Advancing machine learning in additive manufacturing: Perspectives on data challenges, model development, and industrial adoption
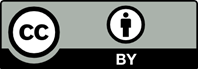
Over the past decade, 100 of scientific studies have harnessed statistical artificial intelligence, specifically machine learning (ML), to address the existing challenges to process repeatability and part quality in additive manufacturing (AM). ML has also been applied to support structure and material design for AM. This rapidly expanding field at the intersection of two growing disciplines provides opportunities to mature AM technology in the industry. There have been numerous review articles and survey reports to summarize ML applications at design, processes, structure, and property phases. While ML models, data handling, and learning techniques for AM concerns have been summarized in these articles, no work has specifically focused on the core ML challenges of data scarcity and model complexity – two important aspects to develop ML models for the AM industry. This perspective highlights existing data and model challenges and discusses the opportunities to address them through advanced techniques in data science and ML. By enhancing the usefulness of AM datasets and by leveraging the strengths of cutting-edge ML models, the research progress at the intersection of ML and AM can be effectively translated into real-world industrial applications making the deployment of ML models in the industry much easier.
- Gibson I, Rosen DW, Stucker B, et al. Additive Manufacturing Technologies. Vol 17. Germany: Springer; 2021.
- Wang C, Tan X, Tor S, Lim C. Machine learning in additive manufacturing: State-of-the-art and perspectives. Addit Manuf. 2020;36:101538. doi: 10.1016/j.addma.2020.101538
- Naser AZ, Defersha F, Pei E, Zhao YF, Yang S. Toward automated life cycle assessment for additive manufacturing: A systematic review of influential parameters and framework design. Sustain Prod Consum. 2023;41:253-274.
- Sun C, Wang Y, McMurtrey MD, Jerred ND, Liou F, Li J. Additive manufacturing for energy: A review. Appl Energy. 2021;282:116041. doi: 10.1016/j.apenergy.2020.116041
- Faegh M, Ghungrad S, Oliveira JP, Rao P, Haghighi A. A review on physics-informed machine learning for process-structure-property modeling in additive manufacturing. J Manuf Processes. 2025;133:524-555. doi: 10.1016/j.jmapro.2024.11.066
- Hu E, Seetoh IP, Lai CQ. Machine learning assisted investigation of defect influence on the mechanical properties of additively manufactured architected materials. Int J Mech Sci. 2022;221:107190. doi: 10.1016/j.ijmecsci.2022.107190
- Samadiani N, Barnard AS, Gunasegaram D, Fayyazifar N. Best practices for machine learning strategies aimed at process parameter development in powder bed fusion additive manufacturing. J Intell Manuf. 2024;1-41. doi: 10.1007/s10845-024-02490-4
- Shevchik SA, Masinelli G, Kenel C, Leinenbach C, Wasmer K. Deep learning for in situ and real-time quality monitoring in additive manufacturing using acoustic emission. IEEE Transact Ind Inform. 2019;15(9):5194-5203. doi: 10.1109/TII.2019.2910524
- Garland AP, White BC, Jensen SC, Boyce BL. Pragmatic generative optimization of novel structural lattice metamaterials with machine learning. Mater Design. 2021;203:109632. doi: 10.1016/j.matdes.2021.109632
- Wang Z, Yang W, Liu Q, et al. Data-driven modeling of process, structure and property in additive manufacturing: A review and future directions. J Manuf Processes. 2022;77:13-31. doi: 10.1016/j.jmapro.2022.02.053
- Alexander Z, Chau DH, Saldaña C. An interrogative survey of explainable AI in manufacturing. IEEE Transact Ind Inform. 2024;99:1-13. doi: 10.1109/TII.2024.3361489
- Zhang Y, Safdar M, Xie J, Li J, Sage M, Zhao YF. A systematic review on data of additive manufacturing for machine learning applications: The data quality, type, preprocessing, and management. J Intell Manuf. 2023;34(8):3305-3340. doi: 10.1007/s10845-022-02017-9
- Qi X, Chen G, Li Y, Cheng X, Li C. Applying neural-network-based machine learning to additive manufacturing: Current applications, challenges, and future perspectives. Engineering. 2019;5(4):721-729. doi: 10.1016/j.eng.2019.04.012
- Valizadeh M, Wolff SJ. Convolutional neural network applications in additive manufacturing: A review. Adv Ind Manuf Eng. 2022;4:100072. doi: 10.1016/j.aime.2022.100072
- Joshi M, Flood A, Sparks T, Liou FW. Applications of Supervised Machine Learning Algorithms in Additive Manufacturing: A Review. Austin: University of Texas at Austin; 2019.
