Development of a machine-simulated human scoring model for assessing child-friendly street environments: A case study of Sham Shui Po, Hong Kong SAR, China
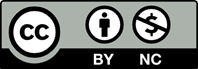
With a growing interest in liveable cities, scholars and urban planners are increasingly studying the characteristics of child-friendly cities, including the ability to walk and move freely in public spaces. While machine learning techniques and street view imagery analysis have enabled the systematic analysis of streets, they have not yet been applied to assess street environments from a child’s perspective. This study explores the use of deep learning models to address this gap by developing a machine-simulated human scoring model to assess health and safety indicators in urban streets. Using a high-density, old urban district in Hong Kong SAR, China, as a case, the study used semantic segmentation to analyze street environmental features and extract elements related to safety, such as greenery, vehicles, and fences. Subsequently, the model generated safety ratings, which were compared with scores provided by volunteer caregivers. The results indicate that natural elements and fences enhance safety, whereas an excess of buildings diminishes it. In contrast to European cities, where high visibility and larger sky proportions are considered beneficial for health, these factors were less relevant in the high-density, tropical context of Hong Kong. This analysis highlights the robustness and efficiency of the model, which can assist researchers in other cities in collecting empirical user rating data and informing strategies for more child-friendly urban planning.
Anguelov, D., Dulong, C., Filip, D., Frueh, C., Lafon, S., Lyon, R., et al. (2010). Google street view: Capturing the world at street level. Computer, 43(6):32-38. https://doi.org/10.1109/MC.2010.170
Badrinarayanan, V., Kendall, A., & Cipolla, R. (2017). SegNet: A deep convolutional encoder-decoder architecture for image segmentation. IEEE Transactions on Pattern Analysis and Machine Intelligence, 39(12):2481-2495. https://doi.org/10.1109/TPAMI.2016.2644615
Bain, L., Gray, B., & Rodgers, D. (2012). Living Streets: Strategies for Crafting Public Space. United States: John Wiley & Sons.
Berk, L. (2015). Child Development. United Kingdom: Pearson Higher Education AU.
Bertolini, L. (2020). From “streets for traffic” to “streets for people”: Can street experiments transform urban mobility? Transport Reviews, 40(6):734-753. https://doi.org/10.1080/01441647.2020.1761907
Bornstein, M. H. (1985). On the development of color naming in young children: Data and theory. Brain and Language, 26(1):72-93. https://doi.org/10.1016/0093-934x(85)90029-x
Brown, C., de Lannoy, A., McCracken, D., Gill, T., Grant, M., Wright, H., et al. (2019). Special issue: Child-friendly cities. Cities & Health, 3(1-2):1-7. https://doi.org/10.1080/23748834.2019.1682836
Burden, D., Davis, D., Sprowls, S., Zykofsky, P., & Wallwork, M. (1999). Street Design Guidelines for Healthy Neighborhoods. Sacramento, CA, USA: Center for Livable Communities, p. 1-15.
Cheng, C. (2013). Sham Shui Po: The centre of poverty in Hong Kong. Journal of the Royal Asiatic Society Hong Kong Branch, 53:7-30.
Cheng, C., Qian, G., & Nie, R. (2024). Research on the evaluation index system of child-friendly street. Modern Transportation Technology, 21(1):80-84.
Ding, X., Ao, Y., & Bahmani, H. (2023). Child friendliness of rural school travel road: Improvement strategies based on rural children’s perception. Journal of Transport & Health, 32:101674. https://doi.org/10.1016/j.jth.2023.101674
Dubey, A., Naik, N., Parikh, D., Raskar, R., & Hidalgo, C. A. (2016). Deep Learning the City: Quantifying Urban Perception at a Global Scale. In: Proceedings of the European Conference on Computer Vision (ECCV). Cham: Springer, pp. 196-212. https://doi.org/10.48550/arXiv.1608.01769
Ewing, R., & Handy, S. (2009). Measuring the unmeasurable: Urban design qualities related to walkability. Journal of Urban Design, 14(1):65-84. https://doi.org/10.1080/13574800802451155
Fischler, M. A., & Bolles, R. C. (1981). Random sample consensus: A paradigm for model fitting with applications to image analysis and automated cartography. Communications of the ACM, 24(6):381-395. https://doi.org/10.1145/358669.358692
Global Designing Cities Initiative. (2019). Designing Streets for Kids. New York: Global Designing Cities Initiative.
