Navigating the complexities of migration data management: Challenges and opportunities
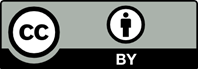
Migration is a complex global phenomenon with profound socioeconomic, political, and humanitarian implications. Effective migration data management is essential for evidence-based policymaking, efficient resource allocation, and the protection of migrant rights. However, several challenges — such as data fragmentation, privacy concerns, rapidly evolving migration patterns, technological limitations, and policy inconsistencies — hinder the comprehensive use of migration data. At the same time, emerging technologies, including artificial intelligence (AI) and big data analytics, offer transformative opportunities to enhance migration governance. Initiatives such as the Big Data for Migration Alliance (BD4M) exemplify how collaborative, data-driven approaches can improve migration management and lead to better outcomes for migrants. This paper seeks to explore both the challenges and opportunities associated with migration data management, with a particular focus on the role of innovative technologies in advancing migration research and policymaking. The analysis revealed that, with significant barriers exist, such as fragmentation, privacy concerns, dynamic patterns, technological constraints, and misaligned policies, there are also substantial opportunities for enhanced collaboration, informed policymaking, efficient resource allocation, research advancements, and increased public engagement. Furthermore, the author highlights AI and the BD4M as key components of a leading collaborative effort to leverage advanced analytics for a deeper understanding and more effective management of global migration. By analyzing diverse datasets through innovative analytical techniques, such initiatives aim to improve outcomes for migrant populations worldwide.
Ahmad Yar, A.W., & Bircan, T. (2023). Challenges with international migration data: An analysis of the experience of national statistical institutions. International Migration Review, 01979183231205564. https://doi.org/10.1177/01979183231205564
Alderson, A.S., & Nielsen, F. (2002). Globalization and the great U-turn: Income inequality trends in 16 OECD countries. American Journal of Sociology, 107(5):1244-1299. https://doi.org/10.1086/341329
Alencar, A. (2018). Refugee integration and social media: A local and experiential perspective. Information, Communication and Society, 21(11):1588-1603. https://doi.org/10.1080/1369118X.2017.1340500
Alexander, J.S., Murr, M.L., & Eddy-Miller, C.A. (2022). Testing the potential of streamflow data to predict spring migration of an ungulate herds. PLoS One, 17(1):e0262078. https://doi.org/10.1371/journal.pone.0262078
Araujo, M., Mejova, Y., Weber, I., & Benevenuto, F. (2017). Using Facebook ads audiences for Global Lifestyle Disease Surveillance: Promises and Limitations. In: Proceedings of the 2017 ACM on Web Science Conference, p.253-257.
Avramescu, A., & Wiśniowski, A. (2021). Now-casting Romanian migration into the United Kingdom by using Google Search engine data. Demographic Research, 45:1219-1254. https://doi.org/10.4054/demres.2021.45.40
Azose, J.J., & Raftery, A.E. (2019). Estimation of emigration, return migration, and transit migration between all pairs of countries. Proceedings of the National Academy of Sciences, 116(1):116-122. https://doi.org/10.1073/pnas.1722334116
Barker, E., & Bijak, J. (2023). Could we have seen it Coming? Towards an Early Warning System for Asylum Applications in the EU. Southampton: University of Southampton.
Bayir, M.A., Demirbas, M., & Eagle, N. (2009). Discovering Spatiotemporal Mobility Profiles of Cellphone Users. In: IEEE International Symposium on a World of Wireless, Mobile and Multimedia Networks and Workshops. IEEE, p.1-9.
Beduschi, A. (2021). International migration management in the age of artificial intelligence. Migration Studies, 9(3):576-596. https://doi.org/10.1093/migration/mnaa003
Berger, M.C., & Blomquist, G.C. (1992). Mobility and destination in migration decisions: The roles of earnings, quality of life, and housing prices. Journal of Housing Economics, 2(1):37-59. https://doi.org/10.1016/1051-1377(92)90018-l
Bijak, J. (2010). Forecasting International Migration in Europe: A Bayesian View. Vol. 24. Germany: Springer Science and Business Media.
