Local population changes as a spatial varying multiscale process: The Italian case
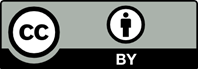
The population dynamics in Italy show a strong spatial heterogeneity within a framework of persistent demographic territorial disparities. From a local point of view, it is necessary to understand what demographic determinants govern this process. In the paper, we model the population change according to a local (i.e., spatial varying coefficients) multiscale approach. To this aim, local demographic growth rates of each Italian municipality for the period 2011 – 2019 were estimated and modeled by means of a classic a-spatial global model (i.e., ordinary least-square), and a multiscale geographically weighted regression. The multiscale dimensions of local population changes are therefore analyzed by means of three sub-dimensions: Level of influence, scalability, and specificity. The results show that the determinants of local population changes are not spatially constant and that they vary in their effect at different geographical scales.
Benassi, F., & Carella, M. (2022). Modelling geographical variations in fertility and population density of Italian and foreign populations at the local scale: A spatial Durbin approach for Italy (2002-2018). Quality and Quantity. https://doi.org/10.1007/s11135-022-01446-1
Benassi, F., & Naccarato, A. (2017). Households in potential economic distress. A geographically weighted regression model for Italy, 2001-2011. Spatial Statistics, 21:362-376. https://doi.org/10.1016/j.spasta.2017.03.002
Benassi, F., Bonifazi, C., Heins, F., Licari, F., & Tucci, E. (2019). Population change and international and internal migration in Italy, 2002-2017: Ravenstein revisited. Comparative Population Studies, 44: 497-531. https://doi.org/10.12765/CPoS-2020-16
Benassi, F., Busetta, A., Gallo, G., & Stranges, M. (2021). The Inequalities between Territories. In: Billari, F.C., & Tomassini, C. (A Cura di). AISP-Population Report. Italy and the challenges of demography. Bologna: Il Mulino, p.135-161. [In Italian].
Benassi, F., Busetta, A., Gallo, G., & Stranges, M. (2023). Neighbourhood Effects and Determinants of Demographic Changes in Italy: A Spatial Perspective. Vienna Yearbook of Population Research. Vienna, Austria: Austrian Academy of Sciences Press. https://doi.org/10.1553/p-5dfz-c44a
Billari, F.C., & Tommasini, C. (2021). Population Report. Italy and the Challenges of Demography. Bologna: Il Mulino. [In Italian].
Bonifazi, C., Heins, F., Licari, F., & Tucci, E. (2021). The regional dynamics of internal migration intensities in Italy. Population, Space and Place, 27(7):e2331. https://doi.org/10.1002/psp.2331
Burillo, P., Salvati, L., Matthews, S.A., & Benassi, F. (2020). Local-scale fertility variations in a low-fertility country: Evidence from Spain (2002-2017). Canadian Studies in Population, 47(4):279-295. https://doi.org/10.1007/s42650-020-00036-6
Burnham, K.P., & Anderson, D.R. (2004). Multimodel inference: Understanding AIC and BIC in model selection. Sociological Methods and Research, 33(2):261-304. https://doi.org/10.1177/0049124104268644
Caltabiano, M., Dreassi, E., Rocco, E., & Vignoli, D. (2019). A subregional analysis of family change: The spatial diffusion of one‐parent families across Italian municipalities, 1991- 2011. Population, Space and Place, 25(4):e2237. https://doi.org/10.1002/psp.2237
Chi, G., & Zhu, J. (2008). Spatial regression models for demographic analysis. Population Research and Policy Review, 27(1):17-42. https://doi.org/10.1007/s11113-007-9051-8
Cupido, K., Fotheringham, A.S., & Jevtic, P. (2021). Local modelling of U.S. mortality rates: A multiscale geographically weighted regression approach. Population Space and Place, 27(151):e2379. https://doi.org/10.1002/psp.2379
De Castro, M.C. (2007). Spatial demography: An opportunity to improve policy making at diverse decision levels. Population Research and Policy Review, 26(5):477-509. https://doi.org/10.1007/s11113-007-9041-x
Fotheringham, A.S., Brunsdon, C., & Charlton, M. (2002). Geographically Weighted Regression: The Analysis of Spatially Varying Relationships. Chichester: Wiley.