- Guo S, Agarwal M, Cooper C, et al. Machine learning for metal additive manufacturing: Towards a physics-informed data-driven paradigm. J Manuf Syst. 2022;62:145-163. doi: 10.1016/j.jmsy.2021.11.003
- Gu GX, Chen CT, Richmond DJ, Buehler MJ. Bioinspired hierarchical composite design using machine learning: Simulation, additive manufacturing, and experiment. Mater Horiz. 2018;5(5):939-945.
- Chen L, Bi G, Yao X, et al. In-situ process monitoring and adaptive quality enhancement in laser additive manufacturing: A critical review. J Manuf Syst. 2024;74:527-574. doi: 10.1016/j.jmsy.2024.04.013
- Oleff A, Küster B, Stonis M, Overmeyer L. Process monitoring for material extrusion additive manufacturing: A state-of-the-art review. Prog Addit Manuf. 2021;6:705-730. doi: 10.1007/s40964-021-00192-4
- Abdelhamid Z, Mohamed H, Kelouwani S. The use of machine learning in process-structure–property modeling for material extrusion additive manufacturing: A state-of-the-art review. J Braz Soc Mech Sci Eng. 2024;46(2):70. doi: 10.1007/s40430-023-04637-5
- Toprak CB, Dogruer C. A critical review of machine learning methods used in metal powder bed fusion process to predict part properties. Int J Precis Eng Manuf. 2024;25(2):429-452. doi: 10.1007/s12541-023-00905-5
- Zhao T, Yan Z, Zhang B, et al. A comprehensive review of process planning and trajectory optimization in arc-based directed energy deposition. J Manuf Processes. 2024;119:235-254. doi: 10.1016/j.jmapro.2024.03.093
- Espino MT, Tuazon BJ, Espera AH Jr., et al. Statistical methods for design and testing of 3D-printed polymers. MRS Commun. 2023;13(2):193-211. doi: 10.1557/s43579-023-00332-7
- Yehia HM, Hamada A, Sebaey TA, Abd-Elaziem W. Selective laser sintering of polymers: Process parameters, machine learning approaches, and future directions. J Manuf Mater Process. 2024;8(5):197. doi: 10.3390/jmmp8050197
- Geng S, Luo Q, Liu K, Li Y, Hou Y, Long W. Research status and prospect of machine learning in construction 3D printing. Case Stud Construct Mater. 2023;18:e01952. doi: 10.1016/j.cscm.2023.e01952
- Yu Q, Zhang M, Mujumdar AS, Li J. AI-based additive manufacturing for future food: Potential applications, challenges and possible solutions. Innov Food Sci Emerg Technol. 2024:103599. doi: 10.1016/j.ifset.2024.103599
- Herzog T, Brandt M, Trinchi A, Sola A, Molotnikov A. Process monitoring and machine learning for defect detection in laser-based metal additive manufacturing. J Intell Manuf. 2024;35(4):1407-1437. doi: 10.1007/s10845-023-02119-y
- Gunasegaram D, Barnard A, Matthews M, et al. Machine learning-assisted in-situ adaptive strategies for the control of defects and anomalies in metal additive manufacturing. Addit Manuf. 2024;81:104013. doi: 10.1016/j.addma.2024.104013
- Huu PN, Van DP, Xuan TH, et al. Enhancing additive digital manufacturing with supervised classification machine learning algorithms. Int J Adv Manuf Technol. 2024;133:1-17. doi: 10.1007/s00170-024-13781-8
- Pant R, Singh R, Gehlot A, Akram SV, Gupta LR, Thakur AK. A systematic review of additive manufacturing solutions using machine learning, internet of things, big data, digital twins and blockchain technologies: A technological perspective towards sustainability. Arch Comput Methods Eng. 2024;31:1-16. doi: 10.1007/s11831-024-10116-4
- Ukwaththa J, Herath S, Meddage D. A review of machine learning (ML) and explainable artificial intelligence (XAI) methods in additive manufacturing (3D printing). Mater Today Commun. 2024;41:110294. doi: 10.1016/j.mtcomm.2024.110294
- Farrag A, Yang Y, Cao N, Won D, Jin Y. Physics-informed machine learning for metal additive manufacturing. Prog Addit Manuf. 2024;10:110008. doi: 10.1007/s40964-024-00612-1
- Castillo M, Monroy R, Ahmad R. Scientometric analysis and systematic review of smart manufacturing technologies applied to the 3D printing polymer material extrusion system. J Intell Manuf. 2024;35(1):3-33. doi: 10.1007/s10845-022-02049-1
- Moradi A, Tajalli S, Mosallanejad MH, Saboori A. Intelligent laser-based metal additive manufacturing: A review on machine learning for process optimization and property prediction. Int J Adv Manuf Technol. 2024;136:527-560. doi: 10.1007/s00170-024-14858-0
- Su J, Jiang F, Teng J, et al. Recent innovations in laser additive manufacturing of titanium alloys. Int J Extrem Manuf. 2024;6(3):032001. doi: 10.1088/2631-7990/ad2545