Bilsborrow, R.E. (1997). International Migration Statistics: Guidelines for Improving Data Collection Systems. Switzerland: International Labour Organization.
Bircan, T., & Korkmaz, E.E. (2021). Big data for whose sake? Governing migration through artificial intelligence. Humanities and Social Sciences Communications, 8(1):1-5. https://doi.org/10.1057/s41599-021-00910-x
Böhme, M.H., Gröger, A., & Stöhr, T. (2020). Searching for a better life: Predicting international migration with online search keywords. Journal of Development Economics, 142:102347. https://doi.org/10.1016/j.jdeveco.2019.04.002
Bolhuis, M.P., & Van Wijk, J. (2021). Seeking asylum in the digital era: Social-media and mobile-device vetting in asylum procedures in five European countries. Journal of Refugee Studies, 34(2):1595-1617. https://doi.org/10.1093/jrs/feaa029
Bordons, M., Morillo, F., & Gómez, I. (2004). Analysis of cross-disciplinary research through bibliometric tools. In: Handbook of Quantitative Science and Technology Research: The Use of Publication and Patent Statistics in Studies of S and T Systems. Dordrecht: Springer Netherlands, p.437-456.
Boswell, C., Geddes, A., & Scholten, P. (2011). The role of narratives in migration policy-making: A research framework. The British Journal of Politics and International Relations, 13(1):1-11. https://doi.org/10.1111/j.1467-856X.2010.00435.x
Bottomley, G. (1992). From Another Place: Migration and the Politics of Culture. Cambridge: Cambridge University Press.
Brollo, B., & Celata, F. (2023). Temporary populations and sociospatial polarisation in the short-term city. Urban Studies, 60(10):1815-1832.
Bruckschen, F., Koebe, T., Ludolph, M., Marino, M.F., & Schmid, T. (2019). Refugees in undeclared employment-a case study in Turkey. Guide to Mobile Data Analytics in Refugee Scenarios: The’Data for Refugees Challenge’Study. Germany: Springer Nature, p.329-346.
Burrell, J. (2016). How the machine ‘thinks’: Understanding opacity in machine learning algorithms. Big Data and Society, 3(1):1-12. https://doi.org/10.1177/2053951715622512
Cahuc, P., Carcillo, S., & Zylberberg, A. (2014). Labor Economics. United States: MIT Press.
Canada Immigration Lowey Association (CILA). (2023). AI Facial Recognition Technology in the Canadian Immigration System. Available from: https://cila.co/ai-facial-recognition-technology-in-the-canadian-immigration-system [Last accessed on 2024 Dec 02].
Carlier, J.Y., Crépeau, F., & Purkey, A. (2020). From the 2015 European “Migration Crisis” to the 2018 Global Compact for Migration. McGill Journal of Sustainable Development Law/Revue de Droit du Développement Durable de McGill, 16(1):37-81.
Castles, S. (2002). Migration and community formation under conditions of globalization. International Migration Review, 36(4):1143-1168. https://doi.org/10.1111/j.1747-7379.2002.tb00121.x
Chi, G., Lin, F., Chi, G., & Blumenstock, J. (2020). A general approach to detecting migration events in digital trace data. PLoS One, 15(10):e0239408. https://doi.org/10.1371/journal.pone.0239408
Chui, M., Harryson, M., Valley, S., Manyika, J., & Roberts, R. (2018). Notes from the AI Frontier Applying AI for Social Good. New York: McKinsey Global Institute.
