Forecasting COVID-19 Cases in Egypt Using ARIMA-Based Time-Series Analysis
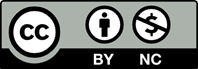
Objectives: The World Health Organization declared the novel coronavirus (COVID-19) outbreak a public health emergency of international concern on January 30, 2020. Since it was first identified, COVID-19 has infected more than one hundred million people worldwide, with more than two million fatalities. This study focuses on the interpretation of the distribution of COVID-19 in Egypt to develop an effective forecasting model that can be used as a decision-making mechanism to administer health interventions and mitigate the transmission of COVID-19.
Methods: A model was developed using the data collected by the Egyptian Ministry of Health and used it to predict possible COVID-19 cases in Egypt.
Results: Statistics obtained based on time-series and kinetic model analyses suggest that the total number of COVID-19 cases in mainland Egypt could reach 11076 per week (March 1, 2020 through January 24, 2021) and the number of simple regenerations could reach 12. Analysis of the ARIMA (2, 1, 2) and (2, 1, 3) sequences shows a rise in the number of COVID-19 events.
Conclusion: The developed forecasting model can help the government and medical personnel plan for the imminent conditions and ensure that healthcare systems are prepared to deal with them.
1.Maier BF, Brockmann D. Effective containment explains subexponential growth in recent confirmed COVID-19 cases in China. Science 2020;368:742–6.
2. Luz PM, Mendes BV, Codeço CT, Struchiner CJ, Galvani AP. Time series analysis of dengue incidence in Rio de Janeiro,Brazil. Am J Trop Med Hyg 2008;79:933–9.
3. Woo PC, Huang Y, Lau SK, Yuen KY. Coronavirus genomics and bioinformatics analysis. Viruses 2010;2:1804–20.
4. Allard R. Use of time-series analysis in infectious disease surveillance. Bull World Health Organ 1998;76:327.
5. Wu JB, Ye LX, You EK. Prediction of incidence of notifiable contagious diseases by application of time series model. Math Med Biol 2007:1:90–2.
6. WHO. Coronavirus disease 2019 (COVID-19): situation report, 82. Available at: https://apps.who.int/iris/handle/10665/331780. Accessed May 21, 2021.
7. Sahin AR, Erdogan A, Agaoglu PM, Dineri Y, Cakirci AY, Senel ME, et al. 2019 novel coronavirus (COVID-19) outbreak: a review of the current literature. EJMO 2020;4:1–7.
8. Iwendi C, Bashir AK, Peshkar A, Sujatha R, Chatterjee JM, Pasupuleti S, et al. COVID-19 patient health prediction using boosted random forest algorithm. Front Public Health 2020;8:357.
9. Bayes C, Sal y Rosas V, Valdivieso L. Modelling death rates due to COVID-19: A Bayesian approach. Cornell University arXiv:2004.02386.
10. Beck BR, Shin B, Choi Y, Park S, Kang K. Predicting commercially available antiviral drugs that may act on the novel coronavirus (SARS-CoV-2) through a drug-target interaction deep learning model. Comput Struct Biotechnol J 2020;18:784–90.
11. Khalifa NEM, Taha MHN, Hassanien AE, Elghamrawy S. Detection of coronavirus (covid-19) associated pneumonia based on generative adversarial networks and a fine-tuned deep transfer learning model using chest x-ray dataset. Cornell University arXiv:2004.01184.
12. Roosa K, Lee Y, Luo R, Kirpich A, Rothenberg R, Hyman JM, et al. Real-time forecasts of the COVID-19 epidemic in China from February 5th to February 24th, 2020. Infect Dis Model 2020;5:256–63.
13. Yang Z, Zeng Z, Wang K, Wong SS, Liang W, Zanin M, et al. Modified SEIR and AI prediction of the epidemics trend of COVID-19 in China under public health interventions. J Thorac Dis 2020;12:165–74.
14. Li Q, Feng W, Quan YH. Trend and forecasting of the COVID-19 outbreak in China. J Infect 2020;80:469–96.
15. Hu Z, Ge Q, Li S, Jin L, Xiong M. Artificial intelligence forecasting of covid-19 in China. Cornell University arXiv:2002.07112.
16. Al-Qaness MAA, Ewees AA, Fan H, Abd El Aziz M. Optimization Method for Forecasting Confirmed Cases of COVID-19 in China. J Clin Med 2020;9:674.
17. Fanelli D, Piazza F. Analysis and forecast of COVID-19 spreading in China, Italy and France. Chaos Solitons Fractals 2020;134:109761
18. Petropoulos F, Makridakis S. Forecasting the novel coronavirus COVID-19. PLoS One 2020;15:e0231236.