Benchmarking machine learning missing data imputation methods in large-scale mental health survey databases
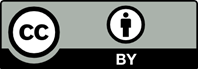
Databases tied to mental and behavioral health surveys suffer from the issue of missing data when participants skip the entire survey, which affects the data quality and sample size. These missing data patterns were investigated and the imputation performance was evaluated in Simons Foundations Powering Autism Research for Knowledge, a large-scale autism cohort consists of over 117,000 participants. Four common methods were assessed – Multiple imputation by chained equations (MICE), K-nearest neighbors (KNN), MissForest, and multiple imputation with denoising autoencoders (MIDAS). In a complete subset of 15,196 autism participants, three types of missingness patterns were simulated. We observed that MIDAS and KNN performed the best as the random missingness rate increased and when blockwise missingness was simulated. The average computational times were each 10 min for MIDAS and KNN, 35 min for MissForest, and 290 min for MICE. MIDAS and KNN both provide promising imputation performance in mental and behavioral health survey data that exhibit blockwise missingness patterns.
- Feliciano P, Daniels AM, Snyder LG, et al. SPARK: A US cohort of 50,000 families to accelerate autism research. Neuron. 2018;97:488-493. doi: 10.1016/j.neuron.2018.01.015
- Davis KAS, Coleman JRI, Adams M, et al. Mental health in UK Biobank - development, implementation and results from an online questionnaire completed by 157 366 participants: A reanalysis. BJPsych Open. 2020;6:e18. doi: 10.1192/bjo.2019.100
- Ramirez AH, Sulieman L, Schlueter DJ, et al. The all of Us research program: Data quality, utility, and diversity. Patterns (N Y). 2022;3:100570. doi: 10.1016/j.patter.2022.100570
- Chesnut SR, Wei T, Barnard-Brak L, Richman DM. A meta-analysis of the social communication questionnaire: Screening for autism spectrum disorder. Autism. 2017;21:920-928. doi: 10.1177/1362361316660065
- Hooker JL, Dow D, Morgan L, Schatschneider C, Wetherby AM. Psychometric analysis of the repetitive behavior scale-revised using confirmatory factor analysis in children with autism. Autism Res. 2019;12:1399-1410. doi: 10.1002/aur.2159
- Van Damme T, Vancampfort D, Thoen A, Sanchez CPR, van Biesen D. Evaluation of the Developmental Coordination Questionnaire (DCDQ) as a screening instrument for co-occurring motor problems in children with autism spectrum disorder. J Autism Dev Disord. 2022;52:4079-4088. doi: 10.1007/s10803-021-05285-1
- Jebb AT, Ng V, Tay L. A review of key likert scale development advances: 1995-2019. Front Psychol. 2021;12:637547. doi: 10.3389/fpsyg.2021.637547
- Mirzaei A, Carter SR, Patanwala AE, Schneider CR. Missing data in surveys: Key concepts, approaches, and applications. Res Soc Adm Pharm. 2022;18:2308-2316. doi: 10.1016/j.sapharm.2021.03.009
- Mack C, Su Z, Westreich D. Managing Missing Data in Patient Registries: Addendum to Registries for Evaluating Patient Outcomes: A User’s Guide, Third Edition. Rockville, MD: Agency for Healthcare Research and Quality (US); 2018.
- Khan SI, Hoque ASM. SICE: An improved missing data imputation technique. J Big Data. 2020;7:37. doi: 10.1186/s40537-020-00313-w
- Phiwhorm K, Saikaew C, Leung CK, Polpinit P, Saikaew KR. Adaptive multiple imputations of missing values using the class center. J Big Data. 2022;9:52. doi: 10.1186/s40537-022-00608-0
- De Goeij MCM, van Diepen M, Jager KJ, Tripepi G, Zoccali C, Dekker FW. Multiple imputation: Dealing with missing data. Nephrol Dial Transplant. 2013;28:2415-2420. doi: 10.1093/ndt/gft221
- Van Buuren S, Groothuis-Oudshoorn K. Mice: Multivariate imputation by chained equations in R. J Stat Softw. 2011;45:1-67. doi: 10.18637/jss.v045.i03
- Azur MJ, Stuart EA, Frangakis C, Leaf PJ. Multiple imputation by chained equations: What is it and how does it work? Int J Methods Psychiatr Res. 2011;20:40-49. doi: 10.1002/mpr.329
- Taunk K, De S, Verma S, Swetapadma A. A Brief Review of Nearest Neighbor Algorithm for Learning and Classification. In: 2019 International Conference on Intelligent Computing and Control Systems (ICCS). IEE; 2019.
