Evaluation of Parametric Method Performance for Left-Censored Data and Recommendation of Using for Covid-19 Data Analysis
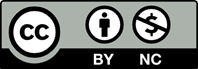
Objectives: Left-censored data, which is commonly seen in clinical studies, are frequently encountered in the literature, especially in the fields of food, environment, microbiology, and biochemistry. In this study, the most appropriate distribution between the negatively skewed distributions for left-censored data in Parametric Inverse Hazard Models was tried to be determined.
Methods: Within the scope of the study, firstly, the data were produced uncensored according to different parameters of each distribution. Then, simulation studies were carried out in different censorship rates (15%, 25% and 35%) and various sample sizes (1000, 2000 and 3000) in order to determine the most appropriate distribution. AIC, AICC, HQIC, and CAIC information criteria were employed to compare the distribution performances. Since it was not possible to study simulations of all possible scenarios, scenarios similar to each other were generally preferred over others.
Results: In the simulation results, the most appropriate distributions to be used for left-censored data in Parametric Inverse Hazard Models were found as Generalized Inverse Weibull as well as Log-Logistic, Log-Normal, Inverse Normal and Gamma distributions. It was also detected that the Marshal-Olkin distribution revealed a superior performance compared to the Modified Weibull, Generalized Gamma, Gamma, and Flexible Weibull distributions. Log logistics distribution gave the most appropriate result among the analyzed distributions in the examination made with real data application.
Conclusion: The use of censored data analysis in evaluations in terms of Covid-19 is quite additive, considering that more statistical evaluation will be needed in the next period of the epidemic. Improved estimates can be obtained with this approach, especially in Covid-19 data analysis.
1.Nelson WB. Applied life data analysis. John Wiley and Sons; 1982.
2. Huston C, Juarez-Colung E. Guidelines for computing summary statistics for data-sets containing non-detects. Smithers, Canada: Bulkley Valley Research Center; 2009. Available at: https://bvcentre.ca/files/research_reports/08-03GuidanceDocument.pdf. Accessed Apr 20, 2021.
3. Lawless JF. Statistical models and methods for lifetime data. New York: John Wiley and Sons; 2003.
4. Horny G. Inference in mixed proportional hazard models with K random effects. Stat Pap 2009;50:481–99.
5. Glass DC, Gray CN. Estimating mean exposures from censored data: exposure to benzene in the Australian petroleum industry. Ann Ocp Hyg 2001;45:275–82.
6. Mulhausen J, Damiano J. A strategy for assessing and managing occupational exposures. 2nd ed. Fairview, VA: American Industrial Hygiene Association; 1998. p. 349.
7. Hawkins NC, Norwood SK, Rock JC. A strategy for occupational exposure assessment. Fairview, VA: American Industrial Hygiene Association; 1991.
8. Lee L, Helsel DR. Statistical analysis of water-quality data containing multiple detection limits: S-language software for regression on order statistics. Comput and Geosci 2005;31:1241–8.
9. Finkelstein MM, Verma DK. Exposure estimation in the presence of nondetectable values: another look. AIHAJ 2001;62:195–8.
10. She N. Analyzing censored water quality data using a non-parametric approach. JAWRA 1997;33:615–24.
11. Shumway RH, Azari RS, Kayhanian M. Statistical approaches to estimating mean water quality concentrations with detection limits. Environ Sci Technol 2002;36:3345–53.
12. Hewett P, Ganser GH. A comparison of several methods for analyzing censored data. Ann Occup Hyg 2007;51:611–32.
13. Islam F. Parametric reversed hazards model for left censored data with application to HIV. Master’s thesis. Carolina, US: University of South Carolina; 2016. Available at: https://scholarcommons.sc.edu/cgi/viewcontent.cgi?article=4894&context=etd. Accessed Apr 20, 2021.
14. Odell PM, Anderson KM, D’Agostino RB. Maximum likelihood estimation for interval-censored data using a Weibull-based accelerated failure time model. Biometrics 1992;48:951–9.
15. Luczynska C, Sterne J, Bond J, Azima H, Burney P. Indoor factors associated with concentrations of house dust mite allergen, Der p 1, in a random sample of houses in Norwich, UK. Clin Exp Allergy 1998;28:1201–9.
16. Gupta RD, Kundu D. Theory & methods: Generalized exponential distributions. Aust N Z J Stat 2002;41:173–88.
17. Thompson RE, Volt EO, Scott GI. Statistical modeling of sediment and oyster PAH contamination data collected at a South Carolina estuary (complete and left‐censored samples). Environmetrics 200;11:99–119.
