Medical imaging technology: Principles and systems
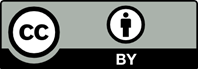
Medical imaging technology is an important course in biomedical engineering. It is a multidisciplinary field integrating advanced technologies from physics, electronic engineering, computer science, engineering mathematics, material science, and fine processing. This course lays the foundation for the implementation of imaging diagnostics essential for medical automation. It enables participants to systematically grasp the fundamental knowledge in medical imaging principles, equipment, and system analysis, as well as to understand the direction of the latest developments in this field. This paper discusses the basic principles and performance of various basic imaging devices, such as X-ray imaging, magnetic resonance imaging, nuclear medicine imaging, and ultrasound imaging. In addition, it explores the systems involved and the future prospects of medical imaging technology.
- Abhisheka B, Biswas SK, Purkayastha B, Das D, Escargueil A. Recent trend in medical imaging modalities and their applications in disease diagnosis: A review. Multimed Tools Appl. 2024;83(14):43035-43070. doi: 10.1007/s11042-023-17326-1
- Rix A, Lederle W, Theek B, et al. Advanced ultrasound technologies for diagnosis and therapy. J Nucl Med. 2018;59(5):740-746. doi: 10.2967/jnumed.117.200030
- Tubiana M. Wilhelm Conrad Röntgen and the discovery of X-rays. Bull Acad Natl Med. 1996;180(1):97-108.
- Withers PJ, Bouman C, Carmignato S, et al. X-ray computed tomography. Nat Rev Methods Prim. 2021;1(1):18. doi: 10.1038/s43586-021-00015-4
- Brenner DJ, Hall EJ. Computed Tomography--an increasing source of radiation exposure. New Engl J Med. 2007;357(22):2277-2284. doi: 10.1056/NEJMra072149
- Pullicino P, du Boulay GH, Kendall BE. Xenon enhancement for computed tomography of the spinal cord. Neuroradiology. 1979;18(2):63-66. doi: 10.1007/bf00344823
- Willemink MJ, Noël PB. The evolution of image reconstruction for CT-from filtered back projection to artificial intelligence. Eur Radiol. 2019;29(5):2185-2195. doi: 10.1007/s00330-018-5810-7
- Xian JF, Chen M, Jin ZY. Magnetic resonance imaging in clinical medicine: Current status and potential future developments in China. Chin Med J (Engl). 2015;128(5):569-570. doi: 10.4103/0366-6999.151637
- Moran CM, Thomson AJW. Preclinical ultrasound imaging-a review of techniques and imaging applications. Front Phys. 2020;8:124. doi: 10.3389/fphy.2020.00124
- Wang RF, Liu M. Study on neuroreceptor imaging with radionuclide tracing in vivo. Beijing Da Xue Xue Bao Yi Xue Ban = J Peking Univ Health Sci. 2007;39(5):550-554.
- Gremlich HU, Martínez V, Kneuer R, et al. Noninvasive assessment of gastric emptying by near-infrared fluorescence reflectance imaging in mice: Pharmacological validation with tegaserod, cisapride, and clonidine. Mol Imaging. 2004;3(4):303-311. doi: 10.1162/15353500200404127
- Kwee RM, Kwee TC. Whole-body MRI for preventive health screening: A systematic review of the literature. J Magn Reson Imaging. 2019;50(5):1489-1503. doi: 10.1002/jmri.26736
- Buxton RB. The physics of functional magnetic resonance imaging (fMRI). Rep Prog Phys. 2013;76(9):096601. doi: 10.1088/0034-4885/76/9/096601
- 1Hansen SB, Bender D. Advancement in production of radiotracers. Semin Nuclear Med. 2022;52(3):266-275. doi: 10.1053/j.semnuclmed.2021.10.003
- Sarıgül M, Ozyildirim BM, Avci M. Differential convolutional neural network. Neural Netw. 2019;116:279-287. doi: 10.1016/j.neunet.2019.04.025
- Lee JG, Jun S, Cho YW, et al. Deep learning in medical imaging: General overview. Korean J Radiol. 2017;18(4):570-584. doi: 10.3348/kjr.2017.18.4.570
- Yasin M, Sarıgül M, Avci M. Logarithmic learning differential convolutional neural network. Neural Netw. 2024;172:106114. doi: 10.1016/j.neunet.2024.106114
- Savadjiev P, Chong J, Dohan A, et al. Demystification of AI-driven medical image interpretation: Past, present and future. Eur Radiol. 2019;29(3):1616-1624. doi: 10.1007/s00330-018-5674-x
- Tatsugami F, Nakaura T, Yanagawa M, et al. Recent advances in artificial intelligence for cardiac CT: Enhancing diagnosis and prognosis prediction. Diagn Interv Imaging. 2023;104(11):521-528. doi: 10.1016/j.diii.2023.06.011
- Zhu B, Liu JZ, Cauley SF, Rosen BR, Rosen MS. Image reconstruction by domain-transform manifold learning. Nature. 2018;555(7697):487-492. doi: 10.1038/nature25988
- Zaharchuk G, Davidzon G. Artificial intelligence for optimization and interpretation of PET/CT and PET/MR images. Semin Nucl Med. 2021;51(2):134-142. doi: 10.1053/j.semnuclmed.2020.10.001