. p. 171-178. doi: 10.1109/IEMCON53756.2022.9623045
- Huang S, Yang J, Fong S, Zhao Q. Artificial intelligence in the diagnosis of COVID-19: Challenges and perspectives. Int J Biol Sci. 2021;17:1581. doi: 10.7150/ijbs.58855
- Silva C, Saraee D. Literature review on epidemiological modelling, spatial modelling and artificial intelligence for COVID-19. J Adv Med Med Res. 2021;33:8-21. doi: 10.9734/jammr/2021/v33i530841
- Vicari R, Komendatova N. Systematic meta-analysis of research on AI tools to deal with misinformation on social media during natural and anthropogenic hazards and disasters. Humanit Soc Sci Commun. 2023;10:1-14. doi: 10.1057/s41599-023-01838-0
- Al Sulais E, Mosli M, AlAmeel T. The psychological impact of COVID-19 pandemic on physicians in Saudi Arabia: A cross-sectional study. Saudi J Gastroenterol. 2020;26:249. doi: 10.4103/sjg.SJG_173_20
- Poon YS, Lin YP, Griffiths P, Yong KK, Seah B, Liaw SY. A global overview of healthcare workers’ turnover intention amid COVID-19 pandemic: A systematic review with future directions. Hum Resour Health. 2022;20:70. doi: 10.1186/s12960-022-00764-7
- Mhlanga D. The role of artificial intelligence and machine learning amid the COVID-19 pandemic: What lessons are we learning on 4IR and the sustainable development goals. Int J Environ Res Public Health. 2022;19:1879. doi: 10.3390/ijerph19041879
- Yogi MK, Garikipati J. Future scope of artificial intelligence in healthcare for COVID-19. In: Emerging Technologies for Combatting Pandemics. United Kingdom: Taylor & Francis; 2022. p. 85-100. doi: 10.1201/9781003324447-5
- Pham QV, Nguyen DC, Huynh-The T, Hwang WJ, Pathirana PN. Artificial intelligence (AI) and big data for coronavirus (COVID-19) pandemic: A survey on the state-of-the-arts. IEEE Access. 2020;8:130820-130839. doi: 10.1109/ACCESS.2020.3009328
- Naudé W. Artificial Intelligence against COVID-19: An Early Review. IZA Discussion Paper No. 13110; 2020. doi: 10.2139/ssrn.3568314
- Adly AS, Adly AS, Adly MS. Approaches based on artificial intelligence and the internet of intelligent things to prevent the spread of COVID-19: Scoping review. J Med Internet Res. 2020;22:e19104. doi: 10.2196/19104
- Jiang F, Jiang Y, Zhi H, et al. Artificial intelligence in healthcare: Past, present and future. Stroke Vasc Neurol. 2017;2:230-243. doi: 10.1136/svn-2017-000101
- Hamet P, Tremblay J. Artificial intelligence in medicine. Metabolism. 2017;69:S36-S40. doi: 10.1016/j.metabol.2017.01.011
- Shortliffe EH. MYCIN: A Rule-Based Computer Program for Advising Physicians Regarding Antimicrobial Therapy Selection. PhD Thesis. Stanford University; 1974. doi: 10.1145/1408800.1408906
- Shortliffe EH, Davis R, Axline SG, Buchanan BG, Green CC, Cohen SN. Computer-based consultations in clinical therapeutics: Explanation and rule acquisition capabilities of the MYCIN system. Comput Biomed Res. 1975;8:303-320. doi: 10.1016/0010-4809(75)90009-9
- Miller RA, Pople HE Jr., Myers JD. Internist-I, an experimental computer-based diagnostic consultant for general internal medicine. In: Computer-assisted Medical Decision Making. Berlin: Springer; 1985. p. 139-158. doi: 10.1007/978-1-4612-5108-8_8
- Bradley AP. Machine Learning for Medical Diagnostics: Techniques for Feature Extraction, Classification, and Evaluation. Australia: The University of Queensland; 1996.