18. Pajek M, Kubala-Kukus A, Banas D, Braziewicz J, Majewska U. Random left‐censoring: a statistical approach accounting for detection limits in x‐ray fluorescence analysis. XRSPAX 2004;33:306–11.
19. Isingo R, Zaba B, Marston M, Ndege M, Mngara J, Mwita W, et al. Survival after HIV infection in the pre-antiretroviral therapy era in a rural Tanzanian cohort. AIDS 2007;21:S5–S13.
20. Annan SY, Liu P, Zhang Y. Comparison of the Kaplan-Meier, maximum likelihood, and ROS estimators for left-censored data using simulation studies; 2009. Available at: https://citeseerx.ist.psu.edu/viewdoc/download?doi= 10.1.1.211.1833&rep=rep1&type=pdf. Accessed Apr 20, 2021.
21. Kremer A, Weißbach R, Liese F. Maximum likelihood estimation for left-censored survival times in an additive hazard model. J Stat Plan 2014;149:33–45.
22. Pesonen M, Pesonen H, Nevalainen J. Covariance matrix estimation for left-cencored data. Comput Stat Data Anal 2015;92:13–25.
23. Achar JA, Coelho-Barros EA, Cuevas JRT, Mazucheli J. Use of Lèvy distribution to analyze longitudinal data with asymmetric distribution and presence of left censored data. CSAM 2018;25:43–60.
24. Fusek M, Michálek J, Buňková L, Buňka F. Modelling biogenic amines in fish meat in Central Europe using censored distributions. Chemosphere 2020;251:126390.
25. Omar S, Bartz C, Becker S, Basenach S, Pfeifer S, Trapp C, et al; Palatina Public Health Study Group. Duration of SARS-CoV-2 RNA detection in COVID-19 patients in home isolation, Rhineland-Palatinate, Germany, 2020 - an interval-censored survival analysis. Euro Surveill 2020;25:2001292.
26. Sreedaevi EP, Sankaran PG. Statistical methods for estimating cure fraction of COVID-19 patients in India. Medrxiv. 2020 Jun 30. Doi: https://doi.org/10.1101/2020.05.30.20117804. [Epub ahead of print].
27. Mollazehi M, Mollazehi M, Abdel-Salam GAS. Modeling survival time to recovery from COVID-19: a case study on Singapore. Research Square. 2020 Mar 30. Doi: 10.21203/rs.3.rs-18600/v2. [Epub ahead of print].
28. Duchateau L, Janssen P. The frailty model. 1st ed. New York: Springer-Verlag; 2008.
29. Variyath AM, Sankaran PG. Parametric regression models using reversed hazard rates. J Probab Stat 2014;2014:645719.
30. Hossain S. Multivariate granger causality between economic growth, electricity consumption, exports and remittance for the panel of three SAARC countries. Eur Sci J 2012;8:347–76.
31. Barlow RE, Marshall AW, Proschan F. Properties of probability distributions with monotone hazard rate. Ann Math Statist 1963;34:375–89.
32. Lwless JF. Statistical models and methods for lifetime data. New York: John Wiley and Sons; 2011.
33. Keilson J, Sumita U. Uniform stochastic ordering and related inequalities. Can J Stat 1982;10:181–98.
34. Block HW, Savits TH, Singh H. The reversed hazard rate function. Probab Eng Inf Sci 1998;12:69–90.
35. Gupta RC, Gupta PL, Gupta RD. Modeling failure time data by lehman alternatives. Commun Stat-Theor M 1998;27:887–904.
36. Lai CD, Xie M. Stochastic ageing and dependence for reliability. 1st ed. New York: Springer-Verlag; 2006.
37. Li X, Xu M. Reversed hazard rate order of equilibrium distributions and a related aging notion. Stat Pap 2008;49:749–67.
38. Marshall AW, Olkin I. Life distributions. 1st ed. New York: Springer-Verlag; 2007.
39. Tojeiro CA, Louzada F. A general threshold stress hybrid hazard model for lifetime data. Stat Pap 2012;53:833–48.
40. Hosmer Jr DW, Lameshow S, May S. Applied Survival Analysis: Regression modeling of time-to-event data. 2nd ed. New York: Wiley; 2008.
41. Gijbels I. Censored data. Wiley Interdiscip Rev Comput Stat 2010;2:178–88.
42. Tekindal MA, Erdoğan BD, Yavuz Y. Evaluating left-censored data through substitution, parametric, semi-parametric, and nonparametric methods: a simulation study. Interdiscip Sci 2017;9:153–72.