Factor analysis of electrocardiographic findings, anthropometric measures, and age in patients with chronic kidney disease
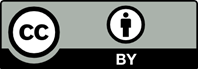
Chronic kidney disease (CKD) is a significant global health concern, frequently associated with cardiovascular complications resulting from autonomic nervous system dysfunction, which can be detected using electrocardiography (ECG). This study employed factor analysis to investigate the association between anthropometric measures, age, and ECG findings in patients with CKD. We conducted a cross-sectional study to evaluate the ECG findings of 25 male participants (aged 36 – 80 years) with stage 5 CKD who were randomly selected from the Nephrology Unit of a hospital in the Amazon region. All participants underwent anthropometric and blood pressure assessment before the ECG recording at a sampling rate of 1,000 Hz. Then, the participants were positioned supine and asked to breathe normally for 3 min. To analyze the ECG data, a bootstrap method was used to estimate statistical parameters from 1,000 resampled datasets. A two-step process involving principal component (PC) extraction and varimax rotation was used for factor analysis. The covariance matrix of the normalized data underwent eigenvalue decomposition. The first three PCs captured 68.7% of the total variability observed in the original dataset. The PR interval (iPR), RR interval (iRR), and corrected QT (QTc) interval contributed 0.843, 0.836, and −0.822, respectively, to PC1; body mass index (BMI) and abdominal circumference (AC) contributed 0.910 and 0.947, respectively, to PC2; and age had the largest contribution of 0.938 to PC3. In conclusion, BMI, AC, and age can be simple and reliable clinical tools for detecting underlying CKD in primary care. ECG changes in iPR, iRR, and QTc are common in patients with CKD, thus highlighting the potential role of machine learning in the early detection of cardiovascular disease.
- Prasad N, Patel MR. Infection-induced kidney diseases. Front Med (Lausanne). 2018;5:327. doi: 10.3389/fmed.2018.00327
- Glassock RJ, Alvarado A, Prosek J, et al. Staphylococcus-related glomerulonephritis and poststreptococcal glomerulonephritis: Why defining “post” is important in understanding and treating infection-related glomerulonephritis. Am J Kidney Dis. 2015;65(6):826-832. doi: 10.1053/j.ajkd.2015.01.023
- Tamaki N. Ischemia and inflammation on chronic kidney disease. J Nucl Cardiol. 2019;26(2):441-442. doi: 10.1007/s12350-017-0997-6
- Poulikakos D, Banerjee D, Malik M. Repolarisation descriptors and heart rate variability in hemodialysed patients. Physiol Res. 2015;64(4):487-493. doi: 10.33549/physiolres.932740
- Lu JL, Kalantar-Zadeh K, Ma JZ, Quarles LD, Kovesdy CP. Association of body mass index with outcomes in patients with CKD. J Am Soc Nephrol. 2014;25(9):2088-2096. doi: 10.1681/ASN.2013070754
- Skampardoni S, Poulikakos D, Malik M, Green D, Kalra PA. The potential of electrocardiography for cardiac risk prediction in chronic and end-stage kidney disease. Nephrol Dial Transplant. 2019;34(7):1089-1098. doi: 10.1093/ndt/gfy255
- Pannu AK, Dutta M, Singh HM. ECG triad of chronic kidney disease. QJM. 2019;112(6):465-466. doi: 10.1093/qjmed/hcy302
- Mulia EPB, Nugraha RA, A’yun MQ, et al. Electrocardiographic abnormalities among late-stage non-dialysis chronic kidney disease patients. J Basic Clin Physiol Pharmacol. 2020;32(3):155-162. doi: 10.1515/jbcpp-2020-0068
- Bignotto LH, Kallás ME, Djouki RJ, et al. Electrocardiographic findings in chronic hemodialysis patients. Braz J Nephrol. 2012;34(3):235-242. doi: 10.5935/0101-2800.20120004
- Zoccali C, Benedetto FA, Mallamaci F, et al. Left ventricular mass monitoring in the follow-up of dialysis patients: Prognostic value of left ventricular hypertrophy progression. Kidney Int. 2004;65(4):1492-1498. doi: 10.1111/j.1523-1755.2004.00530.x
