Algorithm development and metal oxide nanoparticle analysis in magnetic resonance imaging: Advancing neurodegenerative disease diagnostics
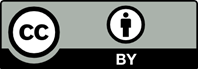
Magnetic resonance imaging (MRI) is critical in the diagnosis of neurodegenerative diseases, enabling the detection of brain lesions. Recent research has examined metallic nanoparticles (NPs) as MRI contrast agents (CAs) that can enhance lesion visibility by altering relaxation times. This study investigates the effects of metal oxide NPs on MRI relaxation times and brain lesion signals and proposes an algorithm for automated relaxation time determination using these NPs. The utilized NPs were synthesized using the sol‒gel method and characterized using Fourier-transform infrared spectroscopy and X-ray diffraction. MRI scans were performed on a phantom infused with varying concentrations of each metal oxide NP to assess changes in pixel signal intensities and relaxation rates. Our analysis involved segmenting the MRI images to focus on regions with different NP concentrations. The algorithm computed the longitudinal relaxation time for each region, revealing that Fe2O3 NPs exhibited the most substantial effect on signal intensity and relaxation time. The results indicated a high correlation (r = 0.9977), demonstrating strong agreement and confirming the reliability of our method. Our findings suggest that metallic oxide NPs, particularly Fe2O3, can considerably alter magnetization and act as effective negative CAs in MRI. These capabilities can improve the monitoring and treatment efficacy of neurodegenerative diseases. Our method for quantifying longitudinal relaxation times can potentially enhance routine clinical MRI assessments, offering a promising tool for future clinical applications.
- Carr DH, Gadian DG. Contrast agents in magnetic resonance imaging. Clin Radiol. 1985;36(6):561-568. doi: 10.1016/S0009-9260(85)80234-8
- Bakshi R. Magnetic resonance imaging advances in multiple sclerosis. J Neuroimaging. 2005;15(4 Suppl):10-14. doi: 10.1177/1051228405283362
- Valverde S, Cabezas M, Roura E, et al. Improving automated multiple sclerosis lesion segmentation with a cascaded 3D convolutional neural network approach. Neuroimage. 2017;155:159-168. doi: 10.1016/j.neuroimage.2017.04.034
- Compston A, Coles A. Multiple sclerosis. Lancet. 2008;372(9648):1502-1517. doi: 10.1016/S0140-6736(08)61620-7
- Wang KY, Chetta J, Bains P, et al. Spectrum of MRI brain lesion patterns in neuromyelitis optica spectrum disorder: A pictorial review. Br J Radiol. 2018;91(1086):20170690. doi: 10.1259/bjr.20170690
- Zhang Y, Yang H, Zhou Z, Huang K, Yang S, Han G. Recent advances on magnetic relaxation switching assay-based nanosensors. Bioconjug Chem. 2017;28(4):869-879. doi: 10.1021/acs.bioconjchem.7b00059
- Na HB, Song IC, Hyeon T. Inorganic nanoparticles for MRI contrast agents. Adv Mater. 2009;21(21):2133-2148. doi: 10.1002/adma.200802366
- Lee N, Hyeon T. Designed synthesis of uniformly sized iron oxide nanoparticles for efficient magnetic resonance imaging contrast agents. Chem Soc Rev. 2012;41(7):2575-2589. doi: 10.1039/C1CS15248C
- Bakshi R, Thompson AJ, Rocca MA, et al. MRI in multiple sclerosis: Current status and future prospects. Lancet Neurol. 2008;7(7):615-625. doi: 10.1016/S1474-4422(08)70137-6
- de Oliveira M, Gianeti TMR, da Rocha FCG, Lisboa- Filho PN, Piacenti-Silva M. A preliminary study of the concentration of metallic elements in the blood of patients with multiple sclerosis as measured by ICP-MS. Sci Rep. 2020;10(1):13112. doi: 10.1038/s41598-020-69979-9
- de Oliveira M, Piacenti-Silva M, da Rocha FCG, Santos JM, Cardoso JS, Lisboa-Filho PN. Lesion volume quantification using two convolutional neural networks in MRIs of multiple sclerosis patients. Diagnostics (Basel). 2022;12(2):230. doi: 10.3390/diagnostics12020230
- Giacoppo S, Galuppo M, Calabrò RS, et al. Heavy metals and neurodegenerative diseases: An observational study. Biol Trace Elem Res. 2014;161(2):151-160. doi: 10.1007/s12011-014-0094-5
- Forte G, Alimonti A, Pino A, et al. Metals and oxidative stress in patients with Parkinson’s disease. Ann Ist Super Sanita. 2005;41(2):189-195.
