A bibliometric analysis of using machine learning and artificial intelligence in prostate cancer detection
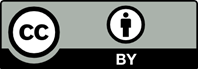
Prostate cancer stands as one of the most prevalent cancers globally among men, exhibiting substantial geographical variations in both incidence and mortality. While developed countries bear a higher incidence, developing countries grapple with elevated mortality rates. The heightened mortality in the latter is attributed to variations in practices that impede early diagnosis. In this context, the integration of artificial intelligence (AI) and machine learning (ML) has become increasingly common to improve the diagnostic accuracy of prostate cancer. This review delves into the existing literature to scrutinize the utilization of AI and ML in the diagnosis of prostate cancer. To compile relevant literature, comprehensive searches were conducted on research databases, including SCOPUS, Web of Science, and Google Scholar, to identify articles related to AI or ML (AI/ML) in the diagnosis and management of prostate cancer. Using a screening criterion, 293 reviewed research papers were identified. The two most consistent themes were predictive modeling and the application of AI/ML tools for cancer grading and radiomics. AI and ML enhance diagnostic accuracy by reducing inter-individual variation in Gleason’s scoring and complimenting the interpretation of multiparametric magnetic resonance imaging (mpMRI). A few publications reported the use of AI/ML tools that combine histopathology with MRI signals. The literature surveyed indicates a compelling potential for AI and ML to improve diagnostic accuracy in prostate cancer. Emerging literature suggests the use of a combination of demographic features, clinical data, serological markers, pathological grading and radiological factors, and genomic data to propose an accurate, non-invasive diagnosis of clinically significant prostate cancer.
- Sung H, Ferlay J, Siegel RL, et al., 2020, Global cancer statistics: GLOBOCAN estimates of incidence and mortality worldwide for 36 cancers in 185 countries. CA Cancer J Clin, 71: 209–249. https://doi.org/0.3322/caac.21660
- Siegel RL, Miller KD, Fuchs HE, et al., 2021, Cancer statistics. CA Cancer J Clin, 71: 7–33. https://doi.org/10.3322/caac.21654
- Center MM, Jemal A, Lortet-Tieulent J, et al., 2012, International variation in prostate cancer incidence and mortality rates. Eur Urol, 61: 1079–1092. https://doi.org/10.1016/j.eururo.2012.02.054
- Rebbeck TR, Devesa SS, Chang BL, et al., 2013, Global patterns of prostate cancer incidence, aggressiveness, and mortality in men of African descent. Prostate Cancer, 2013: 560857. https://doi.org/10.1155/2013/560857
- Zhou CK, Check DP, Lortet-Tieulent J, et al., 2016, Prostate cancer incidence in 43 populations worldwide: An analysis of time trends overall and by age group. Int J Cancer, 138: 1388–1400. https://doi.org/10.1002/ijc.29894
- World Cancer Research Fund/American Institute for Cancer Research, 2018, Continuous Update Project Expert Report. Body Fatness and Weight Gain and the Risk of Cancer. United Kingdom: World Cancer Research Fund.
- Bray F, Pineros M, 2016, Cancer patterns, trends and projections in Latin America and the Caribbean: A global context. Salud Publica Mex, 58: 104–117. https://doi.org/10.21149/spm.v58i2.7779
- Culp MB, Soerjomataram I, Efstathiou JA, et al., 2020, Recent global patterns in prostate cancer incidence and mortality rates. Eur Urol, 77: 38–52. https://doi.org/10.1016/j.eururo.2019.08.005
- Seraphin TP, Joko-Fru WY, Kamate B, et al., 2020, Rising prostate cancer incidence in sub-Saharan Africa: A trend analysis of data from the African Cancer Registry Network. Cancer Epidemiol Biomarkers Prev, 30:158–165. https://doi.org/10.1158/1055-9965.EPI-20-1005
- Lin K, Lipsitz R, Janakiraman S, 2008, Benefits and harms of prostate-specific antigen screening for prostate cancer: An evidence update for the U.S. Preventive Services Task Force. Ann Intern Med, 149: 192–199. https://doi.org/10.7326/0003-4819-149-3-200808050-00009
