Enhancing spinal MRI segmentation with an asymmetric U-Net architecture
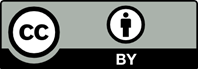
Spinal diseases are among the most prevalent health issues in modern society, significantly impacting patients’ quality of life. Diagnosing conditions such as disc herniation and spinal deformity requires advanced medical imaging techniques, including X-rays, magnetic resonance imaging (MRI), computed tomography, and nuclear magnetic resonance. Spine MRI is particularly crucial due to its ability to provide high-resolution images of soft tissues, essential for accurate diagnosis. However, the manual segmentation of spine MRI images is labor-intensive and inadequate for large-scale quantitative analysis. Thus, developing automated spinal MRI segmentation methods is critical to alleviating doctors’ workload and enhancing diagnostic efficiency. In this study, we propose a novel asymmetric U-Net architecture designed to improve the precision of reconstructing complex structures and details by increasing the depth of the upsampling side. The model incorporates adjacent-scale skip connections to control parameters while maintaining high segmentation accuracy. In addition, residual connections on the upsampling side prevent gradient vanishing, thereby enhancing the network’s feature learning and representation capabilities. Experimental results indicate that this method significantly reduces training time and increases model accuracy compared to traditional approaches, marking a substantial advancement in automated spinal MRI segmentation. This innovative approach holds promise for improving clinical outcomes and optimizing the workflow in medical imaging departments.
- Kubaszewski Ł, Wojdasiewicz P, Rożek M, et al. Syndromes with chronic non-bacterial osteomyelitis in the spine. Reumatologia. 2015;53(6):328-336. doi: 10.5114/reum.2015.57639
- Rajasekaran S, Bajaj N, Tubaki V, Kanna RM, Shetty AP. ISSLS prize winner: The anatomy of failure in lumbar disc herniation: An in vivo, multimodal, prospective study of 181 subjects. Spine (Phila Pa 1976). 2013;38(17):1491-1500. doi: 10.1055/s-0034-1376749
- Adams MA, Roughley PJ. What is intervertebral disc degeneration, and what causes it? Spine (Phila Pa 1976). 2006;31(18):2151-2161. doi: 10.1097/01.brs.0000231761.73859.2c
- Pedersen SJ, Maksymowych WP. The pathogenesis of ankylosing spondylitis: An update. Curr Rheumatol Rep. 2019;21(10):58. doi: 10.1007/s11926-019-0856-3
- Aouad K, Maksymowych WP, Baraliakos X, Ziade N. Update of imaging in the diagnosis and management of axial spondyloarthritis. Best Pract Res Clin Rheumatol. 2020;34(6):101628. doi: 10.1016/j.berh.2020.101628
- Hassan I, Wietfeldt ED. Presacral tumors: Diagnosis and management. Clin Colon Rectal Surg. 2009;22(2):84-93. doi: 10.1055/s-0029-1223839
- Hashimoto K, Nishimura S, Miyamoto H, Toriumi K, Ikeda T, Akagi M. Comprehensive treatment outcomes of giant cell tumor of the spine: A retrospective study. Medicine (Baltimore). 2022;101(32):e29963. doi: 10.1097/MD.0000000000029963
- Kalra MK, Maher MM, Toth TL, et al. Strategies for CT radiation dose optimization. Radiology. 2004;230(3):619-628. doi: 10.1148/radiol.2303021726
- Winn A, Martin A, Castellon I, et al. Spine MRI: A review of commonly encountered emergent conditions. Top Magn Reson Imaging. 2020;29(6):291-320. doi: 10.1097/RMR.0000000000000261
- Castaldo G, Lembo F, Tomaiuolo R. Molecular diagnostics: Between chips and customized medicine. Clin Chem Labo Med. 2010;48(7):973-982. doi: 10.1515/CCLM.2010.182
- Arslantaş A, Dalbayrak S, Şimşek S, et al. Minimally Invasive Spine Surgery Current Aspects. Turkey: Ali Arslantaş. 2016.