Greene, M. R., & Oliva, A. (2009). Recognition of natural scenes from global properties: Seeing the forest without representing the trees. Cognitive Psychology, 58(2):137-176. https://doi.org/10.1016/j.cogpsych.2008.06.001
Han, X., Wang, L., Seo, S. H., He, J., & Jung, T. (2022). Measuring perceived psychological stress in urban built environments using google street view and deep learning. Frontiers in Public Health, 10:891736. https://doi.org/10.3389/FPUBH.2022.891736/BIBTEX
Harirchian, M., Esmaeili, M., & Kermanshahi, S. (2018). A New Perspective on Urban Street Design. In: The Transp Res Boa (TRB) 97th Annual Meeting.
He, N., & Li, G. (2021). Urban neighbourhood environment assessment based on street view image processing: A review of research trends. Environmental Challenges, 4:100090. https://doi.org/10.1016/j.envc.2021.100090
Hong Kong Census and Statistics Department. (2016). Hong Kong Poverty Situation Report on Ethnic Minorities. Available from: https://www.statistics.gov.hk/pub/ b9xx0004e2016xxxxe0100.pdf [Last accessed: 2024 Oct 31].
Hou, Y., Zhai, C., Chen, X., & Li, W. (2022). The effect of the street environment on two types of essential physical activity in industrial neighborhoods from the perspective of public health: A study from the Harbin low-income population health survey, China. BMC Public Health, 22(1):2201. https://doi.org/10.1186/s12889-022-14533-7
Hui, T. W. (2015). High Density Development and Spatiality of Sham Shui Po, Hong Kong: A Lefebvrian Approach. Master’s Thesis. Hong Kong Baptist University.
Jahromi, G. R. Z. (2020). Investigating and evaluation of the indicators affecting development of child-friendly cultural complexes with an approach to reconciliation with historical spaces (case study: Shiraz). Nexo, 33(2):707-724. https://doi.org/10.5377/nexo.v33i02.10803
Johansson, M. (2006). Environment and parental factors as determinants of mode for children’s leisure travel. Journal of environmental psychology, 26(2):156-169. https://doi.org/10.1016/j.jenvp.2006.05.005
Kim, J. H., Lee, S., Hipp, J. R., & Ki, D. (2021). Decoding urban landscapes: Google street view and measurement sensitivity. Computers, Environment and Urban Systems, 88:101626. https://doi.org/10.1016/j.compenvurbsys.2021.101626
Kingston, B., Wridt, P., Chawla, L., Van Vliet, W., & Brink, L. (2007). Creating child friendly cities: The case of denver, USA. ICE Proceedings Municipal Engineer, 160(2):97-102. https://doi.org/10.1680/muen.2007.160.2.97
Koo, B. W., Guhathakurta, S., & Botchwey, N. (2022). How are neighborhood and street-level walkability factors associated with walking behaviors? A big data approach using street view images. Environment and Behavior, 54(1):211-241. https://doi.org/10.1177/00139165211014609
Law, S., Seresinhe, C. I., Shen, Y., & Gutierrez-Roig, M. (2020). Street-Frontage-Net: Urban image classification using deep convolutional neural networks. International Journal of Geographical Information Science, 34(4):681-707. https://doi.org/10.1080/13658816.2018.1555832
Li, D. (2016). Access to Nature and Adolescents’ Psychological Well-being. United States: University of Illinois at Urbana- Champaign.
Li, X., Zhang, C., Li, W., Kuzovkina, Y. A., & Weiner, D. (2015). Who lives in greener neighborhoods? The distribution of street greenery and its association with residents’ socioeconomic conditions in Hartford, Connecticut, USA. Urban Forestry and Urban Greening, 14(4):751-759. https://doi.org/10.1016/J.UFUG.2015.07.006
Lowe, D. G. (2004). Distinctive image features from scale-invariant keypoints. International Journal of Computer Vision, 60:91-110. https://doi.org/10.1023/B: VISI.0000029664.99615.94
Lu, Y., Yang, Y., Sun, G., & Gou, Z. (2019). Associations between overhead-view and eye-level urban greenness and cycling behaviors. Cities, 88:10-18. https://doi.org/10.1016/j.cities.2019.01.003
Lynch, K. (1964). The Image of the City. Cambridge: M.I.T. Press.
McDonald, N. C. (2006). Exploratory analysis of children’s travel patterns. Transportation Research Record: Journal of the Transportation Research Board, 1977(1):1-7.