- Bucharles SGE, Wallbach KKS, Moraes TP, Pecoits-Filho R. Hypertension in patients on dialysis: Diagnosis, mechanisms, and management. Braz J Nephrol. 2019;41(3):400-411. doi: 10.1590/2175-8239-jbn-2018-0155
- Di Lullo L, House A, Gorini A, Santoboni A, Russo D, Ronco C. Chronic kidney disease and cardiovascular complications. Heart Fail Rev. 2015;20(3):259-272. doi: 10.1007/s10741-014-9460-9
- Nagamine T, Watanabe T. QTc interval and sympathetic tone in burning mouth syndrome. Brain Heart. 2024;2(1):2016. doi: 10.36922/bh.2016
- Aftab MF. Intrinsic cardiac neurons as the consulate general of the brain in the heart: A review. Brain Heart. 2024;2(2):2901. doi: 10.36922/bh.2901
- Burton JO, Gray LJ, Webb DR, et al. Association of anthropometric obesity measures with chronic kidney disease risk in a non-diabetic patient population. Nephrol Dial Transplant. 2012;27(5):1860-1866. doi: 10.1093/ndt/gfr574
- Liu P, Quinn RR, Lam NN, et al. Accounting for age in the definition of chronic kidney disease. JAMA Intern Med. 2021;181(10):1359-1366. doi: 10.1001/jamainternmed.2021.4813
- Sankaran K. Data science principles for interpretable and explainable AI. J Data Sci. 2024;1-27. doi: 10.6339/24-JDS1150
- Sangeetha SKB, Mathivanan SK, Mallik S, Li A. Recognition predictive modeling using electroencephalogram. Brain Heart. 2024;2(2):2819. doi: 10.36922/bh.2819
- Chikov A, Egorov N, Medvedev D, et al. Determination of the athletes’ anaerobic threshold using machine learning methods. Biomed Signal Process Control. 2022;73:103414. doi: 10.1016/j.bspc.2021.103414
- Gaskin CJ, Happell B. On exploratory factor analysis: A review of recent evidence, an assessment of current practice, and recommendations for future use. Int J Nurs Stud. 2014;51(3):511-521. doi: 10.1016/j.ijnurstu.2013.10.005
- Nascimento DGB, Materko W, da Pureza Y, de Assis Mello MVF, Pena FSP. Electrocardiographic findings in patients with chronic kidney disease based on principal components analysis. Int J Dev Res. 2021;11(3):45450-45453. doi: 10.37118/ijdr.21360.03.2021
- Malachias MVB, Póvoa Júnior RMS, Nogueira AR, Souza D, Costa LS, Magalhães ME. 7th Brazilian guideline of arterial hypertension: Chapter 3 - Clinical and complementary assessment. Arq Bras Cardiol. 2016;107(3 Suppl 3):14-17. doi: 10.5935/abc.20160153
- Pastore CA, Pinho JA, Pinho C, et al. III guidelines of the Brazilian society of cardiology on the analysis and issuing of electrocardiographic reports. Arq Bras Cardiol. 2016;106(4 Suppl 1):1-23. doi: 10.5935/abc.20160054
- Dahlberg P, Diamant UB, Gilljam T, Rydberg A, Bergfeldt L. QT correction using Bazett’s formula remains preferable in long QT syndrome type 1 and 2. Ann Noninvasive Electrocardiol. 2021;26(1):e12804. doi: 10.1111/anec.12804
- Zhang Z, Castelló A. Principal components analysis in clinical studies. Ann Transl Med. 2017;5(17):351. doi: 10.21037/atm.2017.07.12
- Kaiser HF. The varimax criterion for analytic rotation in factor analysis. Psychometrika. 1958;23(3):187-200. doi: 10.1007/BF02289233
- Carpenter J, Bithell J. Bootstrap confidence intervals: When, which, what? A practical guide for medical statisticians. Stat Med. 2000;19(9):1141-1164. doi: 10.1002/(sici)1097-0258(20000515)19:9<1141:aid-sim479>3.0.co;2-f
- Materko W, Sadala MN, Fernandes DF, Da Pureza Y, Alberto ADA, Pena FSP. Evaluation on heart rate variability parameters in elderly with type 2 diabetes mellitus using principal component analysis. Gazz Med Ital Arch Sci Med. 2022;181:879-884. doi: 10.23736/S0393-3660.22.04782-9
- Zhang H, Chen Y, Li X. A note on exploratory item factor analysis by singular value decomposition. Psychometrika. 2020;85(2):358-372. doi: 10.1007/s11336-020-09704-7