- Stekhoven DJ, Bühlmann P. MissForest--non-parametric missing value imputation for mixed-type data. Bioinformatics. 2011;28:112-118. doi: 10.1093/bioinformatics/btr597
- Lall R, Robinson T. The MIDAS touch: Accurate and scalable missing-data imputation with deep learning. Polit Anal. 2022;30:179-196. doi: 10.1017/pan.2020.49
- Shrive FM, Stuart H, Quan H, Ghali WA. Dealing with missing data in a multi-question depression scale: A comparison of imputation methods. BMC Med Res Methodol. 2006;6:57. doi: 10.1186/1471-2288-6-57
- Peyre H, Leplège A, Coste J. Missing data methods for dealing with missing items in quality of life questionnaires. A comparison by simulation of personal mean score, full information maximum likelihood, multiple imputation, and hot deck techniques applied to the SF-36 in the French 2003 decennial health survey. Qual Life Res. 2011;20:287-300. doi: 10.1007/s11136-010-9740-3
- Emmanuel T, Maupong T, Mpoeleng D, Semong T, Mphago B, Tabona O. A survey on missing data in machine learning. J Big Data. 2021;8:140. doi: 10.1186/s40537-021-00516-9
- Xu X, Xia L, Zhang Q, Wu S, Wu M, Liu H. The ability of different imputation methods for missing values in mental measurement questionnaires. BMC Med Res Methodol. 2020;20:42. doi: 10.1186/s12874-020-00932-0
- Croy CD, Novins DK. Methods for addressing missing data in psychiatric and developmental research. J Am Acad Child Adolesc Psychiatry. 2005;44:1230-1240. doi: 10.1097/01.chi.0000181044.06337.6f
- Lee JH, Huber JC Jr. Evaluation of multiple imputation with large proportions of missing data: How much is too much? Iran J Public Health. 2021;50:1372-1380. doi: 10.18502/ijph.v50i7.6626
- Petrazzini BO, Naya H, Lopez-Bello F, Vazquez G, Spangenberg L. Evaluation of different approaches for missing data imputation on features associated to genomic data. BioData Mining. 2021;14:44. doi: 10.1186/s13040-021-00274-7
- Pedregosa F, Varoquaux G, Gramfort A, et al. Scikit-learn: Machine learning in Python. J Mach Learn Res. 2011;12:2825-2830.
- Lall R, Robinson T. Efficient multiple imputation for diverse data in python and R: MIDASpy and rMIDAS. J Stat Softw. 2023;107:1-38. doi: 10.18637/jss.v107.i09
- Fawns-Ritchie C, Deary IJ. Reliability and validity of the UK Biobank cognitive tests. PLoS One. 2020;15:e0231627. doi: 10.1371/journal.pone.0231627
- Schweren LJS, van Rooij D, Shi H, et al. Diet, physical activity, and disinhibition in middle-aged and older adults: A UK biobank study. Nutrients. 2021;13:1607. doi: 10.3390/nu13051607
- Grau E, Frechtel P, Odom D, Painter D. A Simple Evaluation of the Imputation Procedures Used in NSDUH. In: Proceedings of the 2004 Joint Statistical Meetings, American Statistical Association, Section on Survey Research Methods, Toronto, Ontario, Canada [CD-ROM]. Alexandria, VA: American Statistical; 2004.
- An U, Pazokitoroudi A, Alvarez M, et al. Deep learning-based phenotype imputation on population-scale biobank data increases genetic discoveries. Nat Genet. 2023;55:2269-2276. doi: 10.1038/s41588-023-01558-w