McMillan, T. E. (2005). Urban form and a child’s trip to school: The Current literature and a framework for future research. Journal of Planning Literature, 19(4):440-456. https://doi.org/10.1177/0885412204274173
Mehtap, O. (2016). Designing “Livable Children Spaces” in Urban Space Child-Friendly Streets and Turkey Example. In: 13th International Conference “Standardization, Protypes and Quality: A Means of Balkan Countries Collaboration”. Brasov, Romania.
Montello, D. R., Goodchild, M. F., Gottsegen, J., & Fohl, P. (2017). Where’s downtown? Behavioral methods for determining referents of vague spatial queries. In: Spatial Vagueness, Uncertainty, Granularity. United Kingdom: Psychology Press, pp. 185-204.
Nagata, S., Nakaya, T., Hanibuchi, T., Amagasa, S., Kikuchi, H., & Inoue, S. (2020). Objective scoring of streetscape walkability related to leisure walking: Statistical modeling approach with semantic segmentation of Google Street View images. Health & Place, 66:102428. https://doi.org/10.1016/j.healthplace.2020.102428
Naik, N., Philipoom, J., Raskar, R., & Hidalgo, C.A. (2014). Streetscore -- Predicting the Perceived Safety of One Million Streetscapes. In: 2014 IEEE Conference on Computer Vision and Pattern Recognition Workshops. Columbus, OH, USA: IEEE, pp. 793-799. https://doi.org/10.1109/CVPRW.2014.121
Nasar, J. L. (1990). The evaluative image of the city. Journal of the American Planning Association, 56(1):41-53. https://doi.org/10.1080/01944369008975742
Nikku, B. R., & Pokhrel, R. (2013). Crafting child‐friendly cities: Evidence from Biratnagar sub-Metropolitan City, Eastern Nepal. Asian Social Work and Policy Review, 7(2):135-150. https://doi.org/10.1111/aswp.12013
Ordonez, V., & Berg, T. L. (2014). Learning High-level Judgments of Urban Perception. In: Computer Vision- ECCV 2014: 13th European Conference. Part. VI 13. Zurich, Switzerland: Springer International Publishing, pp. 494-510. https://doi.org/10.1007/978-3-319-10599-4_32
Peng, W. (2020). Child’s infrastructure in cities - urban planning and design to guarantee child rights. Landscape Architecture Frontiers, 8(2):100-109. https://doi.org/10.15302/J-LAF-1-030012
Porzi, L., Rota Bulò, S., Lepri, B., & Ricci, E. (2015). Predicting and Understanding Urban Perception with Convolutional Neural Networks. In: Proceedings of the 23rd ACM International Conference on Multimedia, pp. 139-148. https://doi.org/10.1145/2733373.2806273
Raimbault, M., Lavandier, C., & Bérengier, M. (2003). Ambient sound assessment of urban environments: Field studies in two French cities. Applied Acoustics, 64(12):1241-1256. https://doi.org/10.1016/S0003-682X(03)00061-6
Read, M. A., Sugawara, A. I., & Brandt, J. A. (1999). Impact of space and color in the physical environment on preschool children’s cooperative behavior. Environment and Behavior, 31(3):413-428. https://doi.org/10.1177/00139169921972173
Rini, H. S. (2019). Children in the city park: Rethinking public space accessibility in the Child-Friendly City of Semarang. In: Research for Social Justice. United Kingdom: Routledge, pp. 180-185.
Salesses, P., Schechtner, K., & Hidalgo, C.A. (2013) The collaborative image of the city: Mapping the inequality of urban perception. PLoS One, 8(7):e68400. https://doi.org/10.1371/journal.pone.0068400
Sanoff, H. (2016). Visual Research Methods in Design (Routledge Revivals). United Kingdom: Routledge.
Sapsağlam, Ö., & Eryılmaz, A. (2024). Building child-friendly cities for sustainable child development: Child-friendly city scale-child form. Sustainability, 16(3):1228. https://doi.org/10.3390/su16031228
Shaw, B., Bicket, M., Elliott, B., Fagan-Watson, B., Mocca, E., & Hillman, M. (2015). Children’s Independent Mobility: An International Comparison and Recommendations for Action. London: Policy Studies Institute.
