Mapping breast cancer protein interaction networks as metric spaces: Insights into central zones and drug discovery targets
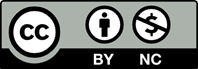
Graph theory was employed in recent advances of cancer research for gain deeper insights into the complex structure and function of protein-protein interaction (PPI) networks. By representing proteins as nodes and their interactions as edges, graph theory offers a comprehensive framework for analyzing the topological properties of these networks and identifying key nodes that regulate critical biological processes. This approach has been widely applied to study various cancers, including breast cancer. To investigate the molecular organization and critical pathways in breast cancer, we constructed a breast cancer protein-protein interaction network (BCPIN) and analyzed its hierarchical structure. The network was modeled as a metric space to delineate its central zones, facilitating the identification of essential hubs enriched with signaling pathways critical for cancer progression. Our study demonstrates the potential of hierarchical modeling of the BCPIN in unraveling its molecular organization and identifying therapeutic opportunities. By analyzing PPI network as a metric space, we highlight central zones 1 – 3 as critical hubs enriched with key signaling pathways, such as DNA repair, Notch signaling, and p53 signaling, which are essential to cancer progression. The identification of MAPK14 as a central node emphasizes its significant role in cancer biology and its value as a therapeutic target. The predominance of signaling proteins within these zones underscores their functional relevance, offering a strong rationale for prioritizing them in drug development. By modeling the PPI network as a metric space, we uncovered important insights into its architecture and the central zone’s critical role in facilitating key cellular processes. Our results indicate that zones 1 – 3, particularly the central zone, may serve as promising targets for drug discovery in cancer biology.
- Siegel RL, Miller KD, Wagle NS, Jemal A. Cancer statistics, 2023. CA Cancer J Clin. 2023;73(1):17-48.
- Hanahan D, Weinberg RA. Hallmarks of cancer: The next generation. Cell. 2011;144(5):646-674. doi: 10.1016/j.cell.2011.02.013
- Barabási AL, Oltvai ZN. Network biology: Understanding the cell’s functional organization. Nat Rev Genet. 2004;5(2): 101-113. doi: 10.1038/nrg1272
- Pavlopoulos GA, Secrier M, Moschopoulos CN, et al. Using graph theory to analyze biological networks. BioData Min. 2011;4:10. doi: 10.1186/1756-0381-4-10
- Zhang Y, Xiang J, Tang L, et al. Identifying breast cancer-related genes based on a novel computational framework involving KEGG pathways and PPI network modularity. Front Genet. 2021;12:596794. doi: 10.3389/fgene.2021.596794
- Shi K, Li Y, Yang M, Li W. Identification of key genes and pathways in female lung cancer patients who never smoked by a bioinformatics analysis. J Cancer. 2019;10(1):51-59. doi: 10.7150/jca.26908
- Liu QQ, Ren K, Liu SH, Li WM, Huang CJ, Yang XH. MicroRNA-140-5p aggravates hypertension and oxidative stress of atherosclerosis via targeting Nrf2 and Sirt2. Int J Mol Med. 2019;43(2):839-849. doi: 10.3892/ijmm.2018.3996.
- Wang E, Zaman N, Mcgee S, Milanese JS, Masoudi-Nejad A, O’Connor-McCourt M. Predictive genomics: A cancer hallmark network framework for predicting tumor clinical phenotypes using genome sequencing data. Semin Cancer Biol. 2015;30:4-12. doi: 10.1016/j.semcancer.2014.04.002
- Chen J, Riazifar H, Guan MX, Huang T. Modeling autosomal dominant optic atrophy using induced pluripotent stem cells and identifying potential therapeutic targets. Stem Cell Res Ther. 2016;7:2. doi: 10.1186/s13287-015-0264-1
- Goh KI, Cusick ME, Valle D, Childs B, Vidal M, Barabási AL. The human disease network. Proc Natl Acad Sci U S A. 2007;104(21):8685-8690. doi: 10.1073/pnas.0701361104
- Ideker T, Sharan R. Protein networks in disease. Genome Res. 2008;18(4):644-652. doi: 10.1101/gr.071852.107
- Cowen L, Ideker T, Raphael BJ, Sharan R. Network propagation: A universal amplifier of genetic associations. Nat Rev Genet. 2017;18(9):551-562. doi: 10.1038/nrg.2017.38
- Csermely P, Korcsmáros T, Kiss HJ, London G, Nussinov R. Structure and dynamics of molecular networks: A novel paradigm of drug discovery: A comprehensive review. Pharmacol Ther. 2013;138(3):333-408. doi: 10.1016/j.pharmthera.2013.01.016
- Menche J, Sharma A, Kitsak M, et al. Uncovering disease-disease relationships through the incomplete interactome. Science. 2015;347(6224):1257601. doi: 10.1126/science.1257601
- Borgatti SP, Everett MG. A graph-theoretic perspective on centrality. Soc Netw. 2006;28(4):466-484.