- Roos PM, Vesterberg O, Syversen T, Flaten TP, Nordberg M. Metal concentrations in cerebrospinal fluid and blood plasma from patients with amyotrophic lateral sclerosis. Biol Trace Elem Res. 2013;151(2):159-170. doi: 10.1007/s12011-012-9547-x
- Squadrone S, Brizio P, Abete MC, Brusco A. Trace elements profile in the blood of Huntington’ disease patients. J Trace Elem Med Biol. 2020;57:18-20. doi: 10.1016/j.jtemb.2019.09.006
- Xiao YD, Paudel R, Liu J, Ma C, Zhang ZS, Zhou SK. MRI contrast agents: Classification and application (Review). Int J Mol Med. 2016;38(5):1319-1326. doi: 10.3892/ijmm.2016.2744
- Liu Z, Zhao M, Wang H, et al. High relaxivity Gd3+-based organic nanoparticles for efficient magnetic resonance angiography. J Nanobiotechnology. 2022;20(1):170. doi: 10.1186/s12951-022-01363-3
- Elhabiri M, Abada S, Sy M, et al. Importance of outer-sphere and aggregation phenomena in the relaxation properties of phosphonated gadolinium complexes with potential applications as MRI contrast agents. Chemistry. 2015;21(17):6535-6546. doi: 10.1002/chem.201500155
- Zheng R, Guo J, Cai X, et al. Manganese complexes and manganese-based metal-organic frameworks as contrast agents in MRI and chemotherapeutics agents: Applications and prospects. Colloids Surfaces B Biointerfaces. 2022;213:112432. doi: 10.1016/j.colsurfb.2022.112432
- Jun YW, Lee JH, Cheon J. Chemical design of nanoparticle probes for high‐performance magnetic resonance imaging. Angew Chem Int Ed Engl. 2008;47(28):5122-5135. doi: 10.1002/anie.200701674
- Cai X, Zhu Q, Zeng Y, Zeng Q, Chen X, Zhan Y. Manganese oxide nanoparticles as MRI contrast agents in tumor multimodal imaging and therapy. Int J Nanomedicine. 2019;14:8321-8344. doi: 10.2147/IJN.S218085
- Olchowy C, Cebulski K, Łasecki M, et al. The presence of the gadolinium-based contrast agent depositions in the brain and symptoms of gadolinium neurotoxicity - A systematic review. PLoS One. 2017;12(2):e0171704. doi: 10.1371/journal.pone.0171704
- Wang J, Mei T, Liu Y, et al. Dual-targeted and MRI-guided photothermal therapy via iron-based nanoparticles-incorporated neutrophils. Biomater Sci. 2021;9(11):3968-3978. doi: 10.1039/D1BM00127B
- Blanco-Andujar C, Walter A, Cotin G, et al. Design of iron oxide-based nanoparticles for MRI and magnetic hyperthermia. Nanomedicine. 2016;11(14):1889-1910. doi: 10.2217/nnm-2016-5001
- Norouzi A, Rahim MSM, Altameem A, et al. Medical image segmentation methods, algorithms, and applications. IETE Tech Rev. 2014;31(3):199-213. doi: 10.1080/02564602.2014.906861
- Castiglioni I, Rundo L, Codari M, et al. AI applications to medical images: From machine learning to deep learning. Phys Med. 2021;83:9-24. doi: 10.1016/j.ejmp.2021.02.006
- Sharma N, Ray A, Shukla K, et al. Automated medical image segmentation techniques. J Med Phys. 2010;35(1):3-14. doi: 10.4103/0971-6203.58777
