The Role of Artificial Intelligence in Diagnosing Malignant Tumors
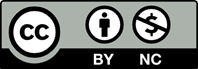
This paper explores the transformative impact of artificial intelligence (AI) in early tumor diagnosis, emphasizing its role in analyzing health records, medical images, biopsies, and blood tests for improved risk stratification. While screening programs have enhanced survival, challenges remain in patient selection and diagnostic workforces. The review covers diverse AI approaches, including logistic regression, deep learning, and neural networks, applied to various data types in oncology. It discusses the clinical implications, current models in practice, and potential limitations such as ethical concerns and resource demands. We provide an overview of the main artificial intelligence approaches, encompassing historical models like logistic regression, alongside deep learning and neural networks, emphasizing their applications in early diagnosis. We describe the role of AI in tumor detection, prognosis, and treatment administration, and we introduce the application of state-of-the-art large language models in oncology clinics. Our exploration extends to AI applications for omics data types, offering perspectives on their combination for decision-support tools. Concurrently, we evaluate existing constraints and challenges in applying artificial intelligence to precision oncology. The overall aim is to showcase AI's promise in revolutionizing tumor diagnosis while acknowledging and addressing associated challenges, thereby advancing patient care.
1. Hunter B, Hindocha S, Lee RW. The role of artificial intelligence in early cancer diagnosis. Cancers (Basel) 2022;14:1524.
2. Chen ZH, Lin L, Wu CF, Li CF, Xu RH, Sun Y. Artificial intelligence for assisting cancer diagnosis and treatment in the era of precision medicine. Cancer Commun (Lond) 2021;41:1100–15.
3. Koh DM, Papanikolaou N, Bick U, Illing R, Kahn CE Jr, KalpathiCramer J, et al. Artificial intelligence and machine learning in cancer imaging. Commun Med (Lond).2022;2:133.
4. Hosny A, Parmar C, Quackenbush J, Schwartz LH, Aerts HJWL. Artificial intelligence in radiology. Nat Rev Cancer 2018;18:500–10.
5. Castellino RA. Computer aided detection (CAD): An overview. Cancer Imaging 2005;5:17–9.
6. Esteva A, Kuprel B, Novoa RA, Ko J, Swetter SM, Blau HM, et al. Dermatologist-level classification of skin cancer with deep neural networks. Nature 2017;542:115–8.
7. Ozkan E, Orhan K, Soydal C, Kahya Y, Seckin Tunc S, Celik O, et al. Combined clinical and specific positron emission tomography/computed tomography-based radiomic features and machine-learning model in prediction of thymoma risk groups. Nucl Med Commun 2022;43:529–39.
8. Dai H, Huang Y, Xiao G, Lan B, Jiang G, Tian J. Predictive features of thymic carcinoma and high-risk thymomas using random forest analysis. J Comput Assist Tomogr 2020;44:857–64.
9. Lin CY, Yen YT, Huang LT, Chen TY, Liu YS, Tang SY, et al. An MRI-based clinical-perfusion model predicts pathological subtypes of prevascular mediastinal tumors. Diagnostics (Basel) 2022;12:889.
10. Pang J, Xiu W, Ma X. Application of artificial intelligence in the diagnosis, treatment, and prognostic evaluation of mediastinal malignant tumors. J Clin Med 2023;12:2818.
11. Wang D, Zhang Y, Li B, Zhuang Q, Zhang X, Lin D. Histological classification and invasion prediction of thymoma by machine learning-based computed tomography imaging. Contrast Media Mol Imaging 2022;2022:4594757.
12. Open Medscience. Revolutionising medical imaging with AI and Big Data analytics. Available at: https://openmedscience.com/revolutionising-medical-imaging-with-ai-and-big-dataanalytics/#:~:text=AI%20algorithms%20can%20detect%20 early,function%20and%20diagnose%20heart%20disease. Accessed Aug 16, 2024.
13. Sajid H. AI in radiology: Pros & cons, applications, and 4 examples. Available at: https://www.v7labs.com/blog/ai-in-radiology. Accessed Aug 16, 2024.
14. Isensee F, Jäger PF, Full PM, Vollmuth P, Maier-Hein KH. nnUNet for brain tumor segmentation. In: Crimi A, Bakas S, editors. Brainlesion: Glioma, Multiple Sclerosis, Stroke and Traumatic Brain Injuries. Cham: Springer; 2021. pp. 118–32.
