Artificial Intelligence and Machine Learning in Oncology: Historical Overview of Documents Indexed in the Web of Science Database
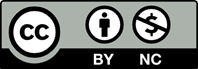
Objectives: Artificial Intelligence (AI) and Machine Learning (ML) are innovations contributing to the diagnosis and treatment of cancer. The aim is to present an overview of AI and ML application in oncology research.
Methods: Data was retrieved from the web of science. Bibliometrics and R packages and VOSviwer software was used for mapping and network analysis.
Results: 214 publications were retrieved written by 1161 authors and published in 133 journals from 1988 to 2021. There has been a steadily increasing trend of research over the past years. AI and ML in oncology research have attracted the interest of the scientific community and the readership. The first ranked documents received a 173 citations score. It covers hot topics related to common mistakes in diagnostic classification in clinical and the potential future opportunities for precision oncology using AI. Aneja S and Thompson RF from the USA are the most productive author. Frontiers in Oncology is the most productive Journal. The United States is leading the research effort on the topics, followed by Korea. The collaboration and network between countries in AL or ML in oncology research were documented.
Conclusion: AI and ML in oncology research have attracted the interest of of the scientific community and readership. The trend of research has been steadily increasing globally.
1.Aminoshariae A, Kulild J, Nagendrababu V. Artificial Intelligence in dndodontics: current applications and future directions. JOE 2021;04:3–4.
2. Iqbal MJ, Javed Z, Sadia H, Qureshi IA, Irshad A, Ahmed R, et al. Clinical applications of artificial intelligence and machine learning in cancer diagnosis: looking into the future. Cancer Cell Int 2021;21:270. [CrossRef]
3. Chua IS, Gaziel-Yablowitz M, Korach ZT, Kehl KL, Levitan NA, Arriaga YE, et al. Artificial intelligence in oncology: Path to implementation. Cancer Med 2021;10:4138–49. [CrossRef] 4. Akintunde TY, Akintunde OD, Musa TH, Sayibu M, Tassang AE, Reed L, et al. Expanding telemedicine to reduce the burden on the healthcare systems and poverty in Africa for a post-coronavirus disease 2019 (COVID-19) pandemic reformation. Global Health Journal Jul 2021. Available at: https:// reader.elsevier.com/reader/sd/pii/S2414644721000671?token=D414E7C7940A7207DF264C4DB52237370E36455 47B7B0B043AE0B36A8C8DE81C8B008AE3A1E52E1F9DCDAD0D8218E0AF&originRegion=eu-west-1&originCreation=20210922103512. Accessed Sep 22, 2021.
5. Akintunde TY, Musa TH, Musa HH, Chen S, Ibrahim E, Muhideen S, et al. Mapping the global research output on Ebola vaccine from research indexed in web of science and scopus: a comprehensive bibliometric analysis. Hum Vaccin Immunother 2021:1–13. [CrossRef]
6. Akintunde TY, Musa TH, Musa HH, Musa IH, Chen S, Ibrahim E, et al. Bibliometric analysis of global scientific literature on effects of COVID-19 pandemic on mental health. Asian J Psychiatr 2021;63:102753. [CrossRef]
7. Musa TH, Akintunde TY, Musa HH, Ghimire U, Gatasi G. Malnutrition research output: a bibliometric analysis for articles index in web of science between 1900 and 2020. Electron J Gen Med 2021;18:em293. [CrossRef]
8. Musa TH, Akintunde TY, Gatasi G, Ghimire U, Kawuki J, Musa HH. A bibliometric analysis of the 100 top-cited articles on global malnutrition indexed in Web of Science. J Public Health Emerg 2021. Available at: https://jphe.amegroups.com/article/view/7533/pdf. Accessed Sep 22, 2021. [CrossRef]
9. Musa, Hassan H, El-Sharief, Monsour, Musa, Idriss H, Musa TH, Akintunde TY. Global scientific research output on sickle cell disease : a comprehensive bibliometric analysis of web of science publication. Sci Afr 2021;12:e00774. [CrossRef]
10. Schwarzer G, Vach W, Schumacher M. On the misuses of artificial neural networks for prognostic and diagnostic classification in oncology. Stat Med 2000;19:541–61. [CrossRef]
11. Bera K, Schalper KA, Rimm DL, Velcheti V, Madabhushi A. Artificial intelligence in digital pathology - new tools for diagnosis and precision oncology. Nat Rev Clin Oncol 2019;16:703–715.
12. Patel SK, George B, Rai V. Artificial intelligence to decode cancer mechanism: beyond patient stratification for precision oncology. Front Pharmacol 2020;11:1177. [CrossRef]
13. Kosvyra A, Maramis C, Chouvarda I. Developing an integrated genomic profile for cancer patients with the use of NGS data. Emerg Sci J 2019;3:157–67. [CrossRef]
14. American Cancer Society. Cancer Facts & Figures 2014. Available at: https://www.cancer.org/research/cancer-facts-statistics/all-cancer-facts-figures/cancer-facts-figures-2014.html. Accessed Sep 22, 2021.
15. National Cancer Policy Forum; Board on health care services; institute of medicine. delivering affordable cancer care in the 21st century: workshop summary. Washington (DC): National Academies Press (US); 2013 May 20.
16. Hesse BW, Ahern DK, Beckjord E. Introduction. Oncology Informatics. Cambridge, Massachusetts: Academic Press; 2016.
17. Levit L, Balogh E, Nass S, Ganz PA. Delivering high-quality cancer care: charting a new course for a system in crisis. committee on improving the quality of cancer care: addressing the challenges of an aging population. Board on Health Care Services, Institute of Medicine. Washington DC: National Academies Press; 2013.
18. James B. Quality improvement opportunities in health care. making it easy to do it right. J Manag Care Pharm 2002;8:394– 9. [CrossRef]
19. Reid PP, Compton WD, Grossman JH FG. Building a better delivery system: a new engineering/health care partnership. In: Reid PP, Compton WD, Grossman JH, Fanjiang G, editors. Washington, DC: National Academy of Engineering Institute of Medicine; 2005. p. 262.
20. Nass SJ, Wizemann TM. Informatics needs and challenges in cancer research: workshop summary. In: Washington DNAP, editor. Washington, DC: National Academies Press; 2012. p. 129. [CrossRef]
21. Hirsch B, Abernethy AP. Informatics support across the cancer continuum. Oncology Informatics. Cambridge, Massachusetts: Academic Press; 2016. p. 145–57. [CrossRef]
22. Horowitz TS, Rensink RA. Extended Vision for Oncology. Cambridge, Massachusetts: Oncology Informatics. Academic Press; 2016. p. 287–303. [CrossRef]