Ciupijus, Z. (2010). Ethical pitfalls of temporary labour migration: A critical review of issues. Journal of Business Ethics, 97:9-18. https://doi.org/10.1007/s10551-011-1075-7
Collins, F.L. (2023). Geographies of migration III: The digital migrant. Progress in Human Geography, 47(5):738-749. https://doi.org/10.1177/03091325231157709
Connor, P. (2017). Can Google Trends Forecast Forced Migration flows? Perhaps, but Under certain conditions. In: Population Association of America Meeting. Vol. 202017. Chicago, Illinois. Available from: https://paa.confex.com/paa/2017/mediafile/extendedabstract/ paper9477/paa%20 [Last accesed on 2025 Feb 03].
Cumbane, S.P., & Gidófalvi, G. (2021). Spatial distribution of displaced population estimated using mobile phone data to support disaster response activities. ISPRS International Journal of Geo-Information, 10(6):421. https://doi.org/10.3390/ijgi10060421
De Beer, J., Raymer, J., Van der Erf, R., & Van Wissen, L. (2010). Overcoming the problems of inconsistent international migration data: A new method applied to flows in Europe. European Journal of Population/Revue Europeenne de Demographie, 26(4):459.
De Jong, G.F., Chamratrithirong, A., & Tran, Q.G. (2002). For better, for worse: Life satisfaction consequences of migration 1. International Migration Review, 36(3):838-863. https://doi.org/10.1111/j.1747-7379.2002.tb00106.x
Derave, C., Genicot, N., & Hetmanska, N. (2022). The risks of trustworthy artificial intelligence: The case of the European travel information and authorisation system. European Journal of Risk Regulation, 13(3):389-420. https://doi.org/10.1017/err.2022.5
Dolado, J., Goria, A., & Ichino, A. (1994). Immigration, human capital and growth in the host country: Evidence from pooled country Data. Journal of Population Economics, 7(2):193-215. https://doi.org/10.1007/bf00173619
Fantazzini, D., Pushchelenko, J., Mironenkov, A., & Kurbatskii, A. (2021). Forecasting internal migration in Russia using Google Trends: Evidence from Moscow and Saint Petersburg. Forecasting, 3(4):774-803. https://doi.org/10.3390/forecast3040048
Fassmann, H. (2009). European migration: Historical overview and statistical problems. Statistics and reality. Concepts and measurements of migration in Europe. Amsterdam: Amsterdam University Press, p.21-44.
Fehr, H., Jokisch, S., & Kotlikoff, L.J. (2008). Fertility, mortality and the developed world’s demographic transition. Journal of Policy Modeling, 30(3):455-473. https://doi.org/10.1016/j.jpolmod.2008.01.002
Floridi, L., Cowls, J., Beltrametti, M., Chatila, R., Chazerand, P., Dignum, V., et al. (2018). AI4People-an ethical framework for a good AI society: Opportunities, risks, principles, and recommendations. Minds and Machines, 28:689-707. https://doi.org/10.1007/s11023-018-9482-5
Forti, M. (2021). AI-driven migration management procedures: Fundamental rights issues and regulatory answers. BioLaw Journal, 2:433-451.
Franklinos, L.H., Parrish, R., Burns, R., Caflisch, A., Mallick, B., Rahman, T., et al. (2021). Key opportunities and challenges for the use of big data in migration research and policy. UCL Open Environment, 3:e027. https://doi.org/10.14324/111.444/ucloe.000027
Giguashvili, G. (2023). Possibilities of using Artificial Intelligence in the process of international migration management. Innovative Economics and Management, 10(3):58-66. https://doi.org/10.46361/2449-2604.10.3.2023.58-66
Gonzalez-Salinas, A.I., Andrade, E.L., Abroms, L.C., Gomez, K., Favetto, C., & Collins, K.K. (2024). Latino parents’ reactions to and engagement with a facebook group-based COVID-19 vaccine promotion intervention: Mixed methods pilot study. JMIR Formative Research, 8(1):e51331. https://doi.org/10.2196/51331
Guo, S. (2010). Toward recognitive justice: Emerging trends and challenges in transnational migration and lifelong learning. International Journal of Lifelong Education, 29(2), 149-167. https://doi.org/10.1080/02601371003616533
Heeks, R., & Renken, J. (2018). Data justice for development: What would it mean? Information Development, 34(1):90-102. https://doi.org/10.1177/0266666916678282
Hughes, C., Zagheni, E., Abel, G.J., Sorichetta, A., Wi’sniowski, A., Weber, I., et al. (2016). Inferring migrations: Traditional methods and new approaches based on mobile phone, social media, and other big data: Feasibility study on inferring (labour) mobility and migration in the European Union from big data and social media data.