- Liu H, Ren L, Fan B, Wang W, Hu X, Zhang X. Artificial intelligence algorithm-based MRI in the diagnosis of complications after renal transplantation. Contrast Media Mol Imaging. 2022;2022:8930584. doi: 10.1155/2022/8930584
- Guo YY, Huang YH, Wang Y, Huang J, Lai QQ, Li YZ. Breast MRI tumor automatic segmentation and triple-negative breast cancer discrimination algorithm based on deep learning. Comput Math Methods Med. 2022;2022:2541358. doi: 10.1155/2022/2541358
- Liu B, Tan B, Huang L, et al. Intelligent algorithm-based picture archiving and communication system of mri images and radiology information system-based medical informatization. Contrast Media Mol Imaging. 2021;2021:4997329. doi: 10.1155/2021/4997329
- Wang X, Li X, Chen H, Peng Y, Li Y. Pulmonary MRI radiomics and machine learning: Effect of intralesional heterogeneity on classification of lesion. Acad Radiol. 2022;29:S73-S81. doi: 10.1016/j.acra.2020.12.020
- Chang S, Han K, Lee S, et al. Automated measurement of native T1 and extracellular volume fraction in cardiac magnetic resonance imaging using a commercially available deep learning algorithm. Korean J Radiol. 2022;23(12):1251-1259. doi: 10.3348/kjr.2022.0496
- Bidhult S, Kantasis G, Aletras AH, Arheden H, Heiberg E, Hedström E. Validation of T1 and T2 algorithms for quantitative MRI: Performance by a vendor-independent software. BMC Med Imaging. 2016;16(1):46. doi: 10.1186/s12880-016-0148-6
- Jibon FA, Khandaker MU, Miraz MH, et al. Cancerous and non-cancerous brain MRI classification method based on convolutional neural network and log-polar transformation. Healthcare (Basel). 2022;10(9):1801. doi: 10.3390/healthcare10091801
- Holzinger A, Plass M, Kickmeier-Rust M, et al. Interactive machine learning: Experimental evidence for the human in the algorithmic loop. Appl Intell. 2019;49(7):2401-2414. doi: 10.1007/s10489-018-1361-5
- Topol EJ. High-performance medicine: The convergence of human and artificial intelligence. Nat Med. 2019;25(1):44-56. doi: 10.1038/s41591-018-0300-7
- Modan EM, Plăiașu AG. Advantages and disadvantages of chemical methods in the elaboration of nanomaterials. Ann Dunarea Jos Univ Galati Fascicle IX Metall Mater Sci. 2020;43(1):53-60. doi: 10.35219/mms.2020.1.08
- Priyadharsini CI, Marimuthu G, Pazhanivel T, et al. Sol-Gel synthesis of Co3O4 nanoparticles as an electrode material for supercapacitor applications. J Sol Gel Sci Technol. 2020;96(2):416-422. doi: 10.1007/s10971-020-05393-x
- Alagiri M, Hamid SBA. Sol-gel synthesis of α-Fe2O3 nanoparticles and its photocatalytic application. J Sol Gel Sci Technol. 2015;74(3):783-789. doi: 10.1007/s10971-015-3663-y
- Marlin V, Lugo C, Manuel P, et al. Synthesis and characterization of magnetic nickel used in dry rerforming of methane. Revista Ciencia e Ingeniería.. 2017;38:31-40.