15. Rehman MU, Ryu J, Nizami IF, Chong KT. RAAGR2-Net: A brain tumor segmentation network using parallel processing of multiple spatial frames. Comput Biol Med 2023;152:106426.
16. Chen H, Qin Z, Ding Y, Tian L, Qin Z. Brain tumor segmentation with deep convolutional symmetric neural network. Neurocomput 2020;392:305–13.
17. Lather M, Singh P. Investigating brain tumor segmentation and detection techniques. Proc Comput Sci 2020;167:121–30.
18. Rehman M, Cho S, Kim JH, Chong KT. BU-Net: Brain tumor segmentation using modified U-net architecture. Electronics 2020;9:2203.
19. Rehman MU, Cho S, Kim J, Chong KT. BrainSeg-Net: brain tumor mr image segmentation via enhanced encoder-decoder network. Diagnostics (Basel) 2021;11:169.
20. Ranjbarzadeh R, Zarbakhsh P, Caputo A, Tirkolaee EB, Bendechache M. Brain tumor segmentation based on optimized convolutional neural network and improved chimp optimization algorithm. Comput Biol Med 2024;168:107723.
21. Vinisha A, Boda R. A novel frameworkfor brain tumor segmentation using neuro trypetidae fruit fly-based U-net. Int J Intell Syst Appl Eng 2024;12:783–96.
22. Institute for Quality and Efficiency in Health Care. In brief: How do cancer cells grow and spread? Available at: https:// www.ncbi.nlm.nih.gov/books/NBK279410/. Accessed Aug 16, 2024.
23. Cooper GM. The development and causes of cancer. Available at: https://www.ncbi.nlm.nih.gov/books/NBK9963/. Accessed Aug 16, 2024.
24. McDaniel B, Badri T, Steele RB. Basal cell carcinoma. Available at: https://www.ncbi.nlm.nih.gov/books/NBK482439/. Accessed Aug 16, 2024.
25. Cleveland Clinic. Carcinoma. Available at: https:// my.clevelandclinic.org/health/diseases/23180-carcinoma. Accessed Aug 16, 2024.
26. Kuhn E, Morbini P, Cancellieri A, Damiani S, Cavazza A, Comin CE. Adenocarcinoma classification: Patterns and prognosis. Pathologica 2018;110:5–11.
27. Basset-Seguin N, Herms F. Update in the management of basal cell carcinoma. Acta Derm Venereol 2020;100:adv00140.
28. Britannica. Carcinoma. Available at: https://www.britannica. com/science/carcinoma. Accessed Aug 16, 2024.
29. Vodanovich DA, M Choong PF. Soft-tissue sarcomas. Indian J Orthop 2018;52:35–44.
30. National Cancer Institute. Soft tissue sarcoma treatment (PDQ®)–patient version. Available at: https://www.cancer.gov/types/soft-tissue-sarcoma/patient/adult-soft-tissuetreatment-pdq. Accessed Aug 16, 2024.
31. Islam MN, Chehal H, Smith MH, Islam S, Bhattacharyya I. Merkel cell carcinoma of the buccal mucosa and lower lip. Head Neck Pathol 2018;12:279–85.
32. Gilbert NF, Cannon CP, Lin PP, Lewis VO. Soft-tissue sarcoma. J Am Acad Orthop Surg 2009;17:40–7.
33. Faouzi S, Ouguellit S, Loriot Y. Stage 1 germ-cell tumour. Bull Cancer 2019;106:887–95. French.
34. Lakpour N, Saliminejad K, Ghods R, Reza Sadeghi M, Pilatz A, Khosravi F, et al. Potential biomarkers for testicular germ cell tumour: Risk assessment, diagnostic, prognostic and monitoring of recurrence. Andrologia 2021;53:e13998.
35. Batool A, Karimi N, Wu XN, Chen SR, Liu YX. Testicular germ cell tumor: A comprehensive review. Cell Mol Life Sci 2019;76:1713–27.
36. Aggarwal P, Sharma SK, Kapila K, Verma K, Agarwal J. Pulmonary blastoma. Indian J Chest Dis Allied Sci 1990;32:59–64.