IOM. (2018). Big Data and Migration. Geneva: IOM.
IOM. (2011). Glossary on Migration. N25. Available from: https://publications.iom.int/system/files/pdf/iml25_1.pdf [Last accessed on 2024 Dec 12].
IOM. (2018). Data Bulletin-Big Data and Migration. ISPRA: International Organization for Migration. Available from: https://publications.iom.int/system/files/pdf/issue_5_big_ data_and_migration.pdf [Last accessed on 2024 Jan 20].
IOM. (2020). Regional Data Overview: Migration Data in Europe. Available from: Available from: https://migrationdataportal.org/regional-data-overview/europe
IOM. (2021). Big Data for Migration Alliance Launches Call to Harness Responsible Data Innovation for Better Migration Governance. Available from: https://gmdac.iom.int/ news/big-data-migration-alliance-launches-call-harness-responsible-data-innovation-better-migration-governance [Last accessed on 2024 Oct 22].
IOM. (2024). World Migration Report 2024. Available from: https://worldmigrationreport.iom.int/msite/wmr-2024- interactive [Last accessed on 2024 Oct 21].
Jacobsen, K., & Landau, L.B. (2003). The dual imperative in refugee research: Some methodological and ethical considerations in social science research on forced migration. Disasters, 27(3):185-206. https://doi.org/10.1111/1467-7717.00228
Jasmontaite-Zaniewicz, L., & Zomignani Barboza, J. (2021). Disproportionate surveillance: Technology-assisted and automated decisions in asylum applications in the EU? International Journal of Refugee Law, 33(1):89-110. https://doi.org/10.1093/ijrl/eeab031
Jaumotte, M.F., Koloskova, K., & Saxena, M.S.C. (2016). Impact of Migration on Income Levels in Advanced Economies. United States: International Monetary Fund.
Josipovic, I. (2023). What can data justice mean for asylum governance? The case of smartphone data extraction in Germany. Journal of Refugee Studies, 36(3):534-551. https://doi.org/10.1093/jrs/fead049
Kahanec, M., & Zimmermann, K.F. (2008). Migration and Globalization: Challenges and Perspectives for the Research Infrastructure. Germany: IZA - Institute of Labor Economics.
Kaneti, M. (2023). What can AI see? The image of the ‘migrant’in the era of AI post- visualization. Journal of Global Ethics, 19(3):307-322. https://doi.org/10.1080/17449626.2023.2279229
Keely, C.B. (2000). Demography and international migration. In: Migration Theory: Talking Across Disciplines. New York: Routledge, p.43-60.
Keijzer, N., & Klingebiel, S. (2017). Realising the Data Revolution for Sustainable Development: Towards Capacity Development 4.0. In: Partnership in Statistics for Development in the 21st Century Discussion Paper No, 9.
Khan, W., & Ullah, H. (2010). Scientific reasoning: A solution to the problem of induction. International Journal of Basic and Applied Sciences, 10(3):58-62.
King, R. (2013). Theories and Typologies of Migration: An Overview and a Primer. Sweden: Malmö Institute for Studies of Migration.