- Pires LA, de Azevedo Silva LJ, Ferrairo BM, et al. Effects of ZnO/TiO2 nanoparticle and TiO2 nanotube additions to dense polycrystalline hydroxyapatite bioceramic from bovine bones. Dent Mater. 2020;36(2):e38-e46. doi: 10.1016/j.dental.2019.11.006
- Gates-Rector S, Blanton T. The Powder Diffraction File: A quality materials characterization database. Powder Diffr. 2019;34(4):352-360. doi: 10.1017/S0885715619000812
- Ahammed KR, Ashaduzzaman M, Paul SC, et al. Microwave assisted synthesis of zinc oxide (ZnO) nanoparticles in a noble approach: Utilization for antibacterial and photocatalytic activity. SN Appl Sci. 2020;2(5):955. doi: 10.1007/s42452-020-2762-8
- Zaparoli HH, De Oliveira M, Lisboa-Filho PN, Piacenti- Silva M. Using zinc particles in a phantom to simulate multiple sclerosis lesions on magnetic resonance imaging. Rev Bras Física Méd. 2021;15:619. doi: 10.29384/rbfm.2021.v15.19849001619
- Oliveira E, Rocha M, Froner AP, Basso N, Zanini M, Papaléo R. Synthesis and nuclear magnetic relaxation properties of composite iron oxide nanoparticles. Quim Nova. 2018;42: 57-64. doi: 10.21577/0100-4042.20170309
- Perona P, Malik J. Scale-space and edge detection using anisotropic diffusion. IEEE Trans Pattern Anal Mach Intell. 1990;12(7):629-639. doi: 10.1109/34.56205
- Gerig G, Kbler O, Kikinis R, Jolesz FA. Nonlinear anisotropic filtering of MRI data. IEEE Trans Med Imaging. 1992;11(2):221-232. doi: 10.1109/42.141646
- Tustison NJ, Avants BB, Cook PA, et al. N4ITK: Improved N3 bias correction. IEEE Trans Med Imaging. 2010;29(6):1310-1320. doi: 10.1109/TMI.2010.2046908
- García-Lorenzo D, Francis S, Narayanan S, Arnold DL, Collins DL. Review of automatic segmentation methods of multiple sclerosis white matter lesions on conventional magnetic resonance imaging. Med Image Anal. 2013;17(1):1-18. doi: 10.1016/j.media.2012.09.004
- Bland JM, Altman DG. Statistical methods for assessing agreement between two methods of clinical measurement. Lancet. 1986;1(8476):307-310.
- Wu S, He M, Yang M, Zhang B, Wang F, Li Q. Near-infrared spectroscopy study of serpentine minerals and assignment of the OH group. Crystals. 2021;11(9):1130. doi: 10.3390/cryst11091130
- Packiaraj R, Devendran P, Venkatesh KS, Asath Bahadur S, Manikandan A, Nallamuthu N. Electrochemical investigations of magnetic Co3O4 nanoparticles as an active electrode for supercapacitor applications. J Supercond Nov Magn. 2019;32(8):2427-2436. doi: 10.1007/s10948-018-4963-6
- Binitha NN, Suraja PV, Yaakob Z, Resmi MR, Silija PP. Simple synthesis of Co3O4 nanoflakes using a low temperature sol-gel method suitable for photodegradation of dyes. J Sol Gel Sci Technol. 2010;53(2):466-469. doi: 10.1007/s10971-009-2098-8
- Farhadi S, Pourzare K, Sadeghinejad S. Simple preparation of ferromagnetic Co3O4 nanoparticles by thermal dissociation of the [CoII(NH3)6](NO3)2 complex at low temperature. J Nanostructure Chem. 2013;3(1):16. doi: 10.1186/2193-8865-3-16
- Sundar S, Venkatachalam G, Kwon S. Biosynthesis of copper oxide (CuO) nanowires and their use for the electrochemical sensing of dopamine. Nanomaterials. 2018;8(10):823. doi: 10.3390/nano8100823
- Usha V, Kalyanaraman S, Thangavel R, Vettumperumal R. Effect of catalysts on the synthesis of CuO nanoparticles: Structural and optical properties by sol-gel method. Superlattices Microstruct. 2015;86:203-210. doi: 10.1016/j.spmi.2015.07.053
- Zayyoun N, Bahmad L, Laânab L, Jaber B. The effect of pH on the synthesis of stable Cu2O/CuO nanoparticles by sol-gel method in a glycolic medium. Appl Phys A. 2016;122(5):488. doi: 10.1007/s00339-016-0024-9
- Abdulkadir I, Abdallah HMI, Jonnalagadda SB, Martincigh BS. The effect of synthesis method on the structure, and magnetic and photocatalytic properties of hematite (α-Fe2O3) nanoparticles. South Afr J Chem. 2018;71:68-78.
