Systemic drug repurposing for pancreatic cancer based on genetic and epigenetic network analysis using a systems biology approach and deep neural learning of drug-target interactions
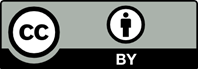
Pancreatic cancer is a malignant tumor associated with a high mortality rate. This research presents a systems biology approach to explore the mechanisms of pancreatic ductal adenocarcinoma (PDAC), aiming to identify significant biomarkers that can serve as drug targets. We propose a systematic drug repurposing strategy that incorporates a deep neural network (DNN)-based drug-target interaction (DTI) model along with drug design specifications to develop a potential multi-molecule drug for PDAC treatment. We first established candidate protein-protein interaction networks and gene regulatory networks using big data mining techniques. Real PDAC and non-PDAC genome-wide genetic and epigenetic networks (GWGENs) were systematically identified using their corresponding microarray data through system identification and system order detection methods. The top 6,000 core GWGENs of PDAC and non-PDAC were extracted using the Principal Network Projection method. Subsequently, we annotated the core GWGENs using the Kyoto Encyclopedia of Genes and Genomes pathways to construct their respective core signaling pathways. By comparing upstream microenvironmental factors, core signaling pathways, and downstream aberrant cellular functions between PDAC and non-PDAC, we investigated the carcinogenic mechanisms of PDAC. Notably, c-MYC, forkhead box O3, and tumor suppressor p53 were identified as significant biomarkers for potential drug targets. Furthermore, the DNN-based DTI model predicted the interaction probabilities between candidate molecular drugs and these biomarkers. Based on drug design specifications such as regulatory ability, sensitivity, and toxicity, suitable multi-molecular potential drugs were selected. Ultimately, gemcitabine and MK-2206 were identified as a promising multi-molecular drug combination for PDAC treatment.
- Ma Y, Wu Q, Li X, Gu X, Xu J, Yang J. Pancreatic cancer: From bench to bedside. Ann Transl Med. 2016;4(23):458. doi: 10.21037/atm.2016.11.57
- Rahib L, Smith BD, Aizenberg R, Rosenzweig AB, Fleshman JM, Matrisian LM. Projecting cancer incidence and deaths to 2030: The unexpected burden of thyroid, liver, and pancreas cancers in the United States. Cancer Res. 2014;74(11):2913-2921. doi: 10.1158/0008-5472.Can-14-0155
- Rahib L, Wehner MR, Matrisian LM, Nead KT. estimated projection of US cancer incidence and death to 2040. JAMA Netw Open. 2021;4(4):e214708. doi: 10.1001/jamanetworkopen.2021.4708
- Strobel O, Lorenz P, Hinz U, et al. Actual five-year survival after upfront resection for pancreatic ductal adenocarcinoma: Who beats the odds? Ann Surg. 2022;275(5):962-971. doi: 10.1097/sla.0000000000004147
- Sung H, Ferlay J, Siegel RL, et al. Global cancer statistics 2020: GLOBOCAN estimates of incidence and mortality worldwide for 36 cancers in 185 countries. CA Cancer J Clin. 2021;71(3):209-249. doi: 10.3322/caac.21660
- Lynch SM, Vrieling A, Lubin JH, et al. Cigarette smoking and pancreatic cancer: A pooled analysis from the pancreatic cancer cohort consortium. Am J Epidemiol. 2009;170(4):403-413. doi: 10.1093/aje/kwp134
- Mela A, Rdzanek E, Tysarowski A, et al. The impact of changing the funding model for genetic diagnostics and improved access to personalized medicine in oncology. Expert Rev Pharmacoecon Outcomes Res. 2023;23(1):43-54. doi: 10.1080/14737167.2023.2140139
- Park W, Chawla A, O’Reilly EM. Pancreatic cancer: A review. JAMA. 2021;326(9):851-862. doi: 10.1001/jama.2021.13027
- Elsayed M, Abdelrahim M. The latest advancement in pancreatic ductal adenocarcinoma therapy: A review article for the latest guidelines and novel therapies. Biomedicines. 2021;9(4):389. doi: 10.3390/biomedicines9040389
