Factors associated with social determinants of health mentions in PubMed clinical case reports from 1975 to 2022: A natural language processing analysis
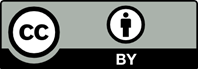
Social determinants of health (SDoH) significantly influence health outcomes, accounting for nearly 40% of such outcomes globally. These determinants, pivotal in understanding health disparities, are insufficiently documented in clinical settings and academic clinical narratives. To address this gap, we examined clinical case reports from PubMed (1975–2022) to identify mentions of six specific SDoH, employing a pre-trained named-entity recognition (NER) model from Spark natural language processing (NLP). Multivariate logistic regression was utilized to investigate associations between article characteristics and the documentation of SDoH. From 463,546 reports, 4.4% mentioned SDoH, with race/ethnicity being the most dominant mention. Race/ethnicity was often cited by sub-Saharan African authors (adjusted odds ratio [AOR]: 4.47) and in general medicine (AOR: 2.18). Marital status mentions appeared predominantly in psychiatry (AOR: 2.60) and gynecology (AOR: 2.47). Sexual orientation mentions were correlated with infectious diseases (AOR: 25.00) and varied by authorship regions, with stronger associations observed in South America (AOR: 4.04) and North America (AOR: 2.15), and comparatively weaker associations noted in the Indian subcontinent and the Middle East (AOR: 0.16). Immigrant status mentions were closely related to infectious diseases (AOR: 4.51), gynecology (AOR: 4.25), and certain geographies. Homelessness mentions were more prominent in forensic medicine (AOR: 14.92) and in both infections (AOR: 6.36) and mental disorders (AOR: 5.80). Spiritual belief mentions were more prominent with sub-Saharan authors (AOR: 9.17) and psychiatry (AOR: 7.61). SDoH mentions in medical literature were also determined by the diagnosis, cultural background, and journal type. The limited SDoH registration emphasized their overlooked significance. Disproportionate emphasis on specific relationships, such as sexual orientation with infectious diseases, can perpetuate biases and stereotypes. Innovative tools such as Spark NLP offer promise in advancing research using electronic health records (EHRs), but a standardized approach to SDoH reporting and vigilant AI training is crucial for unbiased health-care analysis.
- McGinnis JM, Williams-Russo P, Knickman JR. The case for more active policy attention to health promotion. Health Aff (Millwood). 2002;21:78-93. doi: 10.1377/hlthaff.21.2.78
- Galea S, Tracy M, Hoggatt KJ, DiMaggio C, Karpati A. Estimated deaths attributable to social factors in the United States. Am J Public Health. 2011;101:1456-1465. doi: 10.2105/AJPH.2010.300086
- Hatef E, Kharrazi H, Nelson K, et al. The association between neighborhood socioeconomic and housing characteristics with hospitalization: Results of a national study of Veterans. J Am Board Fam Med. 2019;32:890-903. doi: 10.3122/jabfm.2019.06.190138
- Hood CM, Gennuso KP, Swain GR, Catlin BB. County health rankings: Relationships between determinant factors and health outcomes. Am J Prev Med. 2016;50:129-135. doi: 10.1016/j.amepre.2015.08.024
- Walker RJ, Strom Williams J, Egede LE. Influence of race, ethnicity and social determinants of health on diabetes outcomes. Am J Med Sci. 2016;351:366-373. doi: 10.1016/j.amjms.2016.01.008
- Teshale AB, Htun HL, Owen A, et al. The role of social determinants of health in cardiovascular diseases: An umbrella review. J Am Heart Assoc. 2023;12:e029765. doi: 10.1161/JAHA.123.029765
- Enard KR, Coleman AM, Aver Yakubu R, Butcher BC, Tao D, Hauptman PJ. Influence of social determinants of health on heart failure outcomes: A systematic review. J Am Heart Assoc. 2023;12:e026590. doi: 10.1161/JAHA.122.026590
- Ludwig J, Sanbonmatsu L, Gennetian L, et al. Neighborhoods, obesity, and diabetes - a randomized social experiment. N Engl J Med. 2011;365:1509-1519. doi: 10.1056/NEJMsa1103216
- Wang M, Pantell MS, Gottlieb LM, Adler-Milstein J. Documentation and review of social determinants of health data in the EHR: Measures and associated insights. J Am Med Inform Assoc. 2021;28:2608-2616. doi: 10.1093/jamia/ocab194
- Daniel H, Bornstein SS, Kane GC, et al. Addressing social determinants to improve patient care and promote health equity: An American college of physicians position paper. Ann Intern Med. 2018;168:577-578. doi: 10.7326/M17-2441
- Handerer F, Kinderman P, Tai S. The need for improved coding to document the social determinants of health. Lancet Psychiatry. 2021;8:653. doi: 10.1016/S2215-0366(21)00208-X
- Cottrell EK, Dambrun K, Cowburn S, et al. Variation in electronic health record documentation of social determinants of health across a national network of community health centers. Am J Prev Med. 2019;57:S65-S73. doi: 10.1016/j.amepre.2019.07.014
- Guo Y, Chen Z, Xu K, et al. International Classification of Diseases, Tenth Revision, clinical modification social determinants of health codes are poorly used in electronic health records. Medicine (Baltimore). 2020;99:e23818. doi: 10.1097/MD.0000000000023818
- Truong HP, Luke AA, Hammond G, Wadhera RK, Reidhead M, Joynt Maddox KE. Utilization of social determinants of health ICD-10 Z-codes among hospitalized patients in the United States, 2016-2017. Med Care. 2020;58:1037-1043. doi: 10.1097/MLR.0000000000001418