- Wolf AMD, Wender RC, Etzioni RB, et al., 2010, American Cancer Society guideline for the early detection of prostate cancer: Update 2010. CA Cancer J Clin, 60: 70–98. https://doi.org/10.3322/caac.20066
- US Preventive Services Task Force, Grossman DC, Curry SJ, et al., 2018, Screening for prostate cancer: US Preventive Services Task Force recommendation statement. JAMA, 319: 1901–1913. https://doi.org/10.1001/jama.2018.3710
- Kasivisvanathan V, Rannikko AS, Borghi M, et al., 2018, MRI-targeted or standard biopsy for prostate-cancer diagnosis. N Engl J Med, 378: 1767–1777. https://doi.org/10.1056/NEJMoa1801993
- Rouvière O, Puech P, Renard-Penna R, et al., 2019, Use of prostate systematic and targeted biopsy on the basis of multiparametric MRI in biopsynaive patients (MRI-FIRST): A prospective, multicentre, paired diagnostic study. Lancet Oncol, 20: 100–109. https://doi.org/10.1016/S1470-2045(18)30569-2
- Van der Leest M, Cornel E, Israël B, et al., 2019, Head-to-head comparison of transrectal ultrasound-guided prostate biopsy versus multiparametric prostate resonance imaging with subsequent magnetic resonance-guided biopsy in biopsy-naïve men with elevated prostate-specific antigen: A large prospective multicenter clinical study. Eur Urol, 75: 570–578. https://doi.org/10.1016/j.eururo.2018.11.023
- Mottet N, Bellmunt J, Bolla M, et al., 2017, EAU-ESTRO-SIOG guidelines on prostate cancer. Part 1: Screening, diagnosis, and local treatment with curative intent. Eur Urol, 71: 618–629. https://doi.org/10.1016/j.eururo.2016.08.003
- Rosenkrantz AB, Ayoola A, Hoffman D, et al., 2017, The learning curve in prostate MRI interpretation: Self-directed learning versus continual reader feedback. AJR Am J Roentgenol, 208: W92–W100. https://doi.org/10.2214/AJR.16.16876
- Tran BX, Latkin CA, Sharafeldin N, et al., 2019, Characterizing artificial intelligence applications in cancer research: A latent dirichlet allocation analysis. JMIR Med Inform, 7: e14401. https://doi.org/10.2196/14401
- Pantanowitz L, Quiroga-Garza GM, Bien L, et al., 2020, An artificial intelligence algorithm for prostate cancer diagnosis in whole slide images of core needle biopsies: A blinded clinical validation and deployment study. Lancet Digit Health, 2: e407–e416. https://doi.org/10.1016/S2589-7500(20)30159-X
- Twilt JJ, van Leeuwen KG, Huisman HJ, et al., 2021, Artificial intelligence based algorithms for prostate cancer classification and detection on magnetic resonance imaging: A narrative review. Diagnostics (Basel), 11: 959. https://doi.org/10.3390/diagnostics11060959
- Mata LA, Retamero JA, Gupta RT, et al., 2021, Artificial intelligence-assisted prostate cancer diagnosis: Radiologic-pathologic correlation. Radiographics, 41: 1676–1697. https://doi.org/10.1148/rg.2021210020
- Guo Y, Hao Z, Zhao S, et al., 2020, Artificial intelligence in health care: Bibliometric analysis. J Med Internet Res, 22: e18228. https://doi.org/10.2196/18228
- Adam A, Ras R, Bhattu AS, et al., 2017, “Researching the Research” in prostate cancer: A comparative bibliometric analysis of the top 100 cited articles in the field of prostate cancer. Curr Urol, 11: 26–35. https://doi.org/10.1159/000447191
- Tang X, Li F, Yang Y, et al., 2020, The 100 most cited articles in prostate cancer brachytherapy: Systematic review and bibliometric analysis. J Contemp Brachytherapy, 12: 283–289. https://doi.org/10.5114/jcb.2020.96872
- Ma C, Su H, Li H, 2021, Global research trends on prostate diseases and erectile dysfunction: A bibliometric and visualized study. Front Oncol, 10: 627891. https://doi.org/10.3389/fonc.2020.627891
- Takeshima Y, Suzuki M, Miyakawa J, et al., 2021, Latent prostate cancer among Japanese males: A bibliometric study of autopsy reports from 1980–2016. Jpn J Clin Oncol, 51: 156–159. https://doi.org/10.1093/jjco/hyaa161
- Mushtaq R, Loan FA, 2021, Prostate cancer research: A bibliometric study of India and Iran. Library Philos Pract, 2021: 1–11.
- Aria M, Cuccurullo C, 2017, Bibliometrix: An R-tool for comprehensive science mapping analysis. J Informetr, 11: 959–975.