- Hassan M, Misra M, Taylor GW, Mohanty AK. A review of AI for optimization of 3D printing of sustainable polymers and composites. Compos Part C Open Access. 2024;15:100513. doi: 10.1016/j.jcomc.2024.100513
- Bin Abu Sofian ADA, Lim HR, Chew KW, Show PL. Advancing 3D printing through integration of machine learning with algae‐based biopolymers. ChemBioEng Rev. 2024;11(2):406-425. doi: 10.1002/cben.202300054
- Karimzadeh M, Basvoju D, Vakanski A, Charit I, Xu F, Zhang X. Machine learning for additive manufacturing of functionally graded materials. Materials (Basel). 2024;17(15):3673. doi: 10.3390/ma17153673
- Garg A, Sharma A, Zheng W, Li L. A review on artificial intelligence-enabled mechanical analysis of 3D printed and FEM-modelled auxetic metamaterials. Virtual Phys Prototyp. 2025;20(1):e2445712. doi: 10.1080/17452759.2024.2445712
- Nam J, Kim M. Advances in materials and technologies for digital light processing 3D printing. Nano Converg. 2024;11(1):45. doi: 10.1186/s40580-024-00452-3
- Yuan K, Xu Y, Wang X, Ma X, Wang Q, Zhang H. Key technologies and research progress in robotic arc additive remanufacturing. Sens Actuators A Phys. 2024;376:115547. doi: 10.1016/j.sna.2024.115547
- Selot A, Dwivedi R. Machine learning and sensor-based approach for defect detection in MEX additive manufacturing process-a review. J Braz Soc Mech Sci Eng. 2023;45(10):535. doi: 10.1007/s40430-023-04425-1
- Saboori A, Aversa A, Marchese G, Biamino S, Lombardi M, Fino P. Application of directed energy deposition-based additive manufacturing in repair. Appl Sci. 2019;9(16):3316. doi: 10.3390/app9163316
- Liu WW, Tang ZJ, Liu XY, Wang HJ, Zhang HC. A review on in-situ monitoring and adaptive control technology for laser cladding remanufacturing. Proced Cirp. 2017;61:235-240. doi: 10.1016/j.procir.2016.11.217
- Inayathullah S, Buddala R. Review of machine learning applications in additive manufacturing. Results Eng. 2024;25:103676. doi: 10.1016/j.rineng.2024.103676
- Tapia G, Khairallah S, Matthews M, King WE, Elwany A. Gaussian process-based surrogate modeling framework for process planning in laser powder-bed fusion additive manufacturing of 316L stainless steel. Int J Adv Manuf Technol. 2018;94(9):3591-3603. doi: 10.1007/s00170-017-1045-z
- Kamath C, Fan YJ. Regression with small data sets: A case study using code surrogates in additive manufacturing. Knowl Inf Syst. 2018;57:475-493. doi: 10.1007/s10115-018-1174-1
- Kumar HA, Kumaraguru S, Paul C, Bindra K. Faster temperature prediction in the powder bed fusion process through the development of a surrogate model. Optics Laser Technol. 2021;141:107122. doi: 10.1016/j.optlastec.2021.107122
- Chen L, Bi G, Yao X, et al. Multisensor fusion-based digital twin for localized quality prediction in robotic laser-directed energy deposition. Robot Comput Integr Manuf. 2023;84:102581. doi: 10.1016/j.rcim.2023.102581
- Huang X, Xie T, Wang Z, Chen L, Zhou Q, Hu Z. A transfer learning-based multi-fidelity point-cloud neural network approach for melt pool modeling in additive manufacturing. ASCE ASME J Risk Uncertain Eng Syst Part B Mech Eng. 2022;8(1):011104. doi: 10.1115/1.4051749
- Wang Y, Zheng P, Xu X, Yang H, Zou J. Production planning for cloud-based additive manufacturing-a computer vision-based approach. Robot Comput Integr Manuf. 2019;58:145-157. doi: 10.1016/j.rcim.2019.03.003
- Safdar M, Paul PP, Lamouche G, et al. Fundamental requirements of a machine learning operations platform for industrial metal additive manufacturing. Comput Ind. 2024;154:104037. doi: 10.1016/j.compind.2023.104037
- Su J, Ng WL, An J, Yeong WY, Chua CK, Sing SL. Achieving sustainability by additive manufacturing: A state-of-the-art review and perspectives. Virtual Phys Prototyp. 2024;19(1):e2438899. doi: 10.1080/17452759.2024.2438899
- Zhao YF, Xie J, Sun L. On the data quality and imbalance in machine learning-based design and manufacturing-a systematic review. Engineering. 2024;45:105-131. doi: 10.1016/j.eng.2024.04.024
- Fullington D, Yangue E, Bappy MM, Liu C, Tian W. Leveraging small-scale datasets for additive manufacturing process modeling and part certification: Current practice and remaining gaps. J Manuf Syst. 2024;75:306-321. doi: 10.1016/j.jmsy.2024.04.021
- Xie H, Hoskins D, Rowe K, Ju F. Transformer-based offline printing strategy design for large format additive manufacturing. J Comput Inf Sci Eng. 2024;25:021011.
