Optimization of nonlinear controller with an enhanced biogeography approach
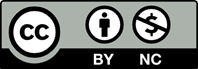
This paper is dedicated to the optimization of nonlinear controllers basing of an enhanced Biogeography Based Optimization (BBO) approach. Indeed, The BBO is combined to a predator and prey model where several predators are used with introduction of a modified migration operator to increase the diversification along the optimization process so as to avoid local optima and reach the optimal solution quickly. The proposed approach is used in tuning the gains of PID controller for nonlinear systems. Simulations are carried out over a Mass spring damper and an inverted pendulum and has given remarkable results when compared to genetic algorithm and BBO.
[1] Johnson M.A and M.H. Moradi, PID Controllers: New Identification and Design Methods. Springer, (2005).
[2] Teng T.K., J.S. Shieh and C.S. Chen, Genetic algorithms applied in online auto tuning PID parameters of a liquid-level control system, Transaction of the Institute of Measurement and control, 25(5), 433- 450, (2003).
[3] Fan L., and E.M. Joo, Design for Autotuning PID Controller Based on Genetic Algorithms, In: 4th IEEE Conference on Industrial Electronics and Applications (ICIEA 2009), 25-27 May 2009, Xian, China, (2009).
[4] Chiha I., N. Liouane, and P. Borne, Tuning PID Controller Using Multiobjective Ant Colony Optimization, Applied Computational Intelligence and Soft Computing, 2012(1), (2012).
[5] Wang Y.B, X. Peng and B.Z. We, A new particle swarm optimization based autotuning of PID controller, Seventh International Conference on Machine Learning and Cybernetics, 12-15 Jul. 2008, Kunming, China, (2008).
[6] Solihin M.I., L.F. Tack and M.L. Kean, Tuning of PID Controller Using Particle Swarm Optimization (PSO), International Conference on Advanced Science, Engineering and Information Technology, Selangor, 14-15 Jan. 2011, Kuala Lumpur, Malaysia, (2011)
[7] Salem M. and M.F. Khelfi, Application of Biogeography based optimization in tuning a PID controller for nonlinear systems, IEEE International Conference on Complex Systems (ICCS), 5-6 Nov 2012, Agadir, Morocco, (2012).
[8] Simon.D, Biogeography-based optimization, IEEE Transactions on Evolutionary Computation, 12(6), 702-713, (2008)
[9] Lohokare M.R., S.S.,Pattnaik. B.K,Panigrahi and S Das, Accelerated biogeography-based optimization with neighborhood search for optimization, Applied Soft Computing, 13 (5), 2318-2342, (2013).
[10] Ma H., and D. Simon, Blended biogeography-based optimization for constrained optimization. Engineering Applications of Artificial Intelligence, 24 (3), 517-525 , (2011).
[11] Ma H., D. Simon, M. Fei and Z. Xie, Variations of biogeography-based optimization and Markov analysis, 2013. Information Sciences, 220(1), 492- 506, (2013).
[12] Sayed M.M., M.S. Saad, H.M. Emara, and A.E.E. El-Zahab, A Novel Method for PID Tuning Using a Modified BiogeographyBased Optimization Algorithm, In: 24th Chinese Control and Decision Conference (CCDC), 23-25 May 2012, Taiyuan, China, (2012).
[13] Silva M.A.C., L. dos S. Coelho and R.Z. Freire, Biogeography-based Optimization approach based on Predator-Prey concepts applied to path planning of 3- Technologies and Factory Automation (ETFA), 13-16 Sept. 2010, Cracow, Poland, 1-8, (2010).
[14] Higashitani M., A. Ishigame, K.Yasuda, Particle Swarm Optimization Considering the Concept of Predator-Prey Behavior, IEEE Congress on Evolutionary Computation (CEC), 16-21 Jul 2006, Vancouver, Canada, 434-437, (2006).
[15] Bagis A., Determination of the PID Controller Parameters by Modified Genetic Algorithm for Improved Performance, Journal of Information Science and Engineering, 23, 1469-1480, (2007).
[16] Kundra H., A. Kaur and V. Panchal, An integrated approach to biogeography based optimization with case based reasoning for retrieving groundwater possibility, in: 8th Annual Asian Conference and Exhibition on Geospatial Information, Technology and Applications, 18-20 Aug. 2009, Singapore, (2009).
[17] Li X., A real-coded predator-prey genetic algorithm for multiobjective optimization, Evolutionary Multi-Criterion Optimization Lecture Notes in Computer Science, 2632,207-221, (2003).
[18] Ovreiu M., and D. Simon, Biogeographybased optimization of neuro-fuzzy system parameters for diagnosis of cardiac disease, In: Genetic and Evolutionary Computation Conference, Portland, Oregon, USA, 1235- 1242, (2010).
[19] Panchal V., P. Singh, N. Kaur, and H. Kundra, Biogeography based satellite image classification. International Journal of Computer Science and Information Security, 6(2), 269-274, (2009).
[20] Simon D., A dynamic system model of biogeo Soft Comput graphy ing -based optimization, Applied 11(8), 5652-5661, (2011)