- Van Eck NJ, Waltman L, 2010, Software survey: VOSviewer, a computer program for bibliometric mapping. Scientometrics, 84: 523–538. https://doi.org/10.1007/s11192-009-0146-3
- Litjens G, Debats O, Barentsz J, et al., 2014, Computer-aided detection of prostate cancer in MRI. IEEE Trans Med Imaging, 33: 1083–1092. https://doi.org/10.1109/TMI.2014.2303821
- Wang J, Wu CJ, Bao ML, et al., 2017, Machine learning-based analysis of MR radiomics can help to improve the diagnostic performance of PI-RADS v2 in clinically relevant prostate cancer. Eur Radiol, 27: 4082–4090. https://doi.org/10.1007/s00330-017-4800-5
- Tabesh A, Teverovskiy M, Pang HY, et al., 2007, Multi-feature prostate cancer diagnosis and Gleason grading of histological images. IEEE Trans Med Imaging, 26: 1366–1378. https://doi.org/10.1109/TMI.2007.898536
- Strom P, Kartasalo K, Olsson H, 2020, Artificial intelligence for diagnosis and grading of prostate cancer in biopsies: A population-based, diagnostic study. Lancet Oncol, 21: 222–232. https://doi.org/10.1016/S1470-2045(19)30738-7
- Chen T, Li M, Gu Y, et al., 2019, Prostate cancer differentiation and aggressiveness: Assessment with a radiomic‐based model vs. PI‐RADS v2. J Magn Reson Imaging, 49: 875–884. https://doi.org/10.1002/jmri.26243
- Bonekamp D, Kohl S, Wiesenfarth M, et al., 2018, Radiomic machine learning for characterization of prostate lesions with MRI: Comparison to ADC values. Radiology, 289: 128–137. https://doi.org/10.1148/radiol.2018173064
- Nir G, Hor S, Karimi D, et al., 2018, Automatic grading of prostate cancer in digitized histopathology images: Learning from multiple experts. Med Image Anal, 50: 167–180. https://doi.org/10.1016/j.media.2018.09.005
- Gorelick L, Veksler O, Gaed M, et al., 2013, Prostate histopathology: Learning tissue component histograms for cancer detection and classification. IEEE Trans Med Imaging, 32: 1804–1818.
- Monaco JP, Tomaszewski JE, Feldman MD, et al., 2010, High-throughput detection of prostate cancer in histological sections using probabilistic pairwise Markov models. Med Image Anal, 14: 617–629. https://doi.org/10.1016/j.media.2010.04.007
- Ginsburg SB, Algohary A, Pahwa S, et al., 2017, Radiomic features for prostate cancer detection on MRI differ between the transition and peripheral zones: Preliminary findings from a multi‐institutional study. J Magn Reson Imaging, 46: 184–193. https://doi.org/10.1002/jmri.25562
- Antonelli M, Johnston EW, Dikaios N, et al., 2019, Machine learning classifiers can predict Gleason pattern 4 prostate cancer with greater accuracy than experienced radiologists. Eur Radiol, 29: 4754–4764. https://doi.org/10.1007/s00330-019-06244-2
- Nir G, Karimi D, Goldenberg SL, et al., 2019, Comparison of artificial intelligence techniques to evaluate performance of a classifier for automatic grading of prostate cancer from digitized histopathologic images. JAMA Netw Open, 2: e190442. https://doi.org/10.1001/jamanetworkopen.2019.0442
- Wang Z, Liu C, Cheng D, et al., 2018, Automated detection of clinically significant prostate cancer in mp-MRI images based on an end-to-end deep neural network. IEEE Trans Med Imaging, 37: 1127–1139. https://doi.org/10.1109/TMI.2017.2789181
- Gertych A, Ing N, Ma Z, et al., 2015, Machine learning approaches to analyze histological images of tissues from radical prostatectomies. Comput Med Imaging Graph, 46: 197–208. https://doi.org/10.1016/j.compmedimag.2015.08.002
- Tiwari P, Kurhanewicz J, Madabhushi A, 2013, Multi-kernel graph embedding for detection, Gleason grading of prostate cancer via MRI/MRS. Med Image Anal, 17: 219–235. https://doi.org/10.1016/j.media.2012.10.004
- Yuan Y, Qin W, Buyyounouski M, et al., 2019, Prostate cancer classification with multiparametric MRI transfer learning model. Med Phys, 46: 756–765. https://doi.org/10.1002/mp.13367
- Kwak JT, Sankineni S, Xu S, et al., 2016, Correlation of magnetic resonance imaging with digital histopathology in prostate. Int J Comput Assist Radiol Surg, 11: 657–666. https://doi.org/10.1007/s11548-015-1287-x
- Ozer S, Langer DL, Liu X, et al., 2010, Supervised and unsupervised methods for prostate cancer segmentation with multispectral MRI. Med Phys, 37: 1873–1883. https://doi.org/10.1118/1.3359459
- Lucas M, Jansen I, Savci-Heijink CD, et al., 2019, Deep learning for automatic Gleason pattern classification for grade group determination of prostate biopsies. Virchows Arch, 475: 77–83. https://doi.org/10.1007/s00428-019-02577-x
- Tiwari P, Viswanath S, Kurhanewicz J, et al., 2012, Multimodal wavelet embedding representation for data combination (MaWERiC): Integrating magnetic resonance imaging and spectroscopy for prostate cancer detection. NMR Biomed, 25: 607–619. https://doi.org/10.1002/nbm.1777
- Hjørland B, 2013, Citation analysis: A social and dynamic approach to knowledge organization. Inform Process Manag, 49: 1313–1325.
