Recognition predictive modeling using electroencephalogram
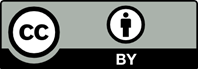
A machine learning model that operates on raw electroencephalogram (EEG) signals is essential for accurately discerning the user’s current thoughts. Given the difficulty of categorizing EEG signals for use in brain-computer interface (BCI) programs, we adopted a systematic approach in this study to select an optimal predictive model. To enhance the effectiveness of our systematic approach, we extracted features such as band powers, averages, and root-mean-squared values. K-nearest neighbor (KNN), principal component analysis, and dual-layer neural networks were employed to evaluate and validate the effectiveness of the extracted features. The BCI IV competition-I dataset was utilized for analysis and validation. KNN achieved an average classification success rate of 98.02% compared to other methods. Furthermore, our research extends the application of this approach using it to create, test, and evaluate human driving behavior as a case study.
- Qi G, Zhao S, Ceder AA, Guan W, Yan X. Wielding and evaluating the removal composition of common artefacts in EEG signals for driving behaviour analysis. Accid Anal Prev. 2021;159:106223. doi: 10.1016/j.aap.2021.106223
- Zero E, Bersani C, Sacile R. EEG based BCI system for driver’s arm movements identification. In: Automation, Robotics and Communications for Industry 4.0. Vol. 77. France: International Frequency Sensor Association; 2021.
- Cao Z, Chuang CH, King JK, Lin CT. Multi-channel EEG recordings during a sustained-attention driving task. Sci Data. 2019;6(1):19. doi: 10.1038/s41597-019-0027-4
- Zhang X, Li J, Liu Y, et al. Design of a fatigue detection system for high-speed trains based on driver vigilance using a wireless wearable EEG. Sensors (Basel). 2017;17(3):486. doi: 10.3390/s17030486
- Nader M, Jacyna-Gołda I, Nader S, Nehring K. Using BCI and EEG to process and analyze driver’s brain activity signals during VR simulation. Arch Transp. 2021;60:137-153. doi: 10.5604/01.3001.0015.6305
- Zhou X, Yao D, Zhu, M, et al. Vigilance detection method for high‐speed rail using wireless wearable EEG collection technology based on low‐rank matrix decomposition. IET Intell Transp Syst. 2018;12(8):819-825. doi: 10.1049/iet-its.2017.0239
- He S, Chen L, Yue M. Reliability analysis of driving behaviour in road traffic system considering synchronization of neural activity. NeuroQuantology. 2018;16(4):62-68. doi: 10.14704/nq.2018.16.4.1209
- Lawhern VJ, Solon AJ, Waytowich NR, Gordon SM, Hung CP, Lance BJ. EEGNet: A compact convolutional neural network for EEG-based brain-computer interfaces. J Neural Eng. 2018;15:056013. doi: 10.1088/1741-2552/aace8c
- Doudou M, Bouabdallah A, Berge-Cherfaoui V. Driver drowsiness measurement technologies: Current research, market solutions, and challenges. Int J Intell Transp Syst Res. 2020;18(2):297-319. doi: 10.1007/s13177-019-00199-w
- Haghani M, Bliemer MC, Farooq B, et al. Applications of brain imaging methods in driving behaviour research. Accid Anal Prev. 2021;154:106093. doi: 10.1016/j.aap.2021.106093
- Murthy GN, Khan ZA. Cognitive attention behaviour detection systems using Electroencephalograph (EEG) signals. Res J Pharm Technol. 2014;7(2):238-247.
- Pal D, Palit S, Dey A. Brain computer interface: A review. In: Computational Advancement in Communication, Circuits and Systems. Cham: Springer; 2022. p. 25-35. doi: 10.1007/978-3-319-10978-7_1
- Zero E, Bersani C, Zero L, Sacile R. Towards real-time monitoring of fear in driving sessions. IFAC-PapersOnLine. 2019;52(19):299-304. doi: 10.1016/j.ifacol.2019.12.068
- Aricò P, Borghini G, Di Flumeri G, Sciaraffa N, Babiloni F. Passive BCI beyond the lab: Current trends and future directions. Physiol Meas. 2018;39(8):08TR02.doi: 10.1088/1361-6579/aad57e
- Hasan MJ, Shon D, Im K, Choi HK, Yoo DS, Kim JM. Sleep state classification using power spectral density and residual neural network with multichannel EEG signals. Appl Sci. 2020;10:7639. doi: 10.3390/app10217639
- Brouwer AM, Snelting A, Jaswa M, Flascher O, Krol L, Zander T. Physiological Effects of Adaptive Cruise Control Behaviour in Real Driving. In: Proceedings of the 2017 ACM Workshop on an Application-oriented Approach to BCI out of the Laboratory. 2017. p. 15-19. doi: 10.1145/3038439.3038441
- Karuppusamy NS, Kang BY. Multimodal system to detect driver fatigue using EEG, gyroscope, and image processing. IEEE Access. 2020;8:129645-129667. doi: 10.1109/Access.2020.3009226
- Sangeetha SKB, Kumar MS, Deeba K, Rajadurai H, Maheshwari V, Dalu GT. An empirical analysis of an optimized pretrained deep learning model for COVID-19 diagnosis. Comput Math Methods Med. 2022;2022:9771212. doi: 10.1155/2022/9771212
- Khalaf OI, Ogudo KA, Sangeetha SKB. Design of Graph-based layered learning-driven model for anomaly detection in distributed cloud IoT network. Mob Inf Syst. 2022;2022:6750757. doi: 10.1155/2022/6750757
- Kanthavel D, Sangeetha SKB, Keerthana KP. An empirical study of vehicle to infrastructure communications-an intense learning of smart infrastructure for safety and mobility. Int J Intell Netw. 2021;2:77-82. doi: 10.1016/j.ijin.2021.06.003
- Aggarwal S, Chugh N. Review of machine learning techniques for EEG based brain computer interface. Arch Comput Methods Eng. 2022;29:3001-3020. doi: 10.1007/s11831-021-09684-6
- Ahn M, Jun SC, Yeom HG, Cho H. Editorial: Deep learning in brain-computer interface. Front Hum Neurosci. 2022;16:927567. doi: 10.3389/fnhum.2022.927567
- Zhu H, Forenzo D, He B. On the deep learning models for EEG-based brain-computer interface using motor imagery. IEEE Trans Neural Syst Rehabil Eng. 2022;30:2283-2291. doi: 10.1109/TNSRE.2022.3198041
- Immanuel RR, Sangeetha SKB. Analysis of EEG Signal with Feature and Feature Extraction Techniques for Emotion Recognition Using Deep Learning Techniques. In: Proceedings of International Conference on Computational Intelligence and Data Engineering. Singapore: Springer Nature Singapore; 2022. p. 141-154. doi: 10.1007/978-981-99-0609-3_10