37. Tsamis I, Chachali SP, Gomatou G, Trontzas I, Mitsogianni M, Syrigos N, et al. Pulmonary blastoma: A comprehensive overview of a rare entity. Adv Respir Med 2021;89:511–9.
38. Alruwaili AA, De Jesus O. Meningioma. Available at: https://www.ncbi.nlm.nih.gov/books/NBK560538/. Accessed Aug 16, 2024.
39. Louis DN, Perry A, Wesseling P, Brat DJ, Cree IA, Figarella-Branger D, et al. The 2021 WHO classification of tumors of the central nervous system: A summary. Neuro Oncol 2021;23:1231–51.
40. Buerki RA, Horbinski CM, Kruser T, Horowitz PM, James CD, Lukas RV. An overview of meningiomas. Future Oncol 2018;14:2161–77.
41. Bera K, Katz I, Madabhushi A. Reimagining T staging through artificial intelligence and machine learning image processing approaches in digital pathology. JCO Clin Cancer Inform 2020;4:1039–50.
42. Rosen RD, Sapra A. TNM classification. In: StatPearls. Treasure Island (FL): StatPearls Publishing; 2024.
43. Feng SH, Yang ST. The new 8th TNM staging system of lung cancer and its potential imaging interpretation pitfalls and limitations with CT image demonstrations. Diagn Interv Radiol 2019;25:270–9.
44. Lu J, Zheng ZF, Wang W, Xie JW, Wang JB, Lin JX, et al. A novel TNM staging system for gastric cancer based on the metroticket paradigm: a comparative study with the AJCC-TNM staging system. Gastric Cancer 2019;22:759–68.
45. Baars A, Buter J, Pinedo HM. Making use of the primary tumour. Bioessays 2003;25:79–86.
46. Brierley J, O'Sullivan B, Asamura H, Byrd D, Huang SH, Lee A, et al. Global consultation on cancer staging: promoting consistent understanding and use. Nat Rev Clin Oncol 2019 ;16:763– 71.
47. Karpf M. Lymphadenopathy. Available at: https://www.ncbi. nlm.nih.gov/books/NBK256/. Accessed Aug 16, 2024.
48. Cleveland Clinic. Swollen lymph nodes. Available at: https:// my.clevelandclinic.org/health/symptoms/15219-swollenlymph-nodes. Accessed Aug 16, 2024.
49. Coumans FA, Siesling S, Terstappen LW. Detection of cancer before distant metastasis. BMC Cancer 2013;13:283.
50. PDQ Adult Treatment Editorial Board. Table 5. Definition of distant metastasis (M)a. Available at: https://www.ncbi.nlm. nih.gov/books/NBK65744/table/CDR0000062787__1944/. Accessed Aug 16, 2024.
51. Tan B. Stages of cancer. Available at: https://www.cancercenter.com/stages-of-cancer. Accessed Aug 16, 2024.
52. American Cancer Society. Cancer staging. Available at: https:// www.cancer.org/cancer/diagnosis-staging/staging.html. Accessed Aug 16, 2024.
53. Markman M. Stage 4 cancer. Available at: https://www.cancercenter.com/stage-four-cancer. Accessed Aug 16, 2024.
54. Nezis IP, Stravopodis DJ, Papassideri I, Robert-Nicoud M, Margaritis LH. Stage-specific apoptotic patterns during Drosophila oogenesis. Eur J Cell Biol 2000;79:610–20.
55. Nezis IP, Modes V, Mpakou V, Stravopodis DJ, Papassideri IS, Mammali I, et al. Modes of programmed cell death during Ceratitis capitata oogenesis. Tissue Cell 2003;35:113–9.
56. Bohr A, Memarzadeh K. The rise of artificial intelligence in healthcare applications. Artific Intell Healthcare 2020:25–60.
57. Ahuja AS. The impact of artificial intelligence in medicine on the future role of the physician. PeerJ 2019;7:e7702.
58. Linardatos P, Papastefanopoulos V, Kotsiantis S. Explainable AI: A review of machine learning interpretability methods. Entropy 2021;23:18.
59. LeCun Y, Bengio Y, Hinton G. Deep learning. Nature 2015;521:436–44.
60. Miotto R, Wang F, Wang S, Jiang X, Dudley JT. Deep learning for healthcare: Review, opportunities and challenges. Brief Bioinform 2018;19:1236–46.
