Innovative carbon emission monitoring in urban mobility: A case study of Beijing
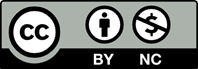
China’s national goals of peaking carbon emissions by 2030 and achieving carbon neutrality by 2060 necessitate a comprehensive transition of Chinese cities from high-carbon to low- and zero-carbon development. The transportation sector, a crucial area for energy conservation and emission reduction, faces significant challenges in achieving carbon neutrality due to rapid urbanization and motorization, which are projected to increase total emissions. Accurate monitoring of carbon emissions is vital for managing these emissions and achieving the “dual carbon” goals. This study provides a detailed analysis of Beijing’s integrated carbon emission monitoring system for urban mobility. It examines the innovative technical framework, which combines top-down and bottom-up approaches, enabling real-time and precise emissions tracking. The findings reveal that the system significantly improves data accuracy, supports effective governance strategies, and offers practical recommendations for scalability and adoption by other cities. In addition, the study evaluates the policy effectiveness of Beijing’s initiatives, providing evidence of substantial progress toward reducing carbon emissions. The research highlights the potential for replicability of Beijing’s system worldwide in transforming urban carbon governance. By addressing challenges such as data integration and resource limitations, the study contributes a comprehensive framework that advances the field of urban mobility emissions monitoring. This approach highlights the necessity of digital transformation in achieving sustainable urban development while setting a benchmark for other cities aiming to align with global climate goals.
Athanasopoulou, A., Deijkers, T., Ozkan, B., & Turetken, O. (2022). MaaS platform features: An exploration of their relationship and importance from supply and demand perspective. Journal of Urban Mobility, 2:100028. https://doi.org/10.1016/j.urb.2022.100028
Ang, L. M., & Seng, K. P. (2016). Big sensor data applications in urban environments. Big Data Research, 4, 1-12. https://doi.org/10.1016/j.bdr.2015.12.003
Bibri, S. E., & Krogstie, J. (2020). Environmentally data-driven smart sustainable cities: Applied innovative solutions for energy efficiency, pollution reduction, and urban metabolism. Energy Informatics, 3(1):59. https://doi.org/10.1186/s42162-020-00130-8
Borja, A., Berg, T., Gundersen, H., Hagen, A.G., Hancke, K., Korpinen, S., et al. (2024). Innovative and practical tools for monitoring and assessing biodiversity status and impacts of multiple human pressures in marine systems. Environmental Monitoring and Assessment, 196(8):694. https://doi.org/10.1007/s10661-024-12861-2
Borken-Kleefeld, J. (2020). Distance-based emission factors from vehicle emission remote sensing measurements. Science of the Total Environment, 739:139688. https://doi.org/10.1016/j.scitotenv.2020.139688
Cai, T., & Hong, Z. (2024). Exploring the structure of the digital economy through block chain technology and mitigating adverse environmental effects with the aid of artificial neural networks. Frontiers in Environmental Science, 12:1315812. https://doi.org/10.3389/fenvs.2024.1315812
Cecchel, S., Chindamo, D., Turrini, E., Carnevale, C., Cornacchia, G., Gadola, M., et al. (2018). Impact of reduced mass of light commercial vehicles on fuel consumption, CO2 emissions, air quality, and socio-economic costs. Science of the Total Environment, 613-614:409-417. https://doi.org/10.1016/j.scitotenv.2017.09.081
Cifuentes, F., González, C. M., Trejos, E. M., López, L. D., Sandoval, F. J., Cuellar, O. A., et al (2021). Comparison of top-down and bottom-up road transport emissions through high-resolution air quality modeling in a city of complex orography. Atmosphere, 12(11):1372. https://doi.org/10.3390/atmos12111372
Chao, M., Maimai, W., Hanzhang, L., Zhibo, C., & Xiaohui, C. (2023). A spatio-temporal neural network learning system for city-scale carbon storage capacity estimating. IEEE Access, 11:31304-31322. https://doi.org/10.1109/ACCESS.2023.3260641
Chen, H., Wang, R., Liu, X., Du, Y., & Yang, Y. (2023). Monitoring the enterprise carbon emissions using electricity big data: A case study of Beijing. Journal of Cleaner Production, 396:136427. https://doi.org/10.1016/j.jclepro.2023.136427
Erie, M. S., & Streinz, T. (2021). The Beijing effect: China’s digital silk road as transnational data governance. NYU Journal of International Law and Politics, 54:1.
Ezenkwu, C. P., Cannon, S., & Ibeke, E. (2024). Monitoring carbon emissions using deep learning and statistical process control: A strategy for impact assessment of governments’ carbon reduction policies. Environ Monit Assess, 196(3):231.
