Application of the concept of neural networks surgery in cerebrovascular disease treatment
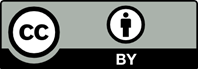
Based on advanced techniques, both the brain structural network and functional network can be reflected, giving rise to a new field: neural networks. Entering the 21st century, along with the extensive research on neural networks and the digital brain imaging field of neuromodulation, the neurosurgical field has entered into a novel stage: neural networks surgery. Neural networks surgery was developed to devote to protecting the cognitive function of patients with central nervous system diseases. By lucubrate, multiple new views of cerebrovascular disease have emerged. In this paper, we review the applications of this novel concept in treating cerebrovascular diseases, primarily through three aspects: disease mechanism, progression, and treatment strategy. Based on recent research, the development of a novel treatment system for cerebrovascular diseases might help clarify the course of these diseases, provide optimal treatment strategies, and protect the cognitive function of patients to the greatest extent.
Sakas DE, Panourias IG, Simpson BA, 2007, An introduction to neural networks surgery, a field of neuromodulation which is based on advances in neural networks science and digitised brain imaging. Acta Neurochir Suppl, 97: 3–13. https://doi.org/10.1007/978-3-211-33081-4_1
Hart MG, Ypma RJ, Romero-Garcia R, et al., 2016, Graph theory analysis of complex brain networks: New concepts in brain mapping applied to neurosurgery. J Neurosurg, 124: 1665–1678. https://doi.org/10.3171/2015.4.jns142683
Herbet G, Duffau H, 2020, Revisiting the functional anatomy of the human brain: Toward a meta-networking theory of cerebral functions. Physiol Rev, 100: 1181–1228. https://doi.org/10.1152/physrev.00033.2019
Sporns O, Tononi G, Kötter R, 2005, The human connectome: A structural description of the human brain. PLoS Comput Biol, 1: e42. https://doi.org/10.1371/journal.pcbi.0010042
Bullmore E, Sporns O, 2009, Complex brain networks: Graph theoretical analysis of structural and functional systems. Nat Rev Neurosci, 10: 186–198. https://doi.org/10.1038/nrn2575
Xu Y, He Y, Bi Y, 2017, A tri-network model of human semantic processing. Front Psychol, 8: 1538. https://doi.org/10.3389/fpsyg.2017.01538
Chen X, Liu M, Wu Z, et al., 2020, Topological abnormalities of functional brain network in early-stage Parkinson’s disease patients with mild cognitive impairment. Front Neurosci, 14: 616872. https://doi.org/10.3389/fnins.2020.616872
Thom T, Haase N, Rosamond W, et al., 2006, Heart disease and stroke statistics--2006 update: A report from the American heart association statistics committee and stroke statistics subcommittee. Circulation, 113: e85–e151. https://doi.org/10.1161/circulationaha.105.171600
Caplan LR, Searls DE, Hon FK, 2009, Cerebrovascular disease. Med Clin North Am, 93: 353–369.
Ma Q, Li R, Wang L, et al., 2021, Temporal trend and attributable risk factors of stroke burden in China, 1990- 2019: An analysis for the global burden of disease study 2019. Lancet Public Health, 6: e897–e906. https://doi.org/10.1016/S2468-2667(21)00228-0
O’Donnell MJ, Chin SL, Rangarajan S, et al., 2016, Global and regional effects of potentially modifiable risk factors associated with acute stroke in 32 countries (INTERSTROKE): A case-control study. Lancet, 388: 761–775. https://doi.org/10.1016/S0140-6736(16)30506-2
Chan A, Ho S, Poon WS, 2002, Neuropsychological sequelae of patients treated with microsurgical clipping or endovascular embolization for anterior communicating artery aneurysm. Eur Neurol, 47: 37–44. https://doi.org/10.1159/000047945
Li C, Zhu H, Zong X, et al., 2018, History, current situation, and future development of endoscopic neurosurgery in China. World Neurosurg, 110: 270–275. https://doi.org/10.1016/j.wneu.2017.11.103