Fotheringham, A.S., Yang, W., & Kang, W. (2017). Multiscale geographically weighted regression (MGWR). Annals of the American Association of Geographers, 107(6):1247-1265. https://doi.org/10.1080/24694452.2017.1352480
Gu, H., Lao, X., & Shen, T. (2020). Research progress on spatial demography. In: Ye, X., & Lin, H. (eds.). Spatial Synthesis: Computational Social Science and Humanities. New York City: Springer International Publishing, p.125-145.
Howell, F.M., Porter, J.R. & Matthews, S.A. (2016). Recapturing Space: New Middle-Range Theory in Spatial Demography. Champ: Springer.
Klingholz, R. (2009). Europe’s Real Demographic Challenge. Policy Review. Vol. 157, p.61-70 Available from: https://www.proquest. com/openview/30d877f0a74a1cb1d568d0a9a57d1f0b/1. pdf?cbl=47546&pq-origsite=gscholar [Last accessed on 2022 Jul 20].
Lamonica, G.R., & Zagaglia, B. (2013). The determinants of internal mobility in Italy, 1995-2006: A comparison of Italians and resident foreigners. Demographic Research, 29:407-440. https://doi.org/10.4054/demres.2013.29.16
Li, Z., & Fotheringham, S. (2020). Computational improvements to multi-scale geographically weighted regression. International Journal of Geographical Information Science, 34(7):1378–1397. https://doi.org/10.1080/13658816.2020.1720692
Lloyd, C.D. (2016). Are spatial inequalities growing? The scale of population concentrations in England and Wales. Environment and Planning A, 48(7):1318-1336, https://doi.org/10.1177/0308518X15621306
Matthews, S.A. (2019). Methods and applications in spatial demography. Mathematical Population Studies, 26(4):183-184. https://doi.org/10.1080/08898480.2019.1653058
Matthews, S.A., & Parker, D.M. (2013). Progress in spatial demography. Demographic Research, 28(10):271-312. https://doi.org/10.4054/demres.2013.28.10
Matthews, S.A., & Yang, T.C. (2012). Mapping the results of local statistics: Using geographically weighted regression. Demographic Research, 26:151-166. https://doi.org/10.4054/DemRes.2012.26.6
Mucciardi, M. (2021). Local and global analysis of fertility rate in Italy. In: Popkova, E.G., & Sergi, B.S. (eds.) Smart Technologies for Society, State and Economy. Cham: Springer, p. 465–474.
Nakaya, T. (2015). Semiparametric geographically weighted generalized linear modelling: The concept and implementation using GWR4. In: Brunsdon, C., & Singleton, A. (eds.). Geocomputation: A Practical Primer. London: Sage, p.201-220.
Nakaya, T., Fotheringham, A.S., Brunsdon, C., & Charlton, M. (2005). Geographically weighted Poisson regression for disease association mapping. Statistics in Medicine, 24:2695-2717. https://doi.org/10.1002/sim.2129
Oshan, T.M., Li, Z., Kang, W., Wolf, L.J., & Fotheringham, A.S. (2019). MGWR: A Python implementation of multiscale geographically weighted regression for investigating process spatial heterogeneity and scale. ISPRS International Journal of Geo-Information, 8(6):269. https://doi.org/10.3390/ijgi8060269
Oshan, T.M., Smith, J.P., & Fotheringham, A.S. (2020). Targeting the spatial context of obesity determinants via multiscale geographically weighted regression. International Journal of Health Geography, 19(1):11. https://doi.org/10.1186/s12942-020-00204-6
Oshan, T.M., Wolf, L.J., Sachdeva, M., Bardin, S., & Fotheringham, A.S. (2022). A scoping review on the multiplicity of scale in spatial analysis. Journal of Geographical Systems, 24:293-324. https://doi.org/10.1007/s10109-022-00384-8
Preston, S.H., Heuveline, P., & Guillot, M. (2001). Demography: Measuring and Modeling Population Processes. Oxford: Blackwell Publishers.