Sholihah, A. B. S., & Heath, T. (2016). Traditional streetscape adaptability: Urban gentrification and endurance of business. Environment-Behaviour Proceedings Journal, 1(4):132-141. https://doi.org/10.21834/e-bpj.v1i4.378
Smith, L. E., Gosselin, V., Collins, P., & Frohlich, K. L. (2022). A tale of two cities: Unpacking the success and failure of school street interventions in two Canadian cities. International Journal of Environmental Research and Public Health, 19(18):11555. https://doi.org/10.3390/ijerph191811555
Song, Q., Dou, Z., Qiu, W., Li, W., Wang, J., van Ameijde, J., et al. (2023). The evaluation of urban spatial quality and utility trade-offs for Post-COVID working preferences: A case study of Hong Kong. Architectural Intelligence, 2(1):1. https://doi.org/10.1007/s44223-022-00020-x
The Official Website of the City of New York. (2010). Active Design Guidelines: Promoting Physical Activity and Health in Design. Available from: https://www.nyc.gov/assets/doh/ downloads/pdf/environmental/active-design-guidelines. pdf [Last accessed: 2024 Oct 31].
Torres, J. (2020). Why is it important to provide child-and youth-friendly streets? In: The Routledge Handbook of Designing Public Spaces for Young People. United Kingdom: Routledge, pp. 52-63.
Trichês Lucchesi, S., de Abreu e Silva, J., Margarita Larranaga,A., Zechin, D., & Beatriz Bettella Cybis, H. (2023). Machine learning and image recognition technologies to identify built environment barriers and incentives to walk. Transportation Research Record, 2677(1):14-24. https://doi.org/10.1177/03611981221097965
Ulrich, R. S. (1979). Visual landscapes and psychological well‐being. Landscape Research, 4(1):17-23. https://doi.org/10.1080/01426397908705892
UNICEF. (2023). Population Under Age 18. Available from: https://data.unicef.org/how-many/how-many-children-under-18-are-in-the-world [Last accessed: 2024 Oct 31].
Vidal, D. G., & Castro Seixas, E. (2022). Children’s green infrastructure: Children and their rights to nature and the city. Frontiers in Sociology, 7:804535. https://doi.org/10.3389/fsoc.2022.804535
Villanueva, K., Giles-Corti, B., Bulsara, M., Timperio, A., McCormack, G., Beesley, B., et al. (2013). Where do children travel to and what local opportunities are available? The relationship between neighborhood destinations and children’s independent mobility. Environment and Behavior, 45(6):679-705. https://doi.org/10.1177/0013916512440705
Wheeler, S. M., Abunnasr, Y., Dialesandro, J., Assaf, E., Agopian, S., & Gamberini, V. C. (2019). Mitigating urban heating in dryland cities: A literature review. Journal of planning literature, 34(4):434-446. https://doi.org/10.1177/0885412219855779
Yang, Y., He, D., Gou, Z., Wang, R., Liu, Y., & Lu, Y. (2019). Association between street greenery and walking behavior in older adults in Hong Kong. Sustainable Cities and Society, 51:101747. https://doi.org/10.1016/j.scs.2019.101747
Yao, Y., Liang, Z., Yuan, Z., Liu, P., Bie, Y., Zhang, J., et al. (2019). A human-machine adversarial scoring framework for urban perception assessment using street-view images. International Journal of Geographical Information Science, 33(12):2363-2384. https://doi.org/10.1080/13658816.2019.1643024
Ye, Y., Xie, H., Fang, J., Jiang, H., & Wang, D. (2019). Daily accessed street greenery and housing price: Measuring economic performance of human-scale streetscapes via new urban data. Sustainability, 11(6):1741. https://doi.org/10.3390/su11061741
Yuniastuti, E., & Hasibuan, H. S. (2019). Child-friendly Green Open Space to Enhance the Education Process for Children. In: IOP Conference Series: Earth and Environmental Science, 243(1):012161. https://doi.org/10.1088/1755-1315/243/1/012161
Zhang, F., Wu, L., Zhu, D., & Liu, Y. (2019). Social sensing from street-level imagery: A case study in learning spatio-temporal urban mobility patterns. ISPRS Journal of Photogrammetry and Remote Sensing, 153:48-58. https://doi.org/10.1016/j.isprsjprs.2019.04.017
Zhang, F., Zhou, B., Liu, L., Liu, Y., Fung, H. H., Lin, H., et al. (2018). Measuring human perceptions of a large-scale urban region using machine learning. Landscape and Urban Planning, 180:148-160. https://doi.org/10.1016/j.landurbplan.2018.08.020
Zhao, H., Shi, J., Qi, X., Wang, X., & Jia, J. (2017). Pyramid Scene Parsing Network. In: 2017 IEEE Conference on Computer Vision and Pattern Recognition (CVPR), pp. 6230-6239.