Kukulska-Hulme, A. (2019). Mobile language learning innovation inspired by migrants. Journal of Learning for Development, 6(2):116-129. https://doi.org/10.56059/jl4d.v6i2.349
Kuner, C., & Marelli, M. (2020). Handbook on Data Protection in Humanitarian Action. 2nd ed. Geneva and Brussels: Brussels Privacy Hub, Vrije Universiteit Brussel, the Data Protection Office of the International Committee of the Red Cross (ICRC).
Kwilinski, A., Lyulyov, O., Pimonenko, T., Dzwigol, H., Abazov, R., & Pudryk, D. (2022). International migration drivers: Economic, environmental, social, and political effects. Sustainability, 14(11):6413. https://doi.org/10.3390/su14116413
Lai, S., Erbach-Schoenberg, E.Z., Pezzulo, C., Ruktanonchai, N.W., Sorichetta, A., Steele, J., et al. (2019). Exploring the use of mobile phone data for national migration statistics. Palgrave Communications, 5(1):1-10. https://doi.org/10.1057/s41599-019-0242-9
Leslie, D., Katell, M., Aitken, M., Singh, J., Briggs, M., Powell, R., et al. (2022). Advancing Data Justice Research and Practice: An Integrated Literature Review. [arXiv Preprint].
Lipton, M. (1980). Migration from rural areas of poor countries: the impact on rural productivity and income distribution. World Development, 8(1):1-24. https://doi.org/10.1016/0305-750x(80)90047-9
Luca, M., Barlacchi, G., Oliver, N., & Lepri, B. (2021). Leveraging Mobile Phone Data for Migration Flows. [arXiv Preprint].
Lutz, W., Amran, G., Bélanger, A., Conte, A., Gailey, N., Ghio, D., et al. (2019). Demographic Scenarios for the EU: Migration, Population and Education. Luxembourg: Publications Office of the European Union.
Mahabir, R., Croitoru, A., Crooks, A., Agouris, P., & Stefanidis, A. (2018). News coverage, digital activism, and geographical saliency: A case study of refugee camps and volunteered geographical information. PLoS One, 13(11):e0206825. https://doi.org/10.1371/journal.pone.0206825
Martin, P.L., & Zürcher, G. (2008). Managing Migration: The Global Challenge. Vol. 63. Washington, DC: Population Reference Bureau.
Mavroudi, E., & Nagel, C. (2016). Global Migration: Patterns, Processes, and Politics. England, UK: Routledge.
Metcalfe, P., & Dencik, L. (2019). The politics of big borders: Data (in) Justice and the governance of refugees. First Monday, 24(4). https://doi.org/10.5210/fm.v24i4.9934
Meyer, B.D., Mok, W.K., & Sullivan, J.X. (2015). Household surveys in crisis. Journal of Economic Perspectives, 29(4):199-226. https://doi.org/10.1257/jep.29.4.199
Micinski, N.R., & Jones, W. (2022). Digitization without digital evidence: Technology and Sweden’s asylum system. Journal of Refugee Studies, 35(2):1011-1029. https://doi.org/10.1093/jrs/feab041
Molnar, P. (2019). Technology on the margins: AI and global migration management from a human rights perspective. Cambridge International Law Journal, 8(2):305-330. https://doi.org/10.4337/cilj.2019.02.07
Napierala, J., Hilton, J., Forster, J.J., Carammia, M., & Bijak, J. (2022). Toward an early warning system for monitoring asylum-related migration flows in Europe. International Migration Review, 56(1):33-62. https://doi.org/10.1177/01979183211035736
Nathan, M. (2014). The wider economic impacts of high-skilled migrants: A survey of the literature for receiving countries. IZA Journal of Migration, 3(1):1-20. https://doi.org/10.1186/2193-9039-3-4
Noori, S. (2024). Navigating the Aegean Sea: Smartphones, transnational activism and viapolitical in (ter) ventions in contested maritime borderzones. In: The Digital Empowerment-Control Nexus. England, UK: Routledge, p.36-52.