- Hatef E, Rouhizadeh M, Tia I, et al. Assessing the availability of data on social and behavioral determinants in structured and unstructured electronic health records: A retrospective analysis of a multilevel health care system. JMIR Med Inform. 2019;7:e13802. doi: 10.2196/13802
- Guevara M, Chen S, Thomas S, et al. Large language models to identify social determinants of health in electronic health records. NPJ Digit Med. 2024;7:6. doi: 10.1038/s41746-023-00970-0
- Jiménez Carrillo M, Fernández Rodker J, Sastre Paz M, Alberquilla Menendez-Asenjo Á. Does the electronic health record reflect the social determinants of health from primary health care? Aten Primaria. 2021;53:36-42. doi: 10.1016/j.aprim.2020.01.007
- Gold R, Bunce R, Cowburn S, et al. Adoption of social determinants of health EHR tools by community health centers. Ann Fam Med. 2018;16:399-407. doi: 10.1370/afm.2275
- Tamang S, Humbert-Droz M, Gianfrancesco M, Izadi Z, Schmajuk G, Yazdany J. Practical considerations for developing clinical natural language processing systems for population health management and measurement. JMIR Med Inform. 2023;11:e37805. doi: 10.2196/37805
- Elbattah M, Arnaud É, Gignon M, Dequen G. The Role of Text Analytics in Healthcare: A Review of Recent Developments and Applications: In: Proceedings of the 14th International Joint Conference on Biomedical Engineering Systems and Technologies SCITEPRESS - Science and Technology Publications, Vienna, Austria; 2021. p. 825-832. doi: 10.5220/0010414508250832
- Devlin J, Chang MW, Lee K, Toutanova K. BERT: Pretraining of Deep Bidirectional Transformers for Language Understanding. In: Proceedings of the 2019 Conference of the North. Vol. 1; 2019. p. 4171-4186. doi: 10.18653/v1/n19-1423
- Lee J, Yoon W, Kim S, et al. BioBERT: A pre-trained biomedical language representation model for biomedical text mining. Bioinformatics. 2020;36:1234-1240. doi: 10.1093/bioinformatics/btz682
- Haq HU, Kocaman V, Talby D. Mining adverse drug reactions from unstructured mediums at scale. In: Shaban- Nejad A, Michalowski M, Bianco S, editors. Multimodal AI in Healthcare. Studies in Computational Intelligence. Vol. 1060. Cham: Springer; 2023. doi: 10.1007/978-3-031-14771-5_26
- Kocaman V, Talby D. Accurate clinical and biomedical named entity recognition at scale. Softw Impacts. 2022;13:100373. doi: 10.1016/j.simpa.2022.100373
- Kocaman V, Talby D. Spark NLP: Natural language understanding at scale. Softw Impacts. 2021;8:100058. doi: 10.1016/j.simpa.2021.100058
- Zhu Y, Yuan H, Wang S, et al. Large language models for information retrieval: A survey; 2023. doi: 10.48550/ARXIV.2308.07107
- Raza S, Dolatabadi E, Ondrusek N, Rosella L, Schwartz B. Discovering social determinants of health from case reports using natural language processing: Algorithmic development and validation. BMC Digit Health. 2023;1:35. doi: 10.1186/s44247-023-00035-y
- Hazlehurst B, Naleway A, Mullooly J. Detecting possible vaccine adverse events in clinical notes of the electronic medical record. Vaccine. 2009;27:2077-2083. doi: 10.1016/j.vaccine.2009.01.105
- Banerji A, Lai KH, Li Y, et al. Natural language processing combined with ICD-9-CM codes as a novel method to study the epidemiology of allergic drug reactions. J Allergy Clin Immunol Pract. 2020;8:1032-1038.e1. doi: 10.1016/j.jaip.2019.12.007
- Detect Sentences in Healthcare Texts. Detect Sentences in Healthcare Texts. John Snow Labs Inc. Available from: https:// nlp.johnsnowlabs.com/2021/08/11/sentence_detector_dl_ healthcare_en.html [Last accessed on 2024 Apr 06].
- Social Determinants of Health. Social Determinants of Health. John Snow Labs Inc.; 2023. Available from: https:// nlp.johnsnowlabs.com/2023/06/13/ner_sdoh_en.html [Last accessed on 2024 Apr 06].
- Kocaman V, Talby D. Biomedical named entity recognition at scale. In: Del Bimbo A, Farinella GM, Escalante HJ, et al, editors. Pattern Recognition. ICPR International Workshops and Challenges. Vol. 12661. Cham: Springer International Publishing; 2021. p. 635-646. doi: 10.1007/978-3-030-68763-2_48
- Brown T, Mann B, Ryder N, et al. Language models are few-shot learners. In: Advances in Neural Information Processing Systems. Curran Associates Inc.: United States; 2020: 1877-1901. doi: 10.48550/ARXIV.2005.14165
- OpenAI. GPT-4 Technical Report; 2023. doi: 10.48550/ARXIV.2303.08774
- Heinze G, Wallisch C, Dunkler D. Variable selection - A review and recommendations for the practicing statistician. Biom J. 2018;60:431-449. doi: 10.1002/bimj.201700067
- Tsai J. Lifetime and 1-year prevalence of homelessness in the US population: Results from the national epidemiologic survey on alcohol and related conditions-III. J Public Health Oxf Engl. 2018;40:65-74. doi: 10.1093/pubmed/fdx034
- Lybarger K, Dobbins NJ, Long R, et al. Leveraging natural language processing to augment structured social determinants of health data in the electronic health record. J Am Med Inform Assoc. 2023;30: 1389-1397. doi: 10.1093/jamia/ocad073
- Stewart De Ramirez S, Shallat J, McClure K, Foulger R, Barenblat L. Screening for social determinants of health: Active and passive information retrieval methods. Popul Health Manag. 2022;25:781-788. doi: 10.1089/pop.2022.0228