- Schelb P, Kohl S, Radtke JP, et al., 2019, Classification of cancer at prostate MRI: Deep learning versus clinical PI-RADS assessment. Radiology, 293: 607–617. https://doi.org/10.1148/radiol.2019190938
- Epstein JI, Egevad L, Amin MB, et al., 2016, The 2014 International Society of Urological Pathology (ISUP) consensus conference on Gleason grading of prostatic carcinoma. Am J Surg Pathol, 40: 244–252. https://doi.org/10.1097/PAS.0000000000000530
- Fehr D, Veeraraghavan H, Wibmer A, et al., 2015, Automatic classification of prostate cancer Gleason scores from multiparametric magnetic resonance images. Proc Natl Acad Sci, 112: E6265–E6273. https://doi.org/10.1073/pnas.1505935112
- Epstein JI, Zelefsky MJ, Sjoberg DD, et al., 2016, A contemporary prostate cancer grading system: A validated alternative to the Gleason score. Eur Urol, 69: 428–435. https://doi.org/10.1016/j.eururo.2015.06.046
- Wibmer A, Hricak H, Gondo T, et al., 2015, Haralick texture analysis of prostate MRI: utility for differentiating non-cancerous prostate from prostate cancer and differentiating prostate cancers with different Gleason scores. Eur Radiol, 25: 2840–2850. https://doi.org/10.1007/s00330-015-3701-8
- Weinreb JC, Barentsz JO, Choyke PL, et al., 2016, PI-RADS prostate imaging-reporting and data system: 2015, version 2. Eur Urol, 69: 16–40. https://doi.org/10.1016/j.eururo.2015.08.052
- Campanella G, Hanna MG, Geneslaw L, et al., 2019, Clinical-grade computational pathology using weakly supervised deep learning on whole slide images. Nat Med, 25: 1301–1309. https://doi.org/10.1038/s41591-019-0508-1
- Matoso A, Epstein JI, 2019, Defining clinically significant prostate cancer on the basis of pathological findings. Histopathology, 74: 135–145. https://doi.org/10.1111/his.13712
- Wason R, 2018, Deep learning: Evolution and expansion. Cogn Syst Res, 52: 701–708.