Nyoni, B. (2017). How Artificial Intelligence can be Used to Predict Africa’s Next Migration Crisis. Geneva: UNHCR Innovation Service.
Olivieri, S., Ortega, F., Rivadeneira, A., & Carranza, E. (2021). Shoring up economic refugees: Venezuelan migrants in the Ecuadoran labor market. Migration Studies, 9(4):1590-1625. https://doi.org/10.1093/migration/mnab037
Ozkul, D. (2023). Automating Immigration and Asylum: The Uses of New Technologies in Migration and Asylum Governance in Europe. Available from: https://www.rsc.ox.ac. uk/publications/automating-immigration-and-asylum-the-uses-of-new-technologies-in-migration-and-asylum-governance-in-europe [Last accessed on 2023 Jan 18].
Poulain, M. (2008). European migration statistics: Definitions, data and challenges. Mapping Linguistic Diversity in Multicultural Contexts, 94:43-68. https://doi.org/10.1515/9783110207347.1.43
Qi, H., & Bircan, T. (2023). Can google trends predict asylum-seekers’ destination choices? EPJ Data Science, 12(1):41. https://doi.org/10.1140/epjds/s13688-023-00419-0
Quach, S., Thaichon, P., Martin, K.D., Weaven, S., & Palmatier, R.W. (2022). Digital technologies: Tensions in privacy and data. Journal of the Academy of Marketing Science, 50(6):1299-1323. https://doi.org/10.1007/s11747-022-00845-y
Rampazzo, F., Rango, M., & Weber, I. (2023). New migration data: Challenges and opportunities. In: Handbook of Computational Social Science for Policy. Germany: Springer Nature, p.345.
Ratha, D., Mohapatra, S., & Scheja, E. (2011). Impact of Migration on Economic and social Development: A Review of Evidence and Emerging Issues. World Bank Policy Research Working Paper, no 5558.
Rees, P., Bell, M., Duke-Williams, O., & Blake, M. (2000). Problems and solutions in the measurement of migration intensities: Australia and Britain compared. Population Studies, 54(2):207-222. https://doi.org/10.1080/713779082
Roos, L., Walld, R., Burchill, C., Nickel, N., & Roos, N.P. (2017). Linkable administrative files: Family information and existing data. Longitudinal and Life Course Studies, 8(3):264-280. https://doi.org/10.14301/llcs.v8i3.406
Ryan, L., & D’Angelo, A. (2018). Changing times: Migrants’ social network analysis and the challenges of longitudinal research. Social Networks, 53:148-158. https://doi.org/10.1016/j.socnet.2017.03.003
Salah, A.A., Pentland, A., Lepri, B., Letouzé, E., De Montjoye, Y.A., Dong, X., et al. (2019). Guide to Mobile Data Analytics in Refugee Scenarios. The Data for Refugees Challenge ‘Study. Cham: Springer. https://doi.org/10.1007/978-3-030-12554-7_1
Salganik, M.J. (2019). Bit by Bit: Social Research in the Digital Age. United States: Princeton University Press.