- Zhu Q, Liu Z, Yan J. Machine learning for metal additive manufacturing: Predicting temperature and melt pool fluid dynamics using physics-informed neural networks. Comput Mech. 2021;67:619-635. doi: 10.1007/s00466-020-01952-9
- Li R, Jin M, Paquit VC. Geometrical defect detection for additive manufacturing with machine learning models. Mater Design. 2021;206:109726. doi: 10.1016/j.matdes.2021.109726
- Zhan Z, Li H. A novel approach based on the elastoplastic fatigue damage and machine learning models for life prediction of aerospace alloy parts fabricated by additive manufacturing. Int J Fatigue. 2021;145:106089. doi: 10.1016/j.ijfatigue.2020.106089
- Ko H, Lu Y, Yang Z, Ndiaye NY, Witherell P. A framework driven by physics-guided machine learning for process-structure-property causal analytics in additive manufacturing. J Manuf Syst. 2023;67:213-228. doi: 10.1016/j.jmsy.2022.09.010
- Lu Y, Xie J, Safdar M, et al. An Overarching Quality Evaluation Framework for Additive Manufacturing Digital Twin. United States: IEEE; 2024. p. 676-682.
- Liu B, Zhang T, Yu Y, Miao L. A data generation method with dual discriminators and regularization for surface defect detection under limited data. Comput Ind. 2023;151:103963. doi: 10.1016/j.compind.2023.103963
- Safdar M, Lamouche G, Paul PP, Wood G, Zhao YF. Feature engineering in additive manufacturing. In: Engineering of Additive Manufacturing Features for Data-Driven Solutions: Sources, Techniques, Pipelines, and Applications. Germany: Springer; 2023. p. 17-43.
- Dharmadhikari S, Menon N, Basak A. A reinforcement learning approach for process parameter optimization in additive manufacturing. Addit Manuf. 2023;71:103556. doi: 10.1016/j.addma.2023.103556
- Ogoke F, Farimani AB. Thermal control of laser powder bed fusion using deep reinforcement learning. Addit Manuf. 2021;46:102033. doi: 10.1016/j.addma.2021.102033
- Li C, Pan Y, Shi Y, Wang W. Optimization of process prediction models for hot-wire laser metal deposition using transfer learning strategies based on simulation datasets. In: Welding in the World. Germany: Springer; 2025. p. 1-13.
- Cheng L, Jiang Z, Wang H, et al. Low-rank adaptive transfer learning based for multi-label defect detection in laser powder bed fusion. Opt Lasers Eng. 2025;184:108683. doi: 10.1016/j.optlaseng.2024.108683
- Wu H, Fan ZM, Gan L. Feature transfer learning for fatigue life prediction of additive manufactured metals with small samples. Fatigue Fract Eng Mater Struct. 2024;48:467-486. doi: 10.1111/ffe.14497
- Kharate N, Anerao P, Kulkarni A, Abdullah M. Explainable AI techniques for comprehensive analysis of the relationship between process parameters and material properties in FDM-Based 3D-printed biocomposites. J Manuf Mater Process. 2024;8(4):171. doi: 10.3390/jmmp8040171
- Drakoulas G, Gortsas T, Polyzos E, Tsinopoulos S, Pyl L, Polyzos D. An explainable machine learning-based probabilistic framework for the design of scaffolds in bone tissue engineering. Biomech Model Mechanobiol. 2024;23:987-1012. doi: 10.1007/s10237-024-01817-7
- Yoo YJ. Thermal imaging-based diagnostic process using explainable artificial intelligence for 3D printing system. Soft Comput. 2024;28:1-12. doi: 10.1007/s00500-023-09530-w
- Zhu Y, Wu X, Gotawala N, Higdon DM, Hang ZY. Thermal prediction of additive friction stir deposition through Bayesian learning-enabled explainable artificial intelligence. J Manuf Syst. 2024;72:1-15. doi: 10.1016/j.jmsy.2023.10.015
- Scime L, Beuth J. A multi-scale convolutional neural network for autonomous anomaly detection and classification in a laser powder bed fusion additive manufacturing process. Add Manuf. 2018;24:273-286.