- Kononenko I. Inductive and Bayesian learning in medical diagnosis. Appl Artif Intell. 1993;7:317-337. doi: 10.1080/08839519308949977
- Zupan B, Demšar J, Kattan MW, Beck JR, Bratko I. Machine learning for survival analysis: A case study on recurrence of prostate cancer. Artif Intell Med. 2000;20:59-75. doi: 10.1016/S0933-3657(00)00053-1
- Miller AS, Blott BH, Hames TK. Review of neural network applications in medical imaging and signal processing. Med Biol Eng Comput. 1992;30:449-464. doi: 10.1007/BF02441652
- Lo SCB, Chan HP, Lin JS, Li H, Freedman MT, Mun SK. Artificial convolution neural network for medical image pattern recognition. Neural Netw. 1995;8:1201-1214. doi: 10.1016/0893-6080(95)00061-5
- Larranaga P, Calvo B, Santana R, et al. Machine learning in bioinformatics. Brief Bioinform. 2006;7:86-112. doi: 10.1093/bib/bbk007
- Dubitzky W, Granzow M, Berrar DP. Fundamentals of Data Mining in Genomics and Proteomics. Berlin: Springer Science & Business Media; 2007. doi: 10.1007/978-0-387-47509-7
- Hayes WS, Borodovsky M. How to interpret an anonymous bacterial genome: Machine learning approach to gene identification. Genome Res. 1998;8:1154-1171. doi: 10.1101/gr.8.11.1154
- Zhavoronkov A, Ivanenkov YA, Aliper A, et al. Deep learning enables rapid identification of potent DDR1 kinase inhibitors. Nat Biotechnol. 2019;37:1038-1040. doi: 10.1038/s41587-019-0224-x
- Burbidge R, Trotter M, Buxton B, Holden S. Drug design by machine learning: Support vector machines for pharmaceutical data analysis. Comput Chem. 2001;26:5-14. doi: 10.1016/S0097-8485(01)00094-8
- Zernov VV, Balakin KV, Ivaschenko AA, Savchuk NP, Pletnev IV. Drug discovery using support vector machines. The case studies of drug-likeness, agrochemical-likeness, and enzyme inhibition predictions. J Chem Inf Comput Sci. 2003;43:2048-2056. doi: 10.1021/ci0341161
- Collins FS, Morgan M, Patrinos A. The human genome project: Lessons from large-scale biology. Science. 2003;300:286-290. doi: 10.1126/science.1084564
- Wallach I, Dzamba M, Heifets A. AtomNet: A deep convolutional neural network for bioactivity prediction in structure-based drug discovery. arXiv preprint arXiv:1510.02855. 2015.
- Piwek L, Ellis DA, Andrews S, Joinson A. The rise of consumer health wearables: Promises and barriers. PLoS Med. 2016;13:e1001953. doi: 10.1371/journal.pmed.1001953
- Kaur G. Pandemic management via technology: A review. Management. 2011;40:181-187. doi: 10.1016/j.indmarman.2010.06.026
- Sadilek A, Kautz H, Silenzio V. Predicting Disease Transmission from Geo-Tagged Micro-Blog Data. Vol. 26. In: Proceedings of the AAAI Conference on Artificial Intelligence. 2012. p. 136-142. doi: 10.1609/aaai.v26i1.8103
- Raza Abidi SS, Goh A. Applying Knowledge Discovery to Predict Infectious Disease Epidemics. In: Pacific Rim International Conference on Artificial Intelligence. Berlin: Springer; 1998. p. 170-181. doi: 10.1007/BFb0095267
- Lampos V, Cristianini N. Tracking the Flu Pandemic by Monitoring the Social Web. In: 2010 2nd International Workshop on Cognitive Information Processing. IEEE; 2010. p. 411-416. doi: 10.1109/CIP.2010.5604088
- Choi S, Lee J, Kang MG, Min H, Chang YS, Yoon S. Large-scale machine learning of media outlets for understanding public reactions to nation-wide viral infection outbreaks. Methods. 2017;129:50-59. doi: 10.1016/j.ymeth.2017.04.004