Baldassarre A, Ramsey L, Rengachary J, et al., 2016, Dissociated functional connectivity profiles for motor and attention deficits in acute right-hemisphere stroke. Brain, 139: 2024–2038. https://doi.org/10.1093/brain/aww107
Baldassarre A, Ramsey L, Hacker CL, et al., 2014, Large-scale changes in network interactions as a physiological signature of spatial neglect. Brain, 137: 3267–3283. https://doi.org/10.1093/brain/awu297
Gordon EM, Laumann TO, Adeyemo B, et al., 2016. Generation and evaluation of a cortical area parcellation from resting-state correlations. Cereb Cortex, 26: 288–303. https://doi.org/10.1093/cercor/bhu239
Varsou O, Macleod MJ, Schwarzbauer C, 2014, Functional connectivity magnetic resonance imaging in stroke: An evidence-based clinical review. Int J Stroke, 9: 191–198. https://doi.org/10.1111/ijs.12033
Martinez-Ramirez S, Greenberg SM, Viswanathan A, 2014, Cerebral microbleeds: Overview and implications in cognitive impairment. Alzheimers Res Ther, 6: 33. https://doi.org/10.1186/alzrt263
Charidimou A, Krishnan A, Werring DJ, et al., 2013, Cerebral microbleeds: A guide to detection and clinical relevance in different disease settings. Neuroradiology, 55: 655–674. https://doi.org/10.1007/s00234-013-1175-4
Al-Masni MA, Kim WR, Kim EY, et al., 2020, A two cascaded network integrating regional-based YOLO and 3D-CNN for cerebral microbleeds detection. Annu Int Conf IEEE Eng Med Biol Soc, 2020: 1055–1058. https://doi.org/10.1109/EMBC44109.2020.9176073
Wadi LC, Grigoryan MM, Kim RC, et al., 2020, Mechanisms of cerebral microbleeds. J Neuropathol Exp Neurol, 42: 1093–1099. https://doi.org/10.1093/jnen/nlaa082
Crouzet C, Jeong G, Chae RH, et al., 2021, Spectroscopic and deep learning–based approaches to identify and quantify cerebral microhemorrhages. Sci Rep, 11: 10725. https://doi.org/10.1038/s41598-021-88236-1
Jayaraman MV, Mayo-Smith WW, Tung GA, et al., 2004, Detection of intracranial aneurysms: Multi-detector row CT angiography compared with DSA. Radiology, 230: 510–518. https://doi.org/10.1148/radiol.2302021465
Brown RD Jr., Broderick JP, 2014, Unruptured intracranial aneurysms: Epidemiology, natural history, management options, and familial screening. Lancet Neurol, 13: 393–404. https://doi.org/1016/S1474-4422(14)70015-8
Nemoto M, Hayashi N, Hanaoka S, et al., 2017, Feasibility study of a generalized framework for developing computer-aided detection systems-a new paradigm. J Digit Imaging, 30: 629–639. https://doi.org/0.1007/s10278-017-9968-3
Ueda D, Yamamoto A, Nishimori M, et al., 2019, Deep learning for MR angiography: Automated detection of cerebral aneurysms. Radiology, 290: 187–194. https://doi.org/10.1148/radiol.2018180901
Westerlaan HE, van Dijk JM, der Weide MC, et al., 2011, Intracranial aneurysms in patients with subarachnoid hemorrhage: CT angiography as a primary examination tool for diagnosis--systematic review and meta-analysis. Radiology, 258: 134–145. https://doi.org/10.1148/radiol.10092373
Bo ZH, Qiao H, Tian C, et al., 2021, Toward human intervention-free clinical diagnosis of intracranial aneurysm via deep neural network. Patterns (N Y), 2: 100197. https://doi.org/10.1016/j.patter.2020.100197
Chen G, Wei X, Lei H, et al., 2020, Automated computer-assisted detection system for cerebral aneurysms in time-of-flight magnetic resonance angiography using fully convolutional network. Biomed Eng Online, 19: 38. https://doi.org/10.1186/s12938-020-00770-7
Findlay JM, Nisar J, Darsaut T, 2016, Cerebral vasospasm: A review. Can J Neurol Sci, 43: 15–32. https://doi.org/10.1017/cjn.2015.288
Dumont TM, 2016, Prospective assessment of a symptomatic cerebral vasospasm predictive neural network model. World Neurosurg, 94: 126–130. https://doi.org/10.1016/j.wneu.2016.06.110
Boulouis G, Charidimou A, Greenberg SM, 2016, Sporadic cerebral amyloid angiopathy: Pathophysiology, neuroimaging features, and clinical implications. Semin Neurol, 36: 233–243. https://doi.org/10.1055/s-0036-1581993