61. Shen D, Wu G, Suk HI. Deep learning in medical image analysis. Annu Rev Biomed Eng 2017;19:221–48.
62. Shin HC, Roth HR, Gao M, Lu L, Xu Z, Nogues I, et al. Deep convolutional neural networks for computer-aided detection: CNN architectures, dataset characteristics and transfer learning. IEEE Trans Med. Imag 2016;35:1285–98.
63. Litjens G, Kooi T, Bejnordi BE, Setio AAA, Ciompi F, Ghafoorian M, et al. A survey on deep learning in medical image analysis. Med Image Anal 2017;42:60–88.
64. Busnatu Ș, Niculescu AG, Bolocan A, Petrescu GED, Păduraru DN, Năstasă I, et al. Clinical applications of artificial intelligence- An updated overview. J Clin Med 2022;11:2265.
65. Espinoza JL, Dong LT. Artificial intelligence tools for refining lung cancer screening. J Clin Med 2020;9:3860.
66. Gatti M, Maino C, Tore D, Carisio A, Darvizeh F, Tricarico E, et al. Benign focal liver lesions: The role of magnetic resonance imaging. World J Hepatol 2022;14:923–43.
67. Younghak Shin, Balasingham I. Comparison of hand-craft feature based SVM and CNN based deep learning framework for automatic polyp classification. Annu Int Conf IEEE Eng Med Biol Soc 2017;2017:3277–80.
68. Orringer DA, Pandian B, Niknafs YS, Hollon TC, Boyle J, Lewis S, et al. Rapid intraoperative histology of unprocessed surgical specimens via fibre-laser-based stimulated Raman scattering microscopy. Nat Biomed Eng 2017;1:0027.
69. Jones S, Thompson K, Porter B, Shepherd M, Sapkaroski D, Grimshaw A, et al. Automation and artificial intelligence in radiation therapy treatment planning. J Med Radiat Sci 2024;71:290–8.
70. Masood A, Al-Jumaily AA. Computer aided diagnostic support system for skin cancer: A review of techniques and algorithms. Int J Biomed Imaging 2013;2013:323268.
71. Jartarkar SR, Cockerell CJ, Patil A, Kassir M, Babaei M, Weidenthaler-Barth B, et al. Artificial intelligence in dermatopathology. J Cosmet Dermatol 2023;22:1163–7.
72. Albarqouni S, Baur C, Achilles F, Belagiannis V, Demirci S, Navap N. AggNet: Deep learning from crowds for mitosis detection in breast cancer histology images. IEEE Trans Med Image 2016;35:1313–21.
73. Djuric U, Zadeh G, Aldape K, Diamandis P. Precision histology: How deep learning is poised to revitalize histomorphology for
personalized cancer care. NPJ Precis Oncol 2017;1:22.
74. Janowczyk A, Madabhushi A. Deep learning for digital pathology image analysis: A comprehensive tutorial with selected use cases. J Pathol Inform 2016;7:29.
75. Bejnordi BE, Lin J, Glass B, Mullooly M, Gierach GL, Sherman ME, et al. DEEP learning-based assessment of tumor-associated stroma for diagnosing breast cancer in histopathology images. Proc IEEE Int Symp Biomed Imaging 2017;2017:929–32.
76. Shafi S, Parwani AV. Artificial intelligence in diagnostic pathology. Diagn Pathol 2023;18:109.
77. Yuan Y, Shi Y, Li C, Kim J, Cai W, Han Z, et al. DeepGene: An advanced cancer type classifier based on deep learning and somatic point mutations. BMC Bioinformatics 2016;17:476.
78. Alipanahi B, Delong A, Weirauch MT, Frey BJ. Predicting the sequence specificities of DNA- and RNA-binding proteins by deep learning. Nat Biotechnol 2015;33:831–8.
79. Jan YT, Tsai PS, Huang WH, Chou LY, Huang SC, Wang JZ, et al. Machine learning combined with radiomics and deep learning features extracted from CT images: A novel AI model to distinguish benign from malignant ovarian tumors. Insights Imaging 2023;14:68.
80. Illumina. Detecting changes in the cancer transcriptome. Available at: https://sapac.illumina.com/areas-of-interest/ cancer/research/sequencing-methods/cancer-rna-seq.html. Accessed Aug 16, 2024.