- Jeong H, Mason SP, Barabási AL, Oltvai ZN. Lethality and centrality in protein networks. Nature. 2001;411(6833):41-42. doi: 10.1038/35075138
- Network YI. High-quality binary protein interaction map of the. Science. 2008;1158684(104):322. doi: 10.1126/science.1158684
- Batada NN, Hurst LD, Tyers M. Evolutionary and physiological importance of hub proteins. PLoS Comput Biol. 2006;2(7):e88. doi: 10.1371/journal.pcbi.0020088
- Fadhal E, Gamieldien J, Mwambene EC. Protein interaction networks as metric spaces: A novel perspective on distribution of hubs. BMC Syst Biol. 2014;8:6. doi: 10.1186/1752-0509-8-6
- Fadhal E. Exploring lung cancer protein network: Understanding structure and function through metric space modeling. Eur J Pure Appl Math. 2024;17(2):905-921.
- Wu G, Feng X, Stein L. A human functional protein interaction network and its application to cancer data analysis. Genome Biol. 2010;11(5):R53. doi: 10.1186/gb-2010-11-5-r53
- Fadhal E, Mwambene EC, Gamieldien J. Modelling human protein interaction networks as metric spaces has potential in disease research and drug target discovery. BMC Syst Biol. 2014;8:68. doi: 10.1186/1752-0509-8-68
- Vogelstein B, Papadopoulos N, Velculescu VE, Zhou S, Diaz LA Jr., Kinzler KW. Cancer genome landscapes. Science. 2013;339(6127):1546-1558.doi: 10.1126/science.1235122
- Madkour MM, Anbar HS, El-Gamal MI. Current status and future prospects of p38α/MAPK14 kinase and its inhibitors. Eur J Med Chem. 2021;213:113216. doi: 10.1016/j.ejmech.2021.113216
- Liu Y, Du Y, Hu X, Zhao L, Xia W. Up-regulation of ceRNA TINCR by SP1 contributes to tumorigenesis in breast cancer. BMC Cancer. 2018;18:367. doi: 10.1186/s12885-018-4255-3
- Mesquita FP, Moreira-Nunes CA, da Silva EL, et al. MAPK14 (p38α) inhibition effects against metastatic gastric cancer cells: A potential biomarker and pharmacological target. Toxicol In Vitro. 2020;66:104839. doi: 10.1016/j.tiv.2020.104839
- Blake JA, Bult CJ, Eppig JT, Kadin JA, Richardson JE, Mouse Genome Database Group. The mouse genome database genotypes: Phenotypes. Nucleic Acids Res. 2009;37(Suppl 1):D712-D719. doi: 10.1093/nar/gkn886
- Blume-Jensen P, Hunter T. Oncogenic kinase signalling. Nature. 2001;411(6835):355-365. doi: 10.1038/35077225
- Sebolt-Leopold JS, Herrera R. Targeting the mitogen-activated protein kinase cascade to treat cancer. Nat Rev Cancer. 2004;4(12):937-947. doi: 10.1038/nrc1503
- Foulstone E, Prince S, Zaccheo O, et al. Insulin‐like growth factor ligands, receptors, and binding proteins in cancer. J Pathol. 2005;205(2):145-153. doi: 10.1002/path.1712
- Lapenna S, Giordano A. Cell cycle kinases as therapeutic targets for cancer. Nat Rev Drug Discov. 2009;8(7):547-566. doi: 10.1038/nrd2907
- Min J, Zaslavsky A, Fedele G, et al. An oncogene-tumor suppressor cascade drives metastatic prostate cancer by coordinately activating Ras and nuclear factor-κB. Nat Med. 2010;16(3):286-294. doi: 10.1038/nm.2100
- Münger K. Disruption of oncogene/tumor suppressor networks during human carcinogenesis. Cancer Investig. 2002;20(1):71-81. doi: 10.1081/cnv-120000369
- Lee EY, Muller WJ. Oncogenes and tumor suppressor genes. Cold Spring Harb Perspect Biol. 2010;2(10):a003236. doi: 10.1101/cshperspect.a003236
- Chen WH, Lu G, Chen X, Zhao XM, Bork P. OGEE v2: An update of the online gene essentiality database with special focus on differentially essential genes in human cancer cell lines. Nucleic Acids Res. 2016;44(D1):D1057-D1063. doi: 10.1093/nar/gkw1013
- Chen WH, Trachana K, Lercher MJ, Bork P. Younger genes are less likely to be essential than older genes, and duplicates are less likely to be essential than singletons of the same age. Mol Biol Evol. 2012;29(7):1703-1706. doi: 10.1093/molbev/mss014
- Kanehisa M, Araki M, Goto S, et al. KEGG for linking genomes to life and the environment. Nucleic Acids Res. 2007;36(suppl 1):D480-D484.