Charidimou A, Gang Q, Werring DJ, 2012, Sporadic cerebral amyloid angiopathy revisited: Recent insights into pathophysiology and clinical spectrum. J Neurol Neurosurg Psychiatry, 83, 124–137. https://doi.org/10.1136/jnnp-2011-301308
Arvanitakis Z, Leurgans SE, Wang Z, et al., 2011, Cerebral amyloid angiopathy pathology and cognitive domains in older persons. Ann Neurol, 69: 320–327. https://doi.org/10.1002/ana.22112
Boyle PA, Yu L, Nag S, et al., 2015, Cerebral amyloid angiopathy and cognitive outcomes in community-based older persons. Neurology, 85: 1930–1936. https://doi.org/10.1212/wnl.0000000000002175
Reijmer YD, Fotiadis P, Martinez-Ramirez S, et al., 2015, Structural network alterations and neurological dysfunction in cerebral amyloid angiopathy. Brain, 138: 179–188. https://doi.org/10.1093/brain/awu316
Drenth N, van der Grond J, Rombouts SA, et al., 2021, Cerebral amyloid angiopathy is associated with decreased functional brain connectivity. Neuroimage Clin, 29: 102546. https://doi.org/10.1016/j.nicl.2020.102546
Yonas H, Smith HA, Durham SR, et al., 1993, Increased stroke risk predicted by compromised cerebral blood flow reactivity. J Neurosurg, 79: 483–489. https://doi.org/10.3171/jns.1993.79.4.0483
Lin CJ, Tu PC, Chern CM, et al., 2014, Connectivity features for identifying cognitive impairment in presymptomatic carotid stenosis. PLoS One, 9: e85441. https://doi.org/10.1371/journal.pone.0085441
Chang TY, Huang KL, Ho MY, et al., 2016, Graph theoretical analysis of functional networks and its relationship to cognitive decline in patients with carotid stenosis. J Cereb Blood Flow Metab, 36: 808–818. https://doi.org/10.1177/0271678X15608390
Calabrò RS, Bramanti P, Baglieri A, et al., 2015, Functional cortical and cerebellar reorganization in a case of moyamoya disease. Innov Clin Neurosci, 12: 24–28.
Jefferson AL, Glosser G, Detre JA, et al., 2006, Neuropsychological and perfusion MR imaging correlates of revascularization in a case of moyamoya syndrome. AJNR Am J Neuroradiol, 27: 98–100.
Aben HP, Biessels GJ, Weaver NA, et al., 2019, Extent to which network hubs are affected by ischemic stroke predicts cognitive recovery. Stroke, 50: 2768–2774. https://doi.org/10.1161/STROKEAHA.119.025637
Gong G, He Y, Concha L, et al., 2009, Mapping anatomical connectivity patterns of human cerebral cortex using in vivo diffusion tensor imaging tractography. Cereb Cortex, 19: 524–536. https://doi.org/10.1093/cercor/bhn102
Hagmann P, Cammoun L, Gigandet X, et al., 2008, Mapping the structural core of human cerebral cortex. PLoS Biol, 6: e159. https://doi.org/10.1371/journal.pbio.0060159
Tomasi D,Volkow ND, 2010, Functional connectivity density mapping. Proc Natl Acad Sci U S A, 107: 9885–9890. https://doi.org/10.1073/pnas.1001414107
Raichle ME, Macleod AM, Snyder AZ, et al., 2001, A default mode of brain function. Proc Natl Acad Sci U S A, 98: 676–682. https://doi.org/10.1073/pnas.98.2.676
Power JD, Schlaggar BL, Lessov-Schlaggar CN, et al., 2013, Evidence for hubs in human functional brain networks. Neuron, 79: 798–813. https://doi.org/10.1016/j.neuron.2013.07.035
Várkuti B, Cavusoglu M, Kullik A, et al., 2011, Quantifying the link between anatomical connectivity, gray matter volume and regional cerebral blood flow: An integrative MRI study. PLoS One, 6: e14801. https://doi.org/10.1371/journal.pone.0014801
Liang X, Zou Q, He Y, et al., 2013, Coupling of functional connectivity and regional cerebral blood flow reveals a physiological basis for network hubs of the human brain. Proc Natl Acad Sci U S A, 110: 1929–1934. https://doi.org/10.1073/pnas.1214900110
Yuan B, Fang Y, Han Z, et al., 2017, Brain hubs in lesion models: Predicting functional network topology with lesion patterns in patients. Sci Rep, 7: 17908. https://doi.org/10.1038/s41598-017-17886-x
Buckner RL, Sepulcre J, Talukdar T, et al., 2009, Cortical hubs revealed by intrinsic functional connectivity: Mapping, assessment of stability, and relation to Alzheimer’s disease. J Neurosci, 29: 1860–1873. https://doi.org/10.1523/JNEUROSCI.5062-08.2009