- Bragazzi NL, Dai H, Damiani G, Behzadifar M, Martini M, Wu J. How big data and artificial intelligence can help better manage the COVID-19 pandemic. Int J Environ Res Public Health. 2020;17:3176. doi: 10.3390/ijerph17093176
- Dong D, Tang Z, Wang S, et al. The role of imaging in the detection and management of COVID-19: A review. IEEE Rev Biomed Eng. 2020;14:16-29. doi: 10.1109/RBME.2020.2990959
- Wang L, Lin ZQ, Wong A. Covid-net: A tailored deep convolutional neural network design for detection of covid- 19 cases from chest x-ray images. Sci Rep. 2020;10:19549. doi: 10.1038/s41598-020-76550-z
- Ozturk T, Talo M, Yildirim EA, Baloglu UB, Yildirim O, Acharya UR. Automated detection of COVID-19 cases using deep neural networks with X-ray images. Comput Biol Med. 2020;121:103792. doi: 10.1016/j.compbiomed.2020.103792
- Li L, Qin L, Xu Z, et al. Artificial intelligence distinguishes COVID-19 from community acquired pneumonia on chest CT. Radiology. 2020;296:E65-E71. doi: 10.1148/radiol.2020200905
- Shi F, Xia L, Shan F, et al. Large-scale screening to distinguish between COVID-19 and community-acquired pneumonia using infection size-aware classification. Phys Med Biol. 2021;66:065031. doi: 10.1088/1361-6560/abe838
- Zhang K, Liu X, Shen J, et al. Clinically applicable AI system for accurate diagnosis, quantitative measurements, and prognosis of COVID-19 pneumonia using computed tomography. Cell. 2020;181:1423-1433.e11. doi: 10.1016/j.cell.2020.04.045
- Jiang X, Coffee M, Bari A, et al. Towards an artificial intelligence framework for data-driven prediction of coronavirus clinical severity. Comput Mater Continua. 2020;63:537-551. doi: 10.32604/cmc.2020.010691
- Ahamad MM, Aktar S, Rashed-Al-Mahfuz M, et al. A machine learning model to identify early stage symptoms of SARS-Cov-2 infected patients. Expert Syst Appl. 2020;160:113661. doi: 10.1016/j.eswa.2020.113661
- Zoabi Y, Deri-Rozov S, Shomron N. Machine learning-based prediction of COVID-19 diagnosis based on symptoms. NPJ Digit Med. 2021;4:3. doi: 10.1038/s41746-020-00372-6
- Menni C, Valdes AM, Freydin MB, et al. Real-time tracking of self-reported symptoms to predict potential COVID-19. Nat Med. 2020;26:1037-1040. doi: 10.1038/s41591-020-0916-2
- Miner AS, Laranjo L, Kocaballi AB. Chatbots in the fight against the COVID-19 pandemic. NPJ Digit Med. 2020;3:65. doi: 10.1038/s41746-020-0280-0
- Almalki M, Azeez F. Health chatbots for fighting COVID- 19: A scoping review. Acta Inform Med. 2020;28:241. doi: 10.5455/aim.2020.28.241-247
- Martin A, Nateqi J, Gruarin S, et al. An artificial intelligence-based first-line defence against COVID-19: Digitally screening citizens for risks via a chatbot. Sci Rep. 2020;10:19012. doi: 10.1038/s41598-020-75912-x
- Boucher EM, Harake NR, Ward HE, et al. Artificially intelligent chatbots in digital mental health interventions: A review. Expert Rev Med Devices. 2021;18:37-49. doi: 10.1080/17434440.2021.2013200
- Channa A, Popescu N, Skibinska J, Burget R. The rise of wearable devices during the COVID-19 pandemic: A systematic review. Sensors. 2021;21:5787. doi: 10.3390/s21175787
- Mishra T, Wang M, Metwally AA, et al. Pre-symptomatic detection of COVID-19 from smartwatch data. Nat Biomed Eng. 2020;4:1208-1220. doi: 10.1038/s41551-020-00640-6
- Gandla K, Reddy KTK, Babu PV, Sagapola R, Sudhakar P. A review of artificial intelligence in treatment of COVID-19. J Pharm Negat Results. 2022;13:254-264.
