Discovering predictive features of multiple sclerosis from clinically isolated syndrome with machine learning
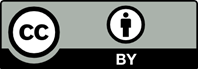
Accurately predicting the progression of clinically isolated syndrome (CIS) to multiple sclerosis (MS) is crucial for early intervention and management. This study employs a range of machine learning models, including categorical boosting, extreme gradient boosting, light gradient boosting machine, random forest, support vector machine, and logistic regression, to classify CIS patients based on their likelihood of developing MS. Our best model achieves and demonstrates superior predictive accuracy of 0.9312, measured using the area under the curve metric. In addition, we apply explainability techniques to determine the most influential features driving the predictions, identifying which CISs are most indicative of MS progression. Furthermore, we explore feature interactions to detect relationships between features, providing a deeper understanding of the underlying mechanisms. The study utilizes public data from 273 CISs patients, offering significant contributions to the clinical management and early diagnosis of MS.
- Compston A, Coles A. Multiple sclerosis. Lancet. 2008;372(9648):1502-1517. doi: 10.1016/S0140-6736(08)61620-7
- Lassmann H. Multiple sclerosis pathology. Cold Spring Harb Perspect Med. 2018;8(3):a028936. doi: 10.1101/cshperspect.a028936
- Multiple Sclerosis International Federation. Atlas of MS. 3rd ed.; 2020. Available from: https://www.msif.org/wp-content/ uploads/2020/10/atlas-3rd-edition-epidemiology-report-en-updated-30-9-20.pdf [Last accessed on 2024 Jul 11].
- Tozlu C, Olafson E, Jamison KW, et al. The sequence of regional structural disconnectivity due to multiple sclerosis lesions. Brain Commun. 2023;5(6):fcad332. doi: 10.1093/braincomms/fcad332
- Solomon AJ, Arrambide G, Brownlee WJ, et al. Differential diagnosis of suspected multiple sclerosis: An updated consensus approach. Lancet Neurol. 2023;22(8):750-768. doi: 10.1016/S1474-4422(23)00148-5
- Dickie DA, Shenkin SD, Anblagan D, et al. Whole brain magnetic resonance image atlases: A systematic review of existing atlases and caveats for use in population imaging. Front Neuroinform. 2017;11:1. doi: 10.3389/fninf.2017.00001
- Wattjes MP, Ciccarelli O, Reich DS, et al. 2021 MAGNIMS-CMSC-NAIMS consensus recommendations on the use of MRI in patients with multiple sclerosis. Lancet Neurol. 2021;20(8):653-670. doi: 10.1016/S1474-4422(21)00095-8
- Henriksson F, Fredrikson S, Masterman T, Jönsson B. Costs, quality of life and disease severity in multiple sclerosis: A cross‐sectional study in Sweden. Eur J Neurol. 2001;8(1):27-35. doi: 10.1046/j.1468-1331.2001.00169.x
- Paz-Zulueta M, Parás-Bravo P, Cantarero-Prieto D, Blázquez-Fernández C, Oterino-Durán A. A literature review of cost-of-illness studies on the economic burden of multiple sclerosis. Mult Scler Relat Disord. 2020;43:102162. doi: 10.1016/j.msard.2020.102162
- Kobelt G, Thompson A, Berg J, et al. New insights into the burden and costs of multiple sclerosis in Europe. Mult Scler. 2017;23(8):1123-1136. doi: 10.1177/1352458517694432
- Miller DH, Chard DT, Ciccarelli O. Clinically isolated syndromes. Lancet Neurol. 2012;11(2):157-169. doi: 10.1016/S1474-4422(11)70274-5
- Polman CH, Reingold SC, Banwell B, et al. Diagnostic criteria for multiple sclerosis: 2010 revisions to the Mcdonald criteria. Ann Neurol. 2011;69(2):292-302. doi: 10.1002/ana.22366
- Thompson AJ, Banwell BL, Barkhof F, et al. Diagnosis of multiple sclerosis: 2017 revisions of the McDonald criteria. Lancet Neurol. 2018;17(2):162-173. doi: 10.1016/S1474-4422(17)30470-2
- McDonald WI, Compston A, Edan G, et al. Recommended diagnostic criteria for multiple sclerosis: Guidelines from the international panel on the diagnosis of multiple sclerosis. Ann Neurol. 2001;50(1):121-127. doi: 10.1002/ana.1032
- Janelle M. Signs and Symptoms of Multiple Sclerosis (MS). Available from: https://www.health.com/multiple-sclerosis-symptoms-8653986 [Last accessed on 2024 Aug 05].