- Shevchik SA, Kenel C, Leinenbach C, Wasmer K. Acoustic emission for in situ quality monitoring in additive manufacturing using spectral convolutional neural networks. Addit Manuf. 2018;21:598-604. doi: 10.1016/j.addma.2017.11.012
- Mozaffar M, Paul A, Al-Bahrani R, et al. Data-driven prediction of the high-dimensional thermal history in directed energy deposition processes via recurrent neural networks. Manuf Lett. 2018;18:35-39. doi: 10.1016/j.mfglet.2018.10.002
- Zhang SU. Degradation classification of 3D printing thermoplastics using fourier transform infrared spectroscopy and artificial neural networks. Appl Sci. 2018;8(8):1224. doi: 10.3390/app8081224
- Lee XY, Saha SK, Sarkar S, Giera B. Automated detection of part quality during two-photon lithography via deep learning. Addit Manuf. 2020;36:101444. doi: 10.1016/j.addma.2020.101444
- Wang Y, Du W, Wang H, Zhao Y. Intelligent generation method of innovative structures based on topology optimization and deep learning. Materials (Basel). 2021;14(24):7680. doi: 10.3390/ma14247680
- Zheng X, Chen TT, Guo X, Samitsu S, Watanabe I. Controllable inverse design of auxetic metamaterials using deep learning. Mater Design. 2021;211:110178. doi: 10.1016/j.matdes.2021.110178
- Challapalli A, Konlan J, Patel D, Li G. Discovery of cellular unit cells with high natural frequency and energy absorption capabilities by an inverse machine learning framework. Front Mech Eng. 2021;7:779098. doi: 10.3389/fmech.2021.779098
- Shim BS, Hou JU. Improving estimation of layer thickness and identification of slicer for 3D printing forensics. Sensors. 2023;23(19):8250. doi: 10.3390/s23198250
- Wang K, Xu J, Zhang S, Tan J. Economically evaluating energy efficiency performance in fused filament fabrication using a multi-scale hierarchical transformer. Int J Adv Manuf Technol. 2023;128(1-2):329-343. doi: 10.1007/s00170-023-11553-4
- Yang Z, Hsu Y-C, Buehler MJ. Generative multiscale analysis of de novo proteome-inspired molecular structures and nanomechanical optimization using a VoxelPerceiver transformer model. J Mech Phys Solids. 2023;170:105098. doi: 10.1016/j.jmps.2022.105098
- Fernandez-Zelaia P, Dryepondt SN, Ziabari AK, Kirka MM. Self-supervised learning of spatiotemporal thermal signatures in additive manufacturing using reduced order physics models and transformers. Comput Mater Sci. 2024;232:112603. doi: 10.2139/ssrn.4325464
- Badini S, Regondi S, Frontoni E, Pugliese R. Assessing the capabilities of ChatGPT to improve additive manufacturing troubleshooting. Adv Ind Eng Polym Res. 2023;6(3):278-287. doi: 10.1016/j.aiepr.2023.03.003
- Chandrasekhar A, Chan J, Ogoke F, Ajenifujah O, Farimani AB. AMGPT: A Large Language Model for Contextual Querying in Additive Manufacturing. [arXiv Preprint arXiv:240600031]; 2024
- Fan H, Zhang H, Ma C, Wu T, Fuh JYH, Li B. Enhancing metal additive manufacturing training with the advanced vision language model: A pathway to immersive augmented reality training for non-experts. J Manuf Syst. 2024;75:257-269. doi: 10.1016/j.jmsy.2024.06.007
- Olleak A, Xi Z. Calibration and validation framework for selective laser melting process based on multi-fidelity models and limited experiment data. J Mech Design. 2020;142(8):081701. doi: 10.1115/1.4045744
- Pandiyan V, Drissi-Daoudi R, Shevchik S, et al. Deep transfer learning of additive manufacturing mechanisms across materials in metal-based laser powder bed fusion process. J Mater Process Technol. 2022;303:117531. doi: 10.1016/j.jmatprotec.2022.117531
- Li J, Hu J, Zhou Q, Zhang Y. Transfer learning-based quality monitoring of laser powder bed fusion across materials. Expert Syst Appl. 2024;252:124150. doi: 10.1016/j.eswa.2024.124150