Hilgetag CC, Burns GA, O’Neill MA, et al., 2000, Anatomical connectivity defines the organization of clusters of cortical areas in the macaque monkey and the cat. Philos Trans R Soc Lond B Biol Sci, 355: 91–110. https://doi.org/10.1098/rstb.2000.0551
Hilgetag CC, O’Neill MA, Young MP, 2000, Hierarchical organization of macaque and cat cortical sensory systems explored with a novel network processor. Philos Trans R Soc Lond B Biol Sci, 355: 71–89. https://doi.org/10.1098/rstb.2000.0550
Sporns O, Zwi JD, 2004, The small world of the cerebral cortex. Neuroinformatics, 2: 145–162. https://doi.org/10.1385/NI:2:2:145
Sporns O, Kötter R, 2004, Motifs in brain networks. PLoS Biol, 2: e369. https://doi.org/10.1371/journal.pbio.0020369
Li Y, Liu Y, Li J, et al., 2009, Brain anatomical network and intelligence. PLoS Comput Biol, 5: e1000395. https://doi.org/10.1371/journal.pcbi.1000395
Lee S, Kim D, Youn H, et al., 2021, Brain network analysis reveals that amyloidopathy affects comorbid cognitive dysfunction in older adults with depression. Sci Rep, 11: 4299. https://doi.org/10.1038/s41598-021-83739-3
Cuadrado-Godia E, Dwivedi P, Sharma S, et al., 2018, Cerebral small vessel disease: A review focusing on pathophysiology, biomarkers, and machine learning strategies. J Stroke, 20: 302–320. https://doi.org/10.5853/jos.2017.02922
Bosnell RA, Kincses T, Stagg CJ, et al., 2011, Motor practice promotes increased activity in brain regions structurally disconnected after subcortical stroke. Neurorehabil Neural Repair, 25: 607–616. https://doi.org/10.1177/1545968311405675
Carrera E, Tononi G, 2014, Diaschisis: Past, present, future. Brain, 137l: 2408–2422. https://doi.org/10.1093/brain/awu101
Boukrina O, Barrett AM, 2017, Disruption of the ascending arousal system and cortical attention networks in post-stroke delirium and spatial neglect. Neurosci Biobehav Rev, 83: 1–10. https://doi.org/10.1016/j.neubiorev.2017.09.024
Bonilha L, Hillis AE, Wilmskoetter J, et al., 2019, Neural structures supporting spontaneous and assisted (entrained) speech fluency. Brain, 142: 3951–3962. https://doi.org/10.1093/brain/awz309
Altinbas A, Van Zandvoort MJ, van den Berg E, et al., 2011, Cognition after carotid endarterectomy or stenting: A randomized comparison. Neurology, 77: 1084–1090. https://doi.org/10.1212/wnl.0b013e31822e55b9
Nauta IM, Kulik SD, Breedt LC, et al., 2021, Functional brain network organization measured with magnetoencephalography predicts cognitive decline in multiple sclerosis. Mult Scler, 27: 1727–1737. https://doi.org/10.1177/1352458520977160
Zhang X, Liu J, Chen Y, et al., 2021, Brain network construction and analysis for patients with mild cognitive impairment and Alzheimer’s disease based on a highly-available nodes approach. Brain Behav, 11: e02027. https://doi.org/10.1002/brb3.2027
Jiao Y, Lin F, Wu J, et al., 2016, Lesion-to-eloquent fiber distance is a crucial risk factor in presurgical evaluation of arteriovenous malformations in the temporo–occipital junction. World Neurosurg, 93: 355–364. https://doi.org/10.1016/j.wneu.2016.06.059
Kazumata K, Tha KK, Narita H, et al., 2015, Chronic ischemia alters brain microstructural integrity and cognitive performance in adult moyamoya disease. Stroke, 46: 354–360. https://doi.org/10.1161/strokeaha.114.007407
Lei Y, Li Y, Ni W, et al., 2014, Spontaneous brain activity in adult patients with moyamoya disease: A resting-state fMRI study. Brain Res, 1546: 27–33. https://doi.org/10.1016/j.brainres.2013.12.022
Lei Y, Song B, Chen L, et al., 2020, Reconfigured functional network dynamics in adult moyamoya disease: A resting-state fMRI study. Brain Imaging Behav, 14: 715–727. https://doi.org/10.1007/s11682-018-0009-8
He S, Liu Z, Xu Z, et al., 2020, Brain functional network in chronic asymptomatic carotid artery stenosis and occlusion: Changes and compensation. Neural Plast, 2020: 9345602. https://doi.org/10.1155/2020/9345602