- Zeng S, Pöttler M, Lan B, Grützmann R, Pilarsky C, Yang H. Chemoresistance in pancreatic cancer. Int J Mol Sci. 2019;20(18):4504. doi: 10.3390/ijms20184504
- Hughes J, Rees S, Kalindjian S, Philpott K. Principles of early drug discovery. Br J Pharmacol. 2011;162(6):1239-1249. doi: 10.1111/j.1476-5381.2010.01127.x
- Weaver MF, Hopper JA, Gunderson EW. Designer drugs 2015: Assessment and management. Addict Sci Clin Pract. 2015;10(1):8. doi: 10.1186/s13722-015-0024-7
- Zhan YP, Chen BS. Drug target identification and drug repurposing in psoriasis through systems biology approach, DNN-based DTI model and genome-wide microarray data. Int J Mol Sci. 2023;24(12):10033. doi: 10.3390/ijms241210033
- Wang CT, Chen BS. Drug discovery for periodontitis treatment based on big data mining, systems biology, and deep learning methods. SynBio. 2023;1(1):116-143. doi: 10.3390/synbio1010009
- Hsu BW, Chen BS. Genetic and epigenetic host-virus network to investigate pathogenesis and identify biomarkers for drug repurposing of human respiratory syncytial virus via real-world two-side RNA-Seq data: Systems biology and deep-learning approach. Biomedicines. 2023;11(6):1531. doi: 10.3390/biomedicines11061531
- Lin YC, Chen BS. Identifying drug targets of oral squamous cell carcinoma through a systems biology method and genome-wide microarray data for drug discovery by deep learning and drug design specifications. Int J Mol Sci. 2022;23(18):10409. doi: 10.3390/ijms231810409
- Kulkarni VS, Alagarsamy V, Solomon VR, Jose PA, Murugesan S. Drug repurposing: An effective tool in modern drug discovery. Russ J Bioorg Chem. 2023;49(2):157-166. doi: 10.1134/s1068162023020139
- Salwinski L, Miller CS, Smith AJ, Pettit FK, Bowie JU, Eisenberg D. The database of interacting proteins: 2004 update. Nucleic Acids Res. 2004;32(suppl_1):D449-D451. doi: 10.1093/nar/gkh086
- Orchard S, Ammari M, Aranda B, et al. The MIntAct project--IntAct as a common curation platform for 11 molecular interaction databases. Nucleic Acids Res. 2013;42(D1):D358-D363. doi: 10.1093/nar/gkt1115
- Stark C, Breitkreutz BJ, Reguly T, Boucher L, Breitkreutz A, Tyers M. BioGRID: A general repository for interaction datasets. Nucleic Acids Res. 2006;34(suppl_1):D535-D539. doi: 10.1093/nar/gkj109
- Zanzoni A, Montecchi-Palazzi L, Quondam M, Ausiello G, Helmer-Citterich M, Cesareni G. MINT: A molecular INTeraction database. FEBS Lett. 2002;513(1):135-140. doi: 10.1016/S0014-5793(01)03293-8
- Bovolenta LA, Acencio ML, Lemke N. HTRIdb: An open-access database for experimentally verified human transcriptional regulation interactions. BMC Genomics. 2012;13(1):405. doi: 10.1186/1471-2164-13-405
- Zheng G, Tu K, Yang Q, et al. ITFP: An integrated platform of mammalian transcription factors. Bioinformatics. 2008;24(20):2416-2417. doi: 10.1093/bioinformatics/btn439
- Wingender E, Chen X, Hehl R, et al. TRANSFAC: An integrated system for gene expression regulation. Nucleic Acids Res. 2000;28(1):316-319. doi: 10.1093/nar/28.1.316
- Friard O, Re A, Taverna D, De Bortoli M, Corá D. CircuitsDB: A database of mixed microRNA/transcription factor feed-forward regulatory circuits in human and mouse. BMC Bioinformatics. 2010;11(1):435. doi: 10.1186/1471-2105-11-435
- Agarwal V, Bell GW, Nam JW, Bartel DP. Predicting effective microRNA target sites in mammalian mRNAs. Elife. 2015;4:e05005. doi: 10.7554/eLife.05005
- Li JH, Liu S, Zhou H, Qu LH, Yang JH. StarBase v2.0: Decoding miRNA-ceRNA, miRNA-ncRNA and protein- RNA interaction networks from large-scale CLIP-Seq data. Nucleic Acids Res. 2013;42(D1):D92-D97. doi: 10.1093/nar/gkt1248
- Chen BS, Wu CC. Systems biology as an integrated platform for bioinformatics, systems synthetic biology, and systems metabolic engineering. Cells. 2013;2(4):635-688. doi: 10.3390/cells2040635
- Sakamoto Y, Ishiguro M, Kitagawa G. Akaike information criterion statistics. Dordrecht Netherlands D Reidel. 1986;81(10.5555):26853.