- Safdar M, Xie J, Ko H, Lu Y, Lamouche G, Zhao YF. Transferability analysis of data-driven additive manufacturing knowledge: A case study between powder bed fusion and directed energy deposition. J Comput Inf Sci Eng. 2024;24(5):051010. doi: 10.1115/1.4065090
- Xie J, Safdar M, Chen L, Moon SK, Zhao YF. Audio-visual cross-modality knowledge transfer for machine learning-based in-situ monitoring in laser additive manufacturing. Addit Manuf. 2025;101:104692. doi: 10.1016/j.addma.2025.104692
- Raihan AS, Khosravi H, Bhuiyan TH, Ahmed I. An augmented surprise-guided sequential learning framework for predicting the melt pool geometry. J Manuf Syst. 2024;75:56-77. doi: 10.1016/j.jmsy.2024.05.023
- Van Houtum GJ, Vlasea ML. Active learning via adaptive weighted uncertainty sampling applied to additive manufacturing. Addit Manuf. 2021;48:102411. doi: 10.1016/j.addma.2021.102411
- Wong VWH, Ferguson M, Law KH, Lee YTT, Witherell P. Segmentation of additive manufacturing defects using U-Net. J Comput Inf Sci Eng. 2022;22(3):1-44. doi: 10.1115/1.4053078
- Kim Y, Lee T, Hyun Y, et al. Self-supervised representation learning anomaly detection methodology based on boosting algorithms enhanced by data augmentation using StyleGAN for manufacturing imbalanced data. Comput Ind. 2023;153:104024. doi: 10.1016/j.compind.2023.104024
- Zhu Z, Ferreira K, Anwer N, Mathieu L, Guo K, Qiao L. Convolutional neural network for geometric deviation prediction in additive manufacturing. Proced Cirp. 2020;91:534-539.
- Tian Q, Guo S, Melder E, Bian L, Guo WG. Deep learning-based data fusion method for in situ porosity detection in laser-based additive manufacturing. J Manuf Sci Eng. 2021;143(4):041011. doi: 10.1115/1.4048957
- Houser E, Shashaani S, Harrysson O, Jeon Y. Predicting additive manufacturing defects with robust feature selection for imbalanced data. IISE Transact. 2024;56(9):1001-1019. doi: 10.1080/24725854.2023.2207633
- Li Y, Shi Z, Liu C, Tian W, Kong Z, Williams CB. Augmented time regularized generative adversarial network (atr-gan) for data augmentation in online process anomaly detection. IEEE Transact Autom Sci Eng. 2021;19(4):3338-3355. doi: 10.1109/TASE.2021.3118635
- Fan SKS, Tsai DM, Yeh PC. Effective variational-autoencoder-based generative models for highly imbalanced fault detection data in semiconductor manufacturing. IEEE Transact on Semicond Manuf. 2023;36(2):205-214. doi: 10.1109/TSM.2023.3238555
- Yangue E, Fullington D, Smith O, Tian W, Liu C. Diffusion generative model-based learning for smart layer-wise monitoring of additive manufacturing. J Comput Inf Sci Eng. 2024;24(6):060903. doi: 10.1115/1.4065092
- Zheng F, Xie L, Bai Q, et al. Semi-supervised learning for laser directed energy deposition monitoring via co-axial dynamic imaging. Addit Manuf. 2025;97:104628.
- Shi N, Kontar RA. Personalized federated learning via domain adaptation with an application to distributed 3D printing. Technometrics. 2023;65(3):328-339. doi: 10.1080/00401706.2022.2157882
- Lei R, Guo Y, Yan J, Guo W. Bridging data gaps: A federated learning approach to heat emission prediction in laser powder bed fusion. J Manuf Sci Eng. 2024;146(10):1-48. doi: 10.1115/1.4065888
- Li Y, Mojumder S, Lu Y, et al. Statistical parameterized physics-based machine learning digital shadow models for laser powder bed fusion process. Addit Manuf. 2024;87:104214. doi: 10.1016/j.addma.2024.104214
- Uhrich B, Pfeifer N, Schäfer M, Theile O, Rahm E. Physics-informed deep learning to quantify anomalies for real-time fault mitigation in 3D printing. Appl Intell. 2024;54(6):4736-4755. doi: 10.1007/s10489-024-05402-4
- Shi T, Ma M, Wu J, Post C, Charles E, Schmitz T. AFSD-Physics: Exploring the Governing Equations of Temperature Evolution During Additive Friction Stir deposition By a Human-AI Teaming Approach. [arXiv Preprint arXiv:240116501]; 2024.