- Smith MD, Smith JC. Repurposing therapeutics for COVID-19: Supercomputer-Based Docking to the SARS-CoV-2 Viral Spike Protein and Viral Spike Protein-Human ACE2 Interface. ChemRxiv. 2020. doi: 10.26434/chemrxiv.11871402
- Zhou Y, Wang F, Tang J, Nussinov R, Cheng F. Artificial intelligence in COVID-19 drug repurposing. Lancet Digit Health. 2020;2:e667-e676. doi: 10.1016/S2589-7500(20)30192-8
- Senior AW, Evans R, Jumper J, et al. Improved protein structure prediction using potentials from deep learning. Nature. 2020;577:706-710. doi: 10.1038/s41586-019-1923-7
- Jang WD, Jeon S, Kim S, Lee SY. Drugs repurposed for COVID-19 by virtual screening of 6,218 drugs and cell-based assay. Proc Natl Acad Sci U S A. 2021;118:e2024302118. doi: 10.1073/pnas.2024302118
- Kandeel M, Al-Nazawi M. Virtual screening and repurposing of FDA approved drugs against COVID-19 main protease. Life Sci. 2020;251:117627. doi: 10.1016/j.lfs.2020.117627
- Rohmetra H, Raghunath N, Narang P, Chamola V, Guizani M, Lakkaniga NR. AI-enabled remote monitoring of vital signs for COVID-19: methods, prospects and challenges. Computing. 2021;105:1-27. doi: 10.1007/s00607-021-00937-7
- Bokolo AJ. Application of telemedicine and eHealth technology for clinical services in response to COVID-19 pandemic. Health Technol (Berl). 2021;11:359-366. doi: 10.1007/s12553-020-00516-4
- Webster P. Virtual health care in the era of COVID-19. Lancet. 2020;395:1180-1181. doi: 10.1016/S0140-6736(20)30818-7
- Judson TJ, Odisho AY, Young JJ, et al. Implementation of a digital chatbot to screen health system employees during the COVID-19 pandemic. J Am Med Inform Assoc. 2020;27:1450-1455. doi: 10.1093/jamia/ocaa130
- Chadi MA, Mousannif H. A reinforcement learning based decision support tool for epidemic control: Validation study for COVID-19. Appl Artif Intell. 2022;36:2031821. doi: 10.1080/08839514.2022.2031821
- Guo X, Chen P, Liang S, et al. PaCAR: COVID-19 pandemic control decision making via large-scale agent-based modeling and deep reinforcement learning. Med Decis Making. 2022;42:1064-1077. doi: 10.1177/0272989X221107902
- Ghahramani M, Pilla F. Leveraging artificial intelligence to analyze the COVID-19 distribution pattern based on socioeconomic determinants. Sustain Cities Soc. 2021;69:102848. doi: 10.1016/j.scs.2021.102848
- Narayan K, Rathore H, Znidi F. Using epidemic modeling, machine learning and control feedback strategy for policy management of COVID-19. IEEE Access. 2022;10:98244- 98258. doi: 10.1109/ACCESS.2022.3206790
- Wang H. The application of artificial intelligence in health care resource allocation before and during the COVID-19 pandemic: Scoping Health Policy. JMIR AI. 2023;5:6.
- Yu L, Halalau A, Dalal B, et al. Machine learning methods to predict mechanical ventilation and mortality in patients with COVID-19. PLoS One. 2021;16:e0249285. doi: 10.1371/journal.pone.0249285
- Douville NJ, Douville CB, Mentz G, et al. Clinically applicable approach for predicting mechanical ventilation in patients with COVID-19. Br J Anaesth. 2021;126:578-589. doi: 10.1016/j.bja.2020.11.034
- Cinelli M, Quattrociocchi W, Galeazzi A, et al. The COVID- 19 social media infodemic. Sci Rep. 2020;10:16598. doi: 10.1038/s41598-020-73510-5
- Pulido CM, Villarejo-Carballido B, Redondo-Sama G, Gómez A. COVID-19 infodemic: More retweets for science-based information on coronavirus than for false information. Int Sociol. 2020;35:377-392. doi: 10.1177/0268580920914755
- Klein AZ, Magge A, O’Connor K, Flores AIJ, Weissenbacher D, Gonzalez Hernandez G. Toward using Twitter for tracking COVID-19: A natural language processing pipeline and exploratory data set. J Med Internet Res. 2021;23:e25314. doi: 10.2196/25314
- Newlands G, Lutz C, Tamò-Larrieux A, Villaronga EF, Harasgama R, Scheitlin G. Innovation under pressure: Implications for data privacy during the Covid-19 pandemic. Big Data Soc. 2020;7:2053951720976680. doi: 10.1177/2053951720976680
- Christofidou M, Lea N, Coorevits P. A literature review on the GDPR, COVID-19 and the ethical considerations of data protection during a time of crisis. Yearb Med Inform. 2021;30:226-232. doi: 10.1055/s-0041-1726512