- Chang S, Wang LHC, Chen BS. Investigating core signaling pathways of hepatitis b virus pathogenesis for biomarkers identification and drug discovery via systems biology and deep learning method. Biomedicines. 2020;8(9):320. doi: 10.3390/biomedicines8090320
- Kanehisa M, Goto S. KEGG: Kyoto encyclopedia of genes and genomes. Nucleic Acids Res. 2000;28(1):27-30. doi: 10.1093/nar/28.1.27
- Wang Y, Zhang S, Li F, et al. Therapeutic target database 2020: Enriched resource for facilitating research and early development of targeted therapeutics. Nucleic Acids Res. 2019;48(D1):D1031-D1041. doi: 10.1093/nar/gkz981
- Consortium TU. UniProt: A hub for protein information. Nucleic Acids Res. 2014;43(D1):D204-D212. doi: 10.1093/nar/gku989
- Knox C, Law V, Jewison T, et al. DrugBank 3.0: A comprehensive resource for ‘Omics’ research on drugs. Nucleic Acids Res. 2010;39(suppl_1):D1035-D1041. doi: 10.1093/nar/gkq1126
- Kim S, Thiessen PA, Bolton EE, et al. PubChem substance and compound databases. Nucleic Acids Res. 2015;44(D1):D1202-D1213. doi: 10.1093/nar/gkv951
- Gaulton A, Hersey A, Nowotka M, et al. The ChEMBL database in 2017. Nucleic Acids Res. 2016;45(D1):D945-D954. doi: 10.1093/nar/gkw1074
- Kuhn M, von Mering C, Campillos M, Jensen LJ, Bork P. STITCH: Interaction networks of chemicals and proteins. Nucleic Acids Res. 2007;36(suppl_1):D684-D688. doi: 10.1093/nar/gkm795
- Ringnér M. What is principal component analysis? Nat Biotechnol. 2008;26(3):303-304. doi: 10.1038/nbt0308-303
- Huber M, Brehm CU, Gress TM, et al. The immune microenvironment in pancreatic cancer. Int J Mol Sci. 2020;21(19):7307. doi: 10.3390/ijms21197307
- Truong LH, Pauklin S. Pancreatic cancer microenvironment and cellular composition: Current understandings and therapeutic approaches. Cancers (Basel). 2021;13(19):5028. doi: 10.3390/cancers13195028
- Cortesi M, Zanoni M, Pirini F, et al. Pancreatic cancer and cellular senescence: Tumor microenvironment under the spotlight. Int J Mol Sci. 2022;23(1):254. doi: 10.3390/ijms23010254
- Arneth B. Tumor microenvironment. Medicina (Kaunas). 2020;56(1):15. doi: 10.3390/medicina56010015
- Stopa KB, Kusiak AA, Szopa MD, Ferdek PE, Jakubowska MA. Pancreatic cancer and its microenvironment-recent advances and current controversies. Int J Mol Sci. 2020;21(9):3218. doi: 10.3390/ijms21093218
- Javadrashid D, Baghbanzadeh A, Derakhshani A, et al. Pancreatic cancer signaling pathways, genetic alterations, and tumor microenvironment: The barriers affecting the method of treatment. Biomedicines. 2021;9(4):373. doi: 10.3390/biomedicines9040373
- Ma Y, Shen N, Wicha MS, Luo M. The roles of the let-7 family of MicroRNAs in the regulation of cancer stemness. Cells. 2021;10(9):2415. doi: 10.3390/cells10092415
- Hessmann E, Schneider G, Ellenrieder V, Siveke JT. MYC in pancreatic cancer: Novel mechanistic insights and their translation into therapeutic strategies. Oncogene. 2016;35(13):1609-1618. doi: 10.1038/onc.2015.216
- Wolfer A, Ramaswamy S. MYC and metastasis. Cancer Res. 2011;71(6):2034-2037. doi: 10.1158/0008-5472.Can-10-3776