- Peng B, Panesar A. Multi-layer thermal simulation using physics-informed neural network. Addit Manuf. 2024;95:104498. doi: 10.1016/j.addma.2024.104498
- Kim J, Yang Z, Lu Y. A physics-guided deep generative model for predicting melt pool behavior in laser powder bed fusion additive manufacturing. J Intell Manuf. 2024:1-21. doi: 10.1007/s10845-024-02504-1
- Sajadi P, Rahmani Dehaghani M, Tang Y, Wang GG. Physics-informed online learning for temperature prediction in metal AM. Materials (Basel). 2024;17(13):3306. doi: 10.3390/ma17133306
- Whitney BC, Spangenberger AG, Rodgers TM, Lados DA. Part-scale microstructure prediction for laser powder bed fusion Ti-6Al-4V using a hybrid mechanistic and machine learning model. Addit Manuf. 2024;94:104500. doi: 10.1016/j.addma.2024.104500
- Chen L, Yang F, Wang R, Zhang Y, Diao Z, Rong M. Optical spectral physics-informed attention network for condition monitoring in WAAM. IEEE Transact Ind Electron. 2023;71:9708-9718. doi: 10.1109/TIE.2023.3325570
- Yu H, Hu Y, Kang G, Peng X, Chen B, Wu S. High-cycle fatigue life prediction of L-PBF AlSi10Mg alloys: A domain knowledge-guided symbolic regression approach. Philos Trans A Math Phys Eng Sci. 2024;382(2264):20220383. doi: 10.1098/rsta.2022.0383
- Li J, Zhou Q, Huang X, Li M, Cao L. In situ quality inspection with layer-wise visual images based on deep transfer learning during selective laser melting. J Intell Manuf. 2023;34:853-867. doi: 10.1007/s10845-021-01829-5
- Zhu X, Jiang F, Guo C, Xu D, Wang Z, Jiang G. Surface morphology inspection for directed energy deposition using small dataset with transfer learning. J Manuf Processes. 2023;93:101-115. doi: 10.1016/j.jmapro.2023.03.016
- Safdar M, Xie J, Ko H, Lu Y, Lamouche G, Zhao YF. Transferability analysis of data-driven additive manufacturing knowledge: A case study between powder bed fusion and directed energy deposition. Am Soc Mech Eng. 2023:V002T02A078. doi: 10.1115/DETC2023-116458
- Wang Z, Dai Z, Póczos B, Carbonell J. Characterizing and Avoiding Negative Transfer. In: 2019 IEEE/CVF Conference on Computer Vision and Pattern Recognition (CVPR); 2019. p. 11293-11302.
- Safdar M, Lamouche G, Paul PP, Wood G, Zhao YF. Engineering of Additive Manufacturing Features for Data- Driven Solutions: Sources, Techniques, Pipelines, and Applications. Germany: Springer Nature; 2023.
- Xie J, Sage M, Zhao YF. Feature selection and feature learning in machine learning applications for gas turbines: A review. Eng Appl Artif Intell. 2023;117:105591. doi: 10.1016/j.engappai.2022.105591
- Becker P, Roth C, Roennau A, Dillmann R. Acoustic Anomaly Detection in Additive Manufacturing with Long Short-term Memory Neural Networks. United States: IEEE; 2020. p. 921-926.
- Figueira A, Vaz B. Survey on synthetic data generation, evaluation methods and GANs. Mathematics. 2022; 10(15):2733. doi: 10.3390/math10152733
- Gui J, Chen T, Zhang J, et al. A survey on self-supervised learning: Algorithms, applications, and future trends. IEEE Transact Pattern Analy Mach Intell. 2024;46:9052-9071. doi: 10.1109/TPAMI.2024.3415112
- Li L, Fan Y, Tse M, Lin KY. A review of applications in federated learning. Comput Ind Eng. 2020;149:106854. doi: 10.1016/j.cie.2020.106854
- Wang H, Li B, Lei L, Xuan F. Uncertainty-aware fatigue-life prediction of additively manufactured Hastelloy X superalloy using a physics-informed probabilistic neural network. Reliabil Eng Syst Saf. 2024;243:109852. doi: 10.1016/j.ress.2023.109852
- Iyer N, Mirzendehdel AM, Raghavan S, et al. PATO: Producibility-aware topology optimization using deep learning for metal additive manufacturing. Int J Interact Design Manuf (IJIDeM). 2024;18:7459-7476. doi: 10.1007/s12008-024-01905-z
- Zhang S, Yang H, Yang Z, Lu Y. Engineering-guided deep learning of melt-pool dynamics for additive manufacturing quality monitoring. J Comput Inf Sci Eng. 2024;24(10):1-17. doi: 10.1115/1.4066026