Raymer, J., Willekens, F., & Rogers, A. (2019). Spatial demography: A unifying core and agenda for further research. Population, Space and Place, 25(4):e2179. https://doi.org/10.1002/psp.2179
Reynaud, C., & Miccoli, S. (2018). Depopulation and the aging population: The relationship in Italian municipalities. Sustainability, 10(4):1004. https://doi.org/10.3390/su10041004
Reynaud, C., Miccoli, S., & Lagona, F. (2018). Population ageing in Italy: An empirical analysis of change in the ageing index across space and time. Spatial Demography, 6(3):235-251. https://doi.org/10.1007/s40980-018-0043-6
Reynaud, C., Miccoli, S., Benassi, F., Naccarato, A., & Salvati, L. (2020). Unravelling a demographic ‘Mosaic’: Spatial patterns and contextual factors of depopulation in Italian municipalities, 1981-2011. Ecological Indicators, 115:106356. https://doi.org/10.1016/j.ecolind.2020.106356
Salvati, L., Benassi, F., Miccoli, S., Rabiei-Dastjerdi, H., & Matthews, S.A. (2020). Spatial variability of total fertility rate and crude birth rate in a low-fertility country: Patterns and trends in regional and local scale heterogeneity across Italy, 2002-2018. Applied Geography, 124:102321. https://dx.doi.org/10.1016/j.apgeog.2020.102321
Song, J., Yu, H., & Lu, Y. (2021). Spatial-scale dependent risk factors of heat-related mortality: A multiscale geographically weighted regression analysis. Sustainable Cities and Society, 74: 103159. https://doi.org/10.1016/j.scs.2021.103159
Strozza, S., Benassi, F., Ferrara, R., & Gallo, G. (2016). Recent demographic trends in the major Italian Urban agglomerations: The role of foreigners. Spatial Demography, 4(1): 39–70, https://doi.org/10.1007/s40980-015-0012-2
Vitali, A., & Billari, F.C. (2017). Changing determinants of low fertility and diffusion: A spatial analysis for Italy. Population, Space and Place 23(2):e1998. https://doi.org/10.1002/psp.1998
Voss, P.R. (2007). Demography as a spatial social science. Population Research and Policy Review, 26(5):457-476. https://doi.org/10.1007/s11113-007-9047-4
Weeks, J.R. (2004). The Role of Spatial Analysis in Demographic Research. In: Goodchild, M.F., & Janelle, D.G. (eds.). Spatially Integrated Social Science. New York: Oxford University Press, p.381-399.
Weeks, J.R. (2016). Demography is an inherently spatial science. In: Howell, F.M., Porter, J.R., & Matthews, S.A. (eds.). Recapturing Space: New Middle-range Theory in Spatial Demography. Cham: Springer, p.99-122.
Wolf, L.J., Oshan, T.M., & Fotheringham, A.S. (2017). Single and multiscale models of process spatial heterogeneity. Geographical Analysis, 50(3):223-246. https://doi.org/10.1111/gean.12147
Yang, T.C., Matthews, S.A., & Sun, F. (2022a). Multiscale dimensions of spatial process: COVID-19 fully vaccinated rates in U.S. counties. American Journal of Preventive Medicine, 63(6):954-961. https://doi.org/10.1016/j.amepre.2022.06.006
Yang, T.C., Shoff, C., Choi, S.W.E., & Sun, F. (2022b). Multiscale dimensions of county-level disparities in opioid use disorder rates among older Medicare beneficiaries. Frontiers in Public Health, 10: 993507. https://doi.org/10.3389/fpubh.2022.993507
Yu, H., Fotheringham, A.S., Li, Z., Oshan, T., Kang, W., & Wolf, L.J. (2020a). Inference in multiscale geographically weighted regression. Geographical Analysis, 52(1):87-106. https://doi.org/10.1111/gean.12189
Zambon, I., Rontos, K., Reynaud, C., & Salvati, L. (2020). Toward an unwanted dividend? Fertility decline and the north-south divide in Italy, 1952-2018. Quality and Quantity, 54(1): 169-187. https://doi.org/10.1007/s11135-019-00950-1