- Liu YH, Gan YX, Chen JZ, Jiang YX, Huang F, Tang D. Targeting MYC to combat pancreatic cancer. J Explor Rese Pharmacol. 2022;7(3):164-178. doi: 10.14218/jerp.2021.00015
- Wirth M, Mahboobi S, Krämer OH, Schneider G. Concepts to target MYC in pancreatic cancer. Mol Cancer Ther. 2016;15(8):1792-1798. doi: 10.1158/1535-7163.Mct-16-0050
- Ala M. Target c-Myc to treat pancreatic cancer. Cancer Biol Ther. 2022;23(1):34-50. doi: 10.1080/15384047.2021.2017223
- Farrell AS, Joly MM, Allen-Petersen BL, et al. MYC regulates ductal-neuroendocrine lineage plasticity in pancreatic ductal adenocarcinoma associated with poor outcome and chemoresistance. Nature Commun. 2017;8(1):1728. doi: 10.1038/s41467-017-01967-6
- Fujiwara-Tani R, Sasaki T, Bhawal UK, et al. Nuclear MAST4 suppresses FOXO3 through interaction with AKT3 and induces chemoresistance in pancreatic ductal carcinoma. Int J Mol Sci. 2024;25(7):4056. doi: 10.3390/ijms25074056
- Luo X, Yang Z, Liu X, et al. The clinicopathological significance of forkhead box P1 and forkhead box O3a in pancreatic ductal adenocarcinomas. Tumor Biol. 2017;39(5):1010428317699129. doi: 10.1177/1010428317699129
- Feng S, Jiang ZJ, Yu D, Li J, Liu G, Sun JJ. FOXO3a expression and its diagnostic value in pancreatic ductal adenocarcinoma. Int J Clin Exp Pathol. 2018;11(11):5422-5429.
- Yu S, Yu Y, Sun Y, et al. Activation of FOXO3a suggests good prognosis of patients with radically resected gastric cancer. Int J Clin Exp Pathol. 2015;8(3):2963-2970.
- McCubrey JA, Yang LV, Abrams SL, et al. Effects of TP53 mutations and mirs on immune responses in the tumor microenvironment important in pancreatic cancer progression. Cells. 2022;11(14):2155. doi: 10.3390/cells11142155
- Stefanoudakis D, Frountzas M, Schizas D, Michalopoulos NV, Drakaki A, Toutouzas KG. Significance of TP53, CDKN2A, SMAD4 and KRAS in pancreatic cancer. Curr Issues Mol Biol. 2024;46(4):2827-2844. doi: 10.3390/cimb46040177
- Subramanian A, Narayan R, Corsello SM, et al. A next generation connectivity map: L1000 platform and the first 1,000,000 profiles. Cell. 2017;171(6):1437-1452.e17. doi: 10.1016/j.cell.2017.10.049
- Corsello SM, Nagari RT, Spangler RD, et al. Discovering the anti-cancer potential of non-oncology drugs by systematic viability profiling. Nat Cancer. 2020;1(2):235-248. doi: 10.1038/s43018-019-0018-6
- Xiong G, Wu Z, Yi J, et al. ADMETlab 2.0: An integrated online platform for accurate and comprehensive predictions of ADMET properties. Nucleic Acids Res. 2021;49(W1):W5-W14. doi: 10.1093/nar/gkab255
- Amrutkar M, Gladhaug IP. Pancreatic cancer chemoresistance to gemcitabine. Cancers (Basel). 2017;9(11):157. doi: 10.3390/cancers9110157
- Adamska A, Domenichini A, Falasca M. Pancreatic ductal adenocarcinoma: Current and evolving therapies. Int J Mol Sci. 2017;18(7):1338. doi: 10.3390/ijms18071338
- Coppola A, Farolfi T, La Vaccara V, et al. Neoadjuvant treatments for pancreatic ductal adenocarcinoma: Where we are and where we are going. J Clin Med. 2023;12(11):3677. doi: 10.3390/jcm12113677
- Kielb SJ, Shah NL, Rubin MA, Sanda MG. Functional p53 mutation as a molecular determinant of paclitaxel and gemcitabine susceptibility in human bladder cancer. J Urol. 2001;166(2):482-487.