- Cao X, Duan C, Luo X, et al. Physics-informed machine learning approach for molten pool morphology prediction and process evaluation in directed energy deposition of 12CrNi2 alloy steel. J Manuf Processes. 2024;119:806-826. doi: 10.1016/j.jmapro.2024.04.023
- Zhu Q, Lu Z, Hu Y. A reality-augmented adaptive physics informed machine learning method for efficient heat transfer prediction in laser melting. J Manuf Processes. 2024;124:444-457. doi: 10.1016/j.jmapro.2024.06.010
- Qin J, Taraphdar P, Sun Y, et al. Knowledge-based bidirectional thermal variable modelling for directed energy deposition additive manufacturing. Virtual Phys Prototyp. 2024;19(1):e2397008. doi: 10.1080/17452759.2024.2397008
- Von Rueden L, Mayer S, Beckh K, et al. Informed machine learning-a taxonomy and survey of integrating prior knowledge into learning systems. IEEE Transact Knowl Data Eng. 2021;35(1):614-633. doi: 10.1109/tkde.2021.3079836
- Zhang P, Zhou X, Ma H, et al. Anomaly detection in laser metal deposition with photodiode-based melt pool monitoring system. Opt Laser Technol. 2021;144:107454. doi: 10.1016/j.optlastec.2021.107454
- Cherif L, Safdar M, Lamouche G, et al. Evaluation of key spatiotemporal learners for print track anomaly classification using melt pool image streams. IFAC PapersOnline. 2023;56(2):4733-4739. doi: 10.48550/arXiv.2308.14861
- Snow Z, Diehl B, Reutzel EW, Nassar A. Toward in-situ flaw detection in laser powder bed fusion additive manufacturing through layerwise imagery and machine learning. J Manuf Syst. 2021;59:12-26. doi: 10.1016/j.jmsy.2021.01.008
- Bommasani R, Hudson DA, Adeli E, et al. On the Opportunities and Risks of Foundation Models. [arXiv Preprint arXiv:210807258]; 2021.
- Beltagy I, Lo K, Cohan A. SciBERT: A Pretrained Language model for scientific Text. [arXiv Preprint arXiv: 190310676]; 2019.
- Manzari ON, Ahmadabadi H, Kashiani H, Shokouhi SB, Ayatollahi A. MedViT: A robust vision transformer for generalized medical image classification. Comput Biol Med. 2023;157:106791. doi: 10.1016/j.compbiomed.2023.106791
- Han Z, Gao C, Liu J, Zhang J, Zhang SQ. Parameter-Efficient Fine-Tuning for Large Models: A Comprehensive Survey. [arXiv Preprint arXiv:240314608]; 2024.
- Cerezo M, Verdon G, Huang HY, Cincio L, Coles PJ. Challenges and opportunities in quantum machine learning. Nat Comput Sci. 2022;2(9):567-576. doi: 10.1038/s43588-022-00311-3
- Kim J, Chang S, Kwak N. PQK: Model Compression Via Pruning, Quantization, and Knowledge Distillation. [arXiv Preprint arXiv:210614681]; 2021.
- Shi W, Cao J, Zhang Q, Li Y, Xu L. Edge computing: Vision and challenges. IEEE Internet Things J. 2016;3(5):637-646. doi: 10.1109/JIOT.2016.2579198
- Răileanu S, Anton F, Borangiu T, Anton S, Nicolae M. A cloud-based manufacturing control system with data integration from multiple autonomous agents. Comput Ind. 2018;102:50-61.
- Leng J, Li R, Xie J, et al. Federated learning-empowered smart manufacturing and product lifecycle management: A review. Adv Eng Inform. 2025;65:103179. doi: 10.1016/j.aei.2025.103179
- An H, Youn BD, Kim HS. Optimal placement of non-redundant sensors for structural health monitoring under model uncertainty and measurement noise. Measurement. 2022;204:112102. doi: 10.1016/j.measurement.2022.112102
- Xie J, Safdar M, Chen L, Moon SK, Zhao YF. Audio-Visual Cross-Modality Knowledge Transfer for Machine Learning-based in-situ Monitoring in Laser Additive Manufacturing. [arXiv Preprint arXiv:240805307]; 2024.
- Baker M. Reproducibility crisis. Nature. 2016;533(26): 353-66.
- Xie J, Safdar M, Romascanu AM, et al. Towards reproducible machine learning-based process monitoring and quality prediction research for additive manufacturing. Am Soc Mech Eng. 2024:V02AT02A033.
- Xie J, Yang Z, Hu CC, Yang HC, Lu Y, Zhao YF. Investigation on Domain Adaptation of Additive Manufacturing Monitoring Systems to Enhance Digital Twin Reusability. United States: IEEE; 2024. p. 690-697. doi: 10.48550/arXiv.2409.12785
- Zemskov AD, Fu Y, Li R, et al. Security and Privacy of Digital Twins for Advanced Manufacturing: A Survey. [arXiv Preprint arXiv:241213939]; 2024.