- Turkbey B, Shah VP, Pang Y, et al., 2011, Is apparent diffusion coefficient associated with clinical risk scores for prostate cancers that are visible on 3-T MR images. Radiology, 258: 488–495. https://doi.org/10.1148/radiol.10100667
- Song Y, Zhang YD, Yan X, et al., 2018, Computer‐aided diagnosis of prostate cancer using a deep convolutional neural network from multiparametric MRI. J Magn Reson Imaging, 48: 1570–1577. https://doi.org/10.1002/jmri.26047
- Artan Y, Haider MA, Langer DL, et al., 2010, Prostate cancer localization with multispectral MRI using cost-sensitive support vector machines and conditional random fields. IEEE Trans Image Process, 19: 2444–2455. https://doi.org/10.1109/TIP.2010.2048612
- Freedman D, Radke RJ, Zhang T, et al., 2005, Model-based segmentation of medical imagery by matching distributions. IEEE Trans Med Imaging, 24: 281–292. htps://doi.org/10.1109/tmi.2004.841228
- Zhan Y, Ou Y, Feldman M, et al., 2007, Registering histologic and MR images of prostate for image-based cancer detection. Acad Radiol, 14: 1367–1381. https://doi.org/10.1016/j.acra.2007.07.018
- Alkadi R, Taher F, El-Baz A, et al., 2019, A deep learning-based approach for the detection and localization of prostate cancer in T2 magnetic resonance images. J Digit Imaging, 32: 793–807. https://doi.org/10.1007/s10278-018-0160-1
- Bernatz S, Ackermann J, Mandel P, et al., 2020, Comparison of machine learning algorithms to predict clinically significant prostate cancer of the peripheral zone with multiparametric MRI using clinical assessment categories and radiomic features. Eur Radiol, 30: 6757–6769. https://doi.org/10.1007/s00330-020-07064-5
- Corradini D, Brizi L, Gaudiano C, et al., 2021, Challenges in the use of artificial intelligence for prostate cancer diagnosis from multiparametric imaging data. Cancers (Basel), 13: 3944. https://doi.org/10.3390/cancers13163944
- Abdelmaksoud IR, Shalaby A, Mahmoud A, et al., 2021, Precise identification of prostate cancer from DWI using transfer learning. Sensors (Basel), 21: 3664. https://doi.org/10.3390/s21113664
- Donisi L, Cesarelli G, Castaldo A, et al., 2021, A combined radiomics and machine learning approach to distinguish clinically significant prostate lesions on a publicly available MRI dataset. J Imaging, 7: 215. https://doi.org/10.3390/jimaging7100215
- Leo P, Lee G, Shih NN, et al., 2016, Evaluating stability of histomorphometric features across scanner and staining variations: Prostate cancer diagnosis from whole slide images. J Med Imaging (Bellingham), 3: 047502. https://doi.org/10.1117/1.JMI.3.4.047502
- Kwak JT, Hewitt SM, 2017b, Multiview boosting digital pathology analysis of prostate cancer. Comput Methods Programs Biomed, 142: 91–99. https://doi.org/10.1016/j.cmpb.2017.02.023
- Kwak, JT, Hewitt SM, 2017a, Nuclear architecture analysis of prostate cancer via convolutional neural networks. IEEE Access, 5: 18526–18533.
- Han W, Johnson C, Warner A, et al., 2020, Automatic cancer detection on digital histopathology images of mid-gland radical prostatectomy specimens. J Med Imaging (Bellingham), 7: 047501. https://doi.org/10.1117/1.JMI.7.4.047501
- Li M, Chen T, Zhao W, et al., 2020, Radiomics prediction model for the improved diagnosis of clinically significant prostate cancer on biparametric MRI. Quant Imaging Med Surg, 10: 368–379. https://doi.org/10.21037/qims.2019.12.06
- Liang L, Zhi X, Sun Y, et al., 2021, A nomogram based on a multiparametric ultrasound radiomics model for discrimination between malignant and benign prostate lesions. Front Oncol, 11: 610785. https://doi.org/10.3389/fonc.2021.610785
- Li W, Li J, Sarma KV, et al., 2018, Path R-CNN for prostate cancer diagnosis and gleason grading of histological images. IEEE Trans Med Imaging, 38: 945–954. https://doi.org/10.1109/TMI.2018.2875868
- Harmon SA, Tuncer S, Sanford T, et al., 2019, Artificial intelligence at the intersection of pathology and radiology in prostate cancer. Diagn Interv Radiol, 25: 183–188. https://doi.org/10.5152/dir.2019.19125
- Hou Y, Bao ML, Wu CJ, et al., 2020, A radiomics machine learning-based redefining score robustly identifies clinically significant prostate cancer in equivocal PI-RADS score 3 lesions. Abdom Radiol (NY), 45: 4223–4234. https://doi.org/10.1007/s00261-020-02678-1
- Lim CS, Abreu-Gomez J, Thornhill R, et al., 2021, Utility of machine learning of apparent diffusion coefficient (ADC) and T2-weighted (T2W) radiomic features in PI-RADS version 2.1 category 3 lesions to predict prostate cancer diagnosis. Abdom Radiol (NY), 46: 5647–5658. https://doi.org/10.1007/s00261-021-03235-0
- Corbet S, Dowling M, Gao X, et al., 2019, An analysis of the intellectual structure of research on the financial economics of precious metals. Resour Policy, 63: 101416.