- Gómez-Melero S, Caballero-Villarraso J, Escribano BM, Galvao-Carmona A, Túnez I, Agüera-Morales E. Impact of cognitive impairment on quality of life in multiple sclerosis patients-a comprehensive review. J Clin Med. 2024;13(11):3321. doi: 10.3390/jcm13113321
- Luchetti S, Fransen NL, van Eden CG, Ramaglia V, Mason M, Huitinga I. Progressive multiple sclerosis patients show substantial lesion activity that correlates with clinical disease severity and sex: A retrospective autopsy cohort analysis. Acta Neuropathol. 2018;135(4):511-528. doi: 10.1007/s00401-018-1818-y
- Lublin FD, Reingold SC, Cohen JA, et al. Defining the clinical course of multiple sclerosis. Neurology. 2014;83(3):278-286. doi: 10.1212/WNL.0000000000000560
- Giovannoni G, Butzkueven H, Dhib-Jalbut S, et al. Brain health: Time matters in multiple sclerosis. Mult Scler Relat Disord. 2016;9 Suppl: S5-S48. doi: 10.1016/j.msard.2016.07.003
- Ro LS, Yang CC, Lyu RK, et al. A prospective, observational study on conversion of clinically isolated syndrome to multiple sclerosis during 4-year period (MS NEO study) in Taiwan. PLoS One. 2019;14(7):e0202453. doi: 10.1371/journal.pone.0202453
- Piri Cinar B, Ozakbas S. Prediction of conversion from clinically isolated syndrome to multiple sclerosis according to baseline characteristics: A prospective study. Noro Psikiyatr Ars. 2018;55:15-21. doi: 10.29399/npa.12667
- Shaheen HA, Sayed SS, Daker LI, Taha MA. Early predictors of conversion in patients with clinically isolated syndrome: A preliminary Egyptian study. Egypt J Neurol Psychiatr Neurosurg. 2018;54(1):21. doi: 10.1186/s41983-018-0021-3
- Bi CF, Qian HR, Peng LJ, et al. The correlation factor analysis for conversion of clinically isolated syndrome to multiple sclerosis and neuromyelitis optica. Zhonghua Nei Ke Za Zhi. 2016;55(6):460-465. doi: 10.3760/cma.j.issn.0578-1426.2016.06.012
- Kuhle J, Disanto G, Dobson R, et al. Conversion from clinically isolated syndrome to multiple sclerosis: A large multicentre study. Mult Scler. 2015;21(8):1013-1024. doi: 10.1177/1352458514568827
- CHAMPS Study Group. MRI predictors of early conversion to clinically definite MS in the CHAMPS placebo group. Neurology. 2002;59(7):998-1005. doi: 10.1212/WNL.59.7.998
- Alroughani R, Al Hashel J, Lamdhade S, Ahmed SF. Predictors of conversion to multiple sclerosis in patients with clinical isolated syndrome using the 2010 revised McDonald criteria. ISRN Neurol. 2012;2012:792192. doi: 10.5402/2012/792192
- Kolčava J, Kočica J, Hulová M, et al. Conversion of clinically isolated syndrome to multiple sclerosis: A prospective study. Mult Scler Relat Disord. 2020;44:102262. doi: 10.1016/j.msard.2020.102262
- Zhang H, Alberts E, Pongratz V, et al. Predicting conversion from clinically isolated syndrome to multiple sclerosis-an imaging-based machine learning approach. Neuroimage Clin. 2019;21:101593. doi: 10.1016/j.nicl.2018.11.003
- Bendfeldt K, Taschler B, Gaetano L, et al. MRI-based prediction of conversion from clinically isolated syndrome to clinically definite multiple sclerosis using SVM and lesion geometry. Brain Imaging Behav. 2019;13(5):1361-1374. doi: 10.1007/s11682-018-9942-9
- Yoo Y, Tang LYW, Li DKB, et al. Deep learning of brain lesion patterns and user-defined clinical and MRI features for predicting conversion to multiple sclerosis from clinically isolated syndrome. Comput Methods Biomech Biomed Eng Imaging Vis. 2019;7(3):250-259. doi: 10.1080/21681163.2017.1356750
- Banerjee T, Saha M, Ghosh E, et al. Conversion of clinically isolated syndrome to multiple sclerosis: A prospective multi-center study in Eastern India. Mult Scler J Exp Transl Clin. 2019;5(2):205521731984972. doi: 10.1177/2055217319849721
- Rommer PS, Milo R, Han MH, et al. Immunological aspects of approved MS therapeutics. Front Immunol. 2019;10:1564. doi: 10.3389/fimmu.2019.01564
- Pinto MF, Oliveira H, Batista S, et al. Prediction of disease progression and outcomes in multiple sclerosis with machine learning. Sci Rep. 2020;10(1):21038. doi: 10.1038/s41598-020-78212-6
- Zhao Y, Healy BC, Rotstein D, et al. Exploration of machine learning techniques in predicting multiple sclerosis disease course. PLoS One. 2017;12(4):e0174866. doi: 10.1371/journal.pone.0174866