- Petrucelli N, Daly MB, Pal T. BRCA1-and BRCA2-Associated Hereditary Breast and Ovarian Cancer. Seattle, WA: University of Washington; 2022.
- Peto J, Collins N, Barfoot R, et al. Prevalence of BRCA1 and BRCA2 gene mutations in patients with early-onset breast cancer. J Natl Cancer Inst. 1999;91(11):943-949. doi: 10.1093/jnci/91.11.943
- Couch FJ, Shimelis H, Hu C, et al. Associations between cancer predisposition testing panel genes and breast cancer. JAMA Oncol. 2017;3(9):1190-1196. doi: 10.1001/jamaoncol.2017.0424
- Guo YJ, Pan WW, Liu SB, Shen ZF, Xu Y, Hu LL. ERK/ MAPK signalling pathway and tumorigenesis. Exp Ther Med. 2020;19(3):1997-2007. doi: 10.3892/etm.2020.8454
- Lee S, Rauch J, Kolch W. Targeting MAPK signaling in cancer: Mechanisms of drug resistance and sensitivity. Int J Mol Sci. 2020;21(3):1102. doi: 10.3390/ijms21031102
- Mukherjee CS, Zhang J. A multi-core periphery perspective: Ranking via relative centrality. arXiv preprint. 2024; arXiv:2406.04487.
- Zhao L, Yang Y, Bai X, et al. Structure, robustness and supply risk in the global wind turbine trade network. Renew Sustain Energy Rev. 2023;177:113214.
- Brown RE, Short SP, Williams CS. Colorectal cancer and metabolism. Curr Colorectal Cancer Rep. 2018;14:226-241. doi: 10.1007/s11888-018-0420-y
- Blevins AS, Kim JZ, Bassett DS. Variability in higher order structure of noise added to weighted networks. Commun Phys. 2021;4(1):233.
- Ramos RH, Ferreira COL, Simao A. Human protein-protein interaction networks: A topological comparison review. Heliyon. 2024;10(5):e27278.
- Fadhal E, Gamieldien J, Mwambene EC. Self-similarity of human protein interaction networks: A novel strategy of distinguishing proteins. Sci Rep. 2015;5(1):7628. doi: 10.1038/srep07628
- Snider J, Kotlyar M, Saraon P, Yao Z, Jurisica I, Stagljar I. Fundamentals of protein interaction network mapping. Mol Syst Biol. 2015;11(12):848. doi: 10.15252/msb.20156351
- Wang Y, Zhang S, Li F, et al. Therapeutic target database 2020: Enriched resource for facilitating research and early development of targeted therapeutics. Nucleic Acids Res. 2020;48:D1031-D1041. doi: 10.1093/nar/gkz981
- Yi Y, Fang Y, Wu K, Liu Y, Zhang W. Comprehensive gene and pathway analysis of cervical cancer progression. Oncol Lett. 2020;19(4):3316-3332. doi: 10.3892/ol.2020.11439
- Liu Z, Huang H, Yu Y, et al. Exploring the potential mechanism of action of ursolic acid against gastric cancer and COVID-19 using network pharmacology and bioinformatics analysis. Curr Pharm Des. 2023;29(16): 1274-1292. doi: 10.2174/1381612829666230510124716