Feng, Q., Zhen, K., Lu, Y., Yang, X., Yang, Y., Liu, B., et al. (2021). Analysis on emission characteristics of urban buses based on remote online monitoring. SAE Technical Paper, 3:24-38. https://doi.org/10.4271/2021-01-0601
Flyvbjerg, B. (2006). Five misunderstandings about case-study research. Qualitative inquiry, 12(2):219-245. https://doi.org/10.1177/1077800405284363
Jiang, H., Han, Y., Zalhaf, A. S., Yang, P., & Wang, C. (2023). Low-cost urban carbon monitoring network and implications for china: A comprehensive review. Environ Sci Pollut Res Int, 30(48):105012-105029. https://doi.org/10.1007/s11356-023-29836-4
Hanelt, A., Bohnsack, R., Marz, D., & Antunes Marante, C. (2021). A systematic review of the literature on digital transformation: Insights and implications for strategy and organizational change. Journal of Management Studies, 58(5):1159-1197. https://doi.org/10.1111/joms.12639
He, S., Shin, H., Xu, S., & Tsourdos, A. (2020). Distributed estimation over a low-cost sensor network: A review of state-of-the-art. Information Fusion, 54:21-43. https://doi.org/10.1016/j.inffus.2019.06.026
Huang, Y., Mok, W. C., Yam, Y. S., Zhou, J. L., Surawski, N. C., Organ, B., et al. (2020). Evaluating in-use vehicle emissions using air quality monitoring stations and on-road remote sensing systems. Science of the Total Environment, 740:139868. https://doi.org/10.1016/j.scitotenv.2020.139868
Jaramillo, P., Kahn Ribeiro, S., Newman, P., Dhar, S., Diemuodeke, O.E., et al. (2022). Transport. Ch. 10. United Kingdom: Cambridge University Press.
Khan, K., Su, C., Tao, R., & Hao, L. (2020). Urbanization and carbon emission: Causality evidence from the new industrialized economies. Environment, Development and Sustainability, 22(8):7193-7213. https://doi.org/10.1007/s10668-019-00479-1
Li, G. H., Gao, J., Deng, S. X., Sun, Z. G., Zhang, S., Lu, Z. Z., et al. (2022). Research on the high - resolution greenhouse gas emission inventory and characteristics of road mobile sources in Weinan City[J]. Acta Scientiae Circumstantiae, 42(12):332-340.
Li, Y., & Siong, Y. (2021). Study on the synergetic emission reduction effect of CO2 and air pollutants from the mobile source of urban roads in Beijing under the target of carbon neutralization. Chinese Journal of Environmental Management, 13(3):113-120.
Liao, K., & Liu, J. (2024). Digital infrastructure empowerment and urban carbon emissions: Evidence from China. Telecommunications Policy, 48(6):102764. https://doi.org/10.1016/j.telpol.2024.102764
Liu, J., Li, J., Chen, Y., Lian, S., Zeng, J., Geng, M., et al. (2023). Multi-scale urban passenger transportation CO2 emission calculation platform for smart mobility management. Applied Energy, 331:120407. https://doi.org/10.1016/j.apenergy.2022.120407
Liu, Z., Ma, L., Huang, X., & Li, T. (2019). The impact of local government policy on innovation ecosystem in knowledge resource scarce regions: Case study of Changzhou, China. Journal of Innovation and Entrepreneurship, 8(4):75-98. https://doi.org/10.1177/0971721818806096
Middela, M. S., Mahesh, S., McNabola, A., Smith, W., Timoney, D., Ekhtiari, A., et al. (2024). Evaluating real-world emissions from in-use buses and taxis using on-road remote sensing. Environmental Pollution, 344:123241. https://doi.org/10.1016/j.envpol.2023.123241
Mitropoulos, L., Kortsari, A., Mizaras, V., & Ayfantopoulou, G. (2023). Mobility as a service (MaaS) planning and implementation: Challenges and lessons learned. Future Transp, 3(2):498-518. https://doi.org/10.3390/futuretransp3020029
Musa, A. A., Malami, S. I., Alanazi, F., Ounaies, W., Alshammari, M., & Haruna, S. I. (2023). Sustainable traffic management for smart cities using Internet-of-Things-oriented intelligent transportation systems (ITS): Challenges and Recommendations. Sustainability, 15(2):9859. https://doi.org/10.3390/su15139859
Pan, J., & Zhang, Y. (2021). Spatiotemporal patterns of energy carbon footprint and decoupling effect in China. Acta Geographica Sinica, 76(1):206-222. https://doi.org/10.11821/dlxb202101016
Pla, M. A. M., Lorenzo-Sáez, S., Luzuriaga, J. E., Prats, S. M., Moreno-Pérez, J. A., Urchueguía, J. F., et al. (2021). From traffic data to GHG emissions: A novel bottom-up methodology and its application to Valencia city. Sustainable Cities and Society, 66:102643. https://doi.org/10.1016/j.scs.2020.102643
Qu, X. M., Qiu, J. D., Lyu, K. C., & Luo, S. L. (2023). Urban road traffic mobile source emission accounting and diffusion methods. Urban Traffic, (6):87–94. https://doi.org/10.13813/j.cn11-5141/u.2023.0606