- Delgado J, Manuel A, Parra I, et al. Bias in algorithms of AI systems developed for COVID-19: A scoping review. J Bioeth Inq. 2022;19:407-419. doi: 10.1007/s11673-022-10200-z
- Queralt-Rosinach N, Kaliyaperumal R, Bernabé CH, et al. Applying the FAIR principles to data in a hospital: Challenges and opportunities in a pandemic. J Biomed Semant. 2022;13:12. doi: 10.1186/s13326-022-00263-7
- Laurencin CT, McClinton A. The COVID-19 pandemic: A call to action to identify and address racial and ethnic disparities. J Racial Ethn Health Disparities. 2020;7:398-402. doi: 10.1007/s40615-020-00756-0
- Garcia Elorrio E, Arrieta J, Arce H, et al. The COVID- 19 pandemic: A call to action for health systems in Latin America to strengthen quality of care. Int J Qual Health Care. 2021;33:mzaa062. doi: 10.1093/intqhc/mzaa062
- Manjarrés Á, Fernández-Aller C, López-Sánchez M, Rodríguez-Aguilar JA, Sierra Castañer M. Artificial intelligence for a fair, just, and equitable world. IEEE Technol Soc Mag. 2021;40:19-24. doi: 10.1109/MTS.2021.3056292
- Sinha A, Rathi M. COVID-19 prediction using AI analytics for South Korea. Appl Intell (Dordr). 2021;51:8579-8597. doi: 10.1007/s10489-021-02352-z
- Heo K, Lee D, Seo Y, Choi H. Searching for digital technologies in containment and mitigation strategies: Experience from South Korea COVID-19. Ann Glob Health. 2020;86:109. doi: 10.5334/aogh.2993
- Chung H, Ko H, Kang WS, et al. Prediction and feature importance analysis for severity of COVID-19 in South Korea using artificial intelligence: Model development and validation. J Med Internet Res. 2021;23:e27060. doi: 10.2196/27060
- Nam T. How did Korea use technologies to manage the COVID-19 crisis? A country report. Int Rev Public Admin. 2020;25:225-242. doi: 10.1080/12294659.2020.1848061
- Lee JK, Lin L, Kang H. The influence of normative perceptions on the uptake of the COVID-19 TraceTogether digital contact tracing system: Cross-sectional study. JMIR Public Health Surveill. 2021;7:e30462. doi: 10.2196/30462
- Stevens H, Haines MB. Tracetogether: Pandemic response, democracy, and technology. East Asian Sci Technol Soc. 2020;14:523-532. doi: 10.1215/18752160-8698301
- Davahli MR, Karwowski W, Fiok K. Optimizing COVID-19 vaccine distribution across the United States using deterministic and stochastic recurrent neural networks. PLoS One. 2021;16:e0253925. doi: 10.1371/journal.pone.0253925
- Jumper J, Evans R, Pritzel A, et al. Highly accurate protein structure prediction with AlphaFold. Nature. 2021;596:583-589. doi: 10.1038/s41586-021-03819-2
- Gunasekeran DV, Tseng RMWW, Tham YC, Wong TY. Applications of digital health for public health responses to COVID-19: A systematic scoping review of artificial intelligence, telehealth and related technologies. NPJ Digital Med. 2021;4:40. doi: 10.1038/s41746-021-00412-9
- Ioannidis JPA, Cripps S, Tanner MA. Forecasting for COVID-19 has failed. Int J Forecast. 2022;38:423-438. doi: 10.1016/j.ijforecast.2020.08.004
- Jayanthi P, Rai BK, Muralikrishna I. The potential of quantum computing in healthcare. In: Technology Road Mapping for Quantum Computing and Engineering. United States: IGI Global; 2022. p. 81-101. doi: 10.4018/978-1-7998-9183-3.ch006
- John G, Sahajpal NS, Mondal AK, et al. Next-generation sequencing (NGS) in COVID-19: A tool for SARS-CoV-2 diagnosis, monitoring new strains and phylodynamic modeling in molecular epidemiology. Curr Issues Mol Biol. 2021;43:845-867. doi: 10.3390/cimb43020061
- Ng WY, Tan TE, Movva PVH, et al. Blockchain applications in health care for COVID-19 and beyond: A systematic review. Lancet Digit Health. 2021;3:e819-e829. doi: 10.1016/S2589-7500(21)00210-7
- Juddoo S, George C, Duquenoy P, Windridge D. Data governance in the health industry: Investigating data quality dimensions within a big data context. Appl Syst Innov. 2018;1:43. doi: 10.3390/asi1040043
- Bernardo T, Sobkowich KE, Forrest RO, et al. Collaborating in the time of COVID-19: The scope and scale of innovative responses to a global pandemic. JMIR Public Health Surveill. 2021;7:e25935. doi: 10.2196/25935
- Dunn P, Hazzard E. Technology approaches to digital health literacy. Int J Cardiol. 2019;293:294-296. doi: 10.1016/j.ijcard.2019.06.039