81. Hrdlickova R, Toloue M, Tian B. RNA-Seq methods for transcriptome analysis. Wiley Interdiscip Rev RNA 2017;8:10.1002/ wrna.1364.
82. Casamassimi A, Federico A, Rienzo M, Esposito S, Ciccodicola A. Transcriptome profiling in human diseases: New advances and perspectives. Int J Mol Sci 2017;18:1652.
83. Lang J, Cho WC, Huang T, Wu T, Xu J. Editorial: Applications of RNA-seq in cancer and tumor research. Front Genet 2023;14:1331576.
84. Delen D, Walker G, Kadam A. Predicting breast cancer survivability: A comparison of three data mining methods. Artif Intell Med 2005;34:113–27.
85. Lu H, Wang H, Yoon SW. A dynamic gradient boosting machine using genetic optimizer for practical breast cancer prognosis. Expert Syst Appl 2019;116:340–50.
86. Vasudevan P, Murugesan T. Cancer subtype discovery using prognosis-enhanced neural network classifier in multigenomic data. Technol Cancer Res Treat 2018;17:1533033818790509.
87. Tian DW, Wu ZL, Jiang LM, Gao J, Wu CL, Hu HL. Neural precursor cell expressed, developmentally downregulated 8 promotes tumor progression and predicts poor prognosis of patients with bladder cancer. Cancer Sci 2019;110:458–67.
88. Hasnain Z, Mason J, Gill K, Miranda G, Gill IS, Kuhn P, et al. Machine learning models for predicting post-cystectomy recurrence and survival in bladder cancer patients. PLoS One 2019;14:e0210976.
89. Biglarian A, Hajizadeh E, Kazemnejad A, Zali M. Application of artificial neural network in predicting the survival rate of gastric cancer patients. Iran J Public Health 2011;40:80–6.
90. Zhu L, Luo W, Su M, Wei H, Wei J, Zhang X, et al. Comparison between artificial neural network and Cox regression model in predicting the survival rate of gastric cancer patients. Biomed Rep 2013;1:757–60.
91. Bottaci L, Drew PJ, Hartley JE, Hadfield MB, Farouk R, Lee PW, et al. Artificial neural networks applied to outcome prediction for colorectal cancer patients in separate institutions. Lancet 1997;350:469–72.
92. Lu TP, Kuo KT, Chen CH, Chang MC, Lin HP, Hu YH, et al. Developing a prognostic gene panel of epithelial ovarian cancer patients by a machine learning model. Cancers (Basel) 2019;11:270.
93. Bychkov D, Linder N, Turkki R, Nordling S, Kovanen PE, Verrill C, et al. Deep learning based tissue analysis predicts outcome in colorectal cancer. Sci Rep 2018;8:3395.
94. Chang SW, Kareem SA, Kallarakkal TG, Merican AF, Abraham MT, Zain RB. Feature selection methods for optimizing clinicopathologic input variables in oral cancer prognosis. Asian Pac J Cancer Prev 2011;12:2659–64.
95. Lynch CM, Abdollahi B, Fuqua JD, de Carlo AR, Bartholomai JA, Balgemann RN, et al. Prediction of lung cancer patient survival via supervised machine learning classification techniques. Int J Med Inform 2017;108:1–8.
96. Sepehri S, Upadhaya T, Desseroit MC, Visvikis D, Le Rest CC, Hatt M. Comparison of machine learning algorithms for building prognostic models in non-small cell lung cancer using clinical and radiomics features from 18F-FDG PET/CT images. J Nucl Med 2018;59:328.
97. Acharya U, Akter A, Chowriappa P, Dua S, Raghavendra U, Koh JEW, et al. Use of nonlinear features for automated characterization of suspicious ovarian tumors using ultrasound images in fuzzy forest framework. Int J Fuzzy Syst 2018;20:1385–402.
98. Qian Z, Li Y, Wang Y, Li L, Li R, Wang K, et al. Differentiation of glioblastoma from solitary brain metastases using radiomic machine-learning classifiers. Cancer Lett 2019;451:128–35.
99. Kuo RJ, Huang MH, Cheng WC, Lin CC, Wu YH. Application of a two-stage fuzzy neural network to a prostate cancer prognosis system. Artif Intell Med 2015;63:119–33.