Raghavan, S. S., & Tal, G. (2021). Behavioral and technology implications of electromobility on household travel emissions. Transportation Research Part D: Transport and Environment, 94:102792. https://doi.org/10.1016/j.trd.2021.102792
Rane, J., Kaya, O., Mallick, S. K., & Rane, N. L. (2024). Influence of digitalization on business and management: A review on artificial intelligence, blockchain, big data analytics, cloud computing, and internet of things. In: Generative Artificial Intelligence in Agriculture, Education, and Business. United Kingdom: Deep Science Publishing, p. 1-26. https://doi.org/10.70593/978-81-981271-7-4_1
Ropkins, K., Beebe, J., Li, H., Daham, B., Tate, J., Bell, M., et al. (2009). Real-world vehicle exhaust emissions monitoring: Review and critical discussion. Environmental Science and Technology, 43(19):79-152. https://doi.org/10.1080/10643380701413377
Salas, V. R., Etuman, A. E., & Coll, I. (2024). Exploring the linkages between urban form, mobility and emissions with OLYMPUS: A comparative analysis in two French regions. Science of the Total Environment, 919:170710. https://doi.org/10.1016/j.scitotenv.2024.170710
Wang, Q., Li, S., & Pisarenko, Z. (2020). Modeling carbon emissions trajectory of China, US, and India. Journal of Cleaner Production, 258:120723. https://doi.org/10.1016/j.jclepro.2020.120723
Wang, Z., Bai, B., Wang, Y., Zhang, Y., Li, S., & Shan, B. (2023). Research paradigm for high-precision, large-scale wind energy potential: An example of a geographically-constrained multi-criteria decision analysis model. Journal of Cleaner Production, 429:39614. https://doi.org/10.1016/j.jclepro.2023.139614
Wu, J., Jia, P., Feng, T., Li, H., Kuang, H., & Zhang, J. (2023). Uncovering the spatiotemporal impacts of built environment on traffic carbon emissions using multi-source big data. Land Use Policy, 129:106621. https://doi.org/10.1016/j.landusepol.2023.106621
Xi, H., Li, M., Hensher, D. A., Xie, C., Gu, Z., & Zheng, Y. (2024). Strategizing sustainability and profitability in electric mobility-as-a-Service (E-MaaS) ecosystems with carbon incentives: A multi-leader multi-follower game. Transportation Research Part C: Emerging Technologies, 166:104758. https://doi.org/10.1016/j.trc.2024.104758
Xing, F., He, S., Sun, M., & Chen, J. (2022). Carbon emission monitoring based on internet of things with cloud-tube edge-end structure. Chinese Journal on Internet of Things, 6(4):53-64. https://doi.org/10.11959/j.issn.2096-3750.2022.00281
Xiong, T., Liu, Y., Yang, C., Cheng, Q., & Lin, S. (2023). Research overview of urban carbon emission measurement and future prospects for GHG monitoring networks. Energy Reports, 9:231-242. https://doi.org/10.1016/j.egyr.2023.04.047
Yang, F., Yang, X., & Li, X. (2024). China’s diverse energy transition pathways toward carbon neutrality by 2060. Sustainable Production and Consumption, 47:236-250. https://doi.org/10.1016/j.spc.2024.04.007
Yin, R. K. (2017). Case study research and applications: Design and methods. Washington DC: Sage Publications.
Zampou, E., Mourtos, I., Pramatari, K., & Seidel, S. (2022). A design theory for energy and carbon management systems in the supply chain. Journal of the Association for Information Systems, 23(1):329-371. https://doi.org/10.17705/1jais.00725
Zhang C., Liao T., Sun Y., et al. (2021). Preliminary study on the method of analyzing the contribution of urbanlocal emission and regional transmission based on space-dense sensor. Acta Scientiae Circumstantiae, 41(9):3683-3695.
Zhang, H., Zhang, J., Wang, R., Huang, Y., Zhang, M., Shang, X., & Gao, C. (2021). Smart carbon monitoring platform under IoT-Cloud architecture for small cities in B5G. Wireless Networks, 27:2459-2472.
Zhao, X., Papaix, C., & Zhou, Y. (2023). Urban mobility and mobility-as-a-service (MaaS) trends. In: Routledge Handbook of Trends and Issues in Tourism Sustainability, Planning and Development, Management, and Technology. United Kingdom: Taylor and Francis, p. 363-382. https://doi.org/10.4324/9781003291763-35
Zhao, X., Zhang, Z., Guo, W., Zhou, Y., Papaix, C., & Sun, Q. (2022). Evidence-based smart transition strategies for long-distance commuters in Beijing. Frontiers in Future Transportation, 3:884949 https://doi.org/10.3389/ffutr.2022.884949
Zheng, Y., Du, S., Zhang, X., Bai, L., & Wang, H. (2022). Estimating carbon emissions in urban functional zones using multi-source data: A case study in Beijing. Building and Environment, 212:108804. https://doi.org/10.1016/j.buildenv.2022.108804
Zhong, H., Chen, K., Liu, C., Zhu, M., & Ke, R. (2024). Models for predicting vehicle emissions: A comprehensive review. Science of the Total Environment, 923:171324. https://doi.org/10.1016/j.scitotenv.2024.171324