- Hill R, Rabb M, Madureira PA, et al. Gemcitabine-mediated tumour regression and p53-dependent gene expression: Implications for colon and pancreatic cancer therapy. Cell Death Dis. 2013;4(9):e791. doi: 10.1038/cddis.2013.307
- Achanta G, Pelicano H, Feng L, Plunkett W, Huang P. Interaction of p53 and DNA-PK in response to nucleoside analogues: Potential role as a sensor complex for DNA damage. Cancer Res. 2001;61(24):8723-8729.
- Cao P, Zhang W, Qiu J, Tang Z, Xue X, Feng T. Gemcitabine inhibits the progression of pancreatic cancer by restraining the WTAP/MYC chain in an m6A-dependent manner. Cancer Res Treat. 2024;56(1):259-271. doi: 10.4143/crt.2022.1600
- Yao J, Huang M, Shen Q, et al. c-Myc-PD-L1 axis sustained gemcitabine-resistance in pancreatic cancer. Front Pharmacol. 2022;13:851512. doi: 10.3389/fphar.2022.851512
- Ebrahimi S, Hosseini M, Shahidsales S, et al. Targeting the Akt/PI3K signaling pathway as a potential therapeutic strategy for the treatment of pancreatic cancer. Curr Med Chem. 2017;24(13):1321-1331. doi: 10.2174/0929867324666170206142658
- Konopleva MY, Walter RB, Faderl SH, et al. Preclinical and early clinical evaluation of the oral AKT inhibitor, MK-2206, for the treatment of acute myelogenous leukemia. Clin Cancer Res. 2014;20(8):2226-2235. doi: 10.1158/1078-0432.Ccr-13-1978
- Simioni C, Martelli AM, Cani A, et al. The AKT inhibitor MK-2206 is cytotoxic in hepatocarcinoma cells displaying hyperphosphorylated AKT-1 and synergizes with conventional chemotherapy. Oncotarget. 2013;4(9):1496-1506. doi: 10.18632/oncotarget.1236
- Hirai H, Sootome H, Nakatsuru Y, et al. MK-2206, an allosteric akt inhibitor, enhances antitumor efficacy by standard chemotherapeutic agents or molecular targeted drugs in vitro and in vivo. Mol Cancer Ther. 2010;9(7):1956-1967. doi: 10.1158/1535-7163.Mct-09-1012
- Yap TA, Yan L, Patnaik A, et al. First-in-man clinical trial of the oral pan-AKT inhibitor MK-2206 in patients with advanced solid tumors. J Clin Oncol. 2011;29(35):4688-4695. doi: 10.1200/jco.2011.35.5263
- Wang Z, Luo G, Qiu Z. Akt inhibitor MK-2206 reduces pancreatic cancer cell viability and increases the efficacy of gemcitabine. Oncol Lett. 2020;19(3):1999-2004. doi: 10.3892/ol.2020.11300
- Elnaggar MS, Sminia P, Shehata S, Fedrigo C, Peters G. 1676P - gemcitabine, PI3kinase-Akt pathway inhibition and radiation in human glioma cell lines. Ann Oncol. 2012;23:ix537. doi: 10.1016/S0923-7534(20)34222-8