Sherbinin, A.D., Carr, D., Cassels, S., & Jiang, L. (2007). Population and environment. Annual Review of Environment and Resources, 32:345-373. https://doi.org/10.1146/annurev.energy.32.041306.100243
Spencer, S., & Charsley, K. (2016). Conceptualising integration: A framework for empirical research, taking marriage migration as a case study. Comparative Migration Studies, 4(1):1-19. https://doi.org/10.1186/s40878-016-0035-x
Spyratos, S., Vespe, M., Natale, F., Weber, I., Zagheni, E., & Rango, M. (2019). Quantifying international human mobility patterns using Facebook Network data. PLoS One, 14(10):e0224134. https://doi.org/10.1371/journal.pone.0224134
State, B., Zagheni, E., Garimella, V.R.K., & Weber, I. (2014). Inferring International and Internal Migration Patterns from Twitter Data. In: Proceedings of the 23rd International Conference on World Wide Web, p.439-444. https://doi.org/10.1145/2567948.2576930
Tatem, A.J. (2017). WorldPop, open data for spatial demography. Scientific Data, 4(1):1-4. https://doi.org/10.1038/sdata.2017.4
Taylor, L. (2017). What is data justice? The case for connecting digital rights and freedoms globally. Big Data and Society, 4(2):2053951717736335. https://doi.org/10.1177/2053951717736335
Ting-Toomey, S., Yee-Jung, K.K., Shapiro, R.B., Garcia, W., Wright, T.J., & Oetzel, J.G. (2000). Ethnic/cultural identity salience and conflict styles in four US ethnic groups. International Journal of Intercultural Relations, 24(1):47-81. https://doi.org/10.1016/s0147-1767(99)00023-1
Tjaden, J. (2021). Measuring migration 2.0: A review of digital data sources. Comparative Migration Studies, 9(1):59. https://doi.org/10.1186/s40878-021-00273-x
Topel, R. (1999). Labor markets and economic growth. In: Handbook of Labor Economics. Vol. 3. Netherlands: Elsevier, p.2943-2984. https://doi.org/10.1016/s1573-4463(99)30035-3
Turper Alışık, S., Bayraktar Aksel, D., Yantaç, A.E., Kayi, İ., Salman, S., İçduygu, A., et al. (2019). Seasonal labor migration among Syrian refugees and urban deep map for integration in Turkey. In: Guide to Mobile Data Analytics in Refugee Scenarios: The’Data for Refugees Challenge’Study. Germany: Springer, p.305-328. https://doi.org/10.1007/978-3-030-12554-7_16
Turper, S. (2023). Big data in migration research: The ethical considerations. Reflektif Sosyal Bilimler Dergisi, 4(3): 795-807. https://doi.org/10.47613/reflektif.2023.139
United Nations. (2017). Data for Democracy Wins Unite Ideas #IDETECT Data Challenge to Monitor Worldwide Patterns of Internal Displacement. In: Meetings Coverage and Press Releases PI/2207. Available from: https://press.un.org/en/2017/pi2207.doc.htm [Last accessed on 2024 Sep 22].
Van Liempt, I., & Bilger, V. (2012). 21 Ethical challenges in research with vulnerable migrants. In: Handbook of Research Methods in Migration. United Kingdom: Edward Elgar Publishing, 451.
Vavoula, N. (2021). Artificial intelligence (AI) at schengen borders: automated processing, algorithmic profiling and facial recognition in the era of techno-solutionism. European Journal of Migration and Law, 23(4):457-484. https://doi.org/10.1163/15718166-12340114
Vicéns-Feliberty, M.A., & Ricketts, C.F. (2016). An analysis of Puerto Rican interest in migrating to the united states using google trends. The Journal of Developing Areas, 50:411-430. https://doi.org/10.1353/jda.2016.0090
Wells, N. (2017). Consumer News and Business Channel (CNBC). How the Yahoo Hack Stacks up to Previous Data Breaches. Available from: https://www.cnbc.com/2017/10/04/how-the-yahoo-hack-stacks-up-to-previous-data-breaches.html [Last accessed on 2024 Sep 21].
Wladyka, D.K. (2017). Queries to google search as predictors of migration flows from latin America to Spain. Journal of Population and Social Studies, 25(4):312-327. https://doi.org/10.25133/jpssv25n4.002
Wong, R.W.M., & Chun, A.H.W. (2007). eBrain-Using AI for Automatic Assessment at the Hong Kong Immigration Department. Available from: https://www.cs.cityu.edu. hk/~hwchun/research/PDF/eBrain_IAAI.pdf
Zagheni, E., Weber, I., & Gummadi, K. (2017). Leveraging Facebook’s advertising platform to monitor stocks of migrants. Population and Development Review, 43(4):721-734. https://doi.org/10.1111/padr.12102