- Ion-Mărgineanu A, Kocevar G, Stamile C, et al. Machine learning approach for classifying multiple sclerosis courses by combining clinical data with lesion loads and magnetic resonance metabolic features. Front Neurosci. 2017;11:398. doi: 10.3389/fnins.2017.00398
- Wottschel V, Alexander DC, Kwok PP, et al. Predicting outcome in clinically isolated syndrome using machine learning. Neuroimage Clin. 2015;7:281-287. doi: 10.1016/j.nicl.2014.11.021
- Jasperse B, Barkhof F. Machine Learning in Multiple Sclerosis. United States: Humana Press Inc.; 2023. p. 899-919. doi: 10.1007/978-1-0716-3195-9_28
- Branco D, di Martino B, Esposito A, Tedeschi G, Bonavita S, Lavorgna L. Machine learning techniques for prediction of multiple sclerosis progression. Soft Comput. 2022;26(22):12041-12055. doi: 10.1007/s00500-022-07503-z
- Haouam KD, Benmalek M. Machine learning algorithms for early prediction of multiple sclerosis progression: A comparative study. Adv Artif Intell Mach Learn. 2024;04(01):2027-2051. doi: 10.54364/AAIML.2024.41116
- Vázquez-Marrufo M, Sarrias-Arrabal E, García-Torres M, Martín-Clemente R, Izquierdo G. A systematic review of the application of machine-learning algorithms in multiple sclerosis. Neurología (Engl Ed). 2023;38(8):577-590. doi: 10.1016/j.nrleng.2020.10.013
- Naji Y, Mahdaoui M, Klevor R, Kissani N. Artificial intelligence and multiple sclerosis: Up-to-date review. Cureus. 2023;15:e45412. doi: 10.7759/cureus.45412
- Patel MA, Villalobos F, Shan K, et al. Generative artificial intelligence versus clinicians: Who diagnoses multiple sclerosis faster and with greater accuracy? Mult Scler Relat Disord. 2024;90:105791. doi: 10.1016/j.msard.2024.105791
- Rasouli S, Dakkali MS, Azarbad R, et al. Predicting the conversion from clinically isolated syndrome to multiple sclerosis: An explainable machine learning approach. Mult Scler Relat Disord. 2024;86:105614. doi: 10.1016/j.msard.2024.105614
- Lundberg SM, Lee SI. A unified approach to interpreting model predictions. Adv Neural Inf Process Syst. 2017;30:4765- 4774. doi: 10.48550/arXiv.1705.07874
- Prokhorenkova L, Gusev G, Vorobev A, Dorogush AV, Gulin A. CatBoost: Unbiased Boosting with Categorical Features. arXiv [Preprint]; 2018. doi: 10.48550/arXiv.1810.11363
- Chen T, Guestrin C. XGBoost. In: Proceedings of the 22nd ACM SIGKDD International Conference on Knowledge Discovery and Data Mining. ACM; 2016. p. 785-794. doi: 10.1145/2939672.2939785
- Machado MR, Karray S, de Sousa IT. LightGBM: An Effective Decision Tree Gradient Boosting Method to Predict Customer Loyalty in the Finance Industry. In: 2019 14th International Conference on Computer Science & Education (ICCSE). IEEE; 2019. p. 1111-1116. doi: 10.1109/ICCSE.2019.8845529
- Breiman L. Random forests. Mach Learn. 2001;45(1):5-32. doi: 10.1023/A:1010933404324
- Cortes C, Vapnik V. Support-vector networks. Mach Learn. 1995;20(3):273-297. doi: 10.1007/BF00994018
- Hosmer DW, Lemeshow S. Applied Logistic Regression. United States: Wiley; 2000. doi: 10.1002/0471722146
- Hastie T, Tibshirani R, Friedman J. The Elements of Statistical Learning. New York: Springer; 2009. doi: 10.1007/978-0-387-84858-7
- Chavarria V, Espinosa-Ramírez G, Sotelo J, et al. Conversion predictors of clinically isolated syndrome to multiple sclerosis in Mexican patients: A prospective study. Arch Med Res. 2023;54(5):102843. doi: 10.1016/j.arcmed.2023.102843
- Bradley AP. The use of the area under the ROC curve in the evaluation of machine learning algorithms. Pattern Recognit. 1997;30(7):1145-1159. doi: 10.1016/S0031-3203(96)00142-2
- Sokolova M, Lapalme G. A systematic analysis of performance measures for classification tasks. Inf Process Manag. 2009;45(4):427-437. doi: 10.1016/j.ipm.2009.03.002
- Shapiro SS, Wilk MB. An analysis of variance test for normality (Complete Samples). Biometrika. 1965;52(3/4):591. doi: 10.2307/2333709
- Friedman M. The use of ranks to avoid the assumption of normality implicit in the analysis of variance. J Am Stat Assoc. 1937;32(200):675-701. doi: 10.1080/01621459.1937.10503522
- Nemenyi P. Distribution-Free Multiple Comparisons. Princeton: Princeton University; 1963.
- Ali S, Akhlaq F, Imran AS, Kastrati Z, Daudpota SM, Moosa M. The enlightening role of explainable artificial intelligence in medical & healthcare domains: A systematic literature review. Comput Biol Med. 2023;166:107555. doi: 10.1016/j.compbiomed.2023.107555