- Raja K, Mary Jaculine M, Jose M, et al. Sol-gel synthesis and characterization of α-Fe2O3 nanoparticles. Superlattices Microstruct. 2015;86:306-312. doi: 10.1016/j.spmi.2015.07.044
- Namduri H, Nasrazadani S. Quantitative analysis of iron oxides using Fourier transform infrared spectrophotometry. Corros Sci. 2008;50(9):2493-2497. doi: 10.1016/j.corsci.2008.06.034
- Shamim A, Ahmad Z, Mahmood S, Ali U, Mahmood T, Nizami ZA. Synthesis of nickel nanoparticles by sol-gel method and their characterization. Open J Chem. 2019;2(1):16-20. doi: 10.30538/psrp-ojc2019.0009
- Gogoi P, Saikia BJ, Dolui SK. Effects of nickel oxide (NiO) nanoparticles on the performance characteristics of the jatropha oil based alkyd and epoxy blends. J Appl Polym Sci. 2015;132(8):n/a-n/a. doi: 10.1002/app.41490
- Jung HJ, Lee S, Yu Y, Hong SM, Choi HC, Choi MY. Low-temperature hydrothermal growth of ZnO nanorods on sol-gel prepared ZnO seed layers: Optimal growth conditions. Thin Solid Films. 2012;524:144-150. doi: 10.1016/j.tsf.2012.10.007
- Patel M, Mishra S, Verma R, Shikha D. Synthesis of ZnO and CuO nanoparticles via sol gel method and its characterization by using XRD and FT-IR analysis. Research Square. 2022;1:1-13. doi: 10.21203/rs.3.rs-1234162/v1
- Kaningini AG, Azizi S, Sintwa N, et al. Effect of optimized precursor concentration, temperature, and doping on optical properties of ZnO nanoparticles synthesized via a green route using bush tea (Athrixia phylicoides DC.) leaf extracts. ACS Omega. 2022;7(36):31658-31666. doi: 10.1021/acsomega.2c00530
- Nayan MB, Jagadish K, Abhilash MR, Namratha K, Srikantaswamy S. Comparative study on the effects of surface area, conduction band and valence band positions on the photocatalytic activity of ZnO-MxOy heterostructures. J Water Resour Prot. 2019;11(3):357-370. doi: 10.4236/jwarp.2019.113021
- Chen C, Ge J, Gao Y, et al. Ultrasmall superparamagnetic iron oxide nanoparticles: A next generation contrast agent for magnetic resonance imaging. Wiley Interdiscip Rev Nanomed Nanobiotechnol. 2022;14(1):e1740. doi: 10.1002/wnan.1740
- Zottis ADA, Beltrame JM, Lara LRS, et al. Pheomelanin-coated iron oxide magnetic nanoparticles: A promising candidate for negative T2 contrast enhancement in magnetic resonance imaging. Chem Commun (Camb). 2015;51(56):11194-11197. doi: 10.1039/C5CC02536B
- Tham M, Frischer JM, Weigand SD, et al. Iron heterogeneity in early active multiple sclerosis lesions. Ann Neurol. 2021;89(3):498-510. doi: 10.1002/ana.25974
- Butterworth RF. Metal toxicity, liver disease and neurodegeneration. Neurotox Res. 2010;18(1):100-105. doi: 10.1007/s12640-010-9185-z
- Kanda T, Nakai Y, Aoki S, et al. Contribution of metals to brain MR signal intensity: Review articles. Jpn J Radiol. 2016;34(4):258-266. doi: 10.1007/s11604-016-0532-8
- Chen P, Miah MR, Aschner M. Metals and neurodegeneration. F1000Research. 2016;5:366. doi: 10.12688/f1000research.7431.1
- Dales JP, Desplat-Jégo S. Metal imbalance in neurodegenerative diseases with a specific concern to the brain of multiple sclerosis patients. Int J Mol Sci. 2020;21(23):9105. doi: 10.3390/ijms21239105