- Mills KT, Stefanescu A, He J. The global epidemiology of hypertension. Nat Rev Nephrol. 2020;16(4):223-237. doi: 10.1038/s41581-019-0244-2
- Comini LO, de Oliveira LC, Borges LD, et al. Prevalence of chronic kidney disease in Brazilians with arterial hypertension and/or diabetes mellitus. J Clin Hypertens (Greenwich). 2020;22(9):1666-1673. doi: 10.1111/jch.13980
- Bird K, Chan G, Lu H, et al. Assessment of hypertension using clinical electrocardiogram features: A first-ever review. Front Med (Lausanne). 2020;7:583331. doi: 10.3389/fmed.2020.583331
- Amami K, Yamada S, Yoshihisa A, et al. Predictive impacts of chronic kidney disease and cardiac sympathetic nervous activity on lethal arrhythmic events in chronic heart failure. Ann Noninvasive Electrocardiol. 2022;27(1):e12900. doi: 10.1111/anec.12900
- Kula AJ, Katz R, Zelnick LR, et al. Association of circulating cardiac biomarkers with electrocardiographic abnormalities in chronic kidney disease. Nephrol Dial Transplant. 2021;36(12):2282-2289. doi: 10.1093/ndt/gfaa296
- Park S, Yum Y, Cha JJ, et al. Prevalence and clinical impact of electrocardiographic abnormalities in patients with chronic kidney disease. J Clin Med. 2022;11(18):5414. doi: 10.3390/jcm11185414
- Liu P, Han D, Sun X, et al. Prevalence and risk factors of acquired long QT syndrome in hospitalized patients with chronic kidney disease. J Investig Med. 2019;67(2):289-294. doi: 10.1136/jim-2018-000798
- Shafi S, Saleem M, Anjum R, Abdullah W, Shafi T. ECG abnormalities in patients with chronic kidney disease. J Ayub Med Coll Abbottabad. 2017;29(1):61-64.
- Kollu K, Altintepe L, Duran C, Topal M, Ecirli S. The assessment of P-wave dispersion and myocardial repolarization parameters in patients with chronic kidney disease. Ren Fail. 2018;40(1):1-7. doi: 10.1080/0886022X.2017.1419962
- Piccirillo G, Magrì D, Ogawa M, et al. Autonomic nervous system activity measured directly and QT interval variability in normal and pacing-induced tachycardia heart failure dogs. J Am Coll Cardiol. 2009;54(9):840-850. doi: 10.1016/j.jacc.2009.06.008
- Ajam F, Akoluk A, Alrefaee A, et al. Prevalence of abnormalities in electrocardiogram conduction in dialysis patients: A comparative study. J Bras Nefrol. 2020;42(4):448-453. doi: 10.1590/10.1590/2175-8239-JBN-2020-0018
- Pedersen LN, Carlson N, Nelveg-Kristensen KE, et al. Prognostic relevance of electrocardiographic abnormalities according to EGFR categories. Nephrol Dial Transplant. 2023;8(Supplement_1):i612-i613. doi: 10.1093/ndt/gfad063c_4771
- Nugraha RA, Khrisna BP, Putra T, et al. Baseline ECG changes could predict major MACE among hospitalized CKD patients. Kidney Int Rep. 2023;379(Supplement 1):S192.
- Edwards JK. Chronic kidney disease: ECGs help predict risk of death in CKD. Nat Rev Nephrol. 2015;11(9):505. doi: 10.1038/nrneph.2015.129
- Liu P, Wu J, Wang L, et al. The prevalence of fragmented QRS and its relationship with left ventricular systolic function in chronic kidney disease. J Int Med Res. 2020;48(4):300060519890792. doi: 10.1177/0300060519890792
- Materko W, Fernandes DF, Façanha CCR, et al. A machine learning approach to developing an accurate stratification of type 2 diabetes mellitus based on heart rate variability parameters using the k-means clustering technique in elderly women. Gazz Med Ital Arch Sci Med. 2024;183:44-50. doi: 10.23736/S0393-3660.23.05096-9
- Leiser F, Rank S, Schmidt-Kraepelin M, Thiebes S, Sunyaev A. Medical informed machine learning: A scoping review and future research directions. Artif Intell Med. 2023;145:102676. doi: 10.1016/j.artmed.2023.102676
- Materko W, Miranda SAM, Bezerra THL, de Oliveira Figueira CAM. Heart rate variability in soccer players and the application of unsupervised machine learning. Explor Cardiol. 2025;3:101241. doi: 10.37349/ec.2025.101241