- Haldeman S, Kopansky-Giles D, Hurwitz EL, et al. Advancements in the management of spine disorders. Best Pract Res Clin Rheumatol. 2012;26(2):263-280. doi: 10.1016/j.berh.2012.03.006
- Azimi P, Yazdanian T, Benzel EC, et al. A review on the use of artificial intelligence in spinal diseases. Asian Spine J. 2020;14(4):543. doi: 10.31616/asj.2020.0147
- Da Costa RV, Moore SA. Differential diagnosis of spinal diseases. Vet Clin North Am Small Anim Pract. 2010;40(5):755-763. doi: 10.1007/978-981-16-9759-3_11
- Cohen-Adad J, Alonso-Ortiz E, Abramovic M, et al. Generic acquisition protocol for quantitative MRI of the spinal cord. Nat Protocols. 2021;16(10):4611-4632.
- Sollmann N, Löffler MT, Kronthaler S, et al. MRI‐based quantitative osteoporosis imaging at the spine and femur. J Magn Reson Imaging. 2021;54(1):12-35. doi: 10.1002/jmri.27260
- Willemink MJ, Koszek WA, Hardell C, et al. Preparing medical imaging data for machine learning. Radiology. 2020;295(1):4-15. doi: 10.1148/radiol.2020192224
- Patel V. A framework for secure and decentralized sharing of medical imaging data via blockchain consensus. Health Informatics J. 2019;25(4):1398-1411. doi: 10.1177/1460458218769699
- Senthilkumaran N, Vaithegi S. Image segmentation by using thresholding techniques for medical images. Comput Sci Eng Int J. 2016;6(1):1-13.
- Song Y, Ma B, Gao W, Fan S. Medical image edge detection based on improved differential evolution algorithm and prewitt operator. Acta Microscopica. 2019;28(1).
- Zhao F, Zhang J, Ma Y. Medical image processing based on mathematical morphology. In: Proceedings of the 2012 International Conference on Computer Application and System Modeling (ICCASM 2012); 2012. p. 948-950. doi: 10.2991/iccasm.2012.241
- Yamanakkanavar N, Choi JY, Lee B. MRI segmentation and classification of human brain using deep learning for diagnosis of Alzheimer’s disease: A survey. Sensors (Basel). 2020;20(11):3243. doi: 10.3390/s20113243
- Li H, Luo H, Huan W, et al. Automatic lumbar spinal MRI image segmentation with a multi-scale attention network. Neural Comput Appl. 2021;33:11589-11602. doi: 10.1007/s00521-021-05856-4
- Ronneberger O, Fischer P, Brox T. U-net: Convolutional Networks for Biomedical Image Segmentation. Berlin: Springer; 2015. p. 234-241. doi: 10.1007/978-3-319-24574-4_28
- Hempe H, Yilmaz EB, Meyer C, Heinrich MP. Opportunistic CT screening for degenerative deformities and osteoporotic fractures with 3D DeepLab. In: Medical Imaging 2022: Image Processing. Bellingham, DC: SPIE. p. 127-134. doi: 10.1117/12.2612848
- Miao S, Piat S, Fischer P, et al. Dilated FCN for Multi-agent 2D/3D Medical Image Registration. In: Proceedings of the AAAI Conference on Artificial Intelligence. doi: 10.1609/aaai.v32i1.11576
- Huang H, Lin L, Tong R, et al. Unet 3+: A full-Scale Connected UNet for Medical Image Segmentation. United States: IEEE. p. 1055-1059. doi: 10.1109/ICASSP40776.2020.9053405
- Pang S, Pang C, Zhao L, et al. SpineParseNet: spine parsing for volumetric MR image by a two-stage segmentation framework with semantic image representation. IEEE Trans Med Imaging. 2020;40(1):262-273. doi: 10.1109/TMI.2020.3025087
- Pang S, Pang C, Su Z, et al. “DGMSNet: Spine segmentation for MR image by a detection-guided mixed-supervised segmentation network. Med Image Anal. 2022;75:102261. doi: 10.1016/j.media.2021.102261
- He K, Zhang X, Ren S, Sun J. Deep residual learning for image recognition. In: Proceedings of the IEEE Conference on Computer Vision and Pattern Recognition; 2016. p. 770-778